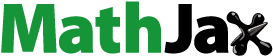
ABSTRACT
The aim of this study was to assess the historical and projected climate trend analysis in the Upper East Region, Ghana, using time series and modelled climate data under shared socio-economic pathways scenarios. Due to the climate data scarcity challenge in Ghana, there is a need to rely on high-resolution satellite-based climate products for climate change studies. Accordingly, temperature and precipitation data of six stations were obtained from Ghana meteorological stations; in addition, precipitation data of five satellite stations were obtained from CHIRPS data with 0.05’ (5 km) resolution to complement the in-situ datasets; and the temperature data for the satellite station obtained from the NASA power project were extracted. Modelled climate data were obtained from five GCMs in the CMIP data portal on the WCRP website. R software was used to extract NetCDF-format GCM data to Excel format and CMhyd was used for downscaling GCM data. The variance scaling method and local intensity scaling methods were used for temperature and precipitation bias correction, respectively. The Mann-Kendall (MK) method was used to determine the statistical significance of a change and identify trends in temperature and precipitation. The results of historical temperatures were significantly increased, while historical precipitation was significantly decreased. The projected mean annual temperature will increase significantly, peaking at 1.80°C by 2065, precipitation will, conversely, show significant spatial variability and seasonal dependency. For instance, by 2065, precipitation is projected to decrease between 10.05% and 12.66%. The results concluded by recommending policymakers develop climate change adaptation strategies guided by future climate scenarios.
1. Introduction
Climate change is defined as a statistically significant change in the average climatic conditions or variability that persists for several decades or longer (Milentijević et al., Citation2022). Temperature changes are notable manifestations of climate change (Kwawuvi et al., Citation2023). The average temperature of our planet might rise by 1.1 to 5.4oC (2–9.7 F) by the end of the 21st century, according to simulations from numerous climate models (IPCC, Citation2022). The demand for long-term climate perspectives has grown due to worries about global climate change (Norrgård, Citation2015). Because of this, historical records have significantly augmented and enhanced our knowledge of historical climatic variability (Norrgård, Citation2014). Advances in historical climatology have drawn attention to the widespread use of documentary materials like weather diaries, private correspondence, trip journals, ship logs, and official and missionary records (Andrijevic et al., Citation2020). Increases in global temperature are predicted to significantly affect several countries, with disastrous consequences for agriculture systems in developing countries that rely solely on rainfall (Klutse et al., Citation2020a). Temperature variability may lead to a rise in the frequency, magnitude, and seasonality of extreme events that are likely to happen in the future (Frimpong et al., Citation2022).
Scientists have agreed that by 2050 the global temperature, on average, will be 2°C higher than the figure before the Industrial Revolution and that the 2°C higher temperatures would result in more extreme weather conditions (Deser et al., Citation2020). Climate change is a global issue, but the impact is not globally even (IPCC, Citation2022), due to the adaptive capacity. Adaptive capacity is low in underdeveloped nations, particularly in Sub-Saharan Africa (SSA). Since, their key economic sectors, such as agriculture, power generation, and industry, strongly depend on the climate, of which seasonal precipitation forms a key component (Atiah et al., Citation2023). At the same time, there is limited information flow, which is one of the necessary ingredients for climate adaptation decision-making (Pörtner et al., Citation2022). In the case of West Africa, the projections are that the sub-region will be warmer than the global average temperature of 1.5°C by 2100 (Klutse et al., Citation2020a).
Ghana faces significant challenges of the negative impacts of LULC and climate change which directly or indirectly affects ecology, economy, and society (Larbi et al., Citation2021). The Ghanaian government predicts an annual increase in the average temperature of up to 0.80 C by 2020 and 5.40 C by 2080 in all agroecological zones (Kosoe & Ahmed, Citation2022). The forecasted temperatures also show a large regional variance, with upper East Ghana expected to rise faster than the nation’s coastal regions (Dutta & Bhattacharjya, Citation2022). As a result, Upper East Ghana is expected to experience the negative consequences of climate change than the rest of the country (MESTI, Citation2015).
To improve climate change knowledge and detect the effect of changes in the past, present, and future, global climate models (GCMs) under the supervision of the Coupled Model Intercomparison Project (CMIP) were established by modelling groups globally (Atiah et al., Citation2023). Climate model data are accessible through the World Climate Research Program (WCRP), which supervises the various phases of the CMIP (Calvin et al., Citation2017). The Coupled Model Inter comparison Project (CMIP) was created as a cooperative climatology framework to better understand climate change (Meehl et al., Citation2000). The CMIP uses several models to better understand past, current, and future climate changes brought on by changes in radiative forcing or natural, unforced variability (Döscher et al., Citation2022). In comparing other new models and earlier models, the climate research community evaluates how well the models perform (Kharin et al., Citation2013). The model ensemble results also support several studies and programs on the impacts of and adaptations to climate change and public outreach and education (Harcourt et al., Citation2019). To address the CMIP5’s remaining scientific gaps and new problems in climate modelling, the CMIP6 was launched in 2014 (Eyring et al., Citation2015). CMIP6 models have improved dynamic processes and higher resolution. They are, therefore, more accurate than earlier iterations (Laube et al., Citation2012). By integrating RCPs into SSPs, CMIP6 improves the robustness of climate projections and better supports climate policies by considering how socioeconomic variables (such as population and economy) affect greenhouse gas emissions (Mohammed et al., Citation2021). The current scenario iteration adopted for CMIP6 (2016–2021) and integrated into the Assessment Report of IPCC Six (AR6) of 2021 is premised on a program of shared socioeconomic pathways (SSPs) (Maharana et al., Citation2021). The SSP-based scenarios are regarded as the most advanced, taking into consideration mitigation to current emissions growth (Masson-Delmotte et al., Citation2021). Five SSPs were created, each depending on a unique set of human development propositions, including those related to population, education, urbanisation, GDP, economic growth, rate of technological advancement, emissions of greenhouse gases (GHG), aerosols, energy supply and demand, and changes in land use (Bauer et al., Citation2017; Chaturvedi et al., Citation2020; O’Neill, Citation2016). A modernised version of the RCPs was intended to be used with the SSPs (Riahi et al., Citation2017).
