ABSTRACT
The purpose of this study was to identify the underlying socio-psychological factors that influence pond and cage farmers’ intentions to adopt Black Soldier Fly Larvae (BSFL) in Kenya. Based on the Technology Acceptance Model (TAM), this study empirically investigated the relationship between TAM constructs, namely Perceived Ease of Use (PEOU), Perceived Usefulness (PU), Attitude towards Use (ATT), and Behavioral Intention (BI) to use BSFL. The study used a cross-sectional survey design to collect primary data from 211 randomly selected cage operators (98) and pond farmers (113) in Kenya’s Siaya, Kisumu, and Homabay Counties. A structural equation model was employed to examine hypothesized paths in the uptake of BSFL meal with the aid of SmartPLS 3. The inner model path coefficients suggested that ATT had the strongest effect on farmers’ intentions to adopt BSFL (0.411). Further, PU had a greater relative influence on intention to adopt BSFL than PEOU based on the model path coefficients of 0.319 and 0.178 respectively. This indicates that the more respondents believe BSFL is useful in their fish farm's production conditions, the more likely they are to adopt BSFL. Consequently, these findings have direct implications for policy development and the potential use of BSFL in aquaculture.
Introduction
Aquaculture continues to grow at a 5.8% annual rate in most regions of the world, owing to the industry’s rapid expansion and intensification (FAO Citation2020). Aquaculture is thus the world’s fastest-growing food-producing industry, driving local economies and employing many people in the Sub-Saharan Africa (SSA) region, including Kenya (Hasimuna et al. Citation2020). Aquaculture uptake has recently improved significantly in most African countries, though its full potential is still far from being realized. Despite the significant potential for aquaculture development, the majority of SSA countries (excluding Nigeria) continue to report low aquaculture production (Tran et al. Citation2019; Mmanda et al. Citation2020). In Kenya’s inland areas, the main culture species is Nile tilapia (Oreochromis niloticus), though African catfish (Clarias gariepinus) is also produced to some extent (Opiyo et al. Citation2018). Tilapia is the preferred culture species due to its rapid growth, disease resistance, ability to withstand low dissolved oxygen (DO) levels, and ease of producing fingerlings in captivity (Githukia et al. Citation2015; Fitzsimmons Citation2016). The domestic market for Nile tilapia is also quite promising (Quagrainie et al. Citation2010).
The sustainable intensification of aquaculture output necessitates an increase in the supply and development of inputs, primarily feedstuff, as well as their formulations and optimization (Alhazzaa et al. Citation2019). The main bottlenecks in boosting aquaculture in Kenya have been identified as a lack of quality and affordable aquafeeds due to the high cost of fish feeds (Amankwah et al. Citation2018). Depending on the farming system, feed accounts for at least 40–80% of the total variable cost (TVC) of production (Chia et al. Citation2020). The availability and accessibility of standardized cost-effective fish feeds is integral to the sustainability of a profitable aquaculture enterprise in Kenya. Because of the high protein requirements of fish, fish meal (FM) and soya bean meal (SBM) have become the primary protein sources (Nogales-Mérida et al. Citation2018). However, due to an increase in global protein demand, the costs of FM and SBM have dramatically grown in the recent decade compared to the other protein sources utilized in feed (Van Huis et al. Citation2013; Muin et al. Citation2017; Nogales-Mérida et al. Citation2018). FM is also utilized in animal feeds, which pushes up demand and market pricing. Significant advancements in feed efficiency have been made in the last two decades as a result of a better understanding of enhanced feed management and the use of a broader choice of alternative components to build balanced digestible diets. Feed management is particularly important in the context of encouraging the utilization of locally sourced non-conventional feed protein ingredients to improve the long-term sustainability of tilapia culture, both with respect to resource utilization and minimization of feeding costs (Mmanda et al. Citation2020). Arru et al. (Citation2019) suggested that alternative protein sources should be readily available and accessible, have amino acids comparable to or better than fishmeal, and have palatability comparable to fishmeal. In this context, there has been an increase in interest in using insect meals as a potential feed alternative in fish farming (Riddick Citation2013; Amza and Tamiru Citation2017; Magalhães et al. Citation2017; Nyakeri et al. Citation2017; Ibitoye and Oyetunji Citation2019; Chia et al. Citation2020). Due to its high energy conversion efficiency, good nutritional content, and benefits in fish development and health improvement, insect protein continues to gain significant scientific interest (Mousavi et al. Citation2020).
For the animal feed industry, the Black Soldier Fly (BSF) is the most commonly farmed insect. It’s also commonly utilized in organic waste treatment as a bio-convertor. At the same time, it can be used as an alternate protein source in livestock and aquaculture (Lalander et al. Citation2015; Vogel et al. Citation2018). In the last ten years, there has been an increase in research and industrial-scale production of Black Soldier Fly Larvae (BSFL) as feed ingredients (FAO Citation2020; Wang and Shelomi Citation2017). The BSFL has a high protein content (40% dry weight (DW)) and a well-balanced essential amino acid profile (AA) (Henry et al. Citation2015; Liland et al. Citation2017; Wang and Shelomi Citation2017).
Some fish feeding trials have effectively established that replacing FM with BSFL meal in aquafeeds has no negative effects on growth or performance (Cummins et al. Citation2017; Renna et al. Citation2017; Belghit et al. Citation2018; Dumas et al. Citation2018; Elia et al. Citation2018).
The use of BSFL as a novel alternative element in aquaculture feeds is an example of innovation.
While the nutritional qualities of these ingredients are vital for fish growth, their perceived utility and possible acceptability by fish farmers are also important, and must be elicited to ensure that these new aquafeeds are used and adopted long-term as part of improved feeding practices (Brugere et al. Citation2021). Farmer decision-making research have generally relied on economic models and socioeconomic considerations. As a result, these two approaches have been employed in a variety of research to better understand how farmers make decisions (Yazdanpanah et al. Citation2022). Previous studies suggest that human decisions do not always seek to maximize profit, with some behaviors based on individual and intrinsic motivation (Zeweld et al. Citation2017; Despotović et al. Citation2019). As a result, greater insights into individual decisions can be gained through cognitive and social-psychological factors. Previous research has also used a quantitative socio-psychological model to understand the factors that influence agricultural adoption decisions. Michels et al. (Citation2021), for example, investigated the use of drones in German agriculture. Lalani et al. (Citation2016) investigated smallholder farmers’ motivations for using conservation agriculture, Bijttebier et al. (Citation2018) investigated farmer adoption of non-inversion tillage as a soil conservation measure, and Dessart et al. (Citation2019) reviewed behavioral factors influencing the adoption of sustainable farming practices. Sok et al. (Citation2021) went on to conduct a systematic review on the use of socio-psychological models among farmers. However, these cognitive and social-psychological factors, which have a high potential for predicting people’s decision making, are typically overlooked and, as a result, are infrequently used in contemporary innovation adoption studies in aquaculture, particularly for BSFL (Zeweld et al. Citation2018). Furthermore, research on the acceptance of BSFL meal is new in Kenya, and few studies exist that investigate and confirm the determinants of BSFL use among fish farmers from a structured perspective. Additionally, drivers of farmers’ intentions to use BSFL meal are not well documented. This study investigates how socio-psychological factors influence fish farmers’ decisions to implement BSFL from a micro-level using a novel perspective.
This study seeks to add to the literature by examining factors influencing the adoption of BSFL meal while taking into account socio-psychological issues. Specifically, it aims to establish the intentions of fish farmers towards adopting BSFL and its various determinants using Structural Equation Model (SEM) so as to provide research recommendations for encouraging the adoption and upscaling of BSFL in Kenya and beyond. Thus, the study intends to validate if the TAM framework can also contribute to the understanding fish farmers’ decision making with respect to the adoption of BSFL.
