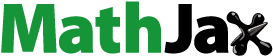
Abstract
Coffee is the most critical stimulant beverage in the world and represents a significant source of income in many tropical and subtropical countries. In this paper, a deterministic mathematical model has been formulated to describe the infestation dynamic of coffee berry borer (CBB) using a system of non-linear ordinary differential equations with farmers' awareness and optimal control. The system has two equilibrium points, namely the CBB free equilibrium point and the endemic equilibrium point which exist conditionally. The basic reproduction number, which plays a vital role in mathematical epidemiology, was derived. The qualitative analysis of the model revealed the scenario for both CBB free equilibrium and endemic equilibrium points. The local stability of the equilibria is established via the Jacobian matrix and Routh–Hurwitz criteria, while the global stability of the equilibria is proven by using an appropriate Lyapunov function. The normalized sensitivity analysis has also been performed to observe the impact of different parameters on the basic reproduction number. We extended the proposed model into an optimal control problem by incorporating two control variables, the effort made to reduce the colonizing females based on chemicals, traps, and biological control using entomopathogenic fungi such as Beauveria bassiana, that are applied to the surface of the coffee berries and kill the colonizing females of CBB when they drill an entry hole into the coffee berry and the effort made to increase the awareness of farmers through media campaign and education for the coffee farmer. Then the optimal control strategy is found by minimizing the number of CBB individuals considering the cost of implementation. The existence of optimal controls is examined using Pontryagin's minimum principle. Finally, the numerical simulations show agreement with the analytical results.
1. Introduction
Coffee is the most critical stimulant beverage in the world and represent a significant source of income in many tropical and subtropical countries. It is produced in more than 80 countries and is worth about $24 billion from exports [Citation1]. Globally, in producing and consuming countries, coffee is an important commodity [Citation2]. Coffee production is affected by several pests, weeds and diseases. Among these pests, coffee berry borer (CBB), Hypothenemus hampei was the most harmful pest that affects commercial coffee production and prevalent in most coffee-producing countries [Citation3]. Antestia bug and coffee blotch miner also widely damage the production of coffee [Citation4].
CBB was first reported in Gabon (1901), and soon after in Kenya in 1928 [Citation5]. Later, it had quickly spread throughout most all the 80 coffee-producing countries [Citation6]. CBB is a pest that harms immature and mature coffee berries without damaging leaves, branches, and stems. The female CBB drills the apex of the coffee berry eats and oviposit inside the coffee berry. The larvae also feed on the same seed until they grow and reach the adult stage. Mature females are responsible for the dispersal of the population: they emerge from the berries to colonize and lay their eggs in new berries, while males and larvae stages remain inside the berries [Citation5]. It damages the weight and quality because it feeds and reproduces inside the coffee berry [Citation7]. Sibling mating inside the coffee berry makes this pest quite difficult to control.
The infestation intensity of CBB in coffee-growing countries is reported as Mexico 60%, Malaysia 50–90%, Colombia 60%, Jamaica 75%, Uganda 80%, and Tanzania 90% [Citation2]. Due to the intensity of the damages, several control methods have been identified and implemented in most coffee-growing countries. The common control methods are improved cultural practices, chemical, biological control, and host plant resistance [Citation8–10]. Moreover, adopting the integrated application of new technologies like television, radio, and mobile telephony provides useful information about pest outbreaks and crop protection during agricultural pest control awareness programmes [Citation11,Citation12].
Mathematical modelling has a crucial role in better understanding the dynamics of plant disease and its control. The literature on the application of mathematical modelling in the area of plant disease is extensive, see for example in [Citation13–21] and references cited therein. In [Citation22], Fotsa et al. introduced and analysed a dynamical spatial model of Anthracnose infection that include optimal control. Anthracnose is another name for CBD. They also showed how to optimize the use of chemical control with respect to a given cost function. Later, this study was generalized by incorporating an impulsive control strategy that represents cultivation practices in [Citation23]. Farmers' awareness plays a significant role in the management of pests, see for example in [Citation24–26]. However, farmers awareness was not considered in these literatures for CBB. This is will be addressed in the present study. Cunniffe and Gilligan [Citation27] investigated an epidemiological model for plant pathogens. This model ensured maximum transferability across the widest range of host–pathogen systems by using the common currency of the field and has illustrated the results in a class of models that have been experimentally tested for plant disease.
