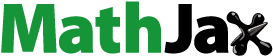
Abstract
Common sense is a dynamic concept and it is natural that our (statistical) common sense lags behind the development of statistical science. What is not so easy to understand is why common sense lags behind as much as it does. We conduct a survey among Japanese students and provide examples and tentative explanations of a number of statistical questions where common sense and statistical science diverge. Supplementary materials for this article are available online.
La théorie des probabilités n’est au fond
que le bon sens réduit au calcul
P. S. Laplace (Citation1814)
1 Introduction
The study of medicine, zoology, geography, astronomy, and of sculpture and music goes back thousands of years; and this applies also—and in particular—to mathematics. As a field of study, mathematics is at least 5000 years old and reached a high point in the third century BC with Euclid of Alexandria and Archimedes of Syracuse. Primary school children learn mathematics through counting, then adding and multiplying, then simple equations like , and this develops into high-school and undergraduate mathematics in a perfectly natural way.1
In contrast, probability theory is a young science. It originated from the need to calculate the odds in games of chance, but although the Greeks presumably played games of chance (according to mythology, both Hermes and Pan gambled), there is no record of any mathematical analysis of gambling and odds until the sixteenth century. A gambler may wish to know how many times one die needs to be thrown so that the probability of obtaining 6 at least once exceeds 50%. Cardano (1501–1575) thought the answer was three (in fact, the answer is four). Or: if we throw with two fair dice, how many times do we need to throw so that the probability of obtaining at least one double-6 exceeds 50%. The Chevalier de Méré (1607–1684) thought that he needed 24 throws. This problem has become famous because it intrigued Blaise Pascal (1623–1662) and Pierre de Fermat (1601–1665), and the solution (25 throws) is contained in a letter of Pascal to Fermat dated July 29, 1654. These two examples show how eminent mathematicians struggled with problems that we now find quite trivial. Probability theory begins with Pascal, Fermat, and Christiaan Huygens (1629–1695), and we place its birth year at 1654; see Kahneman (Citation2011) and Tijms (Citation2021) for examples and historical details of early and current pitfalls in probability.
While probability theory is a young science, mathematical statistics is much younger and we place its birth year at 1809, when Gauss showed that the maximum likelihood estimator of β in the linear model under normality is given by the least-squares formula.2
Probability theory and statistics are generally considered difficult fields. Other fields such as physics or law are also difficult, but the difficulty in dealing with random variables seems to be of a different nature. When we start an undergraduate degree in physics or law we already have some basic understanding of the subject. But dealing with variables that do not take specific values (as in algebra), but rather follow some probabilistic law—this requires a new way of thinking, and our mind is apparently not very well equipped for this task.
Why not? Maybe because probability and statistics are such young fields. Durbin (Citation1985, Citation1988) attempted a Darwinian approach arguing that we acquired just enough thinking capacity to ensure survival as primitive hominids millennia ago, which would explain why we can do mathematics as well as we can, but not probability theory. But why would we not have needed some knowledge of risk and probability to survive?
Suppose you are poisoned in the jungle and the only way to save yourself is to lick a special kind of frog. Only the female of that species will do; licking the male frog does not help. The male and female frogs look identical and appear with equal probabilities. The only difference is that the male frogs sometimes emit a distinctive croak. You spot a frog in front of you, but then you hear a croaking sound behind you. You turn around and spot two frogs there. There’s only time to run to either the front or the back. Which way should you run?
Surely our ancestors would have been much helped in their survival if they could solve this and similar puzzles, which even today cause controversy among non-probabilists.3
Another possible explanation is provided by the idea of “morphic resonance” (Sheldrake Citation1995). When laboratory rats have learned a new maze, rats elsewhere seem to learn it more easily. How can this happen? Perhaps because some form of “collective consciousness” has descended among all rats. This is not a phenomenon that conventional scientific theories can explain, and it remains a precarious argument, easily dismissed as magical thinking and pseudo-science. It is related to Carl Jung’s (Citation1936) idea that “there exists a second psychic system of a collective, universal, and impersonal nature which is identical in all individuals.” Jung calls this the “collective unconscious.”
An easier explanation and less precarious may be how we educate our children. While arithmetic and mathematics are basic school subjects, this is not the case for probability theory and statistics.
What is worse is that common sense and probabilistic and statistical theory often diverge, and this is the subject of the current article. In the quote at the top of this article, Laplace (Citation1814, p. 273) states that “probability theory is au fond nothing but common sense reduced to calculus.” This may be so, but common sense is not a static but a dynamic concept. What is common sense now was not common sense a few hundred years ago, and what is not common sense today may be common sense sometime in the future. Some of the problems and misunderstandings that baffled such minds as Pascal, Fermat, and Leibniz no longer baffle even non-probabilists today. But, at the same time, there are many seemingly simple questions that today even people with quantitative skills find hard to solve. And, even if they can solve such problems, they may find the outcomes counter-intuitive and unacceptable. We shall see examples of this divergence between theory and common sense as we proceed.
The problems associated with making decisions under uncertainty were studied by many, but in particular by Pólya and, most extensively, by Kahneman and Tversky; see for example Pólya (Citation1968), Kahneman and Tversky (Citation1972), Tversky and Kahneman (Citation1973, Citation1974), and Kahneman (2011). While they concentrated on the discrepancy between probability theory on the one hand and human intuition and common sense on the other, our focus lies on statistical questions. We discuss some probabilistic issues as well, but these serve mainly as a background to the statistical issues.
