Abstract
This article presents the Data Science Ethos Lifecycle, a tool for engaging responsible workflow developed by an interdisciplinary team of social scientists and data scientists working with the Academic Data Science Alliance. The tool uses a data science lifecycle framework to engage data science students and practitioners with the ethical dimensions of their practice. The lifecycle supports practitioners to increase awareness of how their practice shapes and is shaped by the social world and to articulate their responsibility to public stakeholders. We discuss the theoretical foundations from the fields of Science, Technology and Society, feminist theory, and critical race theory that animate the Ethos Lifecycle and show how these orient the tool toward a normative commitment to justice and what we call the “world-making” view of data science. We introduce four conceptual lenses—positionality, power, sociotechnical systems, and narratives—that are at work in the Ethos Lifecycle and show how they can bring to light ethical and human issues in a real-world data science project.
1 Articulating a Responsible Ethos of Data Science
The word “ethics,” so closely associated today with what it means to practice responsible data science, comes from the Ancient Greek word “ethos,” which refers to the spirit or character of a people. In public life, it is common to hear qualitative descriptions of the ethos of a historical era. A professional community of doctors, teachers, lawyers, or scientists can also have a discernible ethos. A professional community’s ethos identifies members of the community with certain practices and services to the broader society, and also describes the values and normative commitments that the members adhere to in their professional practice. Considering the ethos of a community invites us to focus on how that community does what it does, such that process and practice are articulated together with spirit and values.
Teaching responsible workflow in data science, a focus of this special issue, is a topic that invites challenging questions about the intersection of ethics (responsibility) and practice or process (workflow), which is captured in the term ethos. What does it mean for a data science workflow to be responsible? To whom or to what does a data scientist owe responsibility? How does the data scientist’s responsibility relate to their workflow? And how can attention to the data science workflow support the teaching and learning of responsible data science?
Without claiming to provide a definitive answer to these important questions, this article introduces a tool called the “Data Science Ethos Lifecycle” (“Ethos Lifecycle” for short), which aims to support data scientists to discover their way to responsible data science practice. The Ethos Lifecycle is a framework, implemented in the form of an interactive online tool (in development), for data science students, teachers, and professional practitioners in research and industry (throughout the article we refer to “practitioners” of data science more generally) to make explicit and consider salient human contexts and consequences at every stage of their work. The tool merges data science workflow with ethical analysis. It embeds consideration of the human contexts and ethics of data directly into the data science workflow, allowing students of data science and experienced data scientists alike to see, experience, and discover the worldly dimensions of their practice.
The tool operationalizes what we understand to be the responsibility of data scientists: to support flourishing and justice. This means supporting the well-being of communities whose data is engaged or whose lives are affected by data science work as well as promoting collaboration and expanding the boundaries of the research community itself. We also recognize that sound workflow is essential to the ability of data scientists to realize this responsibility (Markham Citation2006, Markham, Tiidenberg, and Herman Citation2018). We use the word “ethos” in the title of the tool to draw attention to the interplay between ethics and practice that is at the heart of our project, and to distinguish it from the many lifecycle frameworks that have already been proposed but do not center ethics to the same extent. The lifecycle approach presents an effective means of engaging questions about human flourishing and social justice throughout the process of data science work, actively taking into consideration and inviting the feedback loops and iterations that are part of real-world projects. As such, the tool aims to operationalize ethics, instead of merely suggesting ethical principles—a need that researchers of computing ethics have identified (Morley et al. Citation2020; Ayling and Chapman Citation2021). It is our aspiration that the Ethos Lifecycle will contribute constructively to the development of a data science ethos that recognizes a responsible workflow in which practitioners engage with the human and social dimensions of data science.
This article introduces the tool by presenting the theoretical foundations and the normative commitments from which it arose and which it seeks to promote. We present the advantages of building the tool on the basis of the data science lifecycle and discuss in detail the social science concepts—positionality, power, sociotechnical systems and narratives—that serve as its main analytical lenses. To give an example of the ethical insights the tool can open up about a real-world data science project, we show what can be learned about a single stage of a project to use mobile phone data for population-level analysis.
2 Lifecycle as a Tool for Teaching Responsible Workflow
Approaches to teaching ethics of technology are frequently divided into “stand-alone” or dedicated courses on ethics and societal contexts and “embedded” or “infusion” (National Academy of Engineering Citation2016) approaches where students learn about the ethical contexts in the process of learning technical practices (Saltz et al. Citation2019; Fiesler, Garrett, and Beard Citation2020; Hoffmann and Cross Citation2021). Scholars who study the effectiveness of these different approaches have found instruction that systematically embeds engagement with ethics into technical practice to deepen students’ understanding of the ethical stakes involved in their work beyond what a “stand-alone” course can provide (National Academies of Science, Engineering, and Medicine Citation2018). One specific advantage of embedded instruction is that it helps students realize what they can do to influence the social consequences of their technical products (Verbeek Citation2006; Christiansen Citation2014; Joyce et al. Citation2018; Markham, Tiidenberg, and Herman Citation2018; Grosz et al. Citation2019; Baumer et al. Citation2022). In the neighboring field of computer science, educators who have experimented with teaching “responsible computing” report that students learn more effectively when they are introduced to ethical issues at the beginning of their study of computer science and are given opportunities to deepen and build on their knowledge throughout their studies (Mentis and Ricks 2021). In order to support the educational goal to “produce students who have fully integrated ethics into their understanding of statistics and data science” (Baumer et al. Citation2022), universities are experimenting with approaches to embed ethics education across data science majors and minors, from intro level to capstone courses and all the courses in between (see, e.g., the UC Berkeley Human Contexts and Ethics program in the Division of Computing, Data Science, and Society and the Smith College data ethics curriculum described in Baumer et al. Citation2022).
From the authors’ experience designing and teaching embedded ethics of data and computing, we see that successful approaches are defined by two key qualities: systematic instruction and close interplay of technical and social learning content. Teaching data and computing ethics systematically means planning a programmatic curriculum that builds on itself, as opposed to being ad-hoc and piecemeal. In contrast to abstract or unrelated lessons, ethical lessons in this curriculum are relevant to the specific technical material students are learning and take their full meaning only in this context. In the ideal case, both the technical and the ethical elements of the lesson require its counterpart in order to be complete. We refer to this as the interplay between the technical and social components of the lesson. The Ethos Lifecycle is designed to support these ideals of systematicity and interplay in data ethics education. The success of including instruction on ethics alongside technical instruction through a student’s educational trajectory requires, however, that each component of ethical instruction be itself systematic and well-integrated.
Teaching the meaning and practice of responsible data science at every stage of the data science lifecycle can be a bedrock of systematic and “interplayed” ethical instruction. Data scientists have discussed the importance of the lifecycle concept for effective and responsible data science practice (Keller et al. Citation2020; Stodden Citation2020). For example, Keller et al. developed a “data science framework” in the form of a lifecycle that newly emphasized the importance of data discovery and ethical considerations at every stage as a way to help students and professional data scientists move from doing “simple data analytics” to “actually doing data science” (Keller et al. Citation2020). Similarly, Stodden described how the data science lifecycle as an “intellectual framing” can help not only to train the next generation of researchers and scientists “in the deeply computation and data-driven research methods and processes they will need and use,” but also to “advance [data-centric] research and discovery across disparate disciplines and, in turn, define data science as a scientific discipline in its own right” (Stodden Citation2020, p. 58).
As Stodden indicates, the lifecycle is as important for building data science as a field as it is a helpful teaching tool. Many introductory courses in data science orient the next generation of practitioners to the data science professional community by teaching them workflow stages from problem framing to communication and results (e.g., UC Berkeley’s core upper-division data science course, Data 100: Principles and Techniques of Data Science (ds100.org) teaches the lifecycle in early lectures). The workflow is also used in programs that introduce students to real-world research (e.g., University of Virginia’s Data Science for the Public Good summer program) to help them navigate what it means to carry out a data science project from beginning to end. Overall, the data science lifecycle is a helpful tool to identify best practices of data science and support it to be recognized as a science of its own.