The aim of this study is to provide quantitative and qualitative explanations for the dynamics of CBB infestation by taking into account vectors' population and farmers' awareness. For this, we propose a nonlinear deterministic mathematical model to investigate the dynamics of CBB infestation in the presence of farmers' awareness. Also, we extend the proposed model into an optimal control problem.
The rest of this work is organized as follows. In Section 2, a mathematical model for CBB infestation is derived. The stability analysis of the proposed model is investigated in Section 3. Section 4 deals with the extended mathematical model into an optimal control problem. The obtained analytical results are shown through numerical simulations in Section 5. Cost-effectiveness analysis is discussed in Section 6. Finally, conclusions are drawn in Section 7.
2. Model derivation
In this section, we consider a mathematical model proposed by Abawari [Citation28] to study the infestation dynamics of CBB. In the model, we introduce coffee berry biomass (B), coffee borer population (Y ), and aware farmers (A). Due to the limit size of coffee plantations, however it may be large, we assume logistic growth for the biomass of coffee plant [Citation29], with growth rate r and carrying capacity k. The female CBB attacks immature and mature coffee berries from about eight weeks after flowering up to harvest season. We assumed that females bore a hole into the coffee berry and then construct galleries in the seeds where the eggs are deposited, followed by larval feeding on the coffee seed [Citation30]. The main coffee borer management measures involve different components, including regular monitoring, controlled harvest, and the use of biological control agents. These measures are useful before the females enter the coffee berries. Once, it entered the coffee berries it is difficult to control the pest [Citation2]. Thus, we assumed that infected female bores do not affect the coffee berries. This is due to its lack of power to drill into coffee berries. The natural death rate of coffee berry is denoted by μ. The female borer dies at a rate d.
The per capita rate at which a female borer attacks coffee berry is represented by . Here, γ represents the attack rate of a female borer on coffee berries while c represents the half-saturation constant. Then the founder female remains inside the fruit after oviposition until she dies, taking care of the offspring. Hence, when the new adult females emerge, they are already inseminated and ready to attack another coffee berry, in which they continue the cycle. Most of the life cycle occurs inside the coffee berry.
The farmer awareness measures the information density available among the farmers due to the prevalence of the CBB. It is assumed to increase due to media campaigns and agricultural field workers. We assumed that the rate of farmers' awareness is proportional to the infestation burden and increases with rate α. As a result of awareness farmers surveillance their farm, and use biological control or trap to avoid borer infestation. There is a farmers' activity rate, β, due to self-aware human inter- actions and activity such as the use of bio-pesticides, modelled via the mass action term . Here, δ is the constant related to saturation in information-induced intervention. We also assumed farmers' memory faded and thus, the level of awareness decreased at a rate e.
Based on the above assumptions, the mathematical model is described by nonlinear systems of ordinary differential equations:
(1)
(1) where
.
Here σ and θ denote conversion rate and rate of awareness due to global source, respectively. Compared to the mathematical model introduced in [Citation11], the present model differs due to the logistic growth model and farmer's awareness being included.
3. Model analysis
3.1. Positivity and boundedness of solutions
In this section, we study a region in which the solution of the governing system (Equation1(1)
(1) ) is both epidemiologically and mathematically meaningful. That is, the solution of the proposed model is nonnegative and bounded with positive initial data in a certain feasible region. Now we have the following theorem.