Theoretical framework
This study's conceptual model is based on the Technology Acceptance Model (TAM) theory. TAM is a well-known and significant modification to Fishbein and Ajzen’s theories of planned behavior and theory of reason action (Ajzen Citation1991; Fishbein and Ajzen Citation1977). Davis (Citation1989) developed the TAM, which is based on rational choice theory and provides useful guidelines for identifying the variables influencing users’ acceptance of innovations (Castiblanco Jimenez et al. Citation2021). Because of its high predictive power, the TAM is the most widely used model for the intention to adopt a technology. According to TAM, perceived usefulness (PU) and perceived ease of use (PEOU) determine attitudes toward a novel product or technology and subsequent behavior when using the technology, as shown in Figure . Davis (Citation1989) defines perceived ease of use as a person's belief that using a technology requires no effort. On the other hand, perceived usefulness is an individual’s belief that a technology improves job performance, as defined by Michels et al. (Citation2021). Both PU and PEOU, according to the TAM framework, influence an individual’s intention to use a technology as well as its actual adoption. Furthermore, a person who perceives a technology to be simple also perceives the technology to be more useful (Davis Citation1989). Ulhaq et al. (Citation2022) also note that PEOU can operate via PU, indicating that a technology’s perceived ease of use can make it more useful.
Figure 1. Research model illustrating the hypothetical influence of technology acceptance factors on intentions to use BSFL in fish farming.
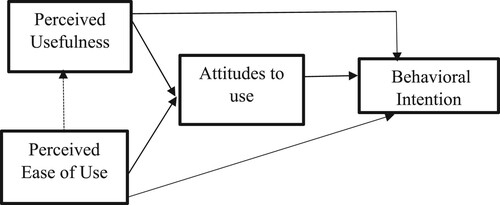
Notably, according to the TAM framework, a fish farmer who perceives using BSFL as simple will be more likely to use it in fish production. Furthermore, if the farmer believes that using BSFL will help increase fish production output, he or she is more likely to adopt it. Furthermore, if a farmer believes that using BSFL is simple, he or she considers this novel feed to be more useful, which is consistent with the previous findings of Michels et al. (Citation2021).
Attitude is defined as an individual’s negative or positive feelings toward the behavior in question (Fishbein and Ajzen Citation1975). TAM considers attitude as a mediator in the model, according to Kurkinen (Citation2012). On the other hand, perceived ease of use and perceived usefulness are important predictors of attitude (Ducey and Coovert Citation2016; Verma et al. Citation2018). Accordingly, in the case of BSFL, if a farmer believes that BSFL are technically easy to use and using BSFL meal as useful, the farmer will form a favorable attitude towards BSFL meal.
In the TAM model, intention indicates how much a person is trying to do a specific behavior. Honkanen & Young (Citation2015) noted that intention captures people’s motivation to perform the behavior and their likelihood of following through it. A stronger intention for a behavior, in general, indicates that the behavior is more likely to be practiced (Ajzen Citation1991). Behavior (actual use) is always after the behavioral intention and is connected to it. Chang et al. (Citation2016) found that farmers’ desirable attitudes toward limiting water use could predict the adoption of water-saving policies. Previous research by Aren et al. (Citation2013) found that perceived ease of use could positively affect intentions.
Based on the research model in Figure , seven hypotheses were formulated;
H1: Perceived ease of use has a positive and significant influence on farmer attitude to use BSFL
H2: Perceived ease of use has a positive and significant influence on farmer intentions to use BSFL
H3: Perceived usefulness has a positive and significant influence on farmer attitude to use BSFL
H4: Perceived usefulness has a positive and significant influence on farmer intentions to use BSFL
H5: Attitude has a positive and significant influence on farmer intentions to use BSFL
H6: Perceived ease of use has an indirect positive and significant influence on farmer intentions to use BSFL
H7: Perceived usefulness has an indirect positive and significant influence on farmer intentions to use BSFL
Materials and methods
Study area
The study was carried out in three riparian counties of Lake Victoria within the borders of Kenya namely Siaya, Kisumu and Homabay Counties. The lake is the largest tropical and the second largest freshwater lake in the world with a surface area of 68,000 km2 (Aura et al. Citation2018). The study sites were selected based on existing geographical zones, high water resource potential, predominance of fish farming activities, adoption of pond and cage culture practices by farmers and presence of large number of active Nile tilapia fish farmers (Opiyo et al. Citation2018; Orina et al. Citation2018).
Research design
A cross-sectional survey design was used in this study to collect primary data on fish farmers’ intentions to use BSFL. This study’s sampling frame was defined as all active fish pond and cage farmers engaged in Nile tilapia culture in the study area. The respondents were chosen using a two-stage sampling technique. The first stage involved the purposive selection of the most tilapia-producing areas in the riparian counties of Lake Victoria Region, Kenya. Siaya, Kisumu, and Homabay counties were purposively selected in this study because they have the highest number of Nile tilapia farmers both in ponds and cage culture farming (Opiyo et al. Citation2018). The second stage involved selection of pond and cage farmers. In a selection of pond farmers for the study, systematic random sampling was applied. This involved using farmers’ lists provided by the Sub-County Fisheries officers in each county. The names of the fish ponds farmers were chosen at an interval in which all the three counties namely Siaya, Kisumu, and Homabay were considered. Established fish pond farmers who have spent more than two years in fish pond farming were considered because they were perceived to have more information on the role of feeds in fish farming. This was also used to reduce the population heterogeneity and increase the efficiency of the estimates. Systematic sampling was also used to select cage farmers in Siaya, Kisumu, and Homabay Counties based on the cage locations along Lake Victoria Beaches. A total of 211 fish farmers were randomly selected from a list of farmers provided by the Sub-County Fisheries Officers in each of the three selected counties. Using the 2019 Kenya National Bureau of Statistics (KNBS) data on the fish farmer’s population of the three counties of interest (clusters) as reported by the Kenya Population and Housing Census, a proportionate to population size (PPS) of respondents for each county was computed to arrive at 211 respondents who were interviewed as in Table .
Table 1. Distribution of respondents by farmer category per county.
A structured questionnaire was designed based on the TAM and included other socio-demographic variables. The face-to-face survey was conducted by trained enumerators between December 2020 and April 2021. The survey tool comprised of two main sections; the first section containing questions on farm and farmer characteristics. Other information collected were feeding practices, cost of feeds, type, and sources of feeds used on the farm and other issues relating to fish farming. In the second section, participants were asked to evaluate a number of statements (constructs derived from literature review) on a five-point Likert scale which ranged from 1 = strongly disagree to 5 = strongly agree, indicating the degree to which they agreed with the set of statements. The statements were designed to reveal their intentions, attitude, perceived usefulness and perceived ease of use of BSFL (Table ). The research constructs were developed solely on already validated measures. All scale items were rearticulated to relate exactly to the context of the current study’s requirement. A minimum of three items were used per construct so as to guarantee suitable reliability in line with the recommendation by Nunnally (Citation1978). The statements serve as the indicators to estimate the latent variables.
The questionnaire was pre-tested in order to eliminate any potential problems in using it such as time management, complexity, suitability, and appropriateness. Feedback from the pre-test was used to refine the questionnaire. The final questionnaire was loaded into Kobo Collect platform, which was used to collect data using smartphones. Informed consent was sought from the respondents before conducting the face-to-face interviews. All interviews were conducted in line with the World Health Organization (WHO) guidelines on COVID-19 prevention.
Data analysis
Data were received on an aggregate server in real time, where regular quality checks were done to ensure that the data collected met the required standards. On completion of the field survey, the final datasets were downloaded from the server as CSV files and exported to SPSS version 25 software for analysis. Descriptive analysis was done by calculating frequencies, means, and standard errors. Factors listed as weak indicators were excluded from subsequent analyses based on significance of bivariate and multivariate relationships. Statistical significance was considered significant at 5% level.