As a thread through the article are 10 questions from a survey we conducted among students at Osaka University in Japan. In Section 2 we explain the survey design. In Section 3 we establish the student’s background in probability theory, test some basic quantitative ability, and discuss unconditional and (more difficult) conditional probabilities. Then, turning to statistical issues, we discuss prediction in Section 4, prediction intervals in Section 5, and testing in Section 6. In Section 7 we investigate to which extent a background in probability theory helps to answer the questions posed in the survey, and we distinguish between males and females, undergraduates and postgraduates, field of study, the order of asking the questions, and cognitive ability. Section 8 concludes.
2 Survey Design
An online survey was conducted between July 27th and July 30th, 2021 by the Experimental Economic Laboratory of the Institute of Social and Economic Research (ISER) at Osaka University. The project was approved by the Research Ethics Committee of the Institute of Social and Economic Research, Osaka University. The survey employed a web-based online recruitment system, specifically designed for organizing economic experiments, called ORSEE (Greiner Citation2015). We invited 415 students from Osaka University (both undergraduates and postgraduates) who had previously participated in other online experiments, so that we know some of their individual characteristics from these previous experiments (Hanaki et al. Citation2021). On July 27 each student received an E-mail with an invitation to participate and an individually customized link to the survey site. Students were asked to fill out the questionnaire by July 30. They had one hour to answer all the questions after accessing the site.4
Of the 415 students, 350 students completed the survey. One student completed the survey twice (which was only possible by using two different browsers); he/she is only counted once. This leaves 349 students.
The survey contained 10 questions plus one “attention-verification” question (in the middle of the survey), where we check whether the respondent is paying attention. Unlike the other questions, we employ for this question a method developed by Oppenheimer et al. (Citation2009) by asking respondents to ignore the standard response format and instead provide confirmation that they have read the instructions, as follows:
Question 0.
What is the likelihood of obtaining head in a throw of a fair coin? Please select “(A) 1” so that we know you are paying attention.
Nine students did not answer (A) and these have been excluded.5 This leaves us with 340 “clean” responses for analysis: 96% Japanese students versus 4% foreign students, 69% undergraduates versus 31% postgraduates, and 61% men versus 39% women.
According to the OECD Programme for International Student Assessment (PISA), the average score of Japanese students in Math in 2018 is sixth in the world. Furthermore, Osaka University is one of the most selective universities in Japan. As a result, we may assume that our respondents are above average in Math skills, both nationally and internationally.
Students are enrolled in a variety of faculties, which we label as
STE: Science, Technology, and Engineering;
Med Medicine (incl. public health, biology, dentistry, pharmaceutical);
HS Humanities and Social Science (incl. literature, foreign languages, law, international public policy, economics).
The distribution of the students in our sample over the faculties was as follows:
The 11 questions are numbered 0–10. In order to minimize possible ordering effects, we prepared two versions of the questionnaire. In the first version the order of the questions is 1, 4, 5, 2, 3, 0, 6, 8, 9, 7, 10. In the second version the order is reversed: 10, 7, …, 1. Allocation to the respondents was random.
The questions fall into different categories. In Question 1 we ask about the student’s background in probability theory. Questions 2 and 3 test some basic quantitative ability. In Questions 4–6 we test knowledge of (unconditional) probabilities and in Question 7 of conditional probability, which is much more difficult. The most important questions are 8–10 about prediction, prediction intervals, and testing.
3 Quantitative and Probabilistic Knowledge
We first ask the students about their background in probability and statistics.
Question 1.
Did you follow and pass a probability or statistics course at university level? If so, did you enjoy it?
We see that 60% of the students received some instruction on probability theory and statistics. Most did not enjoy it.
We next ask two questions on basic quantitative ability.
Question 2.
The average annual salary for an employee at a university is 4,000,000. This year, the management awards the following two bonuses to every employee: an end-of-year bonus of
300,000 and an incentive bonus equal to 10 percent of the employee’s salary. What is the average total bonus received by employees?
Table
The correct answer is , and 95% of the students got this right.
Question 3.
An economist is studying the relationship between the weight of a car, its reliability rating (the higher the rating, the more reliable), and the annual cost of maintenance. The economist reports the following correlations: the correlation between the weight of a car and the car’s reliability rating is –0.20; and the correlation between the weight of a car and the annual maintenance cost is 0.40. Which of the following statements are true?
Heavier cars tend to be more reliable,
Heavier cars tend to be less reliable,
Heavier cars tend to cost more to maintain,
Car weight is related less strongly to its reliability than to its maintenance cost.
The first statement is incorrect, but the other three are correct, so that (D) is the correct answer and 91% had it right. The large majority of the students in our sample thus answered simple quantitative questions correctly, 95% for Question 2 and 91% for Question 3. Still, 5%–9% of the students failed to answer even the simplest questions.
We next exposed our respondents to three questions about basic (unconditional) probabilities.
Question 4.
One die is tossed. What is the probability that the die will land on a number that is smaller than or equal to 4?