Theorem 3.1
If the initial data , then the solutions
of the system (Equation1
(1)
(1) ) are non-negative for all time
and bounded. Moreover, the feasible compact set is given by
where
and
.
Proof.
From the first equation system (Equation1(1)
(1) ), we have
(2)
(2) Integrating Equation (Equation2
(2)
(2) ), we obtain
Similarly, from the second and third equations of system (Equation1
(1)
(1) ), we get
respectively. This implies that all the solution with positive initial data remains in
for all
. This means, the region attracts all solutions of the governing system.
Next, we determine the feasible region in which the solution of the model (Equation1(1)
(1) ) is bounded. Let us consider the first equation of system (Equation1
(1)
(1) )
(3)
(3) Integrating the last inequality of Equation (Equation3
(3)
(3) ) by separation of variables, we obtain
From which we deduce that
and we have
.
Let at any time t and
, then by adding the first and second equation of system (Equation1
(1)
(1) ), we obtain
It follows that
(4)
(4) Solving Equation (Equation4
(4)
(4) ) gives that
. If
, then we have
Now, plunging
in the third equation of system (Equation1
(1)
(1) ) and after manipulation we obtain
This implies that
(5)
(5) Again, solving Equation (Equation5
(5)
(5) ) we get,
If
, then it follows that
Thus, the invariant region is given by
Hence, the solutions of the model equations are bonded and nonnegative in the region Ω.
3.2. Pest-free equilibrium point
Pest-free equilibrium point (PFEP) is steady-state solutions where there is no CBB (i.e. Y = 0). That is, the borer class is zero and the entire population comprises of the coffee berry and the information density available among the farmers. Then, we obtain CBB free equilibrium point:
(6)
(6)
Remark 3.2
PFEP in Equation (Equation6
(6)
(6) ) is biologically feasible when
.
3.3. Basic reproduction number (
)
The basic reproduction number is the average number of new females CBB originating from a single infesting female in the healthy coffee berries in plantation [Citation31]. A method for computing the
in epidemiological models was developed in [Citation32]. Let
be the set of state variables. The system (Equation1
(1)
(1) ) can be rewritten as
where
To obtain the next generation matrix, we compute the Jacobian matrices of
and
denoted by
and
, where
The basic reproductive number
is obtained by computing the spectral radius of the next generation matrix
so that
3.4. Local stability of PFEP
Theorem 3.3
The PFEP is locally asymptotically stable if
and unstable if
in Ω.
Proof.
The Jacobian matrix of system (Equation1(1)
(1) ) at PFEP
is given by
(7)
(7) From the Jacobian matrix (Equation7
(7)
(7) ), we obtained the characteristic polynomial:
(8)
(8) From Equation (Equation8
(8)
(8) ), we obtain that
Here we suppose that the growth rate is greater than natural death rate of coffee berries (i.e.
). Applying the Routh–Hurwitz criterion [Citation33] on Equation (Equation8
(8)
(8) ) shows that all eigenvalues are negative and so
is locally asymptotically stable for
in Ω.
3.5. Global stability of PFEP
Theorem 3.4
The PFEP of the model (Equation1
(1)
(1) ) is globally asymptotically stable if
in Ω.
Proof.
Let us develop the following Lyapunov function:
The Lyapunov function U needs to satisfy the conditions:
for all
and
Then differentiating U with respect to t, we obtain
(9)
(9) Thus if
, then
for all
. Therefore,
is globally asymptotically stable for
in Ω.
3.6. Endemic equilibrium point
In the presence of CBB, the system (Equation1(1)
(1) ) has an equilibrium point called endemic equilibrium point (EEP). That means, EEP exists when
. It is denoted by
and can be obtained by equating the left-hand side of system (Equation1
(1)
(1) ) to zero as follows:
(10)
(10) By solving Equation (Equation10
(10)
(10) ), we get
and
in terms of
:
(11)
(11) From Equation (Equation11
(11)
(11) ) substituting
into the first equation of Equation (Equation10
(10)
(10) ), we obtain
that satisfies the following equation:
(12)
(12) where
3.7. Local stability of EEP
Theorem 3.5
The endemic equilibrium of system (Equation1
(1)
(1) ) is locally asymptotically stable in Ω if
, otherwise unstable.