The variability of temperature and precipitation indices over West Africa is being assessed using 21 CMIP6 models (Jacob et al., Citation2020). The potential impacts of future rainfall and temperature changes on streamflow and evapotranspiration in the Tano River basin of Ghana from 2021 to 2050 was studied by (Larbi et al., Citation2022) by using GCMs (CanESM5, MPI-ESM1-2-LR, MIROC6, and IPSL-CM6A) under RCP 4.5 emission scenarios (Gbangou et al., Citation2019). Conclusively, these studies opine a lack of forecast data on temperature changes and precipitation, making that ecological zone more susceptible to the ravages of extreme weather conditions. Aiming to fill the research gap, this article uses the Coupled Model Intercomparison Project Phase Six (CMIP6) model data to project temperature and precipitation trends from 2025 to 2065 for the Upper East Region of Ghana. Significantly, this article contributes to the literature by highlighting the practical implications of future climate change and the need to develop strategies and policies for long-term environmental management. The article is expected to guide local governments and policymakers in developing and implementing effective and appropriate response strategies to mitigate the negative effects of climate change.
2. Methodology
2.1. Study region
The Upper East Region () is located on the northeastern corner of Ghana between latitudes 10°30′ to 11°00′ north and longitudes 0° to 1°30′ west. The region covers a land surface area of 8842 km2 with a population density of 103 persons/km2. It has two international boundaries with the Republics of Burkina Faso to the north and Togo to the east. The other boundaries are Northern Region and Upper West Region to the south and west, respectively (Larbi et al., Citation2021). The regions’ soil is shallow and low in soil fertility, weak with low organic matter content, and predominantly coarse textured. The region has a tropical continental or interior savanna climate, mostly influenced by the tropical continental air mass. While the movement of the air masses results in two rainy seasons in the southern part of the country, the Upper East Region experiences only one, lasting from May to October (Frimpong et al., Citation2014).
Annual average precipitation is about 1000 mm. About 85% of the region falls within the White Volta Basin, with a network of tributaries, mainly, the White, Red, and Black Voltas and the Sissile River. Also, the Kulpawn River which has its catchment to the southwest of the region is joined by the Sissile just before its confluence with the White Volta. About 80% of the economically active population engages in agriculture (Mostafa et al., Citation2022). The main produce is millet, guinea corn, maize, groundnut, beans, sorghum, and dry season tomatoes and onions. Livestock and poultry production are also important. There are two main irrigation projects, the Vea Project in Bolgatanga covering 850 ha and the Tono Project in Navrongo covering 2490 ha. Altogether they provide employment to about 6000 small-scale farmers. Also, dotted in many parts of the region are dams and dugouts which provide water for both domestic and agricultural purposes (Gbangou et al., Citation2019).
2.2. Data source
2.2.1. Observed climate data source
Some of the Observed daily rainfall, maximum and minimum temperature covering the period from 1982 to 2022 provided by Ghana Meteorological Agency (). Due to the sparse distribution of in situ climate stations throughout the region, additional daily values of rainfall for gridded sites were extracted from the Climate Hazards Group Infrared Precipitation with Station (CHIRPS) data with 0.05’ (5 km) resolution to complement the in-situ datasets (). The temperature data from the NASA POWER project are extracted from Modern Era Retrospective-Analysis for Research and Applications (MERRA-2) assimilation model products and GEOS 5.12.4 near-real-time products (Agbo et al., Citation2022) (). These two satellite products have been selected based on their ability to accurately reproduce the climatology in the upper east region of Ghana (Larbi et al., Citation2022). Data quality control in terms of missing data checks for the six climate stations were performed. Less than 10% missing data records for rainfall and temperature were found for each of the six climate stations.
Table 1. Meteorological station locations in the study region.
Table 2. Climate hazards group infrared precipitation with Station (CHIRPS) and nasa power for temperature locations for the study region.
Table 3. Data source/website for observed climate data from 1982 to 2022.
2.2.2. Global climate models (GCMs) data source
Climate model evaluation presents a crucial pathway into the investigation of the simulation of future climate (Meehl et al., Citation2014). GCMs outputs in CMIP6 are produced by various institutions across the globe due to the high temporal resolutions are within the required domain that is needed for the determination of the features/processes in this study. Some of the models used in west Africa, particularly in Ghana by different Authors are as follows.
In this study, five GCM models from the CMIP6 were used for future climate change projections and assessments under shared socio-economic path ways for the region. shows GCMs models: CanESM5, CNRM-CM6-1, IPSL-CM6A-ATM-HR, MIROC6, and MPI-ESM1-2-LR were selected based on previous studies conducted in the Volta basin and Tono basin of upper east region (Larbi et al., Citation2021; O’Neill et al., Citation2017) (). Future time precipitation, and temperature data for the SSP 2.6, SSP 4.5, SSP 7.0 and SSP 8.5 emission scenarios were downloaded using the CMIP6 model from (https://pcmdi.llnl.gov/mips/cmip6/dataportal.html//) node portal of the IPCC database distribution centre). The list of the GCM model and resolution s are listed in .
Table 4. Gcms used in the previous studies conducted in West Africa, Ghana.
Table 5. Models to download CMIP6 climate data for the study region.
2.3. Downscaling GCM data
The most popular method for predicting how the climate will change in the future is to utilise Global climate models (Taylor et al., Citation2012). The CMIP uses several models to better understand past, current, and future climate changes brought on by changes in radiative forcing or natural, unforced variability (Döscher et al., Citation2022). In comparing other new models and earlier models, the climate research community evaluates how well the models perform. The model ensemble results also support several studies and programs on the impacts of and adaptations to climate change and public outreach and education (Harcourt et al., Citation2019). To address the CMIP5’s remaining scientific gaps and new problems in climate modelling, the CMIP6 was launched in 2014 (Eyring et al., Citation2016). CMIP6 models have improved dynamic processes and higher resolution. They are, therefore, more accurate than earlier iterations (Nuhu & Matsui, Citation2022). By integrating RCPs into SSPs, CMIP6 improves the robustness of climate projections and better support climate policies by considering how socioeconomic variables (such as population and economy) affect greenhouse gas emissions (Vaittinada Ayar et al., Citation2021).