Analytical framework
The inter-relationship between variables in the conceptual model was assessed using a partial least square structural equation modeling (PLS-SEM) technique. TAM constructs are latent, and thus cannot be observed or measured directly. Hence, a set of measures were derived from a list of questions to act as indicators for the underlying latent variable. As a result, the structural equation model includes an outer sub-model that specifies the relationships between the latent variables and their observed indicators. An inner sub-model is then added, which evaluates the relationship between the dependent and independent latent variables, as well as their respective path coefficients (Mutyasira et al. Citation2018). Structural equation modeling (SEM) is a method that combines factor analysis and multiple regression analysis (Chin Citation1998). SEM enables the simultaneous estimation of cause–effect relationships between multiple dependent and independent latent variables, as well as the testing of theoretical relationships. Covariance-based SEM (CB-SEM) and partial least square SEM (PLS-SEM) are the two types of SEM (Haenlein and Kaplan Citation2004). According to Hair et al. (Citation2017), Partial Least Squares SEM (PLS-SEM) was chosen to test the hypotheses in this study for two reasons: (1) the research objective is exploratory theory based on total variance in the area of agricultural technology adoption, and the goal of this analysis is prediction, and (2) the research objective is to use latent variable scores in subsequent analyses (Ringle et al. Citation2014). PLS-SEM was a good fit for data analysis in this study because the data was not normally distributed and the sample size was small. Compared to covariance-based SEM, PLS-SEM was more appropriate for non-normal data and small sample sizes (Hair et al. Citation2014). PLS-SEM is evaluated in two steps, the first step is to assess the relationship between the indicators and the latent variable outer model (measurement model). The causal relationship between the latent variables is estimated in the second step (inner model; structural model). Smart PLS 3.0 was used to examine the structural model and hypothesis. To estimate t-statistics to check for statistical significance of the standardized path coefficients (β), a bootstrapping procedure with 5000 sub-samples was applied.
Results and discussion
Descriptive statistics of fish farmers
Socio-economic characteristics of the respondent’s fish farmers showing categorical and continuous variables are presented in Tables and respectively. Pond farmers constituted 53.6% of the farmers while cage farmers constituted 46.4%. Males constituted 73.6% of all fish farmers with 26.1% being females. The possible reason for male dominance in fish farming is attributed to the tedious and energy-sapping nature of activities involved which most women may not be able to cope with. Previous findings have shown that participation by women in fish farming operations varies greatly between African countries (Veliu et al. Citation2009; FAO Citation2014; Jahan et al. Citation2015). About 52.1% of the fish farmers had attained secondary level of education. Farmers with a higher level of formal education were more likely to adopt fish farming techniques than those with less formal education since they are likely to attend various fish training seminars, comprehend and apply information packages. Majority of the fish farmers (94.3%) were involved in commercial fish production with only 5.7% involved in subsistence fish production. There is an emerging commercial-scale aquaculture industry in Kenya comprising both large- and small-to medium-scale production.
Table 2. Fish Farmer Socio-economic profile for categorical variables.
Table 3. Fish Farmer Socio-economic profile for continuous variables.
About 54% of the fish farmers were involved in fish farming as their major occupation, with 30.8% involved in off-farm business and 15.2% in salaried employment. This implies that the majority of the fish farmers have diversified income sources and as such reduces the vulnerability of farmers to risks. Sixty one percent of fish farmers had not accessed credit facilities to boost their fish farming activities while 56.4% had not accessed extension services and 80.6% were not members of fish farming groups. The extension service has a vital role of increasing and improving fish production through their linkage between researchers and end-users. Olaoye et al. (Citation2016) found that adoption of improved technologies could be easily facilitated to group of fish farmers because it is easier to demonstrate the technologies to a group than to an individual.
The findings reveal that the sampled respondents composed of middle-aged fish farmers with an average age of around 43 years which is in line with findings by Muddassir et al. (Citation2019). In relation to years of fish farming experience, the minimum years of experience was found to be 1 year while the maximum was 12 years with a mean of 3.65 years. Regarding the stocking density for ponds and cages, the average stocking density was 3665 fish per pond/cage. Fish farmers had a minimum of 1 pond/cage and a maximum of 195 ponds/cages. The average number of pond/cage ownership was 8 ponds/cages. There was great variation in the size and number of fishponds and cages between fish farming systems within the study sites. This can be explained by existing conditions on each farm including the nature of soils, climatic conditions, availability of labors, building materials, investment capital, and geographical location. This corresponds with previous findings about pond-based fish farming in Kenya, where the majority of smallholder farmers had a minimum of 1 pond to a maximum of 60 fishponds (Obwanga et al. Citation2017). Fish farmers sourced their fish feeds on an average distance of 52.52 km from the fish farms. The study also found that fish farmers were feeding their fish on average twice a day.
Measurement model analysis
Prior to the measurement model analysis, Harman’s single-factor test as suggested by Podsakoff et al. (Citation2003) was conducted on the four constructs in our proposed model including PEOU, PU, ATT, and INT to address the issue of common method biases. Results show that there are six factors whose eigenvalues are greater than one, and the variation of the first factor is only 31.15% among the unrotated principal component factors, indicating that the common method bias was unlikely a problem in this study (Table ).
Convergent and discriminant validity tests were run as part of the measurement model analysis. Convergent validity is concerned with whether the observable variables (items) share sufficient variance in the construct/latent variable. This was evaluated using three indicators: factor loadings, composite reliability, and extracted average variance (AVE). To be retained for the next analysis, an item should have a minimum of 0.707 loading on its theoretical assigned latent construct. Twelve measurement items (PU1, PU2, PU4, PU5, PU6, PU8, PU9, PEOU4, ATT4, ATT5, ATT6, ATT7) had factor loadings below the minimum value, and were exempted from subsequent analysis. The final results of these analyses are presented in Table and Figure . The results in Table show that all the constructs, the factor loadings are above 0.6. The scales used in this study were derived from previous research. According to the findings, both Cronbach's alpha and composite reliability, which are alternative measures of internal consistency and reliability, revealed that all constructs had high internal consistency (Hair et al. Citation2011). The Cronbach alpha values ranged from 0.684 to 0.811, composite reliability ranged from 0.823 to 0.888 while the average variance extracted ranged from 0.610 to 0.726. The composite reliability estimates are above the recommended threshold of 0.7 and the AVEs of the constructs are above 0.5. These results generally confirm the convergent validity of the measurement model (Table ).
Table 4. Internal consistency: Cronbach's Alpha, composite reliability, and average variance extracted.
Discriminant validity was assessed by comparing the values of correlates to square root of AVE values. The preconditions are that the correlates must be smaller than the square root of AVE to satisfy the condition of discriminant validity (Fornell and Larcker Citation1981). The Fornell–Larcker Criterion results (Table ) show that discriminant validity was well established across all the constructs.
Table 5. Fornell-Larcker criterion analysis for checking discriminant validity.
The results show that the discriminant validity of the model has been established, since the HTMT values are significantly lower than 1 (Henseler et al. Citation2015). The HTMT ratios as in were all within the cut off levels of below 0.9 except for the HTMT for intention to use which was exactly 0.9 (Hair et al. Citation2016).
Structural model analysis
Prior to the analysis of the structural model, a collinearity test using the variance inflation factor (VIF) was performed to assess the level of collinearity threat among the independent variables. The results of the multi-collinearity analysis showed that there was no multi-collinearity between latent variables in the structural model with a VIF < 5 as shown in Table . Ideally, VIF values of 5 or lower are desirable to avoid the collinearity problems (Hair et al. Citation2011).
Table 6. Heterotrait-Monotrait (HTMT) criterion.
