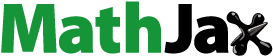
ABSTRACT
Estimation of population mean of study variable Y suffers loss of precision in the presence of high variation in the data set. The use of auxiliary information incorporated in construction of an estimator under Rank set sampling scheme results in efficient estimation of population mean. In this paper, we propose an efficient generalized family of estimators to estimate finite population mean of study variable under ranked set sampling utilizing information on an auxiliary variable. Bias and Mean Square Error (MSE) of the proposed generalized family of estimators are derived. The conditions of efficiency of proposed generalized family of estimators from competitor estimators are also derived. The applications of estimator are discussed using simulation study and real-life data sets for comparisons of efficiency. It is concluded that when correlation between study and auxiliary variables increases, the proposed generalized family of estimators proves to be the efficient estimator of population mean of the study variable.
1. Introduction
In many situations of practical interest, mainly in environmental and ecological studies, the variable of interest, say Y, is not easily observable in the sense that measurement may be expensive, time consuming, invasive or even destructive. Although data collection may be complex, ranking the potential sampled units with respect to an available auxiliary variable can often be relatively simple at no additional cost or for a very little cost. In those situations, where the variations in study variable is high and it is strongly correlated with auxiliary variable, the Ranked Set Sampling (RSS) proposed by Mclntyre (McIntyre, Citation1952) is more efficient as compared to Simple Random Sampling (SRS) (Patil et al., Citation1993, Citation1994; Stokes, Citation1977).
Literature on RSS has rapidly grown and several estimators, originally conceived for the SRS, have been re-proposed to estimate the mean of the study variable by changing their sampling design into RSS framework (Ali et al., Citation2021; Iqbal et al., Citation2020; Kadilar et al., Citation2009; Khan & Shabbir, Citation2016a, Citation2016b, Citation2016c; Mandowara & Mehta, Citation2013, Citation2016; Mehta & Mandowara, Citation2016; Pelle & Perri, Citation2018; Samawi & Muttlak, Citation1996; Singh et al., Citation2014; Vishwakarma et al., Citation2017). Motivated by these studies, and in line with many other contributions present in the literature section of this article, we propose an efficient generalized family of estimators by changing the sampling design of the estimator proposed by Shahzad et al. (Shahzad et al., Citation2019).
Notations under Ranked Set Sampling Design
Let Ω = {1,2, …, N} be a finite population of N units, Y the variable under study and X an auxiliary variable which is highly correlated with Y. Let µy and µx denote the population means of Y and X, respectively, and
the variances, Cy and Cx the coefficients of variation,
the correlation coefficient between X and Y,
and
the kurtosis and skewness, and
. Let us denote
as the pair of the ith-order statistics of X and the associated element Y in the jth cycle. Then the ranked set sample is,
To obtain biases and mean square error, we consider following notations under RSS:
: Deviation of ith cycle ranked mean from population mean µx.
: Deviation of ith cycle ranked mean from population mean µy.
: Cross product of the deviations.
To obtain biases and mean square error, we consider following notations under SRS:
2. Some Existing Estimators under SRS and RSS
Following are given some famous estimators along with their mean square errors.
Mandowara and Mehta (Mandowara & Mehta, Citation2013) proposed following estimators,
The Bias and MSE of ,
and
are,
Which are minimum for,
Vishwakarma, Zeeshan and Bouza (Vishwakarma et al., Citation2017) developed the following exponential estimator,
The MSE of is,
Shahzad et al. (Shahzad et al., Citation2019) introduced the generalized form of the estimators under simple random sampling given as,
Where are all constants. The bias and MSE of
are,
Where,
Which is minimum for,
The minimum MSE of is given by,
3. Proposed Generalized Family of Estimators under RSS
Motivated from Shahzad et al. (Shahzad et al., Citation2019), we propose the following generalized family of estimators under Ranked set sampling,
Where are unknown constants and
are suitably chosen known constants.
Derivation of Bias and Mean Square Error
Rewriting the above estimator with “e” terms under first order of approximation we get,
Where,
Subtracting on both sides,
For bias, we apply expectation on both sides of 3.2, the expression for bias of is given as,
For MSE, we apply square and expectation on both sides of 3.2, the expression for MSE of is given as,
Where,
For minimizing MSE, we obtained the optimum values of and
as follows:
and
Hence, the minimum Bias and MSE are given by,
The estimator of and
based on sample measurements are given as follows:
Where,
All of these are sample observations, so we will calculate these observations for quantifying Bias and MSE of our estimator for any given sample.