Climate projections for the future are typically generated through the use of global climate models (GCMs) (Hewitt et al., Citation2021). GCMs are scientific tools that replicate the complete climate system of the Earth, encompassing the oceans, atmosphere, and land (Van Vuuren et al., Citation2017). They are designed to depict large-scale climate patterns across continents and have been proven to accurately represent general patterns as observed in meteorological datasets from the 20th century (Manski et al., Citation2021). These GCMs offer well-configured environments for examining the impact of various future greenhouse gas emissions on the climate of the Earth. The downscaled model output is more realistic than the GCM output for direct application as regional-scale study of climate change (Orkodjo et al., Citation2022). R software Version 4.6.2. was used for extracting GCM data from NetCDF format to Excel format and CMhyd was used for downscaling GCM to Specific to the study area. Downscaling from GCMs to RCMs is necessary before using GCM model data as input for the future projections (Dessu & Melesse, Citation2013).
2.4. Bias correction of climate data projections
Prior to using climatic data for future projection, biases typically need to be corrected (Hosseinzadehtalaei et al., Citation2021). Because, GCM model output data usually has a significant bias, which necessitates correlation to analyse data bias reduction, improve data quality, and increase data reliability (Hewitt et al., Citation2021). Hydrological modelling software (CMhyd) climate model data were utilised in this study to correct bias and remove bias from future climate daily temperature and precipitation data (Willkofer et al., Citation2018). For this study, we downloaded CMhyd software from https://swat.tamu.edu/software/. The variance scaling bias-correction method was used to correct both the mean and variance of the temperature time series. This methods perfectly match the monthly mean of the corrected values with that of observed data (Eyring et al., Citation2015). The local intensity scaling (LOCI) method of precipitation bias correction. This method can adjust mean as well as both wet-day frequencies and wet-day intensities (Somorin, Citation2010). The bias-corrected rainfall and temperature-simulated data (GCM) were used to assess the projected changes in rainfall and temperature at the region under the SSP2.6, SSP 4.5, SSP 7.0 and SSP8.5 scenarios. The change analysis was conducted for the period 2025–2065 relative to the 1982–2022 reference period.
2.5. Mann-kendall (M.K.) trend test
Mann-Kendall’s tests was used for the trend test. The nonparametric Mann-Kendall test checks for trends in time series without determining whether they are linear or non-linear, making it less vulnerable to outliers (Alves et al., Citation2022). The Z test statistics S figure is presumed to be zero, meaning no trend exists (Agbo et al., Citation2022). The S is increased by one if a data value from a later period is discovered to be higher than a data value from an earlier period (Wong et al., Citation2017). Contrary, if the data from the later period is less than the first period, the Z test statistics S is lessened by one. In this case, the result of all pluses and minuses gives the final S value between −1 and + 1. The null hypothesis of the Z test shows no trend. While the alternative hypothesis of the Z test means there is a trend. The following will be the Mann-Kendall test statistics:
any integer between
and
,
and
are sequential time series values, n is the amount of data in the set,
(
is the sign function and is given as:
It is also assumed that for n = 8, the S test statistics is normally distributed, with a mean value
zero and variance calculated using Equationequation (3)(3)
(3)
Under this situation, the standardised test statistics Z will be:
The choice to accept or reject the null hypothesis is determined by comparing the calculated Z-value with the critical value at a selected significance level.
3. Result and discussion
3.1. Evaluation of historical precipitation and trend analysis
Trends between 1982 and 2022 were statistically significant, according to a 40-year analysis of annual and seasonal variations in precipitation. An evaluation of the 40-year record of annual total rainfall data from the representative stations revealed a coefficient of variation from 20% to 89%. A single rainy season in the Upper East Region occurs from May to October. For the historical period of change in precipitation trend for the region, six (6) precipitation measurement stations and five (5) Gridded stations from CHRIPS data were examined. For the five (5) grid stations and six (6) meteorological measuring stations the annual precipitation data trends of the time series showed seasonal variation trends. All stations in () displayed monotonic trend changes in annual precipitation that were statistically significant, according to the trend detection test.
Sen’s slope estimator was used to analyse the time-series data from 1982 to 2022. Across all scenarios, rainfall exhibits a noticeable and consistent declining trend. The outcomes of the Mann-Kendall test for trend assessment are displayed in , indicating a declining trend in precipitation within the area. The results of the M.K. test for annual precipitation data demonstrate a statistically significant decrease in trend, with a significance level of 1%. Significant statistical trends have been observed for rainfall, with the results remaining statistically significant at a 99% confidence level from 1982 to 2022. It is imperative to observe that the 99% confidence limit was determined based on the ‘z’ score value. These findings align with the results reported by (Klutse et al., Citation2020a) indicating a reducing trend in rainfall levels and highlighting the significance of seasonal distribution within the Region at a 1% significance level. shows the MK test and trends that are statistically significant.
3.2. Evaluation of historical average temperature and trend analysis
The findings of the trend test showed statistically significant positive increasing trend. The results indicated a consistent rise in temperature across all seasons, with significant effects observed in the last four decades at both yearly and seasonal levels. The findings revealed a significantly positive increase trend at six meteorological gauging stations and five grid stations (). The average maximum and minimum temperature increase ranged from 0.6 to 1.5 during the study period. Specifically, the annual maximum and minimum temperature displayed a noteworthy upward trend, and a significant increase was observed as shown in .
The highest temperature during the detected time exhibited a subtle warming or upward trend, with Sen’s slope ranging from 0.01 to 0.02. These findings align with the literature; for example, research by (Constantinidou et al., Citation2020; Derbile et al., Citation2022; Stanturf et al., Citation2011) which also reported increasing trends. Notably, these studies indicated that increasing tendencies of the minimum temperature were more pronounced than the maximum temperature.
