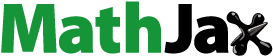
ABSTRACT
Background
In terms of hospitalization risk for the elderly, the evidences for adverse effects and economic losses of air pollutants on different types of cardiovascular diseases (CVDs) are limited. This study aims to quantify the economic losses of five major CVDs affected by air pollutants in Southwestern China.
Methods
Hospitalization information of 358,271 participants in the top five CVDs among the elderly population was collected over a 4-year time span. The generalized additive model (GAM) was used to estimate the short-term exposure response relationship between air pollutants and elderly inpatients with CVDs. The cost of illness method (COI) was used to evaluate the corresponding direct and indirect costs, and we refer to this in the text as economic losses.
Results
Air pollutants significantly increase the risk of hospitalisation in elderly patients with coronary heart disease (CHD), myocardial ischaemia (MI), pulmonary heart disease (PHD), hypertension, and cardiac arrhythmia. CO, NO2, PM10 and SO2 were significant increase the risk of CHD, with the RRs were 0.09, 2.59, 0.31, and 6.54, respectively; CO, NO2, O3, PM10, PM2.5, and SO2 were notably increased the risk of MI, with the RRs were 0.16, 4.19, 0.76, 0.79, 0.92, and 7.49, respectively; CO, NO2, PM10, PM2.5, and SO2 were distinctly increased the risk of PHD, with the RRs were 0.14, 2.79, 0.75, 1.00, and 3.86, respectively; NO2 and SO2 significant increase the risk of hypertension, with the RRs were 1.41 and 5.94, respectively; CO, NO2, PM10, and SO2 were distinctly increased the risk of arrhythmia, with the RRs were 0.09, 2.23, 0.36, and 5.41, respectively. Furthermore, we quantify the economic losses of five major CVDs affected by air pollutants. The economic burden of air pollution on patients with CHD, hypertension, PHD, cardiac arrhythmia, and MI were 66.38, 36.58, 5.76, 5.66 5.21 million yuan, respectively.
Conclusion
Our study found that short-term exposure to pollutants can have a significant impact on hospital admissions for all types of CVDs in the elderly. In terms of hospitalisation rates, SO2 and NO2 had a significant effect on different types of CVDs. Moreover, we found that air pollutants cause a heavy economic burden on patients with hypertension and CHD. It follows that reducing the concentration of air pollutants is an effective way to bring potential health benefits as well as economic benefits to local residents. Government departments should actively formulate relevant policies to reduce the physical and mental damage caused by air pollution.
1. Introduction
Cardiovascular diseases (CVDs) are the leading cause of death globally according to the World Health Organization (WHO, Citation2021). In 2019, the total number of global CVDs cases was 523 million and the number of deaths directly resulting from CVDs reached 18.6 million (Roth et al., Citation2020). A large number of studies have already shown that exposure to pollutants in the air is related to hospitalizations and deaths caused by CVDs (Hystad et al., Citation2020; Y. Wang et al., Citation2021; Kim et al., Citation2017; Bae et al., Citation2022). In addition, some systematic reviews showed that exposure to PM2.5, PM10, NO2, SO2, and CO could increase the events of CVDs, such as coronary heart disease (CHD), myocardial ischemia (MI), atrial fibrillation (AF), and hypertension (K. K. Lee et al., Citation2020; C. Liu et al., Citation2022; Pranata et al., Citation2020; Zhu et al., Citation2021). The main forms for air pollutants affecting the circulatory system include altering vascular tone, disrupting the balance of autonomic regulation of nerves, systemic inflammation, oxidative stress response, and abnormal epigenetic changes, etc. Based on estimates from the Global Burden of Disease, there were approximately 4.14 million excess deaths globally in 2019 that were linked to PM2.5 exposure. Over half of these deaths occurred in China and India, with 3.54 million excess cardiovascular deaths specifically attributed to air pollution (representing 85.5% of the total) (T. Li et al., Citation2023). These manifestations are considered to be the intermediary and influencing factors that constitute the correlation between air pollutants and CVDs (Yang et al., Citation2019).
An increase in the concentrations of air pollutants could distinctly affect cardiovascular health, especially that of older residents who are generally more susceptible to CVDs than their younger counterparts. It cannot only entail cardiac ischemia or infarction symptoms, but also augment the hospitalization risk and economic burden of CVDs (Zhou et al., Citation2021). According to the European Study of Cohorts for Air Pollution Effects Project (ESCAPE), long-term exposure to PM2.5 was associated with an increase in nonfatal acute coronary events, and those participants who were over 60 years had the highest risk associated with PM2.5 compared to younger adults (Cesaroni et al., Citation2014). Miller’s correlation study between long-term exposure to air pollution and the incidence of cardiovascular events found that people above 70 years had a higher risk (Miller et al., Citation2007). In a systematic review by Yue, under exposure to PM2.5 and PM10, people aged 65 and above may be more likely to suffer from AF (Yue et al., Citation2021).
Apart from health hazards, CVDs hospitalization caused by air pollution more often than not leads to economic losses as well. By analyzing the impact of air pollution in Southwest China, Li X found that air pollutants caused an economic loss of $144.3 million for people with CVDs, which accounted for 0.028% of the local GDP (X. Li et al., Citation2022). CVDs caused by environmental pollution increase the risk of hospitalisation, and the direct and indirect costs of hospitalisation impose an economic loss on individuals and society. In an aging society, the elderly unfortunately face a higher risk of CVDs, coupled with heavier economic burden due to the sharp reduction in income. It is valuable to evaluate the CVDs economic burden caused by air pollution from a broader perspective. Recently, Anoop’s study found that reducing the concentration of PM2.5 by 3.9ug/m3 would prevent 7,978 heart failure hospitalizations across the USA and would save around $307 million dollars per year (Shah et al., Citation2013). A study conducted in a cardiovascular hospital of Belgium found that with a 10% reduction in PM10, PM2.5, and NO2 respectively, the corresponding annual cost savings were estimated at $5.2 million euros, $5.0 million euros, and $8.4 million euros, respectively (Devos et al., Citation2015). In China, the total 1-year excess medical losses of CVDs caused by PM2.5 pollutant in Beijing were $147.9 million dollars (Jiang et al., Citation2020), while in Wuhan, the CVDs hospitalizations were attributable to PM2.5 and PM10, equaling an economic loss of $29.27 and $19.34 million RMB (X. Wang et al., Citation2020).