Scholars have also focused specifically on the merits of the lifecycle for addressing and teaching data ethics (Janeja Citation2019; Keller et al. Citation2020). The lifecycle is useful for data scientists to “develop ethical critical thinking while analyzing data” (Janeja Citation2019) and to “implement an ethical review at each stage of research” (Keller et al. Citation2020). Both Janeja and Keller et al.’s approach shows how ethical reflection paired with each stage of the lifecycle can help to keep important human questions close to the technical material and design decisions at hand. To support this process, Janeja identifies the kinds of ethical issues that surface at each stage of the lifecycle, such as issues of privacy in the data collection stage or selection bias in the data preprocessing stage. Keller et al. offer a checklist that asks questions of researchers at each stage of the lifecycle. For example, in the “Data discovery, inventory, screening and acquisition” stage, Keller et al. explain that it is important to consider the potential biases that can be introduced through the choice of datasets and variables, and suggest that researchers ask whether “the data include disproportionate coverage for different communities under study” (Keller et al. Citation2020).
In these applications of the lifecycle as a tool for teaching responsible data science, we see the lifecycle leveraged as what information scholar and ethnographer of data practice and ethics, Katie Shilton, calls “value levers”: “practices that pr[y] open discussions about values in design and [help] the team build consensus around social values as design criteria” (Shilton Citation2013, p. 376). The lifecycle is an instrument through which students and seasoned data science researchers alike learn where values are at stake in the data science workflow and design. The lifecycle can help build understanding of how design decisions are linked to ethical issues. It gives practitioners opportunities to have conversations with peers and team members about ethics during relevant moments of the data science workflow in ways that can in turn inform their practice.
3 Thinking about the Human at Each Stage
To capitalize on the lifecycle’s ability to serve as a value lever for learning and practicing responsible workflow, the Ethos Lifecycle invites data science students and practitioners to learn to see four crucial dimensions of the relationship between technology and the human: positionality, power, sociotechnical systems, and narratives. Each of these dimensions serves as a metaphorical “lens” through which data scientists can view the contexts and consequences of their technical work. The use of conceptual lenses distinguishes the Ethos Lifecycle from previous work. The lenses, derived from the interpretive social sciences, put into action the recommendation to bring together qualitative and quantitative approaches in data science (Tanweer et al. Citation2021). More than identifying for the practitioner a particular issue or value at stake in a given part of the workflow, such as selection bias or privacy, the Ethos Lifecycle invites practitioners to think critically about what is at stake in terms of positionality, power, sociotechnical systems, and narratives in their work. The lenses offer practitioners a more systematic, flexible, and holistic way to consider the ethical and social stakes of their data science practice, and they support the practitioner to see and work out the dynamic interplay of technical and social factors. As practitioners become accustomed to viewing their workflow through each of these lenses, they develop the capacity to identify issues themselves. Furthermore, the lenses not only focus attention on ethical issues, but help the practitioner to reframe the way that they see their entire practice, from problem framing to solution, to include attention to the human dimensions of data science.
The lenses are operationalized in the Ethos Lifecycle, which is an interactive online tool (in development) that data science students or experienced practitioners can engage with through three different views. One view () shows six stages of the data science workflow process: Problem identification; Data discovery; Exploratory data analysis; Use of analytical tools (Modeling); Interpreting, drawing conclusions and predictions; Communication, dissemination and decision. We decided on these six steps by beginning with a simplified lifecycle that is taught to UC Berkeley undergraduates in the course Data 100: Principles and Techniques of Data Science (ds100.org) and expanding it with the lifecycle developed by Keller et al. (Citation2020).Footnote1 The resulting six step-lifecycle captures the distinct high-level stages that most projects in data science must go through while keeping a level of abstraction that is useful pedagogically and highlights the connections of the lifecycle to real-world interests and consequences. Users of the tool read about the kind of work that transpires at each stage of the lifecycle and consider questions they can ask of themselves and their colleagues about the human contexts and consequences at each stage informed by each of the conceptual lenses.
Fig. 1 Six stages of the data science workflow, illustrated for the Ethos Lifecycle online tool. Image by Eva Newsom.
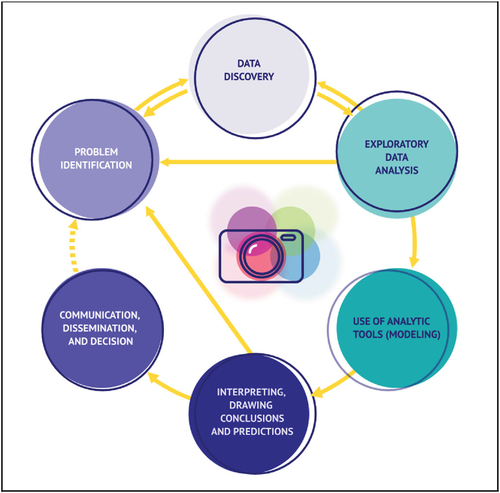
Another view invites users of the tool to familiarize themselves with definitions, examples, and questions for each conceptual lens. A third view presents three different real-world inspired case-studies for users of the tool to walk through the stages and lenses together. By interacting with the tool through these different views, data science practitioners learn how to see through the conceptual lenses, discover examples of how they can be applied to data science projects, and practice applying them to different stages of the data science workflow. Once data scientists have become familiar with the stages, conceptual lenses, and examples, they can use the lifecycle tool to think through their and their collaborators’ assumptions and choices in the context of a specific data science project.
Working with the Ethos Lifecycle provides the data science student and practitioner with opportunities to learn about responsible data science practice and develop ethical habits. In this sense, the Ethos Lifecycle is a tool for learning, but it can also be used in situations when practitioners want to assess a project. In these situations, the open-ended nature of the tool supports practitioners to reconceive the task of assessment as itself a learning process that builds the ethos of the data scientist to consider the human dimensions of their practice in their future work.
4 What makes the Cycle Go Round: Theoretical Foundations and Normative Commitments
Like any tool, the Ethos Lifecycle is a product of a specific set of disciplinary perspectives and normative commitments. First, and most importantly, it is animated by an understanding of data science as a world-making practice. This orientation emphasizes that data science emerges from and shapes the social world. To discover what is at stake ethically in data science, one must first be concerned with the forms of social order that are engendered and supported by data science practice. This includes questions about whose visions of social order are privileged and enacted, and what forms of life and relationships the processes and products of data science enable.
Second, the lifecycle is motivated by the normative project of supporting the visions and experiences of those who are disempowered in today’s technological societies. This normative commitment to justice derives from the scholarly fields that we are practitioners of and that underlie our world-view, including Science, Technology and Society (STS), critical theory, critical race theory, and feminist theory. These fields foreground uneven power relations and social (and sociotechnical) structures that pervade human life and use the analysis of these to explain the way things are. Rather than conceiving of ethics as being first about avoiding harm, the world-making perspective on ethics and justice begins by asking what world is built by data science, for whom and by whom (for an example of an approach to data ethics that centers avoiding harm, see Janeja Citation2019). The world-making perspective on ethics builds upon the relational theory of ethics in philosophy (Lévinas Citation1969; Ricøeur Citation1992), moral anthropology (Lambek et al. Citation2015), and recent work being developed by philosophers of technology (Zigon Citation2019) and scholars who bring African philosophy perspectives on data and computing ethics (Mhlambi 2020; Birhane Citation2021; Wodajo and Ebert Citation2021). These diverse traditions of ethical thought and analysis share the view that ethics is a quotidian practice of being human that takes place in the midst of human relationships, with the attendant responsibilities and duties that each person has to the other. Ethics is thus dynamic, context-specific, constitutive of human selves and societies, and co-evolves with science and technology. The lifecycle, with its attention to practice and process and the feedback between different stages and actors becomes a natural tool for engaging ethics conceived as relational. These two foundational orientations and commitments profoundly shape the Ethos lifecycle in ways we describe below.