4. Efficiency Comparison
We derive the theoretical conditions to compare the efficiency of our proposed generalized family of estimators to their competitor estimators.
By (3.3) and (2.4),
if
By (3.3) and (2.5),
if
By (3.3) and (2.6),
if
By (3.3) and (2.8),
if
By (3.3) and (2.10),
if
Note: When these conditions are satisfied, the proposed estimators will perform more efficiently as compared to their competitor estimators.
5. Simulation Study
A hypothetical data for the study variable (Y) and auxiliary variable (X) is generated by using Bivariate Normal Distribution with parameters,
Samples of different values of n have been simulated 50,000 to calculate their average mean square errors and percent relative efficiencies. Percent Relative Efficiencies (PREs) of our proposed generalized family of estimators along with competitor estimators from literature have been presented in for different values of n and .
Table 1. PRE of Estimators by Simulation Study with = 0.4
Table 2. PRE of Estimators by Simulation Study with = 0.7
Table 3. PRE of Estimators by Simulation Study with = 0.8
Table 4. PRE of Estimators by Simulation Study with = 0.9
Table 5. PRE of Estimators by Simulation Study with = 0.99
shows that, when correlation coefficient of x and y equals to 0.4 and n = 20, our proposed estimator will be 257.31% more efficient then. In the same situation proposed estimator is 204.05%, 201.47%, 223.89% and 224.67% more efficient than
,
,
and
respectively. At the same correlation coefficient, when we increase the sample size by n = 30, our proposed estimator will be 262.10% more efficient then
. In the same situation proposed estimator is 201.59%, 198.80%, 237.06% and 232.31% more efficient than
,
,
and
respectively. shows that, when correlation coefficient of x and y equals to 0.7 and n = 20, our proposed estimator will be 274.65% more efficient then
. In the same situation proposed estimator is 204.64%, 201.69%, 233.34% and 231.27% more efficient than
,
,
and
respectively. At the same correlation coefficient, when we increase the sample size by n = 30, our proposed estimator will be 319.29% more efficient then
. In the same situation proposed estimator is 209.63%, 202.55%, 252.28% and 241.51% more efficient than
,
,
and
respectively. shows that, when correlation coefficient of x and y equals to 0.8 and n = 20, our proposed estimator will be 366.52% more efficient than
. In the same situation proposed estimator will be 218.39%, 209.52%, 249.15% and 241.89% more efficient then
,
,
and
respectively. At the same correlation coefficient, when we increase the sample size by n = 30, our proposed estimator will be 394.62% more efficient then
. In the same situation proposed estimator is 190.97%, 186.10%, 248.46% and 219.85% more efficient than
,
,
and
respectively.
shows that, when correlation coefficient of x and y equals to 0.9 and n = 20, our proposed estimator will be 437.43% more efficient then . In the same situation proposed estimator will be 210.49%, 193.42%, 292.25% and 236.98% more efficient then
,
,
and
respectively. At the same correlation coefficient, when we increase the sample size by n = 30, our proposed estimator will be 516.48% more efficient then
. In the same situation proposed estimator is 185.20%, 178.34%, 318.10% and 258.32% more efficient than
,
,
and
respectively. shows that, when correlation coefficient of x and y equals to 0.99 and n = 20, our proposed estimator will be 614.42% more efficient then
. In the same situation proposed estimator is 278.19%, 269.29%, 367.86% and 331.27% more efficient then
,
,
and
respectively. At the same correlation coefficient, when we increase the sample size by n = 30, our proposed estimator will be 680.86% more efficient then
. In the same situation proposed estimator is 227.26%, 216.05%, 351.55% and 305.36% more efficient than
,
,
and
respectively. Simulated results in show the trend that when we increase the sample size, efficiency of proposed estimators under RSS design also increases as compare to estimator under SRS design.
Results also revealed that as we increase the , proposed estimator in RSS performs more efficiently as compared to its competitor estimator in SRS (i.e.
). Therefore, we may say that as the correlation coefficient of x and y increases, the use of RSS is more appropriate as compared to SRS.
6. Real-Life Applications
To observe performances of the estimators, we use the following real-life data sets. The descriptions of these populations are given below.
Population I [source: (James et al., Citation2013)]
The summary statistics are given below.