However, the epidemiological evidence and the comprehensive economic burden including direct and indirect cost from variant CVDs attributed to air pollutants are still beyond our knowledge, especially in developing areas. We explore the impacts of air pollutants on CVDs hospitalization risk and economic losses for targeted population. It is also necessary to provide empirical evidence to prevent different types of CVDs from becoming prevalent in unfavorable atmosphere.
2. Materials and methods
2.1. Study area and population
Chengdu, located in southwestern China (latitude 30°05`~31°26`N, longitude 102°54` ~104°53`E), is one of the most densely populated cities in the country. By the end of 2020, Chengdu had a population of 20.93 million in an area of 14,335 km2. Due to the rapid economic development and the continuous influx of population, the continuous consumption of natural resources has imposed rising burden on local environment. Meanwhile, the climate of Chengdu is characterized by humidity, and its basin terrain is very unfavorable for the upward diffusion of air pollutants. This may aggregate pollution when meteorological factors are involved (Ning et al., Citation2019), causing serious damage to the health of local residents (Ning et al., Citation2018).
2.2. Data collection
The data for this study came from the local Medical Insurance Database, which contained all admission records of local insured residents in 1,161 public or private hospitals in the region. The residents obtained medical insurance by participating in local Urban Employee Basic Medical Insurance (UEBMI) and Urban and Rural Residency Basic Medical Insurance (URRBMI), both of which covered more than 95% of the permanent population. This study included hospitalization records for CVDs of insured residents over 60 years between January 2014 and November 2017.
As the data did not include personal identifiers, there was no explicit personal consent. Each patient record consisted of the name of the medical institution, gender, age, dwelling address, date of admission and discharge, disease classification (10th edition of the International Classification of Diseases, ICD-10), and detailed medical expenses incurred during hospitalization. According to the definition of ICD-10 digital code, the CVDs code in this study ranged from I00 to I99. Taking the code to one decimal as disease classification, and considering the ranking of inpatients number for different CVDs within 4 years, the top five CVD diseases were as follows: hypertension (I10.0-I10.11), coronary heart disease (CHD, I25.1), myocardial ischemia (MI, I25.6), pulmonary heart disease (PHD, I27.9), and arrhythmia (I49.9) were successively extracted from the dataset.
Air pollution exposure data was obtained through the China Air Quality Online Monitoring Platform (https://www.aqistudy.cn/), and the values were 24-hour averages of pollutant concentrations across 18 air monitoring stations in Chengdu. The main monitored pollutants were daily average concentration of PM2.5 (ug/m3), PM10 (ug/m3), SO2 (ug/m3), NO2 (ug/m3), CO (ug/m3), and O3 (ug/m3). Meteorological data were obtained through the online monitoring system of Chengdu Meteorological Bureau, including daily average temperature (°C), average air pressure (kpa), average wind speed (m/s), and relative humidity (%). Demographic data and GDP were collected on the website of the National Bureau of Statistics (https://www.stats. gov.cn/).
2.3. Methods
The statistical analysis plan consisted of three steps: estimating the exposure response relationship function, calculating the number of CVD admissions caused by various types of air pollutants, and evaluating the corresponding economic losses caused by hospitalization.
2.3.1. Estimating exposure-response function
We applied a generalized additive model to analyze the relationship between air pollutants and the number of hospital admissions for CVDs (Tajudin et al., Citation2019). Due to the different degrees of dispersion of the numbers of daily hospitalizations for different CVDs, the quasi-Poisson function and the Poisson function were, respectively employed in the link function of different CVDs models. Among them, hypertension and CHD adopted Poisson distribution, and the other three adopted quasi-Poisson distribution (P. Zhang & Zhou, Citation2020).
We used natural spline functions to control the effects of temperature, relative humidity, pressure and long-term trends (Sui et al., Citation2021). The model formula is as follows:
Y: Daily number of CVDs inpatients;
μ: The estimator of Y;
α: The intercept;
β: The coefficient;
Z: The concentration of Air pollutants;
NS: The natural spline function;
df: The degree of freedom;
Temp: Daily average temperature;
RH: Daily average relative humidity;
Time: Calendar time, to control long-term temporal trends;
Pressure: Daily average pressure
Wind:Wind is the EMA of wind speed where the lag range is set as 0–7 days.
Dow: Day of week, a dummy variable to control the systematic variation within a week;
SH: Statutory holiday, a dummy variable to control the systematic variation within holiday.
where quasi-Poisson distribution was used to characterize the potential overdispersion. Because meteorological factors, such as pressure, humidity, temperature, time and wind, may confound the results, in Equation (1) we also adjust for such confounding effects by using NS1(Temp), NS2(RH), NS3(Time), NS4(Pressure) and wind. Among them, NS1(Temp), NS2(RH) and NS4(Pressure) were characterized by natural cubic spline functions where the degrees of freedom (df) were set as 3, respectively; Windt is the EMA of wind speed where the lag range is set as 0–7 days. The term NS3(Time), a natural cubic spline function whose df is 4 per year, was used to characterize the long-term trend. All the mentioned df values and the employed confounders forms were determined by minimizing the Bayesian information criterion (BIC). The procedure of selecting df for all covariates is included in the Supplementary material (Figure S1). In addition, DOW and SH were used to adjust for the effects from weekends and holidays, respectively.
In an attempt to explore the overall impact of air pollutants on inpatients with CVDs, we set a multi-day moving average lag line (from lag 00 to lag 07), which was fitted a separate model with the same parameter settings as the main model (Y. Liu et al., Citation2017). Results were presented as percentage change in the number of hospital admissions and confidence interval (CI) for each 10ug/m3 increase in pollutant concentration (D.-W. Lee et al., Citation2021). All analyses were done in R software version 4.0.5, using the ‘mgcv’ software package; the statistical test was two-sided, and p value of 0.05 was considered as statistically significant.