Today, many frameworks are available to data science students, researchers, and industry professionals seeking to learn about and engage with the ethical dimensions of their work. Readers may be familiar, for example, with the Open Data Institute’s Data Ethics Canvas, the UK Government’s Data Ethics Framework, or the Ethics Framework by the Machine Intelligence Garage, to name just a few. The lifecycle-based approaches by Janeja and Keller et al. discussed above or the Toolkit for Centering Racial Equity Throughout Data Integration by Actionable Intelligence for Social Policy are also tools for engaging ethics. The variety and large number of approaches to data and computing ethics have inspired studies to map them and understand their similarities, uniquenesses, methods of implementation and varied utility to different audiences (Jobin, Ienca, and Vayena Citation2019; Morley et al. Citation2020; Ayling and Chapman Citation2021). What distinguishes the Ethos Lifecycle are the combination of the commitment to justice, the orientation to data science as world-making, and a primary focus on supporting data science novices as much as seasoned practitioners in the process of learning about ethical contexts and developing a responsible ethos (in contrast to an evaluative or accounting mode).
4.1 Data Science as World-Making
The lifecycle is informed by what we call a “world-making” perspective on data science, and technical practice more generally. In the “world-making” perspective, when people engage in scientific study or the development of technology they are simultaneously engaged in shaping the social world. They are shaping the future of society at the same time as they are creating knowledge or building a specific device or algorithm for a specific purpose or use. The view of data science as world-making is consistent with how social scientists and philosophers describe data as “made” and “relational,” that is, phenomena become data as a result of human work in the context of specific purposes and processes of inquiry (Edwards et al. Citation2013; Leonelli Citation2015, Citation2019, Citation2020). If we accept that the very object of data science—data—are made through the web of interested relationships among researchers, communities, instruments and institutions, we can see how the practice of producing insights and tools based on these relational objects ripples through and reshapes these relationships. Our goal is that the lifecycle not only support students and experienced practitioners to recognize the world-making implications of their data science work, but also to support their ability to analyze the worlds they contribute to making: to recognize and engage what critical theorist Donna Haraway calls the “thickness of the world” (Nakamura 2012).
To analyze the thickness of the world—such as the kinds of relationships that exist between people, the formal and informal rules according to which people behave, the values and aspirations that people hold, and the manner in which life is organized materially—requires engaging with the technologies that form the backbone of contemporary life. We recognize that the social sphere is not merely the place from which data science derives its questions, power, and legitimacy and to which it contributes its conclusions and products. Rather, we view data science and the social world to be mutually constitutive. In STS, this relationship between technology and society is often referred to as “co-production.” Co-production says that our ideas about what the world is—as a matter of fact—and what the world ought to be—as a matter of social values—are formed together (Jasanoff Citation2004). Instead of privileging either the role of social forces or technological forces as explanations for the way that the world is, the co-productionist approach considers the symmetrical interplay between social and technical forces in weaving the fabric of the world. These co-evolving forces can be observed and discovered and become sites of deliberate work and transformation.
Co-production gives us insight into how the prevailing conditions of life and authorized visions of the future come to define technological design and deployment, and how these technological designs shape what is deemed possible and desirable for society. As a result, we see that technology is not alone responsible for determining the state of human oppression today across axes of race, gender, and class nor does it alone determine the possibility for social justice. And yet technology plays a constitutive role in defining the specific ways in which empowered and marginalized peoples relate to one another, forge their identities and shape their lives. In other words, we are able to see how certain forms of life and technologies cohere together, and how other forms of life are written off as superfluous, unvalued, or impossible. In contrast to these coherent ways of life, alternative visions and resistances to the existing order are thrown starkly into view. By providing insight into the differently possible, the co-productionist approach supports people to make social change.
Another advantage of the world-making view of data science practice is the way that it draws attention to and helps to account for the technical element in the modulation of power. This sensitivity to the socio-technical nature of power enables the Ethos Lifecycle to be a helpful ally to data science initiatives that aim for justice.
4.2 Commitment to Justice
A key motivating factor in our project to build the Ethos Lifecycle tool is the normative project to support the visions and experiences of those who are disempowered and actively harmed in our technological societies. Our pedagogical approach embedded in the Lifecycle grows out of a concern that originates, in part, in STS and critical theory, about the hegemonic power that technologists wield over the lives of others, especially historically and structurally marginalized populations.
Because of this normative orientation, the Ethos Lifecycle can be an ally of anti-racist approaches to pedagogy or education projects animated by the goal of abolition (Freire Citation1970 [2005]; Riley Citation2003; Hooks Citation2014; Kishimoto Citation2018). These modes of critique and action expose the power over knowledge and ways of knowing held by whites, and the inequity and injustice facing marginalized communities at the hands of those holding dominant political power. These critical approaches center the place and identity of people, analysis and critique of power, and attention to dominant and alternative narratives in the context of particular dynamics of the technology and society relationship.
The Lifecycle contributes to anti-racist pedagogy a critique of another fault line of power: that of technology expertise in relation to the lay or the nontechnical. The Lifecycle joins a growing set of scholarship and activism (Benjamin Citation2019; Nelson et al. Citation2020) to develop a crucial dimension of inquiry into how technological power is entangled with the prevailing structures of white supremacy. The technical dimension of human action matters profoundly for how people are able to differentiate among one another so as to govern and subject one another to hegemonic visions and forms of life (Bowker and Star Citation1999; Gibran Citation2010; Browne Citation2015; Murphy Citation2017; Koopman Citation2019). The power of technology, when carefully examined and accounted for in contexts of social structures, can be an effective component of human action that aims at justice (Benjamin Citation2019; Hamid 2020). The Ethos Lifecycle prompts data science practitioners to acknowledge the power they wield and how it informs their practice, and examine for what purposes, on behalf of whom with what visions of the good they deploy it. We recognize that the Ethos Lifecycle is a partial and imperfect intervention. This is in no small part because it was designed as a tool to be used primarily by a technical elite (i.e., instructors and students at higher education institutions) rather than by marginalized peoples and communities. Still, it is our sincere hope that the lessons conveyed by the tool about the power of technology will be useful to all people trying to deploy data science in ways that do not exploit and oppress. We see the Ethos Lifecycle as complementing ongoing efforts to more inclusively develop and deploy technology (Costanza-Chock Citation2020; D’Ignazio and Klein Citation2020; Nelson et al. Citation2020), and hope that by engaging with the Ethos Lifecycle, practitioners will reconstruct an ethos of data science that draws more widely the lines of data science community and responsibility.
These two core elements of the theoretical origins and commitments of the tool—the world-making perspective and the commitment to justice in a world where data and computing technologies play a profound role in defining and contesting justice—give shape to the Ethos Lifecycle as a tool. They reinforce the Lifecycle’s focus on the process of design and practice with data science, drawing attention to the ways in which the capacities for just world-making are lodged in the minutiae of technical decisions in the course of the data workflow.
At the same time as the Lifecycle encourages close explorations of the greater human consequences and contexts of data science practice, it also keeps aligned with a systems-based approach. By this we mean that the Lifecycle helps to account for the way that the social world is the product of underlying causes and forces that interact with one another in complex ways and span time and socio-technical actors. The systems-based approach recognizes the importance of individual action and decision, but also considers its limitations in broader social, cultural, and technological contexts.
Our theoretical orientation also relates to the Ethos Lifecycle as a tool of teaching responsible workflow. Through learning to recognize and practice responsible workflow with the Lifecycle tool, students become aware of their power to intervene in socio-technical reality to shape it consciously and responsibly. Brazilian activist and educational theorist Paulo Freire described how a liberatory relationship between a person and the world requires that people see the world not as a static and closed entity, which they must accept as is and live according to established dictates, but as “posing of the problems of human beings in their relation with the world” (Freire Citation1970 [2005], p. 79). By giving data science practitioners the conceptual lenses through which to consider the origins and consequences of their data science action in the context of the broader social world, data scientists can acquire this freeing awareness and relationship to the world in which they practice.
5 Four Conceptual Lenses
Critiques of data-intensive technologies and methods have laid bare their many ethical shortcomings and challenges (O’Neil Citation2016; Barocas and boyd Citation2017; Hoffmann Citation2019) and highlighted the need for critical humanistic and social scientific thinking to be incorporated into data science (Iliadis and Russo Citation2016; Neff et al. Citation2017; Pink and Lanzeni Citation2018; Selbst et al. Citation2019; Sloane and Moss Citation2019; Bates et al. Citation2020; D’Ignazio and Klein Citation2020; Tanweer et al. Citation2021). The Data Science Ethos Lifecycle does this by applying to each stage of the data science workflow four core humanistic concepts: positionality, power, sociotechnical systems, and narratives. We refer to these concepts as “lenses” because each represents a theoretical stance that brings into view a particular set of problems and prefigures certain kinds of questions about the social world. The lenses draw the practitioner’s attention to the people and web of relations who inhabit it (positionality), the force that defines knowledge and human relations (power), the entwinement and nestedness of social and technical factors (sociotechnical systems), and the promises, visions, imaginaries, and stories that animate human-technology projects (narratives).