Having established the collinearity of the independent variables and validity of the measurement model, the next step was to examine the path coefficients and to test the theoretical relationships (Figure ). Path analysis was applied to show the direct, indirect, and total impacts of the independent constructs on behavioral intentions, and thereby, fish farmers’ application behavior. The determination coefficient (R2) was applied to show the variances accounted for by each of the constructs and total variance accounted (Table ).
Table 7. Results of path coefficients and hypothesis testing, n = 211.
Since standardized path coefficients were estimated, a comparison of the coefficients’ magnitudes indicates the degree each exogenous latent variable influences the endogenous latent variable. The inner model path coefficients suggest that ATT has the strongest effect on farmers’ intentions to adopt BSFL (0.411), followed by PU (0.319), and PEOU (0.178). In line with previous studies (McDonald et al. Citation2016; Obiero et al. Citation2019), PU had a greater relative influencse on intention to adopt BSFL than PEOU based on the model path coefficients of 0.319 and 0.178, respectively.
The structural model (Figure ) shows the relationship between one variable and another variable with beta (β) and R-squared (R2) values. The results showed that the R2 for attitude was 0.521, and the R2 for intention to use BSFL was 0.614. The R2 value of intention to use could be explained or influenced by 61.4% of the independent variables (ATT, PU, and PEOU), and the rest were influenced by other factors outside this model.
Bootstrapping was used to obtain t-statistics to test the statistical significance of both the indicators (outer model) and structural model constructs (inner model). The path significance is used to test the hypotheses formulated in this study. Two-tailed t-tests of significance at 5% level were carried out, with t-statistic values larger than 1.96 indicating significance. Bootstrapping was also used to derive t-statistics in the outer model, and the results showed that all the factor loadings were statistically significant at 5% level. The full results are provided in Figure .
Finally, the predictive relevance (Q2) was determined using the blindfolding procedure in PLS-SEM version 3. According to Hair et al. (Citation2017), the Q2 should be greater than zero which means that the predictor variable possesses the predictive relevance for the criterion variable. The Q2 values of attitude was 0.370 and that of intention to use was 0.424 which signifies that the research model had good predictive relevance.
Hypothesis results
The coefficient of PEOU on ATT was positive and significant confirming hypothesis H1 (β = 0.341, p = 0.001) while the coefficient of PU on ATT was positive and significant confirming hypothesis H3 (β = 0.492, p = 0.000). Thus, both PU and PEOU had significant impacts on fish farmers’ attitudes. Attitude, in turn, had a significant impact on behavioral intention. The coefficient of ATT on INT was positive and significant confirming hypothesis H5 (β = 0.411, p = 0.000). This finding on influence of attitude on intention to use BSFL is similar to the results of Maleksaeidi and Keshavarz (Citation2019), Meijer et al. (Citation2015) and Yazdanpanah and Forouzani (Citation2015). This result implies that farmers who had positive attitude towards using BSFL were more eager to use it in their fish farms.
The coefficient of PEOU on INT was positive and significant confirming hypothesis H2 (β = 0.178, p = 0.004). If the BSFL is perceived as easy to use, fish farmers have a higher intention to use it. PEOU reflects fish farmer’s confidence in using BSFL. This is in line with the findings of Giampietri and Trestini (Citation2020) and Zhou and Abdullah (Citation2017). The coefficient of PU on INT was positive and significant confirming hypothesis H4 (β = 0.319, p = 0.000). This indicates the more the respondents consider BSFL to be useful in their fish farm’s production conditions, the higher is their intention toward adopting BSFL. The findings are consistent with those of Caffaro et al. (Citation2020) who found an association between PU and intention to adopt smart farming technologies. Similarly, Li et al. (Citation2021) found that PU had a positive impact on farmers’ participation intention regarding vegetable traceability systems. These results are consistent with TAM that proposes that PU and PEOU are the two major causal variables in determining acceptance and usage behavior of a technology (Obiero et al. Citation2019). Bagheri et al. (Citation2021) found both positive direct and indirect impacts of PU on INT in the use of biological inputs among cereal farmers. Previous studies have found a direct impact of PU on INT, while PEOU has shown direct impact both on PU and INT (Flett et al. Citation2004). Perceived usefulness is important to change and reinforcement of behavioral attitude and behavioral intention. It has a significant role in the model so that perceived ease of use and attitude of confidence affect behavioral attitude through perceived usefulness. A study by Adrian et al. (Citation2005) confirmed that perceived usefulness positively has indirect effect on intention to adopt precision agriculture thereby confirming H7 on the indirect effect of PU on INT through ATT. However, the conflicting results have been reported regarding its impact on intention (Venkatesh and Davis Citation2000; Venkatesh et al. Citation2003).
Conclusion and policy implications
The TAM model was used in this study to investigate factors influencing fish farmers’ intentions to use BSFL in fish farming. All the seven hypotheses of the TAM could be supported by the model. The results of PLS-SEM therefore confirm the stipulated relationships within the theory and provide further evidence of the profound role of the TAM model used in this study. This implies that the TAM constructs had a direct and indirect effects on intention to BSFL in aquaculture by the fish farmers in Kenya. The study results have revealed that based on the relative sizes of the coefficients, attitude was the main determinant of intention, followed by perceived ease of use and then perceived usefulness. The role of attitude to increase behavioral intention has been emphasized. Since attitude indicated the maximum influence on intention, this means that fish farmers who have positive attitudes toward BSFL are more likely to adopt it in fish production, since they have a higher intention in performing this behavior. As a result, a more positive attitude about the BSFL meal boosts behavioral intent. This study has policy significance for agricultural development policymakers because it can assist extension agents, agricultural educators, and agricultural administrators in providing appropriate training and services to customers in order to improve their attitudes. Setting up nationwide seminars is a good way to boost expert capability and perception.
Perceived usefulness is important to change and reinforcement of behavioral attitude and behavioral intention. It has a significant role in the model so that perceived ease of use and attitude of confidence affect behavioral attitude through usefulness. Thus, trainings should be planned in relation to justifying usefulness of these novel feed technologies by the experts. It is suggested that a practical method instruction should be designed and implemented for raising knowledge and information based on method-demonstration and result-demonstration on use of BSFL in aquaculture.
This study provides strong empirical insights to propose and test a model for assessing technology-acceptance related factors that influence farmers’ intentions to use BSFL for improving their fish production. This implies that the results provide a strong foundation for application in different contexts and theoretical assessments in other research fields. The results of this study can be used by actors along fish feed value chain in understanding the process of technology adoption. Previous TAM studies on adoption have typically focused on quantifying adoption predictions, rather than exploring how social factors interact and influence intentions and behaviors. This study demonstrates how the TAM can be qualitatively applied to better understand farmer decision making, in this instance the use of BSLF meal. The study demonstrates how the TAM can provide an evidence-based framework to qualitatively explore fish farmer intentions and behavior. This approach has led to new insights into farmer decision making that will inform improvements in future extension development.
There is a need to raise awareness and promote the use of BSFL in fish production among fish farmers. Strengthening fish farmer groups could also improve fish farmers’ attitudes towards BSFL. Relevant government departments can expand the influence and awareness of BSFL feeds through multiple publicity channels and media. For example, public programs such as training for farmers should be put in place to help them understand and realize that using BSFL in fish production can be of great economic benefit.
Limitations and future research scope
There are a variety of potential drawbacks that can be highlighted. First, this study was primarily intended to evaluate farmers’ intentions toward BSFL, with little attention devoted to actual measurements of BSFL use (adoption) by fish farmers in the study area. While behavioral intention is a necessary condition for actual adoption, it is not sufficient. Furthermore, socio-demographic factors are not taken into account, despite the fact that these factors are predicted to have direct and indirect effects on intentions, attitudes, and perceived controls. Accordingly, the results may suffer from an omitted variable bias. Nevertheless, the findings of the study are still valuable.