Question 4 is an easy starting question about basic probabilities. There are four “good” (1, 2, 3, 4) outcomes and two “bad” (5, 6) outcomes, and since all are equally likely, the answer is 2/3. Almost all students (97%) got this right.
One level more difficult is throwing with two dice.
Question 5.
You throw with two dice. Then you can throw any number between 2 and 12. Now, you can throw 12 only by throwing six twice. Similarly, you can throw 11 only by throwing 5 and 6 once each. Which of the following is correct?
This is a famous question, because the celebrated German mathematician Gottfried Wilhelm (von) Leibniz (1646–1716) maintained that it was equally likely to throw 12 with two dice than to throw 11, because “l’un et l’autre ne ce peut faire que d’une seule manière” (one or the other can be done in only one way).6 Leibniz’ error is remarkable as it came some 60 years after the discoveries of Pascal and Fermat, which marked the birth of probability theory. It demonstrates just how difficult the basic concepts in probability theory are.
The correct answer is (B) because there are 36 equally likely outcomes, of which one (6–6) yields 12 and two (5–6 and 6–5) yield 11. Most of the students (84%) got this right, not because they were more clever than Leibniz but because basic probability theory has somehow sunk into “collective consciousness,” at least to some extent.
Equally famous is the next question.
Question 6.
You and your friend play a simple game with one die in a tea house. If the outcome of the throw is even, you get 1 point; if it is odd, your friend gets 1 point. Each of you puts 60 on the table, and the first to reach 3 points wins the game and gets the money. One day, at the score 2-1 in your favor, the tea house burns down. You and your friend take the money and run. Next day you meet again (in another tea house), but you do not want to continue the game. Instead you want to divide the money. How should this be done?
Table
Pascal and Fermat corresponded about this question, and the problem was resolved in Pascal (Citation1665) by relating it to Pascal’s triangle. Almost one-half of the students in our sample (46.8%) thought of this as an ethical or legal problem, not as a probabilistic problem, so they answered (A) or (D) neither of which has a probabilistic basis. Our interest is in those who attempted a probabilistic solution, so let us concentrate on (B) and (C). The argument in (B) seems to correspond to common sense: you won 2/3 of the games, so you get 2/3 of the money. The problem with this argument is that it is backward-looking; it only considers the past. Pascal, on the other hand, considered the future, arguing that, since three games had already been played, a maximum of two more games needed to be played. These two games could end in two losses for you (with probability 1/4), but in every other case you win the money. So the probability that you win the match is 3/4, and this means that (C) is the correct answer.
In Question 6 we see for the first time a divergence between common sense and probability theory: more than twice of the students preferred (B) over (C). Even though the correct answer has been known for over 350 years and the question should be an easy one in any introductory probability class, common sense has not yet adjusted to probabilistic truth. Most people’s intuition is simply wrong.
Much more difficult than unconditional probabilities are conditional probabilities.7 Here is a typical counter-intuitive example.
Question 7.
You are worried about your mother’s health, and you are convinced that she suffers from some rare disease you have been reading about. So, your mother visits the doctor. The doctor is not convinced, but she agrees to have a test done anyway. The disease your mother gets tested for is quite rare, occurring in only one of every 10,000 people. If your mother has the disease then there is a 99% probability that the test is positive. But if your mother does not have the disease, then the test can also be positive; this happens with a probability of 0.5%. After a few days the test result becomes available. It is positive. What do you think is the probability that your mother has the disease?
The right answer is (A) and 1/3 of the students got it right. The reasoning, too complex for the untrained probabilist, proceeds as follows. Let A denote the event that your mother has the disease and B the event that she tests positive; and let denote the event that your mother does not have the disease and
the event that she tests negative. Then,
This is all the information we have. The information suffices to obtain the complete joint distribution (in percentages):
Table
This gives
which is less than 2%. Question 7 is not just a probabilistic puzzle but it has potentially serious practical consequences. Only one in three of our respondents got it right. For the human mind it is counter-intuitive that if a test has a 99% probability to be positive when the patient has the disease and if the test turns out positive, then the probability that the patient actually has the disease is less that 2%. Most people simply do not believe it.
4 Prediction
In the previous section we tested our respondents on their quantitative ability (Question 1–3) and on their understanding of the basic laws of probability (Question 4–6) and conditional probability (Question 7). We now turn to our principal interest: statistics. There are many counter-intuitive results in statistics and we shall discuss three of them. Of these, our first question is perhaps the most counter-intuitive.
Question 8.
Suppose the Minister of Economics needs to forecast next year’s inflation. He asks two well-known experts to advise him. The first expert responds that there will be 1% inflation next year, and the second that there will be 2% inflation. The two forecasts are published in the press so that everybody knows about them. The minister then reflects. He realizes that the two experts know each other and that they base their forecasts on the same (or very similar) data sets. Also, from past experience, the minister has more trust in the second expert than the first. After considering the two forecasts he declares the Ministry’s forecast to be 2.25%. What do you think of this?
Let x1 and x2 be two uncorrelated observations with a common mean q and variances and
, respectively. We wish to estimate q as an unbiased estimator with the lowest variance. Hence,
(1)
(1) with mean q and variance
(2)
(2)
where
. The variance is minimized when
, and we obtain
(3)
(3)
We note that estimated mean is in-between x1 and x2 and that its variance is smaller than both and
. Adding information reduces the variance.