Below, we present each lens in turn and then apply it to a case study of a data science project. Our aim is to show how the lenses can support practitioners to conduct an ethical analysis of data science practice. We take a hypothetical case study of a data science project that seeks to use mobile phone records to estimate populations and economic status. Throughout this example, we’ll think specifically about the Data Discovery stage of work. Focusing on just one stage of the data science lifecycle allows us to give readers a taste of the analytical insights the Ethos Lifecycle tool can support. While we are not examining the real-life work of any particular individuals, it is worth noting that numerous examples of extant research take similar data science approaches to the problem (e.g., Deville et al. Citation2014; Blumenstock, Cadamuro, and On Citation2015).
Enumerations of populations and their economic status are used for many important purposes, such as determining electoral representation, allocating tax dollars, and deploying public health interventions, to name a few. Most typically, populations are measured through a census—a nationally-organized complete count of all residents according to where they live that usually includes their income status, gender, and race or ethnicity. Many countries conduct a census at regular intervals, such as every 10 years. But censuses are very expensive and time consuming, so in recent years, researchers have been experimenting with alternative sources of data collection and analysis that could more dynamically, quickly, and cheaply estimate populations and economic status. In particular, mobile phone records are being explored as a data source that could allow governments to infer these measurements (Deville et al. Citation2014; Blumenstock, Cadamuro, and On Citation2015). This approach is seen as particularly promising in countries where census-taking is hindered by a lack of resources or conflict.
While this offers a promising path forward, it is not without ethical challenges. Here, we explore some of the ethical issues that surface when trying to estimate population size and economic status using mobile phone records if we adopt the lenses of positionality, power, sociotechnical systems, and narratives during the stage of Data Discovery. Recall that Data Discovery includes activities such as identifying potential data sources and filtering data, as well as cleaning, transforming and integrating data into a usable dataset.
5.1 Positionality
The concept of positionality () accounts for the ways in which knowledge and actions are inescapably informed by a person’s relative social circumstances, including but not limited to their experience of gender, race, class, culture, education, and status. An intersectional perspective (Crenshaw Citation1991) foregrounds how the overlap and interaction of these various axes of difference influence the distribution of social privilege and disadvantage. The notion of positionality challenges a pretense implicit in much scientific research that knowledge is the discovery of unquestionable and singular truths. Instead, knowledge is always “situated” within “webs of differential positioning” (Haraway Citation1988, p. 590) resulting from the subjective and embodied experiences that every individual lives through. The totality of these experiences influences what questions one asks, how one goes about answering them, and how one interprets the results of that process. Prevailing scientific norms view such subjectivities as “biases” to be treated as contaminants and eliminated from research through the application of rigorous and uniform methods. Feminist epistemologists, on the other hand, have long argued that subjectivities cannot and should not be eliminated, but rather articulated and accounted for in the course of research. The result need not be paralyzing relativism or solipsism. Instead, an interrogation of positionality gets us closer to a more objective view of the world (Harding Citation1992) by honestly admitting the ways in which our knowledge is always necessarily partial. Attending to our own positionality as data science practitioners and to the positionality of stakeholders in our work means becoming, as Donna Haraway has put it, “answerable for what we learn how to see” (p. 583). Viewing the data science workflow through the lens of positionality prompts a data scientist to ask questions such as:
Fig. 2 An illustration from the online Ethos Lifecycle tool (in development) of the concept of positionality inside of a metaphorical camera lens. Image: Anika Cruz and Eva Newsom.
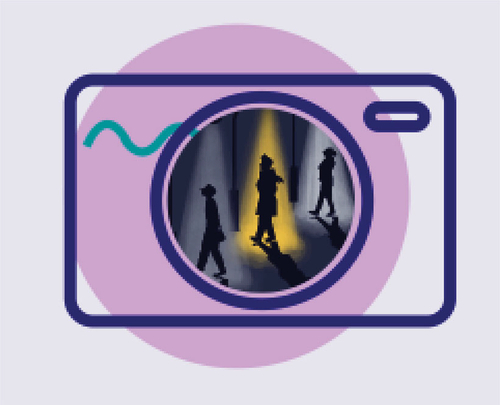
What assumptions do I bring to the work based on my personal life experiences?
What are the opportunities and limits of my own expertise?
What identities are represented among my data subjects, partners, and stakeholders, and how are these identities linked to privilege and disadvantage?
Consider using the lens of positionality to examine the Data Discovery stage of a project using mobile phone records to estimate population size and economic status. In this example, an important part of data discovery is thinking about privacy concerns for people represented in the data. With sensitive information like phone records, researchers typically perform a step during data discovery to prevent individuals from being identified—either by anonymizing the data, aggregating it, or introducing noise into the dataset. But what culturally derived assumptions are made when taking any of these typical approaches to privacy? If you come from a culture that values individual liberties over collective well-being, are you perhaps assuming that the most important thing is preserving the anonymity of individuals while overlooking the harm that can be done to segments of the population if their locations are revealed? For example, could a government use this method to monitor an oppressed minority that is geographically concentrated, even if individuals within that population cannot be reidentified? Upon understanding that their notion of ethics, privacy, and identity are culturally derived and situated, data scientists involved in this case will need to decide how this realization should affect their practice. For example, maybe they choose to abandon the project if the state-of-the-art approaches to de-identification at their disposal can do nothing to protect the privacy of a persecuted ethnic minority in the aggregate. Or perhaps they have the opportunity to work with a dataset from a different country where that particular concern is less salient.
5.2 Power
If positionality draws a practitioner’s attention to the unique situatedness of human actors in the data science process, then the lens of power focuses on the capacity of these actors to exert agency in shaping the world around them. We understand the concept of power () to mean the asymmetric capacity to structure or alter the behavior of others, and are concerned with the ways power is wielded by both people and technologies in the pursuit of knowledge. For Michel Foucault, power is not only concentrated in the hands of those who wield blunt instruments of coercion and control; rather “power is everywhere” (1990 [1976], p. 93)—a force field that constitutes human subjectivities, identities, and knowledge. Diffused and embedded within everyday discourses and practices, power anchors our understandings of what is normal, appropriate, and right (Foucault Citation1990 [1976]; Koopman Citation2017). Knowledge and power are not just intimately connected but inseparable, such that they produce a “regime of truth” (Foucault Citation2008) that justifies and perpetuates a status quo in which some humans have more agency than others. Interrogating power, therefore, means asking how regimes of truth are shaped, who gets to produce knowledge, and who is the subject of knowledge. Technology is a crucial conscript in the formation and perpetuation of power (Jasanoff Citation2016). For example, modern data-intensive and algorithmic technologies alternately render minoritized populations either hypervisible or invisible and reify stereotypes that obviate both nuanced understanding and fair treatment of all people (Benjamin Citation2019). Being attuned to the dynamics of power in the course of data science practice supports data scientists to identify how their project will redistribute or transform the field of power for stakeholders and the broader society in which the project will be deployed. Data science practitioners can interrogate their project from the perspective of power by asking questions such as:
Who has influence over the data science work and who does not have influence even while being affected by it?
Who gets to decide if the work is valid or good and who may dispute that conclusion?
Whose power is increased through the work and whose power is diminished?
How does the transformed field of power reorient identities, relationships, and life chances?