The analysis based on MK test () displays the results of the statistical monotonicity test for the annual temperature trend, which revealed that all stations had a statistically significant positive trend that was increasing. The maximum and minimum temperatures were examined using the MK test on monthly and annual mean time series data spanning 40 years from 1982 to 2022. The MK statistics and corresponding P-values at 5% and 1% significance levels. The analysis revealed significant change in annual temperature throughout the period from 1982 to 2022. As anticipated, both maximum temperature variables exhibited a consistent linear increase since the 1980s. Overall, the trend test results showed that the region has a statistically significant trend of increasing temperature ().
The study shows a general increase in annual temperature. Overall, the upper east region is hotter than other regions in Ghana. The average annual temperature is 32.56ºC (90.61ºF) and it is 3.7% higher than Ghana’s averages. These findings align with previous research conducted by (Frimpong et al., Citation2014) and (Klutse et al., Citation2020b) who also observed higher increasing trends in the minimum temperature and the maximum temperature series in the northern region of Ghana.
3.3. Projection of annual precipitation and trend analysis
Projected future precipitation in the Region shows a significant decreasing trend, which results in a change in cropping times and frequent extreme events like drought and flooding. The results () show the predicted precipitation trend for the Upper East Region was evaluated for 40 years from 2025 to 2065 compared to the reference period. The models outcomes revealed that the general trend of precipitation will decrease in the future compared to the reference period.
The study analysed the anticipated changes in average monthly rainfall over 40 years using Sen’s slope estimator. Sen’s slope estimator was applied to time-series data from 2025 to 2065. The results indicate a significant decreasing trend in precipitation across all scenarios. Furthermore, a significant relationship was observed between the four scenarios based on the linear regression analysis, with R2 values of 0.81, 0.86, 0.71, and 0.81 for SSP1–2.6; SSP2–4.5; SSP3–7.0 and SSP5–8.5 w/m2 (). To project future rainfall, the average outcomes of the models were adopted to compute annual and annual average precipitation. The average outcomes of the GCMs under the SSP1–2.6, SSP2–4.5, SSP3–7.0 and SSP5–8.5 emission scenarios indicate a declining trend in precipitation over the next 40 years.
Table 6. Projected slope of average annual precipitation, 2025–2065.
The MK test was employed to analyse the monthly and annual means of precipitation, using a 40-year time series from 2025 to 2065. presents MK test results and corresponding P-values at 5% and 1% significance levels. The annual precipitation shows significant change between 2025 and 2065. The nonparametric MK method assessed whether the monotonic trend was upward or downward. The results presented in indicate the presence of a trend in the precipitation pattern within the studied region. Specifically, SSP 2.6 and SSP 7.0 show statistical significance at 99% confidence. This suggests that precipitation is projected to decline during the future 40-year period compared to the reference period.
Table 7. MK trend analysis of future precipitation (2025–2065).
illustrates the trend of average annual precipitation during these 40 years. The Future precipitation shows a negative trend under SSP 2.6. The trend result demonstrated a statistically significant trend of decreasing precipitation.
The characteristics of precipitation variability in the region were temporally and spatially examined using rainfall data from the Ghana Meteorological Agency from 1982 to 2022. It was observed that the region experienced its highest levels of precipitation in the central area, with an average annual precipitation ranging from 1000 to 1050 mm. The distribution of monthly precipitation across the region displayed a north-to-south spatial gradient, with a declining trend in quantity. The decrease in rainfall could accelerate the incidence of drought in the Upper East Region. Rivers, dams, and dugouts would dry up and dry season farming would be hampered. Also, crop yield could reduce in the region because farming is rain dependent. However, low crop yield could be averted if storm water is harvested in controlled dams for irrigation because rain amount does not necessarily mean uniform distribution of water. Incidence of climate-related diseases such as cerebrospinal meningitis would increase, and the cost of drugs and food needed for mitigation would increase. This is because global temperature increases combined with increasing food demand as population grows and would pose large risks and increase cost to food security globally, regionally, and locally. The highest rainfall was observed in the central and northwestern areas of the region. This finding Supports the finding of (Acheampong et al., Citation2014; Zhu et al., Citation2021) prediction of a decrease in precipitation over northern Ghana between 12% and 14% by the end of the 2100th century.
3.4. Projected minimum and maximum temperatures and trend analysis
shows the projected maximum temperature will increase by approximately 2°C above current levels by 2065 under the SSP5–8.5 scenario. However, under the scenario of SSP1–2.6, the temperature rise will be less than 1°C. Overall, temperatures in the region were warmer than initially predicted by the SSP-2.6 scenario but lower than those projected by the SSP5–8.5 scenario until 2065. Nevertheless, by 2065, the maximum temperature in the SSP5–8.5 scenario is anticipated to higher than that of the SSP1–2.6 scenario. Similarly (Kosoe & Ahmed, Citation2022), observed a rise in temperatures of 0.075°C per year in the Bawku East District of upper east region between 1961 and 2012. This rate is higher than Ghana’s average of 0.021°C annually, which aligns with the findings of this study.
Additionally, the rate at which the minimum temperature is warming is projected to outpace that of the maximum temperature, and this trend is expected to persist until 2065. Consequently, daytime and night-time temperatures will likely rise, but the increase in night-time temperatures will be more rapid. This indicates a rise in residual heat during the daytime.
Under the SSP1–2.6; SSP2–4.5; SSP3–7.0, and SSP5–8.5 scenarios, the maximum temperature is projected to experience an upward trend with annual increases of 0.818, 0.97, 0.98, and 0.84 degrees Celsius respectively (). The findings from the study are in similar with a previous study by (Gbangou et al., Citation2019) that reported an increasing trend in mean temperature over a specific area within the Upper East Region from 1931 to 1990.
Table 8. Projected slope of maximum temperature from 2025 to 2065.
presents the MK test results and corresponding P-values at 5% and 1% significance levels. It was observed that the annual temperature depicts significant change between 2025 and 2065. As anticipated, both the maximum temperature variables displayed a consistent linear increase since 1982. The nonparametric MK test result shows the downward trend was monotonic in the variable over time of SSP 2.6. ().