But now consider the same situation assuming that x1 and x2 are correlated. Let , where ρ is the correlation coefficient. Again we have
, as in (1), with the same mean q but not the same variance. In fact,
(4)
(4) which is minimized when
(5)
(5)
so that α lies outside the
interval if and only if
. The estimator takes the form
(6)
(6)
which agrees with (3) when ρ = 0. When
and
, then
if and only if
, which can only occur for
and
, for example for ω = 3 and
; see Magnus and Vasnev (Citation2008, Appendix A) for further details and intuitions about this case.
We conclude that in the presence of positive autocorrelation it is perfectly possible (even likely) that a combined forecast lies outside the bounds indicated by the advisors. But, if the advisors’ forecasts are publicly available, then it would take a courageous politician to go outside these bounds, and the outcome of our experiment shows that the public would not understand the rationale for such a deviation. Still, not going outside the bounds would be bad policy and would lead to suboptimal forecasts.
5 Prediction Intervals
When predicting, we are not only concerned with the prediction itself but also with the reliability of the predictor. Here, we are faced with a puzzle for which there is no easy answer, and where common sense and mathematical rigor do not seem to be in line, even for experts. The next case illustrates this situation.
Question 9.
Suppose the Minister of Economics needs to know the value of some unknown quantity in order to formulate ministry policy. Let us call this quantity q. He consults an expert, who tells him that q = 10. The expert cannot be entirely sure about this number, but she is confident that q lies between 8 and 12. The minister then proceeds with policy based on this information. After some time he thinks it wise to consult a second expert. The second expert tells him that q = 30. This expert is not certain either, but he is confident that q lies between 26 and 34. The minister believes that the first expert is slightly more reliable than the second expert, but only slightly. Based on this new information the minister decides to change q from q = 10 (the old information) to q = 20 (the average of the old and the new information). But how much confidence should the minister have in this new number? Indicate below the range that the minister should feel quite confident about. Tick only one box. The minister should be quite confident that:
The problem here is a conflict between two pieces of information, which happens frequently in practice. In Bayesian analysis, for example, the prior and the sample information may deliver conflicting messages. In the normal framework (normal prior, normal likelihood) this implies that the posterior mean is somewhere in-between the mean of the prior and the mean of the sample, which is reasonable. But it also implies that the posterior variance is smaller than the variance of the prior and the variance of the sample. This seems also reasonable because we have added information, so the precision should increase. But it is counter-intuitive (also for the professional), because the conflicting information makes us less confident about the result: more information leads to less confidence.
The example in Question 9 is frequentist rather than Bayesian, but the idea is the same. We have two pieces of information, say and
with standard deviations which are approximately equal to
and
. Then, if the two observations are uncorrelated, the average
has variance
(7)
(7)
The standard deviation of is therefore
and a reasonable confidence interval for the unknown mean q would be
.
More generally, allowing for different weights and for possible correlation, we write again, as in (1), with mean q and variance (4). The variance is minimized when α takes the value in (5), in which case the estimator and its variance take the form
(8)
(8) which reduces to (3) when ρ = 0. In our case, we have
, and
, so that
(9)
(9)
For ρ = 0 we find which is smaller than
because the first advisor is considered more reliable than the second, and
. Taking correlation into account does not help to increase the variance, which achieves a maximum
at
and converges to zero as
.
Hence, from a theoretical point of view the variance remains small, even when we take correlation into account. This, however, does not correspond to common sense, as is clear from our respondents. Only 4% found it reasonable that q lies between 19 and 21, while the majority (51%) voted for q to lie between 8 and 34.
6 Testing
Testing hypotheses is another counter-intuitive enterprise. When we have an idea that perhaps a statement S is true, then the natural and common sense thing to do is to find many examples where S holds. But when we follow a first course in Statistics we learn that a statistician does the opposite. The statistician puts all effort into rejecting the hypothesis and only if they have tried everything and from every angle and still the hypothesis is not rejected, even then the statistician does not conclude that the hypothesis is true, but only that it cannot be rejected.
Most trained statisticians are used to this and do not find it counter-intuitive any more, but for the average citizen it remains counter-intuitive, even though we know since Popper (Citation1962) that if we want to prove a statement like All statisticians lie, then searching for more and more dishonest statisticians may be useful in formulating the hypothesis but not in testing it. In order to test the hypothesis we have to search for honest statisticians. One honest statistician suffices to reject the hypothesis.
In the behavioral sciences most statements are not of the form “all A are B” but rather “most A are B.” For example, we know that men run about 10% faster the women.8 But it is easy to find women who run faster than men, and one counter-example does not refute the hypothesis. While the Popperian approach does not work here, the testing theory in mathematical statistics is unaffected. Unfortunately this testing theory is not in line with common sense.
In daily life, most of us have no wish to challenge our beliefs; we prefer to seek confirmation of our beliefs. We choose friends whose ideas agree with our ideas and we read newspapers that promote views that we find sympathetic. How many trained statisticians subscribe to newspapers that reflect views with which they fundamentally disagree? From a statistical point of view this would be the rational thing to do, but few of us actually do it.
Our final question illustrates this behavior.
Question 10.