Proof.
First, we obtain the Jacobian matrix of system (Equation1(1)
(1) )
(13)
(13) Evaluating the Jacobian matrix (Equation13
(13)
(13) ) at the endemic equilibrium
, we get
(14)
(14) where
From Jacobian matrix (Equation14
(14)
(14) ) one can easily obtain the characteristic polynomial as
(15)
(15) where
According to the Routh–Hurwitz criterion [Citation33], for
, the endemic equilibrium
is locally asymptotically stable if,
and unstable otherwise.
3.8. Global stability of EEP
Theorem 3.6
The EEP of system (Equation1
(1)
(1) ) is globally asymptotically stable if
.
Proof.
To establish the global stability of , we consider the following the Lyapunov function:
(16)
(16) Then by taking the derivative of G with respect to t and let
, we obtain
(17)
(17) On the other hand, from Equations (Equation4
(4)
(4) ) and (Equation5
(5)
(5) ), we have
(18)
(18) where
,
. Substituting expression for
and
from Equations (Equation18
(18)
(18) ) to (Equation19
(19)
(19) ) leads to
(19)
(19) By re-arranging and simplifying Equation (Equation19
(19)
(19) ), we obtain
(20)
(20) Thus,
and
if and only if
,
,
. Thus, the largest compact invariant set in
is the singleton
. Therefore, by LaSalle's invariant principle [Citation34], as
, all the solutions of the system (Equation1
(1)
(1) ) approaches
in Ω for
.
3.9. Sensitivity of the basic reproduction number
In this section, we perform sensitivity analysis in order to determine the relation of model parameters to pest expansions. This help us to check and classify parameters which extremely affect the basic reproduction number and thus determine an appropriate parameter values to minimize pests from coffee berry population.
Definition 3.1
[Citation35] The definition of normalized forward sensitivity index of with respect to P is given by
(21)
(21) where
is a given variable and P is a set of parameter.
By applying Definition Equation21(21)
(21) , sensitivity index of
is computed and their sensitivity indices are given in Table .
Table 1. Sensitivity indices.
3.10. Interpretation of sensitivity indices
The sensitivity indices of (when
and
) with respect to the main parameters are found in Table . Those parameters that have positive indices r, σ, γ, k, δ, and e show that they have a great impact on expanding the female CBB in the farm if their values are increasing. Also, those parameters in which their sensitivity indices are negative such as β, μ, θ, d and c have an effect of minimizing the burden of the borer in the berry population as their values increase while the others are left constant. As their values increase
decreases, which leads to minimizing the endemicity of the borers in the coffee berry population. The bar diagram of the sensitivity indices in Table is represented in Figure .
Figure 1. Normalized sensitivity indices of with respect to parameters of the model (Equation1
(1)
(1) ). (a) When
. Parameter values are taken from Table . (b) When
. Parameter values are taken from Table , except
.
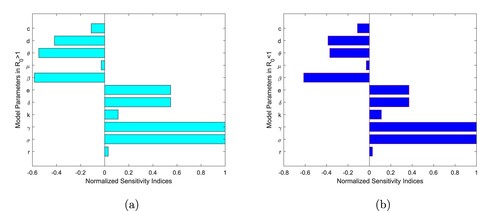
Table 2. The values of parameters used in the simulations.
4. Extension of the model into optimal control
In this section, we extend the proposed model (Equation1(1)
(1) ) into the optimal control problem. Hence, we want to advise the concerned the effectiveness of those strategies if they are fulfilled at an optimal level. This helped us to identify the best control strategies that were used to eradicate the pests from the coffee berry population in the specified time.