2.3.2. Calculating the number of hospital admissions for CVDs due to air pollutants
Based on the exposure-response function calculated by the GAM model results, we further adopted the attribution risk method to obtain the number of air pollutant-related CVDs hospital admissions (Qiu et al., Citation2019). In addition, we referred to the integer number of each pollutant’s average concentrations to determine the reference concentrations of pollutants in this study (63 ug/m3 for PM2.5, 103 ug/m3 for PM10, 15 ug/m3 for SO2, 52 ug/m3 for NO2, 1079 ug/m3 for CO, and 93 ug/m3 for O3). Because the impact of various pollutants on the risk of CVDs hospitalization was different, the coefficient is the sum of the coefficients with significant influence in the previous week. The formula is as follows:
i: The day number of the study period (from 1 to 1430);
j: Air pollutants, PM10, PM2.5, SO2, NO2, CO, O3;
ARij: Attributable risk;
βj: The exposure-response coefficient;
xij: The daily concentration of air pollutants;
x0j: The reference concentration;
Nij: The number of daily hospital admissions of CVDs;
AN: The total attributable number of CVDs.
2.3.3. Calculating the economic losses of hospitalization
In order to estimate the economic losses related to hospitalization caused by air pollutants, we adopted the cost of illness method (COI) (Wei et al., Citation2019). The COI method also considered the medical expenses (direct costs of disease) of the inpatients and the economic losses during the hospitalization (indirect cost of disease) (Ginsberg et al., Citation2016). The per capita GDP and disease costs of different years were adjusted by CPI of the current year.
Ch: The average treatment cost each admission calculated according to our data in Chengdu in 2014–2017;
dGDP: Daily per capita GDP;
meanTh: The average length of stay each admission;
ΔC: The total economic losses due to air pollution during the study period.
3. Results
The descriptive statistical information of CVDs inpatients, air pollutants, and meteorological factors in Chengdu during the 4 years from 2014 to 2017 were summarized (). In the 1,430 observation days, there were a total of 358,271 hospitalized cases, with an average of 251 cases per day. As the top two ailments in CVDs groups, hypertension (n = 154,757) and CHD (n = 148,279) accounted for 84.58%, while PHD (n = 23,019), arrhythmia (n = 17,434), and MI (14,782) took up 15.42%. The local daily average temperature was 16.79°C, and the average relative humidity was 54.92%. During the study period, the daily average concentrations of PM2.5, PM10, SO2, NO2, CO and O3 were 62.58, 103.05, 14.88, 52.04 , 1080, and 92.71 µg/m3 respectively. In addition, we set the normal standard based on the integral average of the concentrations of each pollutant during the study period.
Table 1. Information of air pollutant concentration, meteorological factors, and CVD in patients in Chengdu from 2014 to 2017
Demographic and economic status and the CVDs inpatients’ costs in Chengdu by years were presented (). The cumulative annual hospitalization rate for top five CVDs among elderly population ranged from 2.67% to 3.63%, and the denominator in the calculation was the number of elderly over 60 years in Chengdu. The average hospitalization costs in different years were 8329.471, 8715.434, 8803.879, and 8,713.03 yuan, respectively. In addition, the table shows the average medical fee and the change in the number of inpatients for each of the five diseases year by year during 2014–2017.
Table 2. Demographic, economic status, and the CVD inpatients’ costs in Chengdu by years
The attributable risk and number, economic losses caused by air pollutants accounted for the CVD inpatients in Chengdu from 2014 to 2017 were presented (). For patients with CHD, hypertension, PHD, arrhythmia, and MI diseases, the economic losses attributable to air pollutants were 66.38, 36.58, 5.76, 5.66, and 5.21 million yuan, respectively.
Table 3. The attributable risk and number, economic losses caused by air pollutants accounted for the CVD inpatients in Chengdu from 2014 to 2017
Correlation was explored among between and among air pollutants, meteorological factors, and the number of CVDs inpatients (Figure ). Except for O3, all pollutants had a strong positive correlation with each other. CVDs had a weak positive correlation with the five pollutants of PM2.5, PM10, SO2, NO2, and CO, and a weak negative correlation with O3.
Because of the strong correlation and potential collinearity between various pollutants, the single pollutant effect model was suitable for exposure effect relationship. As the impact of pollutants on health usually had a certain hysteresis effect, the research was generated by the GAM model of single pollutant multi-day moving average hysteresis for each type of CVDs. We found that PM2.5, PM10, NO2, CO2, SO2 and O3 were relevant to hospitalization rate but their effects on CHD, MI, PHD, hypertension, and arrhythmia differed significantly (p = 0.05). We presented the regression results for the CHD and hypertension, which have the heaviest economic losses attributable to pollutants (Figures and Figure ), the results of the air pollutant model for the remaining three diseases were placed in Supplementary Material, with the details shown in Figure S2–S4.
Figure 2. Percentage of change in the number of daily inpatients for CHD affected by air pollutants on the multi-day moving average lag line.
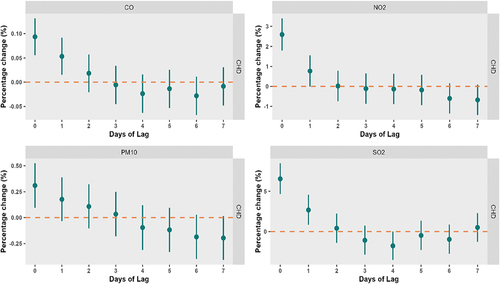
Figure 3. Percentage of change in the number of daily inpatients for hypertension affected by air pollutants on the multi-day moving average lag line.