Despite the limitations mentioned above, this research contributes to a better understanding of the essential theoretical and practical implications of fish farmers’ intentions to adopt BSFL in their fish production. Future research should look into how socio-demographic factors influence differences in attitudes, perceptions, and intentions about BSFL adoption. For example, it would be interesting to study the effect of scale of production or cultural differences on the actual adoption and decision behavior of farmers in Kenya and elsewhere in the world. Secondly, future studies should also focus on analyzing the factors that influence adoption of BSFL, using a sample of fish farmers that adopt and non-adopt BSFL using regression models and analyze the same sample, to verify the characteristics of farmers who adopted BSFL.
Acknowledgement
The researchers thank in advance the anonymous reviewers for their helpful comments.
Disclosure statement
No potential conflict of interest was reported by the author(s).
Data availability
The data that support the findings of this study are openly available in Figshare at https://doi.org/10.6084/m9.figshare.19739023.
Contributions
Conception and design of the research: KOO1, AWM and KOO2; acquisition of data: KOO1 and KOO2; analysis and interpretation of data: KOO1 and FAO; drafting of manuscript: KOO1, AWM, KOO2 and FAO; revision of manuscript for important intellectual content: KOO1, AWM, KOO2 and FAO. All authors read and approved the final manuscript.
Ethics approval
Ethical approval was granted by JOOUST Ethics Review Committee [approval number ERC/28/10/20-12] and National Commission for Science, Technology and Innovation (NACOSTI) [research licence number NACOSTI/P/20/8040].
Additional information
Funding
References
- Adrian AM, Norwood SH, Mask PL. 2005. Producers’ perceptions and attitudes toward precision agriculture technologies. Computers and Electronics in Agriculture. 48(3):256–271. doi:10.1016/j.compag.2005.04.004.
- Ajzen I. 1991. The theory of planned behavior. Organ Behav Hum Decis Process. 50(2):179–211. doi:10.1016/0749-5978(91)90020-T.
- Alhazzaa R, Nichols PD, Carter CG. 2019. Sustainable alternatives to dietary fish oil in tropical fish aquaculture. Rev Aquac. 11(4):1195–1218. doi:10.1111/raq.12287.
- Amankwah A, Quagrainie KK, Preckel PV. 2018. Impact of aquaculture feed technology on fish income and poverty in Kenya. Aquac Econ Manage. 22(4):410–430. doi:10.1080/13657305.2017.1413689.
- Amza N, Tamiru M. 2017. Insects as an option to conventional protein sources in animal feed: a review paper. Glob J Sci Front Res D Agric Vet. 17:12. https://www.researchgate.net/profile/MetekiaTamiru/publication/344264517_Insects_as_an_Option_to_Conventional_Protein_Sources_in_Animal_Feed_A_Review_Paper/links/5f61bc9992851c07896a465a/Insects-as-an-Option-to-Conventional-Protein-Sources-in-Animal-Feed-A-Review-Paper.pdf.
- Aren S, Güzel M, Kabadayı E, Alpkan L. 2013. Factors affecting repurchase intention to shop at the same website. Procedia-Soc Behav Sci. 99:536–544. doi:10.1016/j.sbspro.2013.10.523.
- Arru B, Furesi R, Gasco L, Madau FA, Pulina P. 2019. The introduction of insect meal into fish diet: the first economic analysis on European sea bass farming. Sustainability. 11(6):1697. doi:10.3390/su11061697.
- Aura CM, Musa S, Yongo E, Okechi JK, Njiru JM, Ogari Z, Wanyma R, Karisa HC, Mbugua H, Kidera S, Ombwa V, et al. 2018. Integration of mapping and socio-economic status of cage culture: towards balancing lake-use and culture fisheries in lake Victoria, Kenya. Aquac Res. 49(1):532–545. doi:10.1111/are.13484.
- Bagheri A, Bondori A, Allahyari MS, Surujlal J. 2021. Use of biologic inputs among cereal farmers: application of technology acceptance model. Environ Dev Sustain. 23(4):5165–5181. doi:10.1007/s10668-020-00808-9.
- Belghit I, Liland NS, Gjesdal P, Biancarosa I, Menchetti E, Li Y, Waagbø R, Krogdahl Å, Lock E-J. 2019. Black soldier fly larvae meal can replace fish meal in diets of sea-water phase Atlantic salmon (Salmo salar). Aquaculture. 503:609–619. doi:10.1016/j.aquaculture.2018.12.032.
- Belghit I, Liland NS, Waagbø R, Biancarosa I, Pelusio N, Li Y, Krogda HL, Lock EJ. 2018. Potential of insect-based diets for Atlantic salmon (Salmo salar). Aquaculture. 491:72–81. doi:10.1016/j.aquaculture.2018.03.016.
- Bijttebier J, Ruysschaert G, Hijbeek R, Werner M, Pronk AA, Zavattaro L, Bechini L, Grignani C, ten Berge H, Marchand F, Wauters E. 2018. Adoption of non-inversion tillage across Europe: use of a behavioural approach in understanding decision making of farmers. Land use Policy. 78:460–471. doi:10.1016/j.landusepol.2018.05.044.
- Brugere C, Padmakumar KP, Leschen W, Tocher DR. 2021. What influences the intention to adopt aquaculture innovations? Concepts and empirical assessment of fish farmers’ perceptions and beliefs about aquafeed containing non-conventional ingredients. Aquac Econ Manag. 25(3):339–366. doi:10.1080/13657305.2020.1840661.
- Caffaro F, Cremasco MM, Roccato M, Cavallo E. 2020. Drivers of farmers’ intention to adopt technological innovations in Italy: the role of information sources, perceived usefulness, and perceived ease of use. J Rural Stud. 76:264–271. doi:10.1016/j.jrurstud.2020.04.028.
- Castiblanco Jimenez IA, Cepeda García LC, Marcolin F, Violante MG, & Vezzetti E. 2021. Validation of a TAM extension in agriculture: exploring the determinants of acceptance of an e-learning platform. Applied Sciences. 11(10):4672. doi:10.3390/app11104672.
- Chaalala S, Leplat A, Makkar H. 2018. Importance of insects for use as animal feed in low-income countries. In: Halloran A., Flore R., Vantomme P., Roos N, editors. Edible insects in sustainable food systems. Cham: Springer. doi:10.1007/978-3-319-74011-9_18
- Chang G, Wang L, Meng L, Zhang W. 2016. Farmers’ attitudes toward mandatory water-saving policies: A case study in two basins in northwest China. J Environ Manage. 181:455–464. doi:10.1016/j.jenvman.2016.07.007.
- Chia SY, Macharia J, Diiro GM, Kassie M, Ekesi S, van Loon JJA, Dicke M, Tanga CM, Gao Z. 2020. Smallholder farmers’ knowledge and willingness to pay for insect-based feeds in Kenya. PLOS ONE. 15(3):e0230552. doi:10.1371/journal.pone.0230552.
- Chia SY, Tanga CM, van Loon JJ, Dicke M. 2019. Insects for sustainable animal feed: inclusive business models involving smallholder farmers. Curr Opin Environ Sustain. 41:23–30. doi:10.1016/j.cosust.2019.09.003.
- Chin WW. 1998. The partial least square approach to structural equation modeling. In: Marcoulides G.A., editor. Modern methods for business research. Mahwah: Lawrence Erlbaum; p. 295–358.
- Cummins Jr VC, Rawles SD, Thompson KR, Velasquez A, Kobayashi Y, Hager J, Webster CD. 2017. Evaluation of black soldier fly (Hermetia illucens) larvae meal as partial or total replacement of marine fish meal in practical diets for Pacific white shrimp (Litopenaeus vannamei). Aquaculture. 473:337–344. doi:10.1016/j.aquaculture.2017.02.022.
- Davis FD. 1989. Perceived usefulness, perceived ease of use, and user acceptance. Manage Inf Syst Q. 13:319–339. doi:10.2307/249008.