In the case of using mobile phone records to estimate population size, location, and economic status, it is important to remember during the Data Discovery stage that for-profit businesses have the power to decide what data they will share, with whom, and under what conditions. If a company has made mobile phone records available to you as a researcher, you will want to ask yourself what their motivation is for doing this. Are they acting altruistically, or do they have something to gain politically or financially by sharing their data from a particular country or city? Are they, perhaps, trying to win favor with a governmental body that is responsible for regulating them? Are they hoping to burnish their image by doing something that is “good” for the world? We also want to think about who does not have power in this example. Do mobile phone customers know that their data is being used in this way? Do researchers in this space have an obligation to provide information to phone customers about their research or obtain consent from them? Finally, the power lens draws attention to the ways in which power is not only gained and lost, but re-configures the very playing-field of human relationships. In this case, we can see how the capacity of data scientists to estimate the population without relying on the census mechanism further blurs the distinction between citizen and phone user/consumer.
When responding to these concerns in practice, the data science team has a wide range of options. They may decide they do not trust the data provider enough to use their data at all. Or they may partake in the project, but not without negotiating the terms of the data sharing agreement to ensure that it aligns with their values. They may want to conceal the name of the data provider so as not to be complicit in the company’s public relations goals, or conversely they may determine it is crucial to disclose the name of the provider for the sake of transparency and accountability. They may arrange to have the company contact customers and inform them of the research, or they may prominently post about their study methods on a public-facing website so that mobile phone customers or journalists can better understand the research. And all the while, they must question whether and how any of these steps actually make their work more ethical. For example, if the data subjects in these mobile phone records come from rural communities with little internet access in a country where few people speak English, then posting information in the English language on the website of a U.S.-based institution probably does little to empower their data subjects.
5.3 Sociotechnical Systems
The concept of sociotechnical systems () concerns the ways in which people and technologies are irrevocably entangled across different scales such that human agency is intertwined with technological devices, practices, and infrastructures. Positionality encouraged us to think about the specificity of the human actor involved in the data science process as practitioner or subject. Power made it possible to investigate the actors’ differentiated capacity to act and inform one another’s relative position. The lens of sociotechnical systems adds the crucial dimension of thinking about how human and technical capacities to act are intertwined. This third lens invites practitioners to interrogate the sociotechnical systems in which one’s project intervenes, which may involve considering the systems upon which the project depends in order to function and also how the output of one’s analysis can instantiate novel sociotechnical arrangements. The intertwining of human and technical agency introduces an even more consequential element into the data scientist’s analysis: to consider how human and technical actors are mutually constitutive of one another (Jasanoff Citation2004; Sawyer and Jahari Citation2014). In other words, the lens of the sociotechnical system most directly mobilizes the concept of co-production that is a theoretical foundation of the Ethos Lifecycle. Broadly, it encourages practitioners to consider how their questions, processes and outputs are shaped by the social milieus in which they are produced and how they simultaneously shape the social world by, for example, occasioning new routines and practices (e.g., Akrich Citation1992; Latour Citation1992; Orlikowski Citation2000), affording certain behaviors while constraining others (e.g., Hutchby Citation2001), or distributing agency and accomplishment (e.g., Hutchins Citation1991). Thinking in terms of sociotechnical systems probes how technological artifacts reflect and reproduce the social contexts of their production, how human flourishing can be considered in the design of technology, and how accountability can be achieved when risks and responsibilities are distributed widely and opaquely across human and nonhuman actors. In recent times, failure to think sociotechnically has been characterized as a “trap” that results in ineffective or harmful technologies (Selbst et al. Citation2019) and misplaced responsibility (Elish Citation2019). Data scientists can scrutinize their workflow through the lens of sociotechnical systems by asking questions such as:
What social constructs or assumptions are embedded in my data and presented as fact?
What actions (and whose actions) are enabled by data science tools and what actions are proscribed or hindered by them?
Who or what is held responsible when something goes wrong?
How does my data science project seek to contribute to the modification of an existing sociotechnical system?
When applying the lens of sociotechnical systems to the mobile phone records case, it is helpful to think about how the technical system that generated the mobile phone network data is entwined with the lives of real people who act in varied, inconsistent, and sometimes surprising ways. This means that as a researcher, one cannot necessarily make straightforward assumptions about what the data generated by the system represents. For example, is your first inclination to assume that a mobile phone record represents one unique individual? In a place like the United States, it may be common for most people to have a single phone that is used by themselves alone. But this is not the case in many parts of the world. In some places, it is common for a whole household to share one mobile phone, for one person to use multiple phones, or for one phone to work with multiple SIM cards. How can you account for this variety when deciding what the variables in your data represent during the data discovery phase? And if you do not address this social complexity and variation, what are the repercussions in the world? If you vastly over- or under-estimate a population, could that lead to people being deprived of important resources or democratic representation? In response to realizing that such concerns exist, the data scientists on this project would likely take steps to gain more insights into how the data was produced and what it means in the local context. This might require negotiating ongoing access to officials at the mobile phone company who can provide further insights or metadata as the need arises. Or it might entail first consulting or conducting qualitative research that explores how people use mobile phones in the local context of relevance. Even upon going to these lengths, the practitioner may determine that the data simply are not suitable for answering their questions given the meaning of the data in its specific sociotechnical context.
5.4 Narratives
The concept of narratives () refers to prominently circulated or widely held beliefs about why the world is as it is, what changes are possible and worthwhile, and what should be done to achieve a desired future. Narratives are profoundly important in human life for individual and collective sense-making and accounting (Ricøeur Citation1988; Butler Citation2005; Meretoja Citation2018) and are constructed around any and every facet of existence. In the context of the data science workflow, we are particularly concerned with how narratives garner support for technological and scientific interventions, and how, in turn, technologies underwrite visions of futures worth wanting and working toward (Jasanoff and Kim Citation2015). For example, researchers have found that data are commonly portrayed as an abundant resource that may be exploited to improve nearly any aspect of life by providing convincing analysis that sheds insight onto complex social or natural phenomena, therefore, preparing the way for evidence-based decisions (Puschmann and Burgess Citation2014; Stark and Hoffmann Citation2019). The tight synergy implied in this narrative between the vision of social well-being, reasonable decision-making, and data gives data science significant explanatory power and world-building capacity. This same coupling tends to also erase or relegate to obscurity and falsehood perspectives, explanations, and visions that do not conform to accepted narratives. For example, Abebe et al. (Citation2021) discuss how in the context of Africa, narratives celebrate the potential of using open and shared data to do “good” while marginalizing African stakeholders, ignoring historical and cultural complexity, and perpetuating deficit thinking about local conditions. The concept of narratives also allows us to attend to the more immediate story-telling capacity of data technologies (Nolan and Stoudt Citation2021). Data scientists’ ability to construct persuasive data-based narratives about the world help to frame problems and solutions in specific ways, authorize certain kinds of actions in place of others and construct a narrative of human flourishing that is dependent upon data. Every data science project is animated by narratives about data’s value and utility to the problem at hand, and simultaneously contributes to underwriting and rewriting these narratives. Because of this central role of narrative in data science practice, it is important to ask about the place and significance of narrative at each stage of the workflow. The following questions can start data scientists on the path to assess the narrative dimensions of their practice:
What narratives motivated and informed the creation of this data?
What underlying beliefs inform the idea that data science approaches are appropriate for the question or problem?
What kind of change is the work implicitly or explicitly intended to bring about?
A project to study populations using mobile phone data is likely animated by a narrative that celebrates the ability and innovativeness to use readily available data in place of the painstaking process of data collection involved in conducting a census. In applying the narrative lens to this project, it is important to define this narrative, which includes identifying what specifically makes this a compelling project, according to whom, and against what alternatives. Then it is necessary to consider criticisms of this dominant narrative. For example, some scholars have expressed concerns about efforts to use alternative sources of data in lieu of census data. Richard Shearmur (Citation2015) argues that many researchers are “dazzled by data,” meaning that they have bought into a utopian narrative that claims newly available digital traces of human activity, better known as “Big Data,” will help to answer previously unanswerable questions about the world:
The marvels, infinite possibilities and sheer newness of Big Data are contrasted with the staid and limited information that—it is thought—can be gleaned from the census. For example, Facebook can use its information to track the formation and dissolution of networks in real time, and cell phone companies can map the movements of their customers: can the census do that? (2015, p. 965).