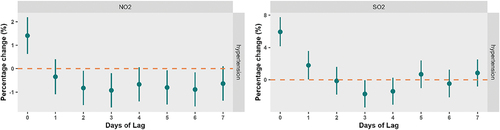
shows the results of the air pollutant model for inpatients with CHD. CO significantly affected admission rate between Lag Days 0 and 1, and NO2 showed impact at Lag Days 0 and 1. While PM10 was significant only at Lag Day 0, SO2 was remarkable from Lag Days 0 and 1. The increase of CO, NO2, PM10 and SO2 by 10ug/m3 (CO is 100ug/m3) were related to the number of inpatients during the observation period, and the coefficients were 0.09% (95% CI: 0.06–0.13%), 2.59% (95% CI: 1.79–3.39%), 0.31% (95% CI: 0.10–0.52%), and 6.54% (95% CI: 4.65–8.46%), respectively.
Figure shows the results of the air pollutant model for inpatients with hypertension. NO2 only had effect at Lag Day 0, while SO2 was significant at Lag Days 0 and 1. The increase of NO2 and SO2 by 10ug/m3 was related to the number of inpatients during the observation period, and the coefficients were 1.41% (95% CI: 0.63–2.19%) and 5.94% (95% CI: 4.16–7.74%), respectively.
During the study period, the total economic losses of CVDs hospitalization for elderly residents caused by air pollutants amounted to 119.6 million yuan. In terms of disease types, the economic losses from CHD were the heaviest (about 66.38 million yuan) and the economic losses of MI was the smallest (about 5.21 million yuan).
4. Discussion
Our study focused on the correlation between different air pollutants and CVDs hospitalization among elderly residents. We found that the main air pollutants that affected the risk of CVDs hospitalization in the local elderly were nitrogen oxides (NO2) and sulfur dioxide (SO2). These findings go in line with the conclusions of Saeed’s studies (Motesaddi Zarandi et al., Citation2022). In terms of CVDs types, we found that short-term exposure to air pollutants played a role in CHD, MI, PHD, hypertension, and arrhythmia, among which the adverse effects were the strongest on CHD and weakest on MI. The economic burden of diseases among the elderly caused by air pollutants accumulated to 119.6 million yuan.
Various air pollutants had different correlations in the different CVDs. The top five hospitalization rates for different CVD types were hypertension, CHD, PHD, arrhythmia, and MI. Although no significant correlation was found between the daily hospitalizations of hypertension and arrhythmia and the air pollutants. However, for CHD, MI, and PHD, which are prone to acute symptoms, these diseases showed a strong correlation with the air pollutants. This indicates that the main impact of air pollutants on the hospitalization risk of CVDs in the elderly may be concentrated in acute CVDs or acute attacks of chronic CVDs.
In studies related to CHD, Chen’s study found that after controlling for daily temperature, relative humidity, and barometric pressure, an IQR concentration increase in levels of sulfur dioxide (SO2) (13 mu g/m(3)) was associated with 7.8% increases in PHD-related mortality in people aged 60 and older (Chen et al., Citation2019). Although we were studying the short-term exposure of pollutants, SO2 was also the pollutant with the highest attributable risk ratio, with a percentage variation of 6.53%. Wang’s study also found that NO2 had a remarkable effect on coronary artery calcification (CAC), a marker of coronary atherosclerosis (M. Wang et al., Citation2019). We found that SO2, NO2, PM10 and CO have an impact on the risk of CHD hospitalization, of which SO2 and NO2 have the stronger impact. This is consistent with Gao’s study (Gao et al., Citation2023).
In our study, we found that all six pollutants have an impact on the risk of MI hospitalization, which is consistent with Davoodabadi’s study (Davoodabadi et al., Citation2019). We also found that the economic losses of hospitalisation for patients with MI were the smallest among five types of CVDs. On the one hand, because MI is an acute cardiovascular disease, the length of hospitalization is shorter than that of patients with chronic cardiovascular diseases, on the other hand, the average hospitalization costs for MI are smaller than for other cardiovascular diseases. This is consistent with Ioannides-Demos’s findings (Ioannides-Demos et al., Citation2010).
Up to now, few studies have been conducted on the relationship between PHD and air pollutants. Chen’ s study on the relationship between air pollutants and PHD mortality in Chengdu during the same period found that PM2.5, SO2, NO2, and O3 all had effects on the mortality of PHD, but as all causes of death were included in that study, it cannot reflect the mortality directly caused by PHD (Chen et al., Citation2019). This is slightly different from our research conclusion. In our study, O3 failed to affect the increase in PHD hospitalization rate, while SO2 and NO2 had a strong influence on PHD hospitalization rate. We thought that the lack of effect may be due to the pathogenesis by which pollutants affect PHD is different from those that cause death in PHD patients.
In similar studies on the impacts of air pollutants on hypertension, Chiu’s study in Taipei concluded that fine particulate matter has no significant effect on the hospitalization rate for the disease (Chiu, Tsai, et al., Citation2017). The review conclusion of Guo’s study indicated that among adults, the long-term effects of NO2 and SO2 on the incidence of hypertension events are significant (Guo et al., Citation2023). We found that the impact of air pollutants on hospitalization for hypertension tends to have a noticeable long-term effect, but the short-term effects are not obvious with the exception of NO2 and SO2.In addition, the study found that the economic losses of hospitalization for hypertensive patients was very high, which we guess is due to the high number of hospitalizations for hypertension.
Regarding researches on arrhythmia, Zhang’s study linked long-term exposure in fine particulate matter to an increase in patients with arrhythmia (Z. Zhang et al., Citation2020). Shahrbaf’s study suggested that both short-term and long-term exposure to air pollutants had an effect on the occurrence of arrhythmia (Shahrbaf et al., Citation2021). This is broadly consistent with our findings. In our study, arrhythmia was associated with exposure in CO, NO2, PM10 and SO2, but not associated with O3, which may be due to the small number of local daily hospitalizations for arrhythmia and short-term exposure to air pollutants.
O3 in our study did not show a significant correlation with the risk of CVDs hospitalization (except MI), and O3 even expressed protective effect in CHD and hypertension, which is contrary to the conclusions of some studies, such as Tian’s and Zheng’s work (Tian et al., Citation2018; Zheng et al., Citation2021). The possible reason was that the annual average concentration of O3 in this area was low, which had little impact on the health of the population.