Table 9. Analysis of M.K. trend for future minimum temperature from 2025 to 2065.
In line with the trends observed in minimum temperatures, the projected minimum temperature experience greater warming by 2065, as depicted in . The mean annual temperature was expected to increase with changes expected to be more pronounced in the regions based on the ensemble mean result of five global climate models. The results indicated that temperature will increase with a reduction in the number of cool nights and an increase in the number of hot days. Other contributing factors that would increase the severity of decreasing precipitation amount and increasing deforestation and desertification as a result in change in land use and increase fuel wood consumption. These findings support the predictions made by Eby et al., (Citation2013) which indicate that global temperatures have risen by approximately 1oC over the last century. The IPCC further suggests that this warming trend is expected to speed up in the next 50 years, potentially resulting in a temperature increase of 1.5–3 oC due to rapid land use change.
Sen’s slope estimator indicates a strong upward trend in minimum temperature across all scenarios. shows the linear regression analysis confirms a significant relationship among the four scenarios, as evidenced by the high coefficient of determination (R2) values. Specifically, R2 values of 0.76, 0.97, 0.97, and 0.97 were observed under SSP1–2.6; SSP2–4.5; SSP3 7.0 and SSP5–8.5 w/m2, respectively. These high R2 values indicate a strong correlation between the scenarios and the observed increase in minimum temperature.
Table 10. Projected slope of maximum temperature over 40 years from 2025 to 2065.
Projections indicate that the warming rate of the minimum temperature will outpace that of the maximum temperature, and this trend is anticipated to persist until 2065. Under the SSP5–8.5 scenario, the upper east Region is projected to experience the most rapid warming rate of the minimum temperature, with an estimated increase of 0.041 °C per year ().
Table 11. M.K. trend analysis of future minimum temperature over 40 years (2025–2065).
As shown in , the minimum temperature from 2025 to 2065 shows decreasing trend under SSP 4.5 and SSP7.0. On the other hand, SSP 2.6 and SSP 8.5 shows a statistically significant increasing trend of minimum temperature.
4. Conclusions
This study utilised five CMIP6 models to examine the projected rainfall and temperature changes from 2025 to 2065. The projection and trend evaluation were conducted under four commonly used socioeconomic scenarios (SSP1–2.6, SSP2–4.5, SSP3–7.0, and SSP5–8.5). Initially, the performance of the CMIP6 models was evaluated based on data from 1982 to 2022 using bias correction method. The Mann-Kendall trend test was used to determine whether a change is statistically significant and to detect trends of the baseline line period and future periods of projected temperature and precipitation. Over the study period, the estimated and expected annual, and monthly temperature changes increased significantly and the expected annual, and monthly precipitation decreased significantly.
The annual temperature projections show a statistically significant upward trend. The results showed that the CMIP6 ensemble accurately reproduced the observed temperature distribution within a range of 0.01°C to 0.031°C (or 0.021°C to 0.075°C) when compared to the historical dataset across the entire study area. Similarly, the projected precipitation distribution was effectively replicated within a range of 10.05% to 12.66% relative to the historical dataset over a significant portion of the study area. However, the models predicted higher seasonal precipitation in the region. Under the four future scenarios, the study investigated the projected temperature and precipitation changes from 2025 to 2065, compared to the historical period of 1982 to 2022. Notably, the Upper-East Region of Ghana experienced a more pronounced temperature increase. Specifically, temperatures in the of the study area are projected to rise by up to 1.80 C by the mid-twentieth century under the SSP5–8.5 scenario. Regarding rainfall, a decrease is expected. Minimum and maximum temperatures are anticipated to rise significantly across the Region in the twenty-first century under each of the four scenarios. From the four different scenarios, the risk of maximum temperature is projected to be 0.020C per decade. Rainfall and temperature projections for the upper east Region remain uncertain. We conclude by recommending policymakers develop climate change adaptation strategies guided by these future climate scenarios. If climate-smart management policies and practices are not vigorously pursued, the region may lose its ecology and economic significance.
Author contributions
All authors conceived of the presented idea, developed the theory, and performed the computations.
Code availability
In this research, CMhyd software, R software, and Excel software were used. However, the code is not used.
Ethics approval and consent to participate
Not applicable because this article does not contain any studies with human or animal subjects. The data of this research were not prepared through a questionnaire.
Consent for publication
There is no conflict of interest regarding the publication of this article. The authors of the article make sure that everyone agrees to submit the article and is aware of the submission.
Disclosure statement
The authors declare that they have no competing interests.
Data availability statement
The data that has been used is confidential. Most of the data used in this research article are obtained from Gmet (National Meteorology Agency of Ghana), CHRIPS https://data.chc.ucsb.edu/products/CHIRPS-2.0/, NASA power https://power.larc.nasa.gov/data-access-viewer/, CMIP6 (https://esgf-node.llnl.gov/search/cmip6/.