- Despotović J, Rodić V, Caracciolo F. 2019. Factors affecting farmers’ adoption of integrated pest management in Serbia: an application of the theory of planned behavior. J Cleaner Prod. 228:1196–1205. doi:10.1016/j.jclepro.2019.04.149.
- Dessart FJ, Barreiro-Hurlé J, van Bavel R. 2019. Behavioural factors affecting the adoption of sustainable farming practices: a policy-oriented review. Eur Rev Agric Econ. 46(3):417–471.
- Dicke M. 2018. Insects as feed and the sustainable development goals. J Insects Food Feed. 4:147–156. doi:10.3920/JIFF2018.0003.
- Domingues CHDF, Borges JAR, Ruviaro CF, Gomes Freire Guidolin D, Rosa Mauad Carrijo J. 2020. Understanding the factors influencing consumer willingness to accept the use of insects to feed poultry, cattle, pigs and fish in Brazil. PloS one. 15(4):e0224059. doi:10.1371/journal.pone.0224059.
- Ducey AJ, Coovert MD. 2016. Predicting tablet computer use: an extended technology acceptance model for physicians. Health Policy Technol. 5(3):268–284. doi:10.1016/j.hlpt.2016.03.010.
- Dumas A, Raggi T, Barkhouse J, Lewis E, Weltzien E. 2018. The oil fraction and partially defatted meal of black soldier fly larvae (Hermetia illucens) affect differently growth performance, feed efficiency, nutrient deposition, blood glucose and lipid digestibility of rainbow trout (Oncorhynchus mykiss). Aquaculture. 492:24–34. doi:10.1016/j.aquaculture.2018.03.038.
- Elia AC, Capucchio MT, Caldaroni B, Magara G, Dörr AJM, Biasato I, Biasibetti E, Righetti M, Pastorino P, Prearo M. 2018. Influence of Hermetia illucens meal dietary inclusion on the histological traits, gut mucin composition and the oxidative stress biomarkers in rainbow trout (Oncorhynchus mykiss). Aquaculture. 496:50–57. doi:10.1016/j.aquaculture.2018.07.009.
- FAO. 2013. Edible insects: Future Prospects for feed and food security. FAO Publication. https://library.wur.nl/WebQuery/wurpubs/fulltext/258042
- FAO. 2014. The state of world fisheries and aquaculture: opportunities and challenges, 223. Rome: Food and Agriculture Organization of the United Nations.
- FAO. 2020. The state of world fisheries and aquaculture 2020: sustainability in action. Rome: Food and Agriculture Organization of the United Nations.
- Fishbein M, Ajzen I. 1977. Belief, attitude, intention, and behavior: an introduction to theory and research. Philos Rhetor. 10:2.
- Fitzsimmons K. 2016. Tilapia aquaculture 2016 and where will we be in 2026. In: 11th international symposium for Tilapia Aquaculture (ISTA), World Aquaculture Society-Asian Pacific Conference; p. 26–29.
- Flett R, Alpass F, Humphries S, Massey C, Morriss S, Long N. 2004. The technology acceptance model and use of technology in New Zealand dairy farming. Agric Sys. 80(2):199–211. doi:10.1016/j.agsy.2003.08.002.
- Fornell C, Larcker DF. 1981. Structural equation models with unobservable variables and measurement error: algebra and statistics. J Mark Res. 18(3):382–388. doi:10.2307/3150980.
- Ghorbani Piralidehi, F., & Ghorbani Piralidehi, A. (2020). Factors affecting the pro-environmental behavior of aquaculture farmers in guilan province. Environ Sci. 18(1):17–32. doi:10.29252/envs.18.1.17
- Giampietri E, Trestini S. 2020. Analysing farmers’ intention to adopt web marketing under a technology-organisation-environment perspective: a case study in Italy. Agric Econ. 66(5):226–233. doi:10.17221/355/2019-AGRICECON.
- Githukia CM, Ogello EO, Kembenya EM, Achieng AO, Obiero KO, Munguti JM. 2015. Comparative growth performance of male monosex and mixed sex nile tilapia (Oreochromis niloticus l.) reared in earthen ponds. Croat J Fish. 73(1):20–25. doi:10.14798/73.1.788.
- Haenlein M, Kaplan AM. 2004. A beginner's guide to partial least squares analysis. Underst Stat. 3(4):283–297.
- Hair JF, Hult GTM, Ringle C, Sarstedt M. 2016. A primer on partial least squares structural equation modeling (PLS-SEM). Thousand Oaks, CA: Sage publications.
- Hair JF, Hult GTM, Ringle CM, Sarstedt M. 2017. A primer on partial least squares structural equation modeling (PLS-SEM) (2nd ed.). Thousand Oaks, CA: Sage.
- Hair JF, Ringle CM, Sarstedt M. 2011. PLS-SEM: indeed, a silver bullet. J Mark Theory Prac. 19(2):139–152. doi:10.2753/MTP1069-6679190202.
- Hair, Jr, J. F., Sarstedt, M., Hopkins, L., & Kuppelwieser, V. G. (2014). Partial least squares structural equation modeling (PLS-SEM): an emerging tool in business research. Eur Bus Rev, 26 (2), 106–121 doi:10.1108/EBR-10-2013-0128
- Hasimuna OJ, Maulu S, Mphande J. 2020. Aquaculture health management practices in Zambia: status, challenges and proposed biosecurity measures. J Aquac Res Dev. 11:3. doi:10.35248/2155-9546.19.10.584.
- Henry M, Gasco L, Piccolo G, Fountoulaki E. 2015. Review on the use of insects in the diet of farmed fish: past and future. Anim Feed Sci Technol. 203:1–22. doi:10.1016/j.anifeedsci.2015.03.001.
- Henseler J, Ringle CM, Sarstedt M. 2015. A new criterion for assessing discriminant validity in variance-based structural equation modeling. J Acad Mark Sci. 43(1):115–135. doi:10.1007/s11747-014-0403-8.
- Higa JE, Ruby MB, Rozin P. 2021. Americans’ acceptance of black soldier fly larvae as food for themselves, their dogs, and farmed animals. Food Qual Prefer. 90:104119. doi:10.1016/j.foodqual.2020.104119.
- Honkanen P, Young JA. 2015. What determines British consumers’ motivation to buy sustainable seafood?. Bri Food J. 117(4):1289–1302. doi:10.1108/BFJ-06-2014-0199.
- Ibitoye OS, Oyetunji PO. 2019. Entrepreneurial potentials of insect farming in Nigeria. J Nat Sci Res. 9(9):57–65. doi:10.7176/JNSR.
- Jahan KM, Belton B, Ali H, Dhar GC, Ara I. 2015. Aquaculture technologies in Bangladesh: an assessment of technical and economic performance and producer behavior, 2015–52. Penang: WorldFish. Program Report
- Joffre OM, De Vries JR, Klerkx L, Poortvliet PM. 2020. Why are cluster farmers adopting more aquaculture technologies and practices? The role of trust and interaction within shrimp farmers’ networks in the Mekong Delta, Vietnam. Aquaculture. 523:735181. doi:10.1016/j.aquaculture.2020.735181.
- Kelemu S, Niassy S, Torto B, Fiaboe K, Affognon H, Tonnang H, Maniania NK, Ekesi S. 2015. African edible insects for food and feed: inventory, diversity, commonalities and contribution to food security. J Insects Food Feed. 1(2):103–119. doi:10.3920/JIFF2014.0016.
- Kurkinen E. 2012. On the exploration of mobile technology acceptance among law enforcement officers using Structural Equation Modelling (SEM): a multi-group analysis of the Finnish police force. Jyväsk Stud Comput. 159. https://jyx.jyu.fi/bitstream/handle/123456789/40655/1/978-951-39-4980-8.pdf
- Lalander CH, Fidjeland J, Diener S, Eriksson S, Vinnerås B. 2015. High waste-to-biomass conversion and efficient Salmonella spp. reduction using black soldier fly for waste recycling. Agron Sustain Dev. 35(1):261–271. doi:10.1007/s13593-014-0235-4.