The relationship between O3 and CVD events has always been controversial, and the conclusions of related studies are different. Halonen’s study believed that O3 has no significant effect on the population’s CVDs hospitalization or death (Halonen et al., Citation2010). The meta-analysis conclusion of Anoop’s study also suggested that O3 has no significant effect on the population’s heart failure hospitalization or death (Shah et al., Citation2013). In a pooled analysis of six European cohorts within the ELAPSE project, Kathrin Wolf found that long-term exposure to low levels of ozone pollutants did not increase the incidence of CVDs or stroke (Wolf et al., Citation2021). However, Chiu’s study believed that at high levels, O3 has a significant effect on increasing the hospitalization rate of MI (Chiu, Weng, et al., Citation2017). Raza’s study considered that rising ozone concentration can affect the mortality of hospitalized patients with MI (Raza et al., Citation2018), which is consistent with our study. Furthermore, it showed protective factors in CHD and hypertension, which may be related to local geographical conditions, climate, and local culture and lifestyle. In summer when O3 concentration is high, the elderly people possibly reduce outdoor activities. In addition, the leisure and entertainment activities of elderly residents in Chengdu are mostly at home and in community (such as playing mahjong). This may partly explain the formation of protective factors, and further research is needed in the future.
Air pollution certainly imposes a huge economic burden of disease on elderly residents. Xie’s study in 74 Chinese cities between 2016 and 2017 found that the additional cost of treating CHD caused by PM2.5 alone amounted to $68.5 million (Xie et al., Citation2021). Zhao’s study in Beijing estimated that in 2012, the medical costs of CVDs caused by PM10 pollutant reached 106.86 million yuan, and the economic losses of human capital caused by premature deaths resulted from CVDs reached 284.56 million yuan (Zhao et al., Citation2016). The economic losses of pollutants on different types of CVDs differed immensly in our study. On the one hand, the daily average number of hospitalizations for CHD and hypertension were prominently larger than those for other types of CVDs. Therefore, economic losses of pollutants on patients with CHD and hypertension were more serious than others. At the same time, because pollutants had a stronger attributable risk to CHD and hypertension, there were more patients with CHD or hypertension attributable to pollutants, too, and the economic losses caused by them was also higher.
5. Conclusion
Our research took the central city of Chengdu in southwestern China as an example, and explored the impact of air pollutants on the hospitalization risk among local elderly residents for CVDs in a basin terrain. We found that the local air pollutants in Chengdu were significantly impact on the CVDs hospitalization risk among the elderly and caused serious economic losses. The extent to which the hospitalization risk of different CVDs was affected by air pollutants is also different, which is very interesting. PHD is the most severely hospitalized CVDs affected by air pollutants among elderly residents, and mainly from SO2 and NO2. Correspondingly, The effect of O3 on cardiovascular diseases is weak. Which provides a reference for studying the pathogenesis of various CVDs.
6. Strength and Limitation
Based on large-sample population registration data and covering a relatively long time span continuously, our study not only effectively explored the impact of different air pollutants on the cardiovascular health among the elderly, but also provided a contrast on the hospitalization risk of different CVDs by air pollutants short-term impact. These results provide strong evidence for studying the disease burden of CVDs in the context of aging problem.
Despite these findings, however, our study also has some limitations. First, our classification of diseases was only accurate to one decimal of ICD code. This means that some unrelated diseases could not be excluded. Second, as the data comes from the Sichuan Provincial Medical Insurance Database, there is a possibility of misclassification, which is unavoidable and will cause a certain degree of bias. Third, the population flow and the declining of hospital medical services on holidays affected the occurrence of hospitalization events, and the substitution of mean values failed to accurately reflect the actual situation. Finally, we cannot rule out bias due to unmeasured confounding factors, such as occupational history. Future studies of this kind are expected to provide better solutions.
Authors’ contributions
Conceptualization, XY. Z, SM. J, N. W and PY.Q; methodology, XY. Z, SM.J, N. W, L.W, P.Z and W.W; software, SM.J and W.W; validation, XY. Z, N. W and SM.J; formal analysis, SM.J; investigation, XY.Z.; writing—original draft preparation, SM.J and N. W; writing—review and editing, SY.L, PY.Q and XY.Z; supervision, XY.Z.; project administration, XY.Z.; funding acquisition, XY.Z.
Abbreviations
CVDs, | = | cardiovascular diseases; |
GAM, | = | generalized additive model; |
COI, | = | cost of illness method; |
CHD, | = | coronary heart disease; |
MI, | = | myocardial ischemia; |
PHD, | = | pulmonary heart disease; |
GDP, | = | Gross Domestic Product; |
HR, | = | Hazard Ratio; |
AR, | = | attributable risk; |
AN, | = | attributable number; |
CI, | = | confidence interval; |
PM10 | = | particulate matter with an aerodynamic diameter less than 10 mm; |
PM2.5 | = | fine particles with an aerodynamic diameter less than 2.5 mm; |
SO2 | = | Sulfur dioxide; |
NO2 | = | Nitrogen dioxide; |
CO, | = | Carbon monoxide; |
O3 | = | Ozone. |
Availability of data and materials
The dataset generated during and/or analyzed during the current study are not publicly available due to ethics reason.
Consent for publication
All authors have read and agreed to the published version of the manuscript.
Ethics approval and consent to participate
The ethical committee of West China school of Public Health and West China Fourth Hospital approved the research.
Supplementary_materials.docx
Download MS Word (742 KB)Acknowledgements
We are sincerely grateful to all participants for their support of this study.
Disclosure statement
No potential conflict of interest was reported by the author(s).
Supplementary material
Supplemental data for this article can be accessed online at https://doi.org/10.1080/27658511.2024.2362515
Additional information
Funding
References
- Bae, H. R., Chandy, M., Aguilera, J., Smith, E. M., Nadeau, K. C., Wu, J. C., & Paik, D. T. (2022). Adverse effects of air pollution-derived fine particulate matter on cardiovascular homeostasis and disease. Trends in Cardiovascular Medicine, 32(8), 487–12.