Additional information
Funding
References
- Acheampong, E. N., Ozor, N., & Owusu, E. S. (2014). Vulnerability assessment of northern Ghana to climate variability. Climatic Change, 126(1–2), 31–44. https://doi.org/10.1007/s10584-014-1195-z
- Agbo, E. P., Nkajoe, U., & Edet, C. O. (2022). Comparison of Mann–Kendall and Şen’s innovative trend method for climatic parameters over Nigeria’s climatic zones. Climate Dynamics, 1–17. https://doi.org/10.21203/rs.3.rs-1731818/v1
- Alves, K. S., Shah, D. A., Dillard, H. R., Del Ponte, E. M., & Pethybridge, S. J. (2022). From reanalysis data to inference: A framework for linking environment to plant disease epidemics at the regional scale. https://osf.io/v53py/
- Andrijevic, M., Crespo Cuaresma, J., Muttarak, R., & Schleussner, C.-F. (2020). Governance in socioeconomic pathways and its role for future adaptive capacity. Nature Sustainability, 3(1), 35–41. https://doi.org/10.1038/s41893-019-0405-0
- Atiah, W. A., Johnson, R., Muthoni, F. K., Mengistu, G. T., Amekudzi, L. K., Kwabena, O., & Kizito, F. (2023). Bias correction and spatial disaggregation of satellite-based data for the detection of rainfall seasonality indices. Heliyon, 9(7), e17604. https://doi.org/10.1016/j.heliyon.2023.e17604
- Bauer, N., Calvin, K., Emmerling, J., Fricko, O., Fujimori, S., Hilaire, J., Eom, J., Krey, V., Kriegler, E., & Mouratiadou, I. (2017). Shared socioeconomic pathways of the energy sector–quantifying the narratives. Global Environmental Change, 42, 316–330. https://doi.org/10.1016/j.gloenvcha.2016.07.006
- Calvin, K., Bond-Lamberty, B., Clarke, L., Edmonds, J., Eom, J., Hartin, C., Kim, S., Kyle, P., Link, R., Moss, R., McJeon, H., Patel, P., Smith, S., Waldhoff, S., & Wise, M. (2017). The SSP4: A world of deepening inequality. Global Environmental Change, 42, 284–296. https://doi.org/10.1016/j.gloenvcha.2016.06.010
- Chaturvedi, V., Koti, P. N., Sugam, R., Neog, K., & Hejazi, M. (2020). Cooperation or rivalry? Impact of alternative development pathways on India’s long-term electricity generation and associated water demands. Energy, 192, 116708. https://doi.org/10.1016/j.energy.2019.116708
- Constantinidou, K., Hadjinicolaou, P., Zittis, G., & Lelieveld, J. (2020). Performance of land surface schemes in the WRF model for climate simulations over the MENA-CORDEX domain. Earth Systems and Environment, 4(4), 647–665. https://doi.org/10.1007/s41748-020-00187-1
- Derbile, E. K., Chirawurah, D., & Naab, F. X. (2022). Vulnerability of smallholder agriculture to environmental change in Northwestern Ghana and implications for development planning. Climate and Development, 14(1), 39–51. https://doi.org/10.1080/17565529.2021.1881423
- Deser, C., Lehner, F., Rodgers, K. B., Ault, T., Delworth, T. L., DiNezio, P. N., Fiore, A., Frankignoul, C., Fyfe, J. C., Horton, D. E., Kay, J. E., Knutti, R., Lovenduski, N. S., Marotzke, J., McKinnon, K. A., Minobe, S., Randerson, J., Screen, J. A., Simpson, I. R., & Ting, M. (2020). Insights from Earth system model initial-condition large ensembles and future prospects. Nature Climate Change, 10(4), 277–286. https://doi.org/10.1038/s41558-020-0731-2
- Dessu, S. B., & Melesse, A. M. (2013). Impact and uncertainties of climate change on the hydrology of the Mara River basin, Kenya/Tanzania. Hydrological Processes, 27(20), 2973–2986. https://doi.org/10.1002/hyp.9434
- Döscher, R., Acosta, M., Alessandri, A., Anthoni, P., Arsouze, T., Bergman, T., Bernardello, R., Boussetta, S., Caron, L.-P., Carver, G., Castrillo, M., Catalano, F., Cvijanovic, I., Davini, P., Dekker, E., Doblas-Reyes, F. J., Docquier, D., Echevarria, P. … Yepes-Arbós, X. (2022). The EC-Earth3 earth system model for the coupled model intercomparison project 6. Geoscientific Model Development, 15(7), 2973–3020. https://doi.org/10.5194/gmd-15-2973-2022
- Dutta, D., & Bhattacharjya, R. K. (2022). A statistical bias correction technique for global climate model predicted near-surface temperature in India using the generalized regression neural network. Journal of Water and Climate Change, 13(2), 854–871. https://doi.org/10.2166/wcc.2022.214
- Eby, M., Weaver, A. J., Alexander, K., Zickfeld, K., Abe-Ouchi, A., Cimatoribus, A., Crespin, E., Drijfhout, S., Edwards, N., Eliseev, A., Feulner, G., Fichefet, T., Forest, C. E., Goosse, H., Holden, P. B., Joos, F., Kawamiya, M., Kicklighter, D. … Zeng, N. (2013). Historical and idealized climate model experiments: An intercomparison of Earth system models of intermediate complexity. Climate of the Past, 9(3), 1111–1140. https://doi.org/10.5194/cp-9-1111-2013
- Eyring, V., Bony, S., Meehl, G. A., Senior, C. A., Stevens, B., Stouffer, R. J., & Taylor, K. E. (2016). Overview of the coupled model intercomparison project phase 6 (CMIP6) experimental design and organization. Geoscientific Model Development, 9(5), 1937–1958. https://doi.org/10.5194/gmd-9-1937-2016
- Eyring, V., Bony, S., Meehl, G., Senior, C., Stevens, B., Stouffer, R., & Taylor, K. (2015). Overview of the coupled model intercomparison project phase 6 (CMIP6) experimental design and organization. Geoscientific Model Development Discussions, 8(12), 10539–10583. https://doi.org/10.5194/gmdd-8-10539-2015
- Frimpong, B. F., Koranteng, A., & Molkenthin, F. (2022). Analysis of temperature variability utilising Mann–Kendall and Sen’s slope estimator tests in the Accra and Kumasi Metropolises in Ghana. Environmental Systems Research, 11(1), 1–13. https://doi.org/10.1186/s40068-022-00269-1
- Frimpong, K., Oosthuizen, J., & Van Etten, E. J. (2014). Recent trends in temperature and relative humidity in Bawku East. Canadian Center of Science and Education.