Suppose you are a high-school student in your final year; next year you’ll be going to university. In choosing your field of study, you waver between business economics (choice A) and Japanese literature (choice B).
Studying business economics (choice A) will give you a qualification that will make you attractive to companies so you can obtain an amazing internship. It is also a great foundation for an MBA or a finance degree, or a degree in public policy. During your studies you’ll enjoy the problem-solving and strategic thinking the discipline requires. After completing your studies it will be easy to find a job, and you will earn a good salary. A degree in business economics will be useful if you wish to start your own business, and it may help you become a successful investor. You will understand how economies work: to understand economics is to understand how the world works. You will be able to predict trends of businesses and economies based on your knowledge rather than based only on what is reported in the media. You’ll also develop an informed perspective on social and political issues.
Studying Japanese literature (choice B) will help you understand Japanese history and culture. You will learn Japanese expressions that are not used in daily life. Japanese literature is rich in history and tradition, and it offers a vast array of genres, authors, and styles that you can explore. Your studies will help you communicate in more meaningful and expressive ways, and they will allow you to understand literature at a deeper level. Literature gives us glimpses of other times, places, and lives that we will never experience otherwise; it offers invaluable insights into what it means to be human. The field offers unlimited directions for creative analysis and original work. After completing your studies you may become a teacher of Japanese or possibly a famous writer, and you will enjoy a richer intellectual life.
Now, what do you choose: A or B?
Most of the respondents (76%) chose for business economics, but this is not really what interests us. After choosing A or B, the question continues:
Next I offer you some further advice. If you want advice in favor of A, click A. If you want advice in favor of B, click B.
This second question does interest us. There is little point in asking positive advice in favor of your preferred option, because this should not change your decision. Asking advice favoring the opposite view might change your decision, so this is the sensible thing to do. But this is not how people behave. Apparently, they wish to be confirmed in their view and they are not interested in listening to a deviating view. Of those who chose A in our sample, 61% want advice in favor of A; and of those who chose B, 73% want advice in favor of B.
Depending on their answer the advice would be revealed:
(if clicked A:) What will your parents think? They will see that you’re headed toward a well-paying job, and this will make them happy.
(if clicked B:) What will your parents think? They will see that you’re headed toward a rewarding life where you will enjoy your work, and this will make them happy.
Now, what do you choose: A or B?
Very few students changed their minds. Of those who chose business economics and received affirmative advice, 99% chose business economics again; the minority of students who asked confrontational advice still remained with their original choice (94%). Of those who chose Japanese literature and received affirmative advice, 97% chose Japanese literature again; the minority of students who asked confrontational advice still remained with their original choice (82%).
Steps 2 and 3 are then repeated.
I offer you some further (final) advice. Again, if you want advice in favor of A, click A. If you want advice in favor of B, click B.
Of those who chose A, wanted advice about A and affirmed their choice A after the advice (155 students), 66% chose to receive further advice on A again. Of those who chose B, wanted advice about B and affirmed their choice B after the advice (59 students), 71% chose to receive further advice on B again. This is the largest group (214 students, 65% of the sample), and they represent the people whose interest is in affirming their prior views. They have no interest in the alternative and do not want to put their idea to the test.
At the other end of the scale are those who are willing to challenge their prior ideas. Of those who chose A, wanted advice about B, and affirmed their choice A after the advice (94 students), 55% chose to receive further advice on B again. Of those who chose B, wanted advice about A and affirmed their choice B after the advice (18 students), 33% chose to receive further advice on A again. This means that 112 students (33% of the sample) behaved rationally, following the ideas of statistical testing.
Only 14 students changed their choice after receiving advice: 4 changed their mind in spite of affirming advice, but 10 were apparently convinced by the argument in favor of the alternative. Of those 10 students, 7 behaved rationally by challenging their latest choice again.
Depending on their answer the advice would then be revealed:
(if clicked A:) Studying business economics will help you become a rational person.
(if clicked B:) Literature is the pinnacle of civilization — studying it honors the very best humankind has to offer.
Now, what do you choose: A or B?
Of the 257 students who chose A at the beginning, 246 (96%) chose A again at the end; and of the 83 students who chose B at the beginning 78 (94%) chose B again at the end.
7 Do Basic Knowledge or Basic Ability Help in Understanding Statistics?
Our respondents are part of a student data base, and therefore we know something about them. We know, for example, whether they are male or female, undergraduate or postgraduate, what their field of study is, and we also know something about their “cognitive ability.”9 We now investigate the relevance of these additional pieces of information.
Gender—Female versus male
In our sample about 60% of the respondents followed a course in probability or statistics (Question 1), and this is roughly the same for men and women: 62% for men versus 60% for women. But, of those who followed such a course, men enjoyed it much more than women: 78% versus 50%. This explains, perhaps, why the men in our sample performed better than the women. Of the easy questions (Question 2–5), men “scored” 93% and women 88%; while on the difficult questions (Question 6–10), men scored 33% and women 21%. (The score is the average proportion of participants that correctly answered the relevant questions.)
Undergraduates versus postgraduates
The undergraduates in our sample had roughly the same exposure to a previous class in probability and statistics as the postgraduates, and their enjoyment of such a class was also roughly the same. Undergraduates performed slightly better than postgraduates: 92% versus 91% on the easy questions, and 29% versus 27% on the difficult questions. In particular in Question 10 (testing), the undergraduates proved themselves more rational than the postgraduates.