Shearmur argues that the existence of this utopian narrative has contributed to decisions by governments like his in Canada to disinvest from collecting census data. This concerns him because data collected during a census is “authoritative, open to scrutiny, representative of the entire population, and resting on slowly evolving and relatively consensual definitions”—all things Big Data gathered from mobile phone records can never be because it is inherently about customers and markets, not citizens. Upon recognizing the existence of the “dazzling” utopian data narrative, the data scientists involved in this hypothetical project might respond in a number of ways. Perhaps they reconsider whether they should embark on the project at all, or perhaps they find ways to complement their analysis with more traditional administrative data, or perhaps they choose to be assiduously circumspect and cautious when interpreting their findings and disclosing the limitations of their study.
To summarize this example of using the Ethos Lifecycle, applying the conceptual lenses of positionality, power, sociotechnical systems and narratives at the stage of Data Discovery raised fundamental questions about the goal of using mobile phone records for demographic research. Each concern elicited myriad possibilities for how to proceed (of which we have articulated only a few). Importantly, in each case, not proceeding with the work was one of the options to be considered; recognizing that some projects may be fundamentally misguided and impervious to ethical repair is an important lesson that all technologists must learn (Barocas et al. Citation2020). But assuming that the work goes on, the greatest advantage of the Ethos Lifecycle lies in its potential to support practitioners in generating creative ideas for remediating data science practice themselves, rather than working through a checklist or decision tree that someone else has developed.
Each of these lenses offers a crucial analytical view of the world and of the role of data science in it. The Ethos Lifecycle invites data scientists to apply each lens to describe the “thickness” of the socio-technical world, as both the immediate context of their practice and site of transformation by their practice. We have introduced each of the four conceptual lenses separately, both for the sake of clarity and because we believe that each one on its own lends an important perspective to the ethical interrogation of data science practice. Yet these concepts are complementary and to some extent co-constitutive of one another, such that when applied to the phenomenon of data science, any one concept cannot be fully articulated or understood without drawing on the others. In we show some of the ways in which these four concepts are interdependent. On the whole, this conceptual framework offers a holistic way to analyze ethics in data science because it accounts for how asymmetries in agency (power) intersect with the multiplicity of human experience (positionality) within a web of complex technologically-mediated relationships and institutions (sociotechnical systems) that are sustained by dominant discourses about how the world works and what futures are worth pursuing (narratives).
As data scientists consider each lens through the course of their workflow, they may notice certain lenses yield more insight than others. The capacity of a lens to unlock opportunities for reflection and open up choices depends upon the specific data science task or project and on the stage of the lifecycle. For example, the positionality lens can prompt reflection on the practitioner’s own positionality in relation to the work during the Problem Identification stage of the lifecycle, or draw attention to the positionality of the data subjects in the research during the Data Discovery stage. Then, in the modeling stage of the lifecycle, the positionality lens might seem less salient than the sociotechnical systems lens for probing questions about the qualities of the chosen model. Similarly to how specific contexts, people, and institutions produce specific types of research problems and solutions in data science, so do the lenses of the Ethos Lifecycle open up recombinable and inexhaustible points-of-view on the human and social dimensions of data science practice. While some lenses may prove more fruitful than others for certain projects, questions of positionality, power, sociotechnical systems and narratives are always at play in consequential ways in data science.
6 Conclusion
This article defines responsible data science to include considering the human dimensions of data science practice at each stage of the workflow. The Ethos Lifecycle tool that we have described, and which is being currently built as an online interactive tool for students and researchers to use, supports practitioners in this task by putting into action qualitative interpretive social science thinking. As with any tool, the Ethos Lifecycle intervenes in the sociotechnical world, and this is by design. Our intention is for the Ethos Lifecycle tool to help shape the data science ethos, such that practicing data science includes, at its heart, generating insight into the dynamic place of the human in data science and of data science in the making of human identities, livelihoods, and ways of knowing.
This project requires that the tool remain flexible and evolving in relationship with the communities who choose to use it. We are currently exploring how to put the tool into practice in data ethics courses for students as well as in communities of scientific researchers and industry professionals interested in the human and ethical contexts and consequences of their work. In the initial stages of the tool’s deployment in these communities, we feel it would contribute positively to the development of responsible data science if:
practitioners who work with the tool are able to discuss issues of positionality, power, sociotechnical systems and narratives with their colleagues and communities of practice involved in their work;
practitioners feel that working with the tool adds valuable insights about the human dimensions of their workflow that they can act upon in various stages of the lifecycle. For example, by revising their initial questions, choosing a different method of analysis, or informing how and to whom they provide their conclusions;
the boundaries of data science practice and practitioner community is enlarged, such that people in communities in which and with whose data the practitioners work are empowered to meaningfully engage with practitioners and co-shape the research questions and projects.
As we work with a wider circle of researchers, educators, and data science practitioners in the public and private sectors to design the online interactive tool and develop more real-world case studies for it, we are committed to reflect upon and deepen the theoretical foundations and normative commitments that we describe in this article. When interpretive social science thinking is in interplay with the inferential and computational thinking that today is recognized as data science (Adhikari, DeNero, and Jordan Citation2021), then people can truly have a science that supports the making of just human-technology futures.
Academic Data Science Alliance, Hopewell Foundation;
Acknowledgments
This article describes an interactive Ethos Lifecycle tool that is being developed by the authors and members of the Academic Data Science Alliance (ADSA) Ethics Working Group. We wish to thank in particular Stephanie Shipp, Cathryn Carson, Micaela Parker, Steve Van Tuyl, Maria Smith, Tiana Curry, Anna-Maria Gueourguieva, Carlos Ortiz, Eva Newsom and Anika Cruz who have engaged and debated the ideas presented here and have provided the vision, design and institutional support to put them into practice in the tool. This article benefited from Margarita Boenig-Liptsin’s fellowship at the Paris Institute for Advanced Study (France), with the financial support of the French State, programme “Investissements d’avenir,” managed by the Agence Nationale de la Recherche (ANR-11-LABX-0027-01 Labex RFIEA+).
Additional information
Funding
Notes
1 Currently, there is no standard data science lifecycle with a set number of steps that is accepted by everyone in the data science community. For example, Stodden (Citation2020) discusses a lifecycle with 11 steps, Janeja (Citation2019) uses a lifecycle with 6 steps, but these do not correspond exactly to the steps we have identified.
References
- Abebe, R., Aruleba, K., Birhane, A., Kingsley, S., Obaido, G., Remy, S. L., and Sadagopan, S. (2021). “Narratives and Counternarratives on Data Sharing in Africa,” in Proceedings of the 2021 ACM Conference on Fairness, Accountability, and Transparency, 329–341. DOI: 10.1145/3442188.3445897.
- Adhikari, A., DeNero, J., and Jordan, M. I. (2021), “Interleaving Computational and Inferential Thinking: Data Science for Undergraduates at Berkeley,” Harvard Data Science Review, 3(2). DOI: 10.1162/99608f92.cb0fa8d2.
- Akrich, M. (1992). “The De-Scription of Technological Objects,” in Shaping Technology/Building Society, eds. W. E. Bijker and J. Law, pp. 205–224, Cambridge, MA: MIT Press.
- Ayling, J., and Chapman, A. (2021), “Putting AI Ethics to Work: Are the tools fit for purpose?,” AI and Ehics. DOI: 10.1007/s43681-021-00084-x.
- Barocas, S., Biega, A. J., Fish, B., Niklas, J., and Stark, L. (2020), “When Not to Design, Build, or Deploy,” in Proceedings of the 2020 Conference on Fairness, Accountability, and Transparency, 695. DOI: 10.1145/3351095.3375691.
- Barocas, S., and boyd, d. (2017), “Engaging the Ethics of Data Science in Practice,” Communications of the ACM, 60, 23–25. DOI: 10.1145/3144172.
- Bates, J., Cameron, D., Checco, A., Clough, P., Hopfgartner, F., Mazumdar, S., Sbaffi, L., Stordy, P., and de la Vega de León, A. (2020), “Integrating Fate/Critical Data Studies into Data Science Curricula: Where are We Going and How Do We Get There?” in FAT* 2020 – Proceedings of the 2020 Conference on Fairness, Accountability, and Transparency, 425–435. DOI: 10.1145/3351095.3372832.