As one approach, we proposed educating coffee farmers on the CBB life cycle and its reservoir for effective management in combination with the trap. Because after harvest, all the remaining coffee berries that have fallen must be removed as they are a reservoir in which the CBB can live until the next coffee crop is ripe enough to infest. The method is environmentally friendly but labour intensive. The trap also helps to minimize the female borer, but it is useful only when the pest is outside the fruit.
To apply optimal control, we classify those activities into two groups listed below. The first control represents the effort made to reduce the colonizing females by using chemicals, traps, and biological control (Beauveria bassiana), that are applied to the surface of the coffee berries and kill the colonizing females of CBB when they drill an entry hole into the coffee berry. The second control
represents the effort made to increase the awareness of farmers through media campaign and education for coffee farmer. The corresponding state system for model (Equation1
(1)
(1) ) is given by
(22)
(22) where
.
The number of CBB Y and the implementation cost of strategies related to the controls and
are considered in cost functional (Equation23
(23)
(23) ). The cost functional can be defined for the minimization problem as
(23)
(23) where constants C,
and
are positive. The weight constants
and
are the measure of relative costs of interventions associated with the controls
and
, respectively, and also balance the units of the integrand. Larger values of
and
tell us the expensiveness of implementation cost. The terms
and
describe the cost associated with the traps, biological control, and awareness campaign, respectively. In the cost function, the term
describes the cost related to killing colonizing female borers at the end of the season. The quadratic term in the objective function is taken because the intervention is nonlinear [Citation36,Citation37]. Moreover, the functional
corresponds to the total cost due to CBB infestation and its control strategies.
Further, the integrand function measures the current cost at time t. The fixed constant T denotes the terminal treatment time. The set of admissible controls is defined as
(24)
(24) Then, we consider the optimal control problem of determining
associated with an admissible control
on the intervention time interval
, subjected to the state control system (Equation22
(22)
(22) ) in
with its initial data and minimizing the cost functional (Equation23
(23)
(23) ). More precisely, the optimal control problem can be defined as
(25)
(25) satisfying (Equation22
(22)
(22) ) and its initial data.
4.1. Existence of the optimal control
In this subsection, we prove the existence of optimal control functions which minimize the cost function in the finite intervention period. The following result guarantees the existence of optimal control functions. A detailed and similar analysis of the existence of optimal control can be found in [Citation38,Citation39].
Theorem 4.1
There exists an optimal control and a corresponding solution vector
to the state system (Equation22
(22)
(22) ) that minimize the cost functional
of (Equation23
(23)
(23) ) over the set of admissible control V.
Proof.
All the state variables involved in the model are continuously differentiable. Therefore, we need to verify the following four conditions given in [Citation38].
The set of all solution to state system (Equation22
(22)
(22) ) with corresponding control functions in (Equation24
(24)
(24) ) is non-empty;
The control set is convex and closed;
The right-hand side of the state system (Equation22
(22)
(22) ) is bounded by a linear function in the state and control variable;
The integrand L of (Equation23
(23)
(23) ) is convex on V and
, where g is continuous and
as
.
The existence of the solution of the system (Equation22(22)
(22) ) is obtained in using result from [Citation40], (Theorem 9.2.1), since state system (Equation22
(22)
(22) ) has bounded coefficients and any solution is bounded on the finite interval time
, so condition (i) is satisfied. To proof (ii), consider
Let
such that
and
. Then for any
,
This implies that the control set V is convex and closed. The state system (Equation22
(22)
(22) ) is clearly linear in control variables
and
with coefficients depending on state variables. With this condition (iii) is satisfied. The integrand of the cost functional
(26)
(26) let
, and define a continuous function
. Then from Equation (Equation26
(26)
(26) ) we have
. Clearly
as
. Thus, condition (iv) is achieved. Therefore, the existence of an optimal control pair
satisfying Equations (Equation22
(22)
(22) ) and (Equation25
(25)
(25) ) is assured by results given in [Citation38].