Y: Acceleration of automobiles
X: Engine horsepower of automobiles
Objective: To estimate population mean of Acceleration of automobiles.
Population II [source: (Multiple Indicator Cluster Survey (MICS, 2018–19)]
The summary statistics are given as below.
Y: Body Mass Index (BMI)
X: Weight
Objective: To estimate population mean of Body Mass Index (BMI).
Population III [source: (Multiple Indicator Cluster Survey (MICS, 2018–19)]
The summary statistics are:
Y: Weight
X: Height
Objective: To estimate population mean of Weight.
Population IV [source: (Daly et al., Citation2001)]
The summary statistics are:
Y: Body Mass Index (BMI) of Crohn’s disease patients
X: Weight of Crohn’s disease patients
Objective: To estimate population mean of Body Mass Index (BMI) of Crohn’s disease patients.
Population V [source: (Husby et al., Citation2005)]
The summary statistics are:
Y: Body Mass Index (BMI)
X: Thigh Circumference
Objective: To estimate population mean of Body Mass Index (BMI).
Percent Relative Efficiencies (PREs) of our proposed generalized family of estimators along with competitor estimators from literature have been presented in for different real-life populations. shows that, when we consider the population I, our proposed estimator will be 363.74% more efficient then . In the same situation the proposed estimator is 150.18%, 150.18%, 155.15% and 153.49% more efficient than
,
,
and
respectively. shows that, when we consider the population II, our proposed estimator will be 418.12% more efficient than
. In the same situation proposed estimator will be 140.35%, 140.35%, 177.79% and 151.89% more efficient then
,
,
and
respectively. shows that, when we consider the population III, our proposed estimator will be 149.93% more efficient then
. In the same situation proposed estimator will be 118.81%, 118.81%, 127.66% and 121.52% more efficient then
,
,
and
respectively. shows that, when we consider the population IV, our proposed estimator will be 221.91% more efficient then
. In the same situation proposed estimator will be 107.11%, 107.11%, 100.32% and 120.72% more efficient then
,
,
and
respectively. shows that, when we consider the population V, our proposed estimator will be 410.47% more efficient then
. In the same situation proposed estimator will be 104.32%, 104.32%, 124.13% and 186.79% more efficient then
,
,
and
respectively.
Table 6. PRE of Estimators for Population I
Table 7. PRE of Estimators for Population II
Table 8. PRE of Estimators for Population III
Table 9. PRE of Estimators for Population IV
Table 10. PRE of Estimators for Population V
7. Conclusion
In this study, we proposed generalized family of estimators under RSS to estimate the finite population mean motivated from Shahzad et al. (Shahzad et al., Citation2019). The biases and MSEs of the proposed estimators were derived up to first order of approximation. The efficiency conditions for the proposed generalized estimator were also derived. On the basis of simulation study and real-life data sets, MSEs of all estimators have been computed and it is shown that the proposed generalized family of estimators are more efficient than the competitor estimators under SRS and RSS. It may concluded that with an increase in sample size and the proposed estimator in RSS performs more efficiently as compared to its competitor estimators in SRS (i.e.
).
Public interest statement
Estimation of population parameters with minimum mean square error is very important issue of survey sampling. Different sampling designs and estimators have been proposed by researchers to deal with this issue. In this study, we proposed a generalized family of estimators for estimating population mean under classical ranked set sampling. Mathematical comparison, Simulation study and real-life applications have been utilized for comparison of efficiency.
Acknowledgements
The authors wish to thanks DG-Bureau of Statistics Punjab for providing the data about Multiple Indicator Cluster Survey (MICS) for the year 2018–19.
Disclosure statement
No potential conflict of interest was reported by the authors.
Additional information
Funding
References
- Ali, A., Butt, M. M., Azad, M. D., Ahmed, Z., & Hanif, M. (2021). Stratified Extreme-cum-Median Ranked Set sampling. Pakistan Journal of Statistics, 37(3), 215–9.