- Baumer, B., Garcia, R., Kim, A., Kinnaird, K., and Ott, M. (2022), “Integrating Data Science Ethics Into an Undergraduate Major: A Case Study,” Journal of Statistics and Data Science Education, 30, 15–28. DOI: 10.1080/26939169.2022.203804.
- Benjamin, R. (2019), Race after Technology: Abolitionist Tools for the New Jim Code, Cambridge: Polity Press. DOI: 10.1093/sf/soz162.
- Birhane, A. (2021), “Algorithmic Injustice: A Relational Ethics Approach,” Patterns, 2, 100205. DOI: 10.1016/j.patter.2021.100205.
- Blumenstock, J., Cadamuro, G., and On, R. (2015), “Predicting Poverty and Wealth from Mobile Phone Metadata,” Science, 350, 1073–1076. DOI: 10.1126/science.aac4420.
- Bowker, G. C., and Star, S. L. (1999), Sorting Things Out: Classification and its Consequences, Cambridge, MA. MIT Press.
- Browne, S. (2015), Dark Matters: On the Surveillance of Blackness, Durham, NC: Duke University Press. DOI: 10.1515/9780822375302.
- Butler, J. (2005), Giving an Account of Oneself (Vol. 1st), New York: Fordham University Press. Available at http://discovery.lib.harvard.edu/?itemid=%7Clibrary/m/aleph%7C009831658.
- Christiansen, E. (2014), “From “Ethics of the Eye” to “Ethics of the Hand” by Collaborative Prototyping,” Journal of Information, Communication and Ethics in Society, 12, 3–9. DOI: 10.1108/JICES-11-2013-0048.
- Costanza-Chock, S. (2020), Design Justice: Community-Led Practices to Build the Worlds We Need, Cambridge, MA: MIT Press.
- Crenshaw, K. (1991), “Mapping the Margins: Intersectionality, Identity Politics, and Violence against Women of Color,” Stanford Law Review, 43, 1241–1299. DOI: 10.2307/1229039.
- Deville, P., Linard, C., Martin, S., Gilbert, M., Stevens, F. R., Gaughan, A. E., Blondel, V. D., and Tatem, A. J. (2014), “Dynamic Population Mapping using Mobile Phone Data,” Proceedings of the National Academy of Sciences, 111, 15888–15893. DOI: 10.1073/pnas.1408439111.
- D’Ignazio, C., and Klein, L. F. (2020), Data Feminism, Cambridge, MA: MIT Press.
- Edwards, P. N., Jackson, S. J., Chalmers, M. K., Bowker, G. C., Borgman, C. L., Ribes, D., Burton, M., and Calvert, S. (2013), “Knowledge Infrastructures: Intellectual Frameworks and Research Challenges,” working paper. Available at http://deepblue.lib.umich.edu/handle/2027.42/97552.
- Elish, M. C. (2019), “Moral Crumple Zones: Cautionary Tales in Human-Robot Interactions,” Engaging Science, Technology, and Society, 5, 40–60. DOI: 10.17351/ests2019.260.
- Fiesler, C., Garrett, N., and Beard, N. (2020), “What Do We Teach When We Teach Tech Ethics?: A Syllabi Analysis,” in Proceedings of the 51st ACM Technical Symposium on Computer Science Education, 289—-295. DOI: 10.1145/3328778.3366825.
- Foucault, M. (1990), The History of Sexuality. Volume 1: An Introduction, London: Random House. Available at https://www.penguinrandomhouse.com/books/55036/the-history-of-sexuality-by-michel-foucault/.
- Foucault, M. (2008), The Birth of Biopolitics: Lectures at the Collège de France, 1978–79, Basingstoke: Palgrave Macmillan. DOI: 10.1057/9780230594180.
- Freire, P. (1970), Pedagogy of the Oppressed, New York: Herder and Herder.
- Gibran, M. K. (2010), The Condemnation of Blackness: Race, crime, and the making of modern urban America, Cambridge, MA: Harvard University Press.
- Grosz, B. J., Grant, D. G., Vredenburgh, K., Behrends, J., Hu, L., Simmons, A., and Waldo, J. (2019), “Embedded EthiCS: Integrating ethics across CS education,” Communications of the ACM, 62, 54–61. DOI: 10.1145/3330794.
- Hamid, S. (2020, August 31), “Community Defense: Sarah T. Hamid on Abolishing Carceral Technologies,” Logic Magazine. Available at https://logicmag.io/care/community-defense-sarah-t-hamid-on-abolishing-carceral-technologies/.
- Haraway, D. (1988), “Situated Knowledges: The Science Question in Feminism and the Privilege of Partial Perspective,” Feminist Studies, 14, 575–599. DOI: 10.2307/3178066.
- Harding, S. (1992), “Rethinking Standpoint Epistemology: What is “Strong Objectivity”?,” The Centennial Review, 36, 437–470.
- Hoffmann, A. L. (2019), “Where Fairness Fails: Data, Algorithms, and the Limits of Antidiscrimination Discourse,” Information, Communication & Society, 22, 900–915. DOI: 10.1080/1369118X.2019.1573912.
- Hoffmann, A. L., and Cross, K. A. (2021), “Teaching Data Ethics: Foundations and Possibilities from Engineering and Computer Science Ethics Education,” available at https://digital.lib.washington.edu:443/researchworks/handle/1773/46921.
- Hooks, B. (2014), Teaching to Transgress: Education as the Practice of Freedom, New York: Routledge. DOI: 10.4324/9780203700280.
- Hutchby, I. (2001), “Technologies, Texts and Affordances,” Sociology, 35, 441–456. DOI: 10.1177/S0038038501000219.
- Hutchins, E. (1991), “The Social Organization of Distributed Cognition,” in Perspectives on Socially Shared Cognition, eds. L. B. Resnick, J. M. Levine, and S. D. Teasley, pp. 283–307, Washington DC: American Psychological Association.
- Iliadis, A., and Russo, F. (2016), “Critical Data Studies: An Introduction,” Big Data & Society, 3, 1–7. DOI: 10.1177/2053951716674238.
- Janeja, V. (2019, April 4), “Do No Harm: An Ethical Data Life Cycle |S&T Policy FellowsCentral,” AAAS Science and Technology Policy Fellowships. Available at https://www.aaaspolicyfellowships.org/blog/do-no-harm-ethical-data-life-cycle.
- Jasanoff, S. (2004), States of Knowledge: The co-Production of Science and Social Order, New York: Routledge.
- Jasanoff, S. (2016), The Ethics of Invention: Technology and the Human Future, New York: WW Norton & Company.
- Jasanoff, S., and Kim, S.-H., eds. (2015), Dreamscapes of Modernity: Sociotechnical Imaginaries and the Fabrication of Power, Chicago, IL: Chicago University Press. Available at https://press.uchicago.edu/ucp/books/book/chicago/D/bo20836025.html.
- Jobin, A., Ienca, M., and Vayena, E. (2019), “Artificial Intelligence: The Global Landscape of Ethics Guidelines,” Nature Machine Intelligence, 1, 389–399. DOI: 10.1038/s42256-019-0088-2.
- Joyce, K. A., Darfler, K., George, D., Ludwig, J., and Unsworth, K. (2018), “Engaging STEM Ethics Education,” Engaging Science, Technology, and Society, 4, 1–7. DOI: 10.17351/ests2018.221.
- Keller, S. A., Shipp, S. S., Schroeder, A. D., and Korkmaz, G. (2020), “Doing Data Science: A Framework and Case Study,” Harvard Data Science Review, 2. DOI: 10.1162/99608f92.2d83f7f5.
- Kishimoto, K. (2018), “Anti-Racist Pedagogy: From Faculty’s Self-reflection to Organizing Within and Beyond the Classroom,” Race Ethnicity and Education, 21, 540–554. DOI: 10.1080/13613324.2016.1248824.
- Koopman, C. (2017, March 15), “The Power Thinker: Why Foucault’s Work on Power is More Important than Ever,” Aeon. Available at https://aeon.co/essays/why-foucaults-work-on-power-is-more-important-than-ever.
- Koopman, C. (2019), How We Became Our Data: A Genealogy of the Informational Person, Chicago, IL: University of Chicago Press. DOI: 10.7208/9780226626611.