4.2. Characterization of the optimal control solution
In order to derive the necessary conditions for the optimal control pair, Pontryagin's minimum principle [Citation41] is used. According to the this principle, for to be optimal with corresponding optimal state
which minimizes the cost functional (Equation22
(22)
(22) ) for a fixed final time T, then there exists a non-trivial absolutely continuous mapping
called the adjoint vector such that
the Hamiltonian function is defined as
(27)
(27) where
stands for the right hands of the constraints (Equation22
(22)
(22) ) for i = 1, 2, 3.
The control system
(28)
(28)
The adjoint system
(29)
(29)
The optimality condition
(30)
(30) holds for almost all
Moreover, the transversality condition
(31)
(31)
Theorem 4.2
There exists an optimal solution and corresponding optimal control
that minimize
over the set of admissible control Ω. Moreover, there exists an adjoint function
such that
(32)
(32) with transversality conditions
.
Furthermore, the following properties holds: (33)
(33) where
.
Proof.
The Hamiltonian function associated with the system is defined as follows:
The adjoint system can be obtained as
The state variables are not assigned at the final time T. Hence, from [Citation37], the transversality conditions (or boundary conditions) become
for i = 1, 2, 3. By the optimality condition, we have:
That is,
⇔
at
.
⇔
at
.
From boundedness on and minimality condition, we have:
Here also from boundedness on
and the minimality condition, we have:
In compact notation:
5. Numerical simulations
In this section, we provide numerical simulations obtained from the application of our analytical results, as given in previous sections. For this purpose, we use the forward-backward sweep method [Citation42]. In order to find numerical solutions of the optimality system, first the state system (Equation22(22)
(22) ) is computed forward with the given initial condition and controls initial guess in time by using a Runge–Kutta method of fourth order. Next, the adjoint system (Equation32
(32)
(32) ) is computed backward with the transversality condition in time by using the Runge–Kutta algorithm of fourth order. Each control variable value is modified by averaging the new value and old value arising from the characteristic control (Equation33
(33)
(33) ). This steps continues many times up to successive iterations are close enough to each other.
To perform the simulation, a set of meaningful values are assigned to the model parameters. These values are either taken from the literature or assumed. Accordingly, parameter values are given in Table , and with initial data: and
. To achieve optimal control strategies, the weight constants of the objective function are assumed:
and the adjoint system with terminal condition:
for the implementation time
months.
We begin by simulating system (1) without controls. Figure (a,b) shows that CBB decreases with aware farmer growth rate and awareness rate increase. From Figure , we observe that the number of CBB decreases in the presence.
In Figure , the behavioural patterns of the densities of coffee berry biomass, CBB, and aware farmers are presented. All the system populations oscillate initially and finally become asymptotically stable and converge to the endemic state value. Here, it is to be noted that all conditions of Theorem 4 are satisfied and thus the endemic equilibriumStrategy A: Applying traps or biological control (
).
Strategy B: Using farmers awareness (
).
Strategy C: Using all controls (
and
).
Figure 4. Stability behaviour of the endemic state for the system (Equation1(1)
(1) ) when
and
.
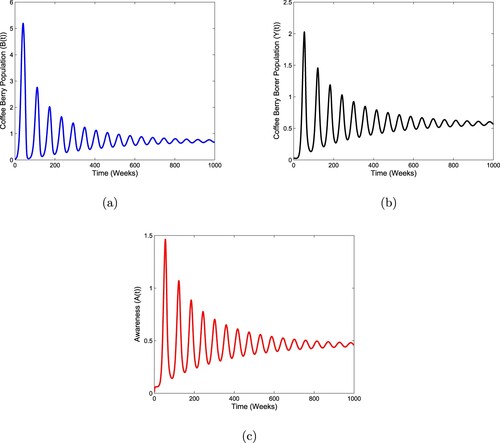
6. Cost-effectiveness analysis
In this section, we study cost-effectiveness for three different control strategies. To obtain this, we follow the method applied in [Citation44]. It depends on computing the incremental cost-effectiveness ratio (ICER) and given by
That is, the ICER of strategy A, strategy B, and strategy C is given as
(34)
(34) where
is a CBB without control and
is a CBB with control. Each control strategy is compared with the next less effective alternative strategies. The total control cost
is given by
(35)
(35) The functions
and
represent the cost weights associated with control
and
measure, respectively. The numerical output for the three control strategies are rated incrementally in the form of CBB averted as shown in Table .