- Lalani B, Dorward P, Holloway G, Wauters E. 2016. Smallholder farmers’ motivations for using conservation agriculture and the roles of yield, labour and soil fertility in decision making. Agric Sys. 146:80–90. doi:10.1016/j.agsy.2016.04.002.
- Li L, Paudel KP, Guo J. 2021. Understanding Chinese farmers’ participation behavior regarding vegetable traceability systems. Food Control. 108325. doi:10.1016/j.foodcont.2021.108325.
- Liland NS, Biancarosa I, Araujo P, Biemans D, Bruckner CG, Waagbø R, Torstwensen EBZ, Lock EJ. 2017. Modulation of nutrient composition of black soldier fly (Hermetia illucens) larvae by feeding seaweed-enriched media. PloS one. 12(8):e0183188. doi:10.1371/journal.pone.0183188.
- Magalhães R, Sánchez-López A, Leal RS, Martínez-Llorens S, Oliva-Teles A, Peres H. 2017. Black soldier fly (Hermetia illucens) pre-pupae meal as a fish meal replacement in diets for European seabass (Dicentrarchus labrax). Aquaculture. 476:79–85. doi:10.1016/j.aquaculture.2017.04.021.
- Makkar HP, Tran G, Heuzé V, Ankers P. 2014. State-of-the-art on use of insects as animal feed. Anim Feed Sci Technol. 197:1–33.
- Maleksaeidi H, Keshavarz M. 2019. What influences farmers’ intentions to conserve on-farm biodiversity? An application of the theory of planned behavior in fars province, Iran. Glob Ecol Conserv. 20:e00698. doi:10.1016/j.gecco.2019.e00698.
- McDonald R, Heanue K, Pierce K, Horan B. 2016. Factors influencing new entrant dairy farmer’s decisionmaking process around technology adoption. J Agric Educ Ext. 22:163–177. doi:10.1080/1389224 X.2015.1026364.
- Meijer SS, Catacutan D, Sileshi GW, Nieuwenhuis M. 2015. Tree planting by smallholder farmers in Malawi: using the theory of planned behaviour to examine the relationship between attitudes and behaviour. J Environ Psychol. 43:1–12. doi:10.1016/j.jenvp.2015.05.008.
- Michels M, von Hobe CF, Weller von Ahlefeld PJ, Musshoff O. 2021. The adoption of drones in German agriculture: a structural equation model. Precis Agric. 22(6):1728–1748. doi:10.1007/s11119-021-09809-8.
- Mmanda FP, Mulokozi DP, Lindberg JE, Norman Haldén A, Mtolera M, Kitula R, Lundh T. 2020. Fish farming in Tanzania: the availability and nutritive value of local feed ingredients. J Appl Aquac. 32(4):341–360. doi:10.1080/10454438.2019.1708836.
- Mogendi JB, De Steur H, Gellynck X, Makokha A. 2016. A novel framework for analysing stakeholder interest in healthy foods: a case study on iodine biofortification. Ecol Food Nutr. 55(2):182–208. doi:10.1080/03670244.2015.1112283.
- Mousavi S, Zahedinezhad S, Loh JY. 2020. A review on insect meals in aquaculture: the immunomodulatory and physiological effects. Int Aquat Res. 12(2):100–115. doi:10.22034/IAR(20).2020.1897402.1033.
- Muddassir M, Noor MA, Ahmed A, Aldosari F, Waqas MA, Zia MA, Mubushar M, Zuhaibe AH, Jalip MW. 2019. Awareness and adoption level of fish farmers regarding recommended fish farming practices in Hafizabad, Pakistan. J Saudi Soc Agric Sci. 18(1):41–48. doi:10.1016/j.jssas.2016.12.004.
- Muin H, Taufek NM, Kamarudin MS, Razak SA. 2017. Growth performance, feed utilization and body composition of Nile tilapia, Oreochromis niloticus (Linnaeus, 1758) fed with different levels of black soldier fly, Hermetia illucens (Linnaeus, 1758) maggot meal diet. Iran J Fish Sci. 16(2):567–577. http://jifro.ir/article-1-2721-en.html.
- Mulumpwa M. 2018. The potential of insect meal in improving food security in Malawi: an alternative of soybean and fishmeal in livestock feed. J Insects Food Feed. 4(4):301–312. doi:10.3920/JIFF2017.0090.
- Mutyasira V, Hoag D, Pendell D. 2018. The adoption of sustainable agricultural practices by smallholder farmers in Ethiopian highlands: an integrative approach. Cogent Food Agric. 4(1):1552439. doi:10.1080/23311932.2018.1552439.
- Nogales-Mérida S, Gobbi P, Józefiak D, Mazurkiewicz J, Dudek K, Rawski M, Kierończyk B, Józefiak A. 2018. Insect meals in fish nutrition. Rev Aquac. 11(4):1080–1103. doi:10.1111/raq.12281.
- Nunnally JC. 1978. Psychometric theory. 2nd ed. New York: McGraw-Hill.
- Nyakeri EM, Ogola HJ, Ayieko MA, Amimo FA. 2017. An open system for farming black soldier fly larvae as a source of proteins for smallscale poultry and fish production. J Insects Food Feed. 3(1):51–56. doi:10.3920/JIFF2016.0030.
- Obiero KO, Waidbacher H, Nyawanda BO, Munguti JM, Manyala JO, Kaunda-Arara B. 2019. Predicting uptake of aquaculture technologies among smallholder fish farmers in Kenya. Aquac Int. 27(6):1689–1707.
- Obwanga B, Lewo MR, Bolman B, van Rijn F, Musyoke MP. 2017. From aid to responsible trade: driving competitive aquaculture sector development in Kenya; quick scan of robustness, reliability and resilience of the aquaculture sector. Wageningen: Wageningen University & Research; p. 68. Report 2017-092 3R Kenya. doi:10.18174/421667
- Olaoye OJ, Ezeri GO, Akegbejo-Samsons Y, Awotunde JM, Ojebiyi WG. 2016. Dynamics of the adoption of improved aquaculture technologies among fish farmers in Lagos State, Nigeria. Croat J Fish. 74(2):56–70. doi: 10.1515/cjf-2016-0012.
- Opiyo MA, Marijani E, Muendo P, Odede R, Leschen W, Charo-Karisa H. 2018. A review of aquaculture production and health management practices of farmed fish in Kenya. Int J Vet Sci Med. 6(2):141–148. doi:10.1016/j.ijvsm.2018.07.001.
- Oppong MIRIAM. 2017. Black soldier fly larvae-based fish feed production: financial feasibility and acceptability analysis (Doctoral dissertation). University Of Ghana. http://ugspace.ug.edu.gh/handle/123456789/23632
- Orina S, Ogello EO, Kembenya EM, Muthoni C. 2018. State of Cage Culture in Lake Victoria, Kenya. June 2019 http://41.89.141.8/kmfri/bitstream/123456789/1107/1/STATE%20OF%20CAGE%20CULTURE%20%20for%20mail-1_Laxpress.pdf.
- Podsakoff PM, Mackensie SB, Le E JY. 2003. Common method biases in behavioural research: a critical review of the literature and recommended remedies. J Appl Psychol. 88(5):879–903. doi:10.1037/0021-9010.88.5.879.
- Quagrainie KK, Ngugi CC, Amisah S. 2010. Analysis of the use of credit facilities by small-scale fish farmers in Kenya. Aquac Int. 18(3):393–402. doi:10.1007/s10499-009-9252-8.