- Lambek, M., Das, V., Fassin, D., and Keane, W. (2015), Four Lectures on Ethics: Anthropological Perspectives, Chicago, IL: Hau Books.
- Latour, B. (1992), “Where are the Missing Masses? The Sociology of a Few Mundane Artifacts,” In Shaping Technology/Building Society, eds. W. E. Bijker and J. Law, pp. 225–258, Cambridge, MA: MIT Press.
- Leonelli, S. (2015), “What Counts as Scientific Data? A Relational Framework,” Philosophy of Science, 82, 810–821. DOI: 10.1086/684083.
- Leonelli, S. (2019), “Data Governance is Key to Interpretation: Reconceptualizing Data in Data Science,” Harvard Data Science Review, 1. DOI: 10.1162/99608f92.17405bb6.
- Leonelli, S. (2020), “Learning from Data Journeys,” in Data Journeys in the Sciences, eds. S. Leonelli, and N. Tempini, pp. 1–24, Cham: Springer. Available at https://link.springer.com/book/10.1007/978-3-030-37177-7.
- Lévinas, E. (1969), Totality and Infinity: An Essay on Exteriority, Pittsburgh, PA: Duquesne University Press.
- Markham, A. (2006), “Method as Ethic, Ethic as Method,” Journal of Information Ethics, 15, 37–55. DOI: 10.3172/JIE.15.2.37.
- Markham, A. N., Tiidenberg, K., and Herman, A. (2018), “Ethics as Methods: Doing Ethics in the Era of Big Data Research—Introduction,” Social Media + Society, 4, 1–9. DOI: 10.1177/2056305118784502.
- Mentis, H., and Ricks, V. (2021, June), “Making Lessons Stick [Mozilla].” Teaching Responsible Computing Playbook. Available at https://foundation.mozilla.org/en/what-we-fund/awards/teaching-responsible-computing-playbook/topics/lessons-stick/.
- Meretoja, H. (2018), The Ethics of Storytelling: Narrative Hermeneutics, History, and the Possible, Oxford: Oxford University Press.
- Morley, J., Floridi, L., Kinsey, L., and Elhalal, A. (2020), “From What to How: An Initial Review of Publicly Available AI Ethics Tools, Methods and Research to Translate Principles into Practices,” Science and Engineering Ethics, 26, 2141–2168. DOI: 10.1007/s11948-019-.00165-5.
- Mhlambi, S. (2020, July 8), “From Rationality to Relationality: Ubuntu as an Ethical and Human Rights Framework for Artificial Intelligence Governance.” Carr Center for Human Rights Policy. Available at https://carrcenter.hks.harvard.edu/publications/rationality-relationality-ubuntu-ethical-and-human-rights-framework-artificial.
- Murphy, M. (2017), The Economization of Life, Durham, NC: Duke University Press.
- Nakamura, L. (2012, January 31), “Prospects for a Materialist Informatics: An Interview with Donna Haraway.” Electronic Book Review. Available at http://electronicbookreview.com/essay/prospects-for-a-materialist-informatics-an-interview-with-donna-haraway/.
- National Academies of Science, Engineering, and Medicine (2018), Data Science for Undergraduates: Opportunities and Options. National Academies of Science, Engineering, and Medicine. DOI: 10.17226/25104.
- National Academy of Engineering (2016), NAE Annual Report 2016. National Academy of Engineering. Available at https://nae.edu/174319/NAE-Annual-Report-2016.
- Neff, G., Tanweer, A., Fiore-Gartland, B., and Osburn, L. (2017), “Critique and Contribute: A Practice-Based Framework for Improving Critical Data Studies and Data Science,” Big Data, 5, 85–97. https://doi.org/10.1089/big.2016.0050.
- Nelson, A. H., Jenkns, D., Zanti, S., Katz, M., and Berkowitz, E. (2020), A Toolkit for Centering Racial Equity throughout Data Integration. Actionable Intelligence for Social Policy, Philadelphia, PA: University of Pennsylvania.
- Nolan, D., and Stoudt, S. (2021), “The Promise of Portfolios: Training Modern Data Scientists,” Harvard Data Science Review, 3.3. DOI: 10.1162/99608f92.3c097160.
- O’Neil, C. (2016), Weapons of Math Destruction: How Big Data Increases Inequality and Threatens Democracy, New York: Crown.
- Orlikowski, W. J. (2000), “Using Technology and Constituting Structures: A Practice Lens for Studying Technology in Organizations,” Organization Science, 11, 404–428. DOI: 10.1287/orsc.11.4.404.14600.
- Pink, S., and Lanzeni, D. (2018), “Future Anthropology Ethics and Datafication: Temporality and Responsibility in Research,” Social Media + Society, 4, 1–9. DOI: 10.1177/2056305118768298.
- Puschmann, C., and Burgess, J. (2014), “Metaphors of Big Data,” International Journal of Communication, 8, 1690–1709.
- Ricøeur, P. (1988), Time and Narrative, Chicago, IL: University of Chicago Press.
- Ricøeur, P. (1992), Oneself as Another, Chicago, IL: University of Chicago Press.
- Riley, D. (2003), “Employing Liberative Pedagogies in Engineering Education,” Journal of Women and Minorities in Science and Engineering, 9, 137–158. DOI: 10.1615/JWomenMinorScienEng.v9.i2.20.
- Saltz, J., Skirpan, M., Fiesler, C., Gorelick, M., Yeh, T., Heckman, R., Dewar, N., and Beard, N. (2019), “Integrating Ethics Within Machine-learning Courses,” ACM Transactions on Computing Education, 19, 1–26. DOI: 10.1145/3341164.
- Sawyer, S., and Jahari, M. H. (2014), “Sociotechnical Approaches to the Study of Information,” in Computing Handbook (3rd ed), eds. H. Topi and A. Tucker, pp. 1–27. Boca Raton, FL: CRC Press.
- Selbst, A. D., Friedler, S. A., Venkatasubramanian, S., Vertesi, J., Boyd, D., and Venkatasubrama, S. (2019), “Fairness and Abstraction in Sociotechnical Systems,” in Proceedings of the 2019 Conference on Fairness, Accountability, and Transparency (FAT* ’19), 59–68. DOI: 10.1145/3287560.3287598.
- Shearmur, R. (2015), “Dazzled by Data: Big Data, the Census and Urban Geography,” Urban Geography, 36, 965–968. DOI: 10.1080/02723638.2015.1050922.
- Shilton, K. (2013), “Values Levers: Building Ethics into Design,” Science, Technology, & Human Values, 38, 374–397.
- Sloane, M., and Moss, E. (2019), “AI’s Social Sciences Deficit,” Nature Machine Intelligence, 1, 330–331. DOI: 10.1038/s42256-019-0084-6.
- Stark, L., and Hoffmann, A. L. (2019), “Data Is the New What? Popular Metaphors & Professional Ethics in Emerging Data Culture,” Journal of Cultural Analytics, 1–22. DOI: 10.31235/osf.io/2xguw.
- Stodden, V. (2020), “The Data Science Life Cycle: A Disciplined Approach to Advancing Data Science as a Science,” Communications of the ACM, 63, 58–66. DOI: 10.1145/3360646.
- Tanweer, A., Gade, E. K., Krafft, P. M., and Dreier, S. K. (2021), “Why the Data Revolution Needs Qualitative Thinking,” Harvard Data Science Review, 3(1). DOI: 10.1162/99608f92.eee0b0da.
- Verbeek, P.-P. (2006), “Materializing Morality: Design Ethics and Technological Mediation,” Science, Technology, & Human Values, 31, 361–380. DOI: 10.1177/0162243905285847.
- Wodajo, K., and Ebert, I. (2021, October 15), “Reimagining Corporate Responsibility for Structural (In)justice in the Digital Ecosystem: A Perspective from African Ethics of Duty,” Afronomics Law. Available at https://www.afronomicslaw.org/category/analysis/reimagining-corporate-responsibility-structural-injustice-digital-ecosystem.
- Zigon, J. (2019), “Can Machines Be Ethical? On the Necessity of Relational Ethics and Empathic Attunement for Data-Centric Technologies,” Social Research: An International Quarterly, 86, 1001–1022. DOI: 10.1353/sor.2019.0046.