Table 3. Total coffee berry borer averted and control costs.
We calculate the ICER for strategy A, strategy B, and strategy C which are given in Table and then compare their results as follows:
The comparison between strategy B and strategy A showed a cost saving of 0.4149 for strategy B over strategy A. This shows that strategy A is more expensive and less effective compared to strategy B. Thus, strategy A is excluded and we continue to compare the ICER for strategy B over strategy C from Table as follows.
Comparing strategy B and strategy C, we observe that ICER (B) is greater than ICER (C). Since strategy B consumes more resources, it has to be excluded from the set of alternatives. This value shows that strategy C is the cheapest. As a result, strategy C is the most cost-effective among the control strategies for the control of coffee berry borer.
7. Conclusions
In this study, we have developed a mathematical model which describes the infestation dynamic of CBB with farmer awareness and optimal control using a system of nonlinear ordinary differential equations. The qualitative analysis reveals that CBB-free equilibrium point is both locally and globally stable for . If the impact of awareness campaigns increases, the density of crop increases. On the other hand, EEP is both locally and globally stable if
. Sensitivity analyses of the model have been studied and determine a parameter that plays a greater role in the infestation dynamics of CBB. We have used two controls:
(traps and biological control) and
(awareness of farmers). The Hamiltonian, the adjoint variables, the characterization of the controls, and the optimality system of our model were derived and analysed using Pontryagin's minimum principle. We performed numerical simulations on the optimality system considering integrated strategies, as on seasonal coffee infestation by CBB has used. The numerical results clearly indicate that each integrated management strategy has the power to combat the pest. Therefore, the results of this study show that optimal control is sufficient to decrease pests from the coffee berry population with lowered cost at the end of the forty fifth month.
Table 4. Total coffee berry borer averted, control costs and ICER.
Acknowledgments
The authors thank Adama Science and Technology University for its hospitality and support during this work.
Disclosure statement
No potential conflict of interest was reported by the author(s).
References
- Coffee FSP. Food and agriculture organization of the United Nations. 2015.
- Vega F. Coffee berry borer Hypothenemus hampei (ferrari) (coleoptera: scolytidae). Encycl Entomol. 2004;1:575–576.
- Murphy ST, Moore D. Biological control of the coffee berry borer, Hypothenemus hampei, (ferrari) (coleoptera: scolytida): previous programmes and possibilities for the future. Biocontrol News Info. 1990;11:107–117.
- Fuad A. Studies on the diversity of insect pests in wild and cultivated coffee plantations in and around Jimma, southwest Ethiopia. Int J Biodivers Conserv. 2010;8(10):233–243.
- Vega FE, Mercadier G, Dowd PF, et al. Fungi associated with the coffee berry borer Hypothenemus hampei (ferrari)(coleoptera: scolytidae). Colloq Sci Int Cafe (C.R.). 1999;18:229–238.
- Burbano E, Wright M, Bright DE, et al. New record for the coffee berry borer, Hypothenemus hampei, in Hawaii. J Insect Sci. 2011;11(117):1–3. Available online: insectscience.org/11.117.
- Chaves B, Riley J. Determination of factors influencing integrated pest management adoption in coffee berry borer in Colombian farms. Agric Ecosyst Environ. 2001;87(2):159–177.