- Cesaroni, G., Forastiere, F., Stafoggia, M., Andersen, Z. J., Badaloni, C., Beelen, R., Caracciolo, B., de Faire, U., Erbel, R., Eriksen, K. T., Fratiglioni, L., Galassi, C., Hampel, R., Heier, M., Hennig, F., Hilding, A., Hoffmann, B., Houthuijs, D. … Brunekreef, B. (2014). Long term exposure to ambient air pollution and incidence of acute coronary events: Prospective cohort study and meta-analysis in 11 european cohorts from the escape project. BMJ: British Medical Journal, 348(Jan 21), f7412. https://doi.org/10.1136/bmj.f7412
- Chen, J., Zeng, J., Shi, C., Liu, R., Lu, R., Mao, S., & Zhang, L. (2019). Associations between short-term exposure to gaseous pollutants and pulmonary heart disease-related mortality among elderly people in chengdu, china. Environmental Health: A Global Access Science Source, 18(1), 64. https://doi.org/10.1186/s12940-019-0500-8
- Chiu, H. F., Tsai, S. S., & Yang, C. Y. (2017). Short-term effects of fine particulate air pollution on hospital admissions for hypertension: A time-stratified case-crossover study in taipei. Journal of Toxicology and Environmental Health Part A, 80(5), 258–265. https://doi.org/10.1080/15287394.2017.1321095
- Chiu, H. F., Weng, Y. H., Chiu, Y. W., & Yang, C. Y. (2017). Short-term effects of ozone air pollution on hospital admissions for myocardial infarction: A time-stratified case-crossover study in taipei. Journal of Toxicology and Environmental Health Part A, 80(5), 251–257. https://doi.org/10.1080/15287394.2017.1321092
- Davoodabadi, Z., Soleimani, A., Pourmoghaddas, A., Hosseini, S. M., Jafari-Koshki, T., Rahimi, M., Shishehforoush, M., Lahijanzadeh, A., Sadeghian, B., Moazam, E., Mohebi, M. B., Ezatian, V., Rabiei, K., & Sarrafzadegan, N. (2019). Correlation between air pollution and hospitalization due to myocardial infarction. ARYA Atherosclerosis, 15(4), 161–167. https://doi.org/10.22122/arya.v15i4.1834
- Devos, S., Cox, B., Dhondt, S., Nawrot, T., & Putman, K. (2015). Cost saving potential in cardiovascular hospital costs due to reduction in air pollution. Science of the Total Environment, 527-528, 413–419. https://doi.org/10.1016/j.scitotenv.2015.04.104
- Gao, Y., Sheng, W., & Yang, Y. (2023). Air pollution and coronary heart disease-related hospital visits in Beijing, China: Time-series analysis using a generalized additive model. Environmental Science and Pollution Research International, 30(13), 36938–36951. https://doi.org/10.1007/s11356-022-24803-x
- Ginsberg, G. M., Kaliner, E., & Grotto, I. (2016). Mortality, hospital days and expenditures attributable to ambient air pollution from particulate matter in israel. Israel Journal of Health Policy Research, 5(1), 51. https://doi.org/10.1186/s13584-016-0110-7
- Guo, L. H., Zeeshan, M., Huang, G. F., Chen, D. H., Xie, M., Liu, J., & Dong, G. H. (2023). Influence of air pollution exposures on cardiometabolic risk factors: A review. Current Environmental Health Reports, 10(4), 501–507. https://doi.org/10.1007/s40572-023-00423-6
- Halonen, J. I., Lanki, T., Tiittanen, P., Niemi, J. V., Loh, M., & Pekkanen, J. (2010). Ozone and cause-specific cardiorespiratory morbidity and mortality. Journal of Epidemiology and Community Health, 64(9), 814–820. https://doi.org/10.1136/jech.2009.087106
- Hystad, P., Larkin, A., Rangarajan, S., AlHabib, K. F., Avezum, Á., & Calik, K. B. T., et al. (2020). Associations of outdoor fine particulate air pollution and cardiovascular disease in 157 436 individuals from 21 high-income, middle-income, and low-income countries (pure): A prospective cohort study. The Lancet Planetary Health, 4, e235–e245. https://doi.org/10.1016/S2542-5196(20)30103-0
- Ioannides-Demos, L. L., Makarounas-Kirchmann, K., Ashton, E., Stoelwinder, J., & McNeil, J. J. (2010). Cost of myocardial infarction to the Australian community: A prospective, multicentre survey. Clinical Drug Investigation, 30(8), 533–543. https://doi.org/10.2165/11536350-000000000-00000
- Jiang, Y., Jiang, S., & Ni, W. (2020). Burden of cardiovascular diseases associated with fine particulate matter in beijing, china: An economic modelling study. BMJ Global Health, 5(10), e003160. https://doi.org/10.1136/bmjgh-2020-003160
- Kim, H., Kim, J., Kim, S., Kang, S. H., Kim, H. J., Kim, H., Heo, J., Yi, S.-M., Kim, K., Youn, T.-J., & Chae, I.-H. (2017). Cardiovascular effects of long-term exposure to air pollution: A population-based study with 900 845 person-years of follow-up. Journal of the American Heart Association, 6(11). https://doi.org/10.1161/JAHA.117.007170
- Lee, D.-W., Han, C.-W., Hong, Y.-C., Oh, J.-M., Bae, H.-J., Kim, S., & Lim, Y.-H. (2021). Short-term exposure to air pollution and hospital admission for heart failure among older adults in metropolitan cities: A time-series study. International Archives of Occupational and Environmental Health, 94(7), 1605–1615. https://doi.org/10.1007/s00420-021-01724-9
- Lee, K. K., Spath, N., Miller, M. R., Mills, N. L., & Shah, A. S. V. (2020). Short-term exposure to carbon monoxide and myocardial infarction: A systematic review and meta-analysis. Environment International, 143, 105901. https://doi.org/10.1016/j.envint.2020.105901
- Li, X., Li, Y., Yu, B., Zhu, H., Zhou, Z., Yang, Y., Liu, S., Tian, Y., Xiao, J., Xing, X., & Yin, L. (2022). Health and economic impacts of ambient air pollution on hospital admissions for overall and specific cardiovascular diseases in Panzhihua, Southwestern China. Journal of Global Health, 12, 11012. https://doi.org/10.7189/jogh.12.11012
- Liu, C., Cai, J., Chen, R., Sera, F., Guo, Y., Tong, S., Li, S., Lavigne, E., Matus Correa, P., Valdes Ortega, N., Orru, H., Maasikmets, M., Jaakkola, J. J. K., Ryti, N., Breitner, S., Schneider, A., Katsouyanni, K., Samoli, E. … Kan, H. (2022). Coarse Particulate Air Pollution and Daily Mortality a Global Study in 205 Cities. American Journal of Respiratory and Critical Care Medicine, 206(8), 999–1007.