Field of study
As discussed in Section 2, we distinguish between three fields of study, labeled STE (Science, Technology, and Engineering; 40%), Med (Medicine; 17%), and HS (Humanities and Social Science; 43%). Of the Med students, 85% had followed some course in probability and statistics, but only 24% of those had enjoyed the course (as measured in Question 0). Of the STE students, fewer students (65%) followed such a course but they enjoyed it more (49%). As expected, the number of students in HS with a background in probability and statistics is relatively small (45%) and of those only 37% enjoyed the course.
The lack of enjoyment among Med students is reflected in how well the students performed in our survey. The STE students performed best, followed by the Med students, and the HS students. On the easy questions the STE students scored 95% (36% on the difficult questions), while the scores for the Med students were 90% (25%) and for the HS students 89% (22%). The difference between the three groups shows up most markedly in the difficult questions.
Order of asking the questions
Not much difference is detected between the two orderings. One might think that students would find “easy-to-difficult” more congenial and therefore perform better than “difficult-to-easy,” but this hypothesis is rejected: “easy-to-difficult” scored 57% while “difficult-to-easy” scored 56%, and the difference is not statistically significant.
Cognitive ability
Most students in the data base have been subjected to a six-question cognitive reflection test, where the first three questions are taken from Finucane and Gullion (Citation2010) and the last three from Toplak et al. (Citation2014); see also Frederick (Citation2005). The score CRT is the number of correct answers: . For example, one question from the first group is
Soup and salad cost 5.50 euros in total. The soup costs 5 euros more than the salad. How much does the salad cost (in euros)?and one question from the second group:
If John can drink one barrel of water in 6 days, and Mary can drink one barrel of water in 12 days, how long would it take them to drink one barrel of water together (in days)?
The correct answer for the first question is 0.25 euro, but the intuitive answer is 0.50 euro; while for the second question the correct answer is 4 days and the intuitive answer 9 days.
The CRT score is, not surprisingly, highly related to Question 0. Of those with a high CRT score (), 62% followed an earlier probability and statistics course, while of those with a lower score (
) only 49% followed such a course. The CRT score is also positively correlated with the performance on our test, and this is especially true for the easy questions (Question 0–4). Those who got all four questions right have a score of
, which suggests that a correct solution to the easy questions is affected by prior education and field of study, but that difficult questions are difficult regardless of the respondents’ background and cognitive ability.
Table
The effects of basic knowledge (as reflected in Question 1) and basic ability (as reflected in Question 2 and 3) are further analyzed in the displayed table. Let x = 1 if the student followed and passed a course in probability or statistics (Question 1), 0 otherwise; and let yj = 1 if the student answered Question j correctly, 0 otherwise. Then we can test the relationship between the quantities and
using the usual
test for two-way tables. In the table we present
(10)
(10) together with the appropriate test statistic and the associated p-value. (Question 10 is omitted from this table because it has multiple answers.)
We see that for all probability questions (
), showing that perhaps basic quantitative knowledge helps, but not in the statistics questions (j = 8 and 9), suggesting that more basic knowledge makes it less likely to answer statistical questions correctly. However, none of the
-tests are significant at the 5% level, since all p-values are much larger than 0.05. This means that we find no evidence of dependence in any of the eight cases.
We define similarly, but now based on x = 1 if the student answered both Question 2 and 3 correctly, 0 otherwise. Thus,
if and only if basic ability is useful in answering Question j. Now,
for all j except the statistics question j = 9. Hence, basic ability seems to help, but the formal test is only significant for Question 5, the question that Leibniz got wrong. There is no evidence of dependence in the other five cases.
Neither basic quantitative knowledge nor basic quantitative ability seems to help in answering questions involving random variables, and this is especially true for statistical questions.
8 Conclusion
The fact that probability, especially conditional probability, is difficult is well-known. In the current article we concentrated on statistics rather than on probability, and we asked the following two questions.
First, are there common situations where theoretical results are counter-intuitive for all but the best-trained of us, that is, do statistics and common sense diverge; and if so, to what degree? Second, why are probability theory and statistics perceived as particularly difficult? Is it their short history (perhaps using a Darwinian argument), morphic resonance, lack of exposure through education? To these two questions we may add a third: If there is such a gap, what can we do about it?
Regarding the first question: Yes, statistics and common sense often diverge. We have seen that “easy” probabilistic questions can now be solved even by students without any background in probability and statistics. So, these untrained students can solve problems that puzzled Pascal and Leibniz, presumably because the required knowledge has somehow sunk into “collective consciousness.” In contrast, “difficult” questions remain difficult regardless of the respondents’ background and cognitive ability. Casual observation and a little introspection seem to confirm this. It is unlikely that an academic with at least some quantitative background will make a mistake in a simple arithmetic exercise, say 321 × 123. But the same academic is not going to be equally confident about a simple question in probability or statistics, such as
Assume that each born child is equally likely to be a boy or a girl. If a family has two children, then what is the probability that both are girls if we know that the youngest is a girl? And what is the probability if we know that one of them is a girl?