- Rana KS, Salam MA, Hashem S, Islam MA. 2015. Development of black soldier fly larvae production technique as an alternate fish feed. Int J Res Fish Aquac. 5(1):41–47. https://www.researchgate.net/publication/315574295_Development_of_Black_Soldier_Fly_Larvae_Production_Technique_as_an_Alternate_Fish_Feed.
- Renna M, Schiavone A, Gai F, Dabbou S, Lussiana C, Malfatto V, Prearo M, Capucchio MT, Biasato I, Biasibetti E, et al. 2017. Evaluation of the suitability of a partially defatted black soldier fly (Hermetia illucens L.) larvae meal as ingredient for rainbow trout (Oncorhynchus mykiss Walbaum) diets. J Anim Sci Biotechnol. 8(1):1–13. doi:10.1186/s40104-017-019.
- Riddick EW. 2013. Insect protein as a partial replacement for fishmeal in the diets of juvenile fish and crustaceans. In: Morales-Ramos J, Rojas GM, Shapiro-Ilan D, editors. Mass production of beneficial organisms. Elsevier; p. 565–582. doi:10.1016/B978-0-12-391453-8.00016-9.
- Ringle CM, Wende S, Becker JM. 2014. Smartpls 3. Hamburg: SmartPLS. http://www.smartpls.com.
- Roffeis M, Wakefield ME, Almeida J, Alves Valada TR, Devic E, Koné N, Kenis M, Nacambo S, Fitches EC, Koko GKD, et al. 2018. Life cycle cost assessment of insect based feed production in West Africa. J Clean Prod. 199:792–806. doi:10.1016/j.jclepro.2018.07.179.
- Sharfuddin J, Mohammed Z, Teramo R. 2018. Paddy farmer’s perception and factors influencing attitude and intention on adoption of organic rice farming. Int Food Res J. 11(3):1–6. http://www.ifrj.upm.edu.my/25%20(08)%202018%20supplementary%202/3%20-%20IFRJ18255.R2%20edited.pdf
- Silva A, Canavero M, Sedale KL. 2017. A technology acceptance model of common bean growers’ intention to adopt integrated production in the Brazilian Central Region = Ein Technologie-Akzeptanzmodell zur Absicht von Bohnenproduzenten, Integrierte Produktion im brasilianischen Zentralgebiet einzuführen. Bodenkultur-Wien and Munchen. 68(3):131–143.
- Sinansari S, Fahmi MR. 2020, May. Black soldier fly larvae as nutrient-rich diets for ornamental fish. In: IOP conference series: earth and environmental science. IOP Publishing. Vol. 493, No. 1, p. 012027 doi:10.1088/1755-1315/493/1/012027
- Sok J, Borges JR, Schmidt P, Ajzen I. 2021. Farmer behaviour as reasoned action: a critical review of research with the theory of planned behaviour. J Agric Econ. 72(2):388–412. doi:10.1111/1477-9552.12408.
- Ssepuuya G, Sebatta C, Sikahwa E, Fuuna P, Sengendo M, Mugisha J, Fiaboe KKM, Nakimbugwe D. 2019. Perception and awareness of insects as an alternative protein source among fish farmers and fish feed traders. J Insects Food Feed. 5(2):107–116. doi:10.3920/JIFF2017.0056.
- Tiu LG. 2012. Enhancing sustainability of freshwater prawn production in Ohio. Ohio State University South Center Newsletter. 11: 4.
- Tran N, Chu L, Chan CY, Genschick S, Phillips MJ, Kefi AS. 2019. Fish supply and demand for food security in Sub-saharan Africa: an analysis of the Zambian fish sector. Mar Policy. 99:343–350. doi:10.1016/j.marpol.2018.11.009.
- Ulhaq I, Pham NTA, Le V, Pham HC, Le TC. 2022. Factors influencing intention to adopt ICT among intensive shrimp farmers. Aquaculture. 547:737407. doi:10.1016/j.aquaculture.2021.737407.
- Van Huis A, Van Itterbeeck J, Klunder H, Mertens E, Halloran A, Muir G, Vantomme P. 2013. Edible insects: future prospects for food and feed security. FAO Forestry Paper no. 171. http://www.fao.org/docrep/018/i3253e/i3253e.pdf.
- Veliu A, Gessese N, Ragasa C, Okali C. 2009. Gender analysis of aquaculture value chain in northeast Vietnam and Nigeria. Agriculture and Rural Development Discussion Paper 44. The World Bank, Washington.
- Venkatesh V, Davis FD. 2000. A theoretical extension of the technology acceptance model: four longitudinal field studies. Manage Sci. 46(2):186–204. doi:10.1287/mnsc.46.2.186.11926.
- Venkatesh V, Morris MG, Davis FD, Davis GB. 2003. User acceptance of information technology: toward a unified view. Manage Inf Syst Q. 27(3):425–478. doi:10.2307/30036540.
- Verbeke W, Spranghers T, De Clercq P, De Smet S, Sas B, Eeckhout M. 2015. Insects in animal feed: acceptance and its determinants among farmers, agriculture sector stakeholders and citizens. Anim Feed Sci Technol. 204:72–87. doi:10.1016/j.anifeedsci.2015.04.001.
- Verma S, Bhattacharyya SS, Kumar S. 2018. An extension of the technology acceptance model in the big data analytics system implementation environment. Inf Process Manag. 54(5):791–806. doi:10.1016/j.ipm.2018.01.004.
- Vogel Heiko, Müller Ariane, Heckel David G, Gutzeit Herwig, Vilcinskas Andreas. 2018. Nutritional immunology: Diversification and diet-dependent expression of antimicrobial peptides in the black soldier fly Hermetia illucens. Developmental & Comparative Immunology. 78:141–148. doi:10.1016/j.dci.2017.09.008.
- Wang YS, Shelomi M. 2017. Review of black soldier fly (Hermetia illucens) as animal feed and human food. Foods. 6(10):91. doi:10.3390/foods6100091.
- Yazdanpanah M, Forouzani M. 2015. Application of the theory of planned behaviour to predict Iranian students’ intention to purchase organic food. J Cleaner Prod. 107:342–352. doi:10.1016/j.jclepro.2015.02.071.
- Yazdanpanah M, Moghadam MT, Zobeidi T, Turetta APD, Eufemia L, Sieber S. 2022. What factors contribute to conversion to organic farming? Consideration of the health belief model in relation to the uptake of organic farming by Iranian farmers. J Environ Planning Manage. 65(5):907–929. doi:10.1080/09640568.2021.1917348.
- Yildirim-Aksoy M, Eljack R, Schrimsher C, Beck BH. 2020. Use of dietary frass from black soldier fly larvae, Hermetia illucens, in hybrid tilapia (Nile x Mozambique, Oreochromis niloticus x O. mozambique) diets improves growth and resistance to bacterial diseases. Aquac Rep. 17:100373. doi:10.1016/j.aqrep.2020.100373.
- Zeweld W, Van Huylenbroeck G, Tesfay G, Azadi H, Speelman S. 2018. Impacts of socio-psychological factors on actual adoption of sustainable land management practices in dryland and water stressed areas. Sustainability. 10(9):2963. doi:10.3390/su10092963.
- Zeweld W, Van Huylenbroeck G, Tesfay G, Speelman S. 2017. Smallholder farmers’ behavioural intentions towards sustainable agricultural practices. J Environ Manag. 187:71–81. doi:10.1016/j.jenvman.2016.11.014.
- Zhou D, Abdullah. 2017. The acceptance of solar water pump technology among rural farmers of northern Pakistan: a structural equation model. Cogent Food Agric. 3(1):1280882. doi:10.1080/23311932.2017.1280882.
Appendix
Table A1. Latent variables, indicator ID and the corresponding questionnaire statements used within the survey.
Table A2. Test for common method bias.
Table A3. Variance inflation factor (VIF) values.
Table A4. Outer loadings (factor loadings).