- Liu, Y., Xie, S., Yu, Q., Huo, X., Ming, X., Wang, J., Zhou, Y., Peng, Z., Zhang, H., Cui, X., Xiang, H., Huang, X., Zhou, T., Chen, W., & Shi, T. (2017). Short-term effects of ambient air pollution on pediatric outpatient visits for respiratory diseases in yichang city, china. Environmental Pollution, 227, 116–124. https://doi.org/10.1016/j.envpol.2017.04.029
- Li, T., Zhang, Y., Jiang, N., Du, H., Chen, C., Wang, J., Li, Q., Feng, D., & Shi, X. (2023). Ambient fine particulate matter and cardiopulmonary health risks in China. Chinese Medical Journal, 136(3), 287–294.
- Miller, K. A., Siscovick, D. S., Sheppard, L., Shepherd, K., Sullivan, J. H., Anderson, G. L., & Kaufman, J. D. (2007). Long-term exposure to air pollution and incidence of cardiovascular events in women. New England Journal of Medicine, 356(5), 447–458. https://doi.org/10.1056/NEJMoa054409
- Motesaddi Zarandi, S., Hadei, M., Hashemi, S. S., Shahhosseini, E., Hopke, P. K., Namvar, Z., & Shahsavani, A. (2022). Effects of ambient air pollutants on hospital admissions and deaths for cardiovascular diseases: A time series analysis in Tehran. Environmental Science and Pollution Research International, 29(12), 17997–18009. https://doi.org/10.1007/s11356-021-17051-y
- Ning, G., Wang, S., Ma, M., Ni, C., Shang, Z., Wang, J., & Li, J. (2018). Characteristics of air pollution in different zones of sichuan basin, china. Science of the Total Environment, 612, 975–984. https://doi.org/10.1016/j.scitotenv.2017.08.205
- Ning, G., Yim, S. H. L., Wang, S., Duan, B., Nie, C., Yang, X., Wang, J., & Shang, K. (2019). Synergistic effects of synoptic weather patterns and topography on air quality: A case of the sichuan basin of china. Climate Dynamics, 53(11), 6729–6744. https://doi.org/10.1007/s00382-019-04954-3
- Pranata, R., Vania, R., Tondas, A. E., Setianto, B., & Santoso, A. (2020). A time-to-event analysis on air pollutants with the risk of cardiovascular disease and mortality: A systematic review and meta-analysis of 84 cohort studies. Journal of Evidence-Based Medicine, 13(2), 102–115. https://doi.org/10.1111/jebm.12380
- Qiu, H., Zhu, X., Wang, L., Pan, J., Pu, X., Zeng, X., Zhang, L., Peng, Z., & Zhou, L. (2019). Attributable risk of hospital admissions for overall and specific mental disorders due to particulate matter pollution: A time-series study in chengdu, china. Environmental Research, 170, 230–237. https://doi.org/10.1016/j.envres.2018.12.019
- Raza, A., Dahlquist, M., Lind, T., & Ljungman, P. L. S. (2018). Susceptibility to short-term ozone exposure and cardiovascular and respiratory mortality by previous hospitalizations. Environmental Health: A Global Access Science Source, 17(1), 37. https://doi.org/10.1186/s12940-018-0384-z
- Roth, G. A., Mensah, G. A., Johnson, C. O., Addolorato, G., Ammirati, E., Baddour, L. M., Barengo, N. C., Beaton, A. Z., Benjamin, E. J., Benziger, C. P., Bonny, A., Brauer, M., Brodmann, M., Cahill, T. J., Carapetis, J., Catapano, A. L., Chugh, S. S., Cooper, L. T. … Murray, C. J. L. (2020). Global Burden of Cardiovascular Diseases and Risk Factors, 1990–2019. Journal of the American College of Cardiology, 76(25), 2982–3021. https://doi.org/10.1016/j.jacc.2020.11.010
- Shah, A. S. V., Langrish, J. P., Nair, H., McAllister, D. A., Hunter, A. L., Donaldson, K., Newby, D. E., & Mills, N. L. (2013). Global association of air pollution and heart failure: A systematic review and meta-analysis. The Lancet, 382(9897), 1039–1048. https://doi.org/10.1016/S0140-6736(13)60898-3
- Shahrbaf, M. A., Akbarzadeh, M. A., Tabary, M., & Khaheshi, I. (2021). Air pollution and cardiac arrhythmias: A comprehensive review. Current Problems in Cardiology, 46(3), 100649. https://doi.org/10.1016/j.cpcardiol.2020.100649
- Sui, X., Zhang, J., Zhang, Q., Sun, S., Lei, R., Zhang, C., Cheng, H., Ding, L., Ding, R., Xiao, C., Li, X., & Cao, J. (2021). The short-term effect of pm2.5/o3 on daily mortality from 2013 to 2018 in Hefei, China. Environmental Geochemistry and Health, 43(1), 153–169. https://doi.org/10.1007/s10653-020-00689-x
- Tajudin, M., Khan, M. F., Mahiyuddin, W. R. W., Hod, R., Latif, M. T., Hamid, A. H., Rahman, S. A., & Sahani, M. (2019). Risk of concentrations of major air pollutants on the prevalence of cardiovascular and respiratory diseases in urbanized area of Kuala Lumpur, Malaysia. Ecotoxicol Environ Safety, 171, 290–300. https://doi.org/10.1016/j.ecoenv.2018.12.057
- Tian, Y., Liu, H., Liang, T., Xiang, X., Li, M., Juan, J., Song, J., Cao, Y., Wang, X., Chen, L., Wei, C., Gao, P., & Hu, Y. (2018). Ambient air pollution and daily hospital admissions: A nationwide study in 218 chinese cities. Environmental Pollution, 242, 1042–1049. https://doi.org/10.1016/j.envpol.2018.07.116