A simple question, but it has puzzled many. Let BG denote the case where the oldest child is a boy and the youngest a girl, and similar for BB, GB, and GG. Then the sample space reduces to (BG, GG) in the first case and to (BG, GB, GG) in the second case. Hence, the conditional probabilities are 1/2 and 1/3, respectively.
In our statistical questions 8–10 the lack of statistical understanding is quite remarkable. In particular, the concept of statistical testing in Section 6 remains a mystery for most of our respondents. They seek confirmation rather than allowing their view to be challenged. Even properly trained quantitative students do not understand some of the basic ideas of estimation and testing theory, and their intuition is often contrary to statistical theory.
The second question is more challenging. Why is statistics so difficult? Maybe because it has such a short history, maybe because we do not (or hardly) learn it at school or from our parents, maybe because it requires a way of thinking that is alien to the human mind, or maybe because statistics (and probability theory) are isolated subjects: knowledge of another subject does not help much.
Another reason might be that there is almost no “easy” probability theory or statistics. Calculating simple probabilities after throwing with one die may be easy, but throwing with two dice is already much more difficult, let alone questions involving risk taking and testing. This is very different in mathematics, where there exists a whole hierarchy of arithmetical questions which are well within a primary school child’s grasp. At some point we arrive at questions like . This is still arithmetic, but it is close to becoming mathematics. The following problem involves proper mathematics in its simplest form.10
A wall and a ladder have the same height. One meter under the top of the wall is a marker and we put the top of the ladder at that marker. The foot of the ladder is then three meters away from the wall. How high is the wall?
Here we have to introduce x (the height of the wall) and then obtain one equation with one unknown using Pythagoras’ theorem: , from which we solve x = 5.
The difference between the ladder problem and the equation is that the former is a story that first needs to be translated into a mathematical equation. This makes it more difficult, although it helps that the story is unambiguous. In sharp contrast, statistical stories are seldom unambiguous. Even in a simple story like the above two-child problem, the phrase “If a family has two children” suggests that we have sampled a couple first, but this is not stated explicitly. If we had written “if a girl is from a family with two children,” then this would have suggested that we had sampled the girl, and this makes a difference. Similarly, in the frog problem of Section 1 it is assumed that there is time to lick both frogs. But if there is only time to lick one frog (chosen at random if there is more than one frog), then the conclusion is reversed. In general, it is difficult to deduce the statistical experiment from the story.
The third question is: what to do about it? We must do something, because a proper understanding of risk, probability, and testing is getting increasingly important in our society, and a lack of understanding can be quite dangerous. Our society is now data-driven and this has increased the importance of a better understanding of statistics and of statistical literacy (Rumsey Citation2002; Gal Citation2003; Sharma Citation2017). Since we cannot change the history of our field or the human mind, there is only one way to increase the understanding of random variables and statistics, and that is by introducing children to it at a young age. But is has to be the right kind of education, because there is some evidence (also from the current study) that more education may be a liability rather than an asset in solving probability and statistics problems; see Herbranson (Citation2012) who found that pigeons outperform humans (especially more educated humans) in solving the Monty Hall problem.
Again, there seems to be only one way of achieving this, and that is to provide some serious probability and statistics teaching to the children’s teachers. This journal (under its previous name Journal of Statistics Education) has contributed to this aim (Eadie et al. Citation2019; Hoegh Citation2020; Hu Citation2020), by promoting Bayesian thinking into the undergraduate statistics curriculum. The next step is to promote statistical thinking into the high school and perhaps even primary school curriculum.
Supplementary Materials
The original data and replication files are included as supplementary materials, consisting of the following five files: Readme.pdf (instruction document), HMY_CodeBook.xlsx (excel codebook), HMY_Data_May2022. xlsx (dataset), HMY_Data.dta (STATA dataset), and HMY_do.do (replication file).
Supplemental Material
Download Zip (272.7 KB)Acknowledgments
We thank the editor, associate editor, and two referees for their constructive comments, and Jan van Maanen, Steven Tijms, and Aad van der Vaart for illuminating discussions.
Additional information
Funding
References
- Durbin, J. (1985), “Evolutionary Origins of Statisticians and Statistics,” in A Celebration of Statistics: The ISI Centenary Volume, eds. A. C. Atkinson, and S. E. Fienberg, New York: Springer.
- Durbin, J. (1988), “Is a Unified Consensus of Statistics Attainable?,” Journal of Econometrics, 37, 51–61.
- Eadie, G., Huppenkothen, D., Springford, A., and McCormick, T. (2019), “Introducing Bayesian Analysis with M&M’s: An Active-Learning Exercise for Undergraduates,” Journal of Statistics Education, 27, 60–67.
- Finucane, M. L., and Gullion, C. M. (2010), “Developing a Tool for Measuring the Decision-Making Competence of Older Adults,” Psychology and Aging, 25, 271–288. DOI: 10.1037/a0019106.
- Frederick, S. (2005), “Cognitive Reflection and Decision Making,” Journal of Economic Perspectives, 19, 25–42. DOI: 10.1257/089533005775196732.
- Gal, I. (2003), “Teaching for Statistical Literacy and Services of Statistics Agencies,” The American Statistician, 57, 80–84. DOI: 10.1198/0003130031469.