- Gbangou, T., Ludwig, F., van Slobbe, E., Hoang, L., & Kranjac-Berisavljevic, G. (2019). Seasonal variability and predictability of agro-meteorological indices: Tailoring onset of rainy season estimation to meet farmers’ needs in Ghana. Climate Services, 14, 19–30. https://doi.org/10.1016/j.cliser.2019.04.002
- Harcourt, R., Bruine de Bruin, W., Dessai, S., & Taylor, A. (2019). Investing in a good pair of wellies: How do non-experts interpret the expert terminology of climate change impacts and adaptation? Climatic Change, 155(2), 257–272. https://doi.org/10.1007/s10584-019-02455-0
- Hewitt, C. D., Guglielmo, F., Joussaume, S., Bessembinder, J., Christel, I., Doblas-Reyes, F. J., Djurdjevic, V., Garrett, N., Kjellström, E., Krzic, A., Costa, M. M., & St. Clair, A. L. (2021). Recommendations for future research priorities for climate modeling and climate services. Bulletin of the American Meteorological Society, 102(3), E578–E588. https://doi.org/10.1175/BAMS-D-20-0103.1
- Hosseinzadehtalaei, P., Ishadi, N. K., Tabari, H., & Willems, P. (2021). Climate change impact assessment on pluvial flooding using a distribution-based bias correction of regional climate model simulations. Canadian Journal of Fisheries and Aquatic Sciences, 598, 126239. https://doi.org/10.1016/j.jhydrol.2021.126239
- IPCC. (2022) . Climate change 2022: Impacts. Adaptation and Vulnerability.
- Jacob, D., Teichmann, C., Sobolowski, S., Katragkou, E., Anders, I., Belda, M., Benestad, R., Boberg, F., Buonomo, E., Cardoso, R. M., Casanueva, A., Christensen, O. B., Christensen, J. H., Coppola, E., De Cruz, L., Davin, E. L., Dobler, A., Domínguez, M. … Warrach-Sagi, K. (2020). Regional climate downscaling over Europe: Perspectives from the EURO-CORDEX community. Regional Environmental Change, 20(2), 1–20. https://doi.org/10.1007/s10113-020-01606-9
- Kharin, V. V., Zwiers, F. W., Zhang, X., & Wehner, M. (2013). Changes in temperature and precipitation extremes in the CMIP5 ensemble. Climatic Change, 119(2), 345–357. https://doi.org/10.1007/s10584-013-0705-8
- Klutse, N. A. B., Owusu, K., & Boafo, Y. A. (2020a). Projected temperature increases over northern Ghana. SN Applied Sciences, 2(8). https://doi.org/10.1007/s42452-020-3095-3
- Klutse, N. A. B., Owusu, K., & Boafo, Y. A. (2020b). Projected temperature increases over northern Ghana. SN Applied Sciences, 2(8), 1–14. https://doi.org/10.1007/s42452-020-3095-3
- Kosoe, E. A., & Ahmed, A. (2022). Climate change adaptation strategies of cocoa farmers in the Wassa East District: Implications for climate services in Ghana. Climate Services, 26, 100289. https://doi.org/10.1016/j.cliser.2022.100289
- Kwawuvi, D., Mama, D., Agodzo, S. K., Bessah, E., Issoufou, Y. G., & Wisdom, A. S. (2023). Potential catastrophic consequences for rising temperature trends in the Oti River basin, West Africa. Frontiers in Climate, 5, 1184050. https://doi.org/10.3389/fclim.2023.1184050
- Larbi, I., Hountondji, F. C. C., Dotse, S.-Q., Mama, D., Nyamekye, C., Adeyeri, O. E., Djan’na Koubodana, H., Odoom, P. R. E., & Asare, Y. M. (2021). Local climate change projections and impact on the surface hydrology in the Vea catchment, West Africa. Hydrology Research, 52(6), 1200–1215. https://doi.org/10.2166/nh.2021.096
- Larbi, I., Nyamekye, C., Dotse, S.-Q., Danso, D. K., Annor, T., Bessah, E., Limantol, A. M., Attah-Darkwa, T., Kwawuvi, D., & Yomo, M. (2022). Rainfall and temperature projections and the implications on streamflow and evapotranspiration in the near future at the Tano River basin of Ghana. Scientific African, 15, e01071. https://doi.org/10.1016/j.sciaf.2021.e01071
- Laube, W., Schraven, B., & Awo, M. (2012). Smallholder adaptation to climate change: Dynamics and limits in northern Ghana. Climatic Change, 111(3–4), 753–774. https://doi.org/10.1007/s10584-011-0199-1
- Maharana, P., Kumar, D., Das, S., & Tiwari, P. R. (2021). Present and future changes in precipitation characteristics during Indian summer monsoon in CORDEX‐CORE simulations. International Journal of Climatology, 41(3), 2137–2153. https://doi.org/10.1002/joc.6951
- Manski, C. F., Sanstad, A. H., & DeCanio, S. J. (2021). Addressing partial identification in climate modeling and policy analysis. Proceedings of the National Academy of Sciences, 118(15), e2022886118. https://doi.org/10.1073/pnas.2022886118
- Masson-Delmotte, V., Zhai, P., Pirani, A., Connors, S. L., Péan, C., Berger, S., Caud, N., Chen, Y., Goldfarb, L., & Gomis, M. (2021). Climate change 2021: The physical science basis. Contribution of Working Group I to the Sixth Assessment Report of the Intergovernmental Panel on Climate Change, 2(1), 2391. https://doi.org/10.1017/9781009157896
- Meehl, G. A., Boer, G. J., Covey, C., Latif, M., & Stouffer, R. J. (2000). The coupled model intercomparison project (CMIP). Bulletin of the American Meteorological Society, 81(2), 313–318. https://doi.org/10.1175/1520-0477(2000)081<0313:TCMIPC>2.3.CO;2
- Meehl, G. A., Teng, H., & Arblaster, J. M. (2014). Climate model simulations of the observed early-2000s hiatus of global warming. Nature Climate Change, 4(10), 898–902. https://doi.org/10.1038/nclimate2357
- MESTI. (2015). Ghana’s Third National Communication Report to the UNFCCC.
- Milentijević, N., Valjarević, A., Bačević, N., Ristić, D., Kalkan, K., Cimbaljević, M., Dragojlović, J., Savić, S., & Pantelić, M. (2022). Assessment of observed and projected climate changes in Bačka (Serbia) using trend analysis and climate modeling. Időjárás, 126(1), 47–68. https://doi.org/10.28974/idojaras.2022.1.3
- Mohammed, K., Batung, E., Kansanga, M., Nyantakyi-Frimpong, H., & Luginaah, I. (2021). Livelihood diversification strategies and resilience to climate change in semi-arid northern Ghana. Climatic Change, 164(3–4), 1–23. https://doi.org/10.1007/s10584-021-03034-y
- Mostafa, S. M., Anwar, S. A., Zakey, A. S., Wahab, M. M. A., Authority, E. M., & Qobry, E.-K. (2022). Bias-correcting the temperature extremes of Egypt using a high-resolution regional climate model (RegCM4). Presented at 3rd International Electronic Conference on Applied Sciences, Switzerland.