- Wang, M., Hou, Z. H., Xu, H., Liu, Y., Budoff, M. J., Szpiro, A. A., Kaufman, J. D., Vedal, S., & Lu, B. (2019). Association of estimated long-term exposure to air pollution and traffic proximity with a marker for coronary atherosclerosis in a nationwide study in china. JAMA Network Open, 2(6), e196553. https://doi.org/10.1001/jamanetworkopen.2019.6553
- Wang, Y., Li, J., Wang, L., Lin, Y., Zhou, M., Yin, P., & Yao, S. (2021). The impact of carbon monoxide on years of life lost and modified effect by individual- and city-level characteristics: Evidence from a nationwide time-series study in china. Ecotoxicol Environ Safety, 210, 111884. https://doi.org/10.1016/j.ecoenv.2020.111884
- Wang, X., Yu, C., Zhang, Y., Shi, F., Meng, R., & Yu, Y. (2020). Attributable risk and economic cost of cardiovascular hospital admissions due to ambient particulate matter in wuhan, china. International Journal of Environmental Research Public Health, 17(15), 5453. https://doi.org/10.3390/ijerph17155453
- Wei, Y., Wang, Y., Di, Q., Choirat, C., Wang, Y., Koutrakis, P., Zanobetti, A., Dominici, F., & Schwartz, J. D. (2019). Short term exposure to fine particulate matter and hospital admission risks and costs in the medicare population: Time stratified, case crossover study. BMJ: British Medical Journal, 367, l6258. https://doi.org/10.1136/bmj.l6258
- WHO. (2021, June 11). “Cardiovascular diseases (CVDs).” from https://www.who.int/news-room/fact-sheets/detail/cardiovascular-diseases-(CVDs)
- Wolf, K., Hoffmann, B., Andersen, Z. J., Atkinson, R. W., Bauwelinck, M., Bellander, T., Brandt, J., Brunekreef, B., Cesaroni, G., Chen, J., de Faire, U., de Hoogh, K., Fecht, D., Forastiere, F., Gulliver, J., Hertel, O., Hvidtfeldt, U. A., Janssen, N. A. H. … Peters, A. (2021). Long-term exposure to low-level ambient air pollution and incidence of stroke and coronary heart disease: A pooled analysis of six european cohorts within the elapse project. The Lancet Planetary Health, 5(9), e620–e632. https://doi.org/10.1016/S2542-5196(21)00195-9
- Xie, Y., Li, Z., Zhong, H., Feng, X. L., Lu, P., Xu, Z., Guo, T., Si, Y., Wang, J., Chen, L., Wei, C., Deng, F., Baccarelli, A. A., Zheng, Z., Guo, X., & Wu, S. (2021). Short-term ambient particulate air pollution and hospitalization expenditures of cause-specific cardiorespiratory diseases in China: A multicity analysis. The Lancet Regional Health Western Pacific, 15, 100232. https://doi.org/10.1016/j.lanwpc.2021.100232
- Yang, B. Y., Guo, Y., Markevych, I., Qian, Z. (., Bloom, M. S., Heinrich, J., Dharmage, S. C., Rolling, C. A., Jordan, S. S., Komppula, M., Leskinen, A., Bowatte, G., Li, S., Chen, G., Liu, K.-K., Zeng, X.-W., Hu, L.-W., & Dong, G.-H. (2019). Association of long-term exposure to ambient air pollutants with risk factors for cardiovascular disease in China. JAMA Network Open, 2(3), 190–318. https://doi.org/10.1001/jamanetworkopen.2019.0318
- Yue, C., Yang, F., Li, F., & Chen, Y. (2021). Association between air pollutants and atrial fibrillation in general population: A systematic review and meta-analysis. Ecotoxicol Environ Safety, 208, 111508. https://doi.org/10.1016/j.ecoenv.2020.111508
- Zhang, Z., Kang, J., Hong, Y. S., Chang, Y., Ryu, S., Park, J., Cho, J., Guallar, E., Shin, H. C., & Zhao, D. (2020). Long-term particulate matter exposure and incidence of arrhythmias: A cohort study. Journal of the American Heart Association, 9(22), e016885. https://doi.org/10.1161/JAHA.120.016885
- Zhang, P., & Zhou, X. (2020). Health and economic impacts of particulate matter pollution on hospital admissions for mental disorders in chengdu, southwestern china. Science of the Total Environment, 733, 139114. https://doi.org/10.1016/j.scitotenv.2020.139114
- Zhao, X., Yu, X., Wang, Y., & Fan, C. (2016). Economic evaluation of health losses from air pollution in beijing, china. Environmental Science and Pollution Research International, 23(12), 11716–11728. https://doi.org/10.1007/s11356-016-6270-8
- Zheng, X. Y., Orellano, P., Lin, H. L., Jiang, M., & Guan, W. J. (2021). Short-term exposure to ozone, nitrogen dioxide, and sulphur dioxide and emergency department visits and hospital admissions due to asthma: A systematic review and meta-analysis. Environment International, 150, 106435. https://doi.org/10.1016/j.envint.2021.106435
- Zhou, H., Geng, H., Dong, C., & Bai, T. (2021). The short-term harvesting effects of ambient particulate matter on mortality in taiyuan elderly residents: A time-series analysis with a generalized additive distributed lag model. Ecotoxicology and Environmental Safety, 207, 111235. https://doi.org/10.1016/j.ecoenv.2020.111235
- Zhu, W., Cai, J., Hu, Y., Zhang, H., Han, X., Zheng, H., & Wu, J. (2021). Long-term exposure to fine particulate matter relates with incident myocardial infarction (mi) risks and post-mi mortality: A meta-analysis. Chemosphere, 267, 128903. https://doi.org/10.1016/j.chemosphere.2020.128903