- Gauss, C. F. (1809), Theoria Motus Corporum Coelestium, Hamburg, Germany: Perthes and Besser. (Translated as “Theory of Motion of the Heavenly Bodies Moving About the Sun in Conic Sections” by C. H. Davis (1857), Boston: Little Brown & Co., Reprinted in 1963, New York: Dover).
- Greiner, B. (2015), “An Online Recruitment System for Economic Experiments,” Journal of the Economic Science Association, 1, 114–125. DOI: 10.1007/s40881-015-0004-4.
- Hanaki, N., Inukai, K., Masuda, T., and Shimodaira, Y. (2021), “Participants’ Characteristics at ISER-Lab in 2020,” Discussion paper no. 1141, Institute of Social and Economic Research, Osaka University, Osaka, Japan.
- Herbranson, W. T. (2012), “Pigeons, Humans, and the Monty Hall Dilemma,” Current Directions in Psychological Science, 21, 297–301. DOI: 10.1177/0963721412453585.
- Hoegh, A. (2020), “Why Bayesian Ideas Should be Introduced in the Statistics Curricula and How to Do so,” Journal of Statistics Education, 28, 222–228. DOI: 10.1080/10691898.2020.1841591.
- Hu, J. (2020), “A Bayesian Statistics Course for Undergraduates: Bayesian Thinking, Computing, and Research,” Journal of Statistics Education, 28, 229–235. DOI: 10.1080/10691898.2020.1817815.
- Jung, C. G. (1936), “The Concept of the Collective Unconscious,” in Collected Works (Vol. 9) (1959), p. 42.
- Kahneman, D. (2011), Thinking Fast and Slow, New York: Farrar, Straus and Giroux.
- Kahneman, D., and Tversky, A. (1972), “Subjective Probability: A Judgment of Representativeness,” Cognitive Psychology, 3, 430–454. DOI: 10.1016/0010-0285(72)90016-3.
- Laplace, P. S. (1814), Essai Philosophique sur les Probabilités, Paris, France: Courcier, 6th edition, 1840. (Translated as “A Philosophical Essay on Probabilities” by F. W. Truscott and F. L. Emory (1902), Reprinted 1951, New York: Dover).
- Magnus, J. R. (2022), “Gauss on Least-Squares and Maximum-Likelihood Estimation,” Archive for History of Exact Sciences, 76, 425–430. DOI: 10.1007/s00407-022-00291-w.
- Magnus, J. R., and Vasnev, A. (2008), “Using Macro Data to Obtain Better Micro Forecasts,” Econometric Theory, 24, 553–579. DOI: 10.1017/S0266466608080237.
- Matthews, R. A. J. (2001), “Why Should Clinicians Care About Bayesian Methods?” Journal of Statistical Planning and Inference, 94, 43–58.
- Oppenheimer, D. M., Meyvis, T., and Davidenko, N. (2009), “Instructional Manipulation Checks: Detecting Satisficing to Increase Statistical Power,” Journal of Experimental Social Psychology, 45, 867–872. DOI: 10.1016/j.jesp.2009.03.009.
- Pascal, B. (1665), Traité du Triangle Arithmétique, Paris: G. Desprez. Available at www.dspace.cam.ac.uk/handle/1810/244353
- Pólya, G. (1968), Patters of Plausible Inference (2nd ed.), Princeton, NJ: Princeton University Press.
- Popper, K. R. (1962), Conjectures and Refutations: The Growth of Scientific Knowledge, Abingdon-on-Thames, UK: Routledge.
- Rumsey, D. J. (2002), “Statistical Literacy as a Goal for Introductory Statistics Courses,” Journal of Statistics Education, 10(3). DOI: 10.1080/10691898.2002.11910678.
- Satake, E., and Vashlishan Murray, A. (2014), “Teaching an Application of Bayes’ Rule for Legal Decision-Making: Measuring the Strength of Evidence,” Journal of Statistics Education, 22(1). DOI: 10.1080/10691898.2014.11889692.
- Satake, E., and Vashlishan Murray, A. (2015), “An Alternative Teaching Method of Conditional Probabilities and Bayes’ Rule: An Application of the Truth Table,” Teaching Statistics, 37, 89–95. DOI: 10.1111/test.12080.
- Sharma, S. (2017), “Definitions and Models of Statistical Literacy: A Literature Review,” The American Statistician, 4, 118–133.
- Sheldrake, R. (1995), A New Science of Life, Rochester, VT: Park Street Press.
- Tijms, S. (2021), Chance, Logic and Intuition, Singapore: World Scientific.
- Toplak, M. E., West, R. F., and Stanovich, K. E. (2014), “Assessing Miserly Information Processing: An Expansion of the Cognitive Reflection Test,” Thinking and Reasoning, 20, 147–168. DOI: 10.1080/13546783.2013.844729.
- Tversky, A., and Kahneman, D. (1973), “Availability: A Heuristic for Judging Frequency and Probability,” Cognitive Psychology, 5, 207–232. DOI: 10.1016/0010-0285(73)90033-9.
- Tversky, A., and Kahneman, D. (1974), “Judgment under Uncertainty: Heuristics and Biases,” Science, 185, 1124–1131.
- Vredenduin, P. G. J. (1991), De Geschiedenis van Positief en Negatief, Groningen: Wolters–Noordhoff.