- Daly, M. J., Rioux, J. D., Schaffner, S. F.,Hudson, T. J., & Lander, E. S. (2001). High-resolution haplotype structure in the human genome. Nature Genetics, 29(2), 229–232. https://doi.org/https://doi.org/10.1038/ng1001-229
- Husby, C. E., Stasny, E. A., & Wolfe, D. A. (2005). An application of ranked set sampling for mean and median estimation using USDA crop production data. Journal of Agricultural, Biological, and Environmental Statistics, 10(3), 354–373. https://doi.org/https://doi.org/10.1198/108571105X58234
- Iqbal, K., Moeen, M., Ali, A., & Iqabl, A. (2020). Mixture regression cum ratio estimators of population mean under stratified random sampling. Journal of Statistical Computation and Simulation, 90(5), 854–868. https://doi.org/https://doi.org/10.1080/00949655.2019.1710149
- James, G., Witten, D., Hastie, T.,& Tibshirani, R. (2013). An introduction to statistical learning (Vol. 112, pp. 18). springer.
- Kadilar, C., Unyazici, Y., & Cingi, H. (2009). Ratio estimator for the population mean using ranked set sampling. Statistical Papers, 50(2), 301–309. https://doi.org/https://doi.org/10.1007/s00362-007-0079-y
- Khan, L., & Shabbir, J. (2016a). A class of Hartley-Ross type unbiased estimators for population mean using ranked set sampling. Hacettepe Journal of Mathematics and Statistics, 45(3), 917–928.
- Khan, L., & Shabbir, J. (2016b). An efficient class of estimators for the finite population mean in ranked set sampling. Open Journal of Statistics, 6(3), 426–435. https://doi.org/https://doi.org/10.4236/ojs.2016.63038
- Khan, L., & Shabbir, J. (2016c). Hartley-Ross type unbiased estimators using ranked set sampling and stratified ranked set sampling. The North Carolina Journal of Mathematics and Statistics, 2, 10–22.
- Mandowara, V. L., & Mehta, N. (2013). Efficient generalized ratio-product type estimators for finite population mean with ranked set sampling. Austrian Journal of Statistics, 42(3), 137–148. https://doi.org/https://doi.org/10.17713/ajs.v42i3.147
- Mandowara, V. L., & Mehta, N. (2016). On the improvement of product method of estimation in ranked set sampling. Chilean Journal of Statistics (Chjs), 7(1), 43–53.
- McIntyre, G. A. (1952). A method for unbiased selective sampling, using ranked sets. Australian Journal of Agricultural Research, 3(4), 385–390. https://doi.org/https://doi.org/10.1071/AR9520385
- Mehta, N., & Mandowara, V. L. (2016). A is the modified ratio-cum-product estimator of finite population mean using ranked set sampling. Communications in Statistics-Theory and Methods, 45(2), 267–276. https://doi.org/https://doi.org/10.1080/03610926.2013.830748
- Multiple Indicator Cluster Survey (MICS, 2018-19). Unpublished Survey, Bureau of Statistics Punjab. [http://bos.gop.pk/mics]
- Patil, G. P., Sinha, A. K., & Taille, C. (1993). Relative precision of ranked set sampling: A comparison with the regression estimator. Environmetrics, 4(4), 399–412. https://doi.org/https://doi.org/10.1002/env.3170040404
- Patil, G. P., Sinha, A. K., & Taillie, C. (1994). Ranked set sampling. Handbook of Statistics, 12, 167–200.
- Pelle, E., & Perri, P. F. (2018). Improving mean estimation in ranked set sampling using the Rao regression-type estimator. Brazilian Journal of Probability and Statistics, 32(3), 467–496. https://doi.org/https://doi.org/10.1214/17-BJPS350
- Samawi, H. M., & Muttlak, H. A. (1996). Estimation of ratio using rank set sampling. Biometrical Journal, 38(6), 753–764. https://doi.org/https://doi.org/10.1002/bimj.4710380616
- Shahzad, U., Hanif, M., Koyuncu, N.,Luengo, A. V. G., & Khan, N. (2019). An efficient generalized family of estimators for mean estimation under simple random sampling. Investigación Operacional, 40(1), 28–45.
- Singh, H. P., Tailor, R., & Singh, S. (2014). General procedure for estimating the population mean using ranked set sampling. Journal of Statistical Computation and Simulation, 84(5), 931–945. https://doi.org/https://doi.org/10.1080/00949655.2012.733395
- Stokes, L. S. (1977). Ranked set sampling with concomitant variables. Communications in Statistics-Theory and Methods, 6(12), 1207–1211. https://doi.org/https://doi.org/10.1080/03610927708827563
- Vishwakarma, G. K., Zeeshan, S. M., & Bouza, C. N. (2017). Ratio and product type exponential estimators for population mean using ranked set sampling. Investigación Operacional, 38(3), 266–271.