- Norrgård, S. (2014). Practising historical climatology in West Africa: A climatic periodization 1750–1800. Climatic Change, 129(1–2), 131–143. https://doi.org/10.1007/s10584-014-1307-9
- Norrgård, S. (2015). Practising historical climatology in West Africa: a climatic periodization 1750–1800. Climatic Change, 129(1–2), 131–143. https://doi.org/10.1007/s10584-014-1307-9
- Nuhu, M. G., & Matsui, K. (2022). Gender dimensions of climate change adaptation needs for smallholder farmers in the upper east region of Ghana. Sustainability, 14(16), 10432. https://doi.org/10.3390/su141610432
- O’Neill, B. (2016). The shared socioeconomic pathways (SSPs) and their extension and use in impact, adaptation and vulnerability studies. The World’s Largest Open Access Agricultural & Applied Economics Digital Library.
- O’Neill, B. C., Kriegler, E., Ebi, K. L., Kemp-Benedict, E., Riahi, K., Rothman, D. S., Van Ruijven, B. J., Van Vuuren, D. P., Birkmann, J., Kok, K., Levy, M., & Solecki, W. (2017). The roads ahead: Narratives for shared socioeconomic pathways describing world futures in the 21st century. Global Environmental Change, 42, 169–180. https://doi.org/10.1016/j.gloenvcha.2015.01.004
- O’Neill, B. C., Tebaldi, C., Van Vuuren, D. P., Eyring, V., Friedlingstein, P., Hurtt, G., Knutti, R., Kriegler, E., Lamarque, J.-F., Lowe, J., Meehl, G. A., Moss, R., Riahi, K., & Sanderson, B. M. (2016). The scenario model intercomparison project (ScenarioMIP) for CMIP6. Geoscientific Model Development, 9(9), 3461–3482. https://doi.org/10.5194/gmd-9-3461-2016
- Orkodjo, T. P., Kranjac-Berisavijevic, G., & Abagale, F. K. (2022). Impact of climate change on future availability of water for irrigation and hydropower generation in the Omo-Gibe basin of Ethiopia. Journal of Hydrology: Regional Studies, 44, 101254. https://doi.org/10.1016/j.ejrh.2022.101254
- Pörtner, H.-O., Roberts, D. C., Adams, H., Adler, C., Aldunce, P., Ali, E., Begum, R. A., Betts, R., Kerr, R. B., & Biesbroek, R. (2022). Climate change 2022: Impacts, adaptation and vulnerability. IPCC.
- Riahi, K., Van Vuuren, D. P., Kriegler, E., Edmonds, J., O’neill, B. C., Fujimori, S., Bauer, N., Calvin, K., Dellink, R., Fricko, O., Lutz, W., Popp, A., Cuaresma, J. C., KC, S., Leimbach, M., Jiang, L., Kram, T., Rao, S. … Tabeau, A. (2017). The shared socioeconomic pathways and their energy, land use, and greenhouse gas emissions implications: An overview. Global Environmental Change, 42, 153–168. https://doi.org/10.1016/j.gloenvcha.2016.05.009
- Somorin, O. A. (2010). Climate impacts, forest-dependent rural livelihoods and adaptation strategies in Africa: A review. African Journal of Environmental Science and Technology, 4(13), 903–912.
- Stanturf, J., Warren, M., Charnley, S., Polasky, S. C., Goodrick, S. L., Armah, F., & Nyako, Y. A. (2011). Ghana climate change vulnerability and adaptation assessment. United States agency for international development.
- Taylor, K. E., Stouffer, R. J., & Meehl, G. A. (2012). An overview of CMIP5 and the experiment design. Bulletin of the American Meteorological Society, 93(4), 485–498. https://doi.org/10.1175/BAMS-D-11-00094.1
- Vaittinada Ayar, P., Vrac, M., & Mailhot, A. (2021). Ensemble bias correction of climate simulations: Preserving internal variability. Scientific Reports, 11(1), 1–9. https://doi.org/10.1038/s41598-021-82715-1
- Van Vuuren, D. P., Stehfest, E., Gernaat, D. E., Doelman, J. C., Van den Berg, M., Harmsen, M., de Boer, H. S., Bouwman, L. F., Daioglou, V., Edelenbosch, O. Y., Girod, B., Kram, T., Lassaletta, L., Lucas, P. L., van Meijl, H., Müller, C., van Ruijven, B. J., van der Sluis, S., & Tabeau, A. (2017). Energy, land-use and greenhouse gas emissions trajectories under a green growth paradigm. Global Environmental Change, 42, 237–250. https://doi.org/10.1016/j.gloenvcha.2016.05.008
- Willkofer, F., Schmid, F.-J., Komischke, H., Korck, J., Braun, M., & Ludwig, R. (2018). The impact of bias correcting regional climate model results on hydrological indicators for Bavarian catchments. Journal of Hydrology: Regional Studies, 19, 25–41. https://doi.org/10.1016/j.ejrh.2018.06.010
- Wong, J. S., Razavi, S., Bonsal, B. R., Wheater, H. S., & Asong, Z. E. (2017). Inter-comparison of daily precipitation products for large-scale hydro-climatic applications over Canada. Hydrology and Earth System Sciences, 21(4), 2163–2185. https://doi.org/10.5194/hess-21-2163-2017
- Zhu, X., Ji, Z., Wen, X., Lee, S. Y., Wei, Z., Zheng, Z., & Dong, W. (2021). Historical and projected climate change over three major river basins in China from fifth and sixth coupled model intercomparison project models. International Journal of Climatology, 41(15), 6455–6473. https://doi.org/10.1002/joc.7206