- Damon A. A review of the biology and control of the coffee berry borer, Hypothenemus hampei (coleoptera: scolytidae). Bull Entomol Res. 2000;90(6):453–465.
- Aristizabal LF, Bustillo AE, Arthurs SP. Integrated pest management of coffee berry borer: strategies from Latin America that could be useful for coffee farmers in Hawaii. Insects. 2016;7(1):6.
- Mercanta Coffee Hunter. Coffee berry borer: What is and what damages it causes; 2016.
- Khan GA, Muhammad S, Khan MA. Information regarding agronomic practices and plant protection measures obtained by the farmers through electronic me dia. J Anim Plant Sci. 2013;23(2):647–650.
- Marcano M, Bose A, Bayman P. A one dimensional map to study multiseasonal coffee infestation by the coffee berry borer. Math Biosci. 2021;333:Article ID 108530.
- Horub KE, Julius T. A mathematical model for the vector transmission and control of banana xanthomonas wilt. J Math Res. 2017;9(4):101–113.
- Jeger MJ, Holt J, Van Den Bosch F, et al. Epidemiology of insect-transmitted plant viruses: modelling disease dynamics and control interventions. Physiol Entomol. 2004;29(3):291–304.
- Fitri IR, Hanum F, Kusnanto A, et al. Optimal pest control strategies with cost-effectiveness analysis. Sci World J. 2021;2021:Article ID 6630193. doi:10.1155/2021/6630193.
- Marcano M, Bose A, Bayman P. A one-dimensional map to study multi-seasonal coffee infestation by the coffee berry borer. Math Biosci. 2021;333:Article ID 108530.
- Rodríguez D, Cure JR, Cotes JM, et al. A coffee agroecosystem model: I. Growth and development of the coffee plant. Ecol Modell. 2011;222(19):3626–3639.
- Rodríguez D, Cure JR, Gutierrez AP, et al. A coffee agroecosystem model: II. Dynamics of coffee berry borer. Ecol Modell. 2013;248:203–214.
- Rodríguez D, Cure JR, Gutierrez AP, et al. A coffee agroecosystem model: III. Parasitoids of the coffee berry borer (Hypothenemus hampei). Ecol Modell. 2017;363:96–110.
- Melese AS, Makinde OD, Obsu LL. Modelling and analysis of pathogens impact on the plant disease transmission with optimal control. J Appl Nonlinear Dyn. 2022;11(3):499–521.
- Melese AS, Makinde OD, Obsu LL. Mathematical modelling and analysis of coffee berry disease dynamics on a coffee farm. Math Biosci Eng. 2022;19(7):7349–7373.
- Fotsa D, Houpa E, Bekolle D, et al. Mathematical modelling and optimal control of anthracnose. arXiv preprint arXiv:1307.1754. 2013.
- Mbogne DJF, Thron C. Optimal control of anthracnose using mixed strategies. Math Biosci. 2015;269:186–198.
- Abraha T, Al Basir F, Obsu LL, et al. Pest control using farming awareness: impact of time delays and optimal use of biopesticides. Chaos Solit Fractals. 2021;146(2021):Article ID 110869.
- Al BasIr F, Banerjee A, Ray S. Role of farming awareness in crop pest management – a mathematical model. J Theoret Biol. 2019;461:59–67.
- Khan GA, Muhammad S, Khan MA. Information regarding agronomic practices and plant protection measures obtained by the farmers through electronic media. J Anim Plant Sci. 2013;23(2):647–650.
- Cunniffe NJ, Gilligan CA. Invasion, persistence and control in epidemic models for plant pathogens: the effect of host demography. J R Soc Interface. 2010;7(44):439–451.
- Abawari MA. A mathematical model for coffee berry borer management in coffee farm with optimal control [dissertation]. Adama (Ethiopia): ASTU; 2021.
- Chowdhurya J, Basir FA, Takeuchic Y, et al. A mathematical model for pest management in Jatropha curcas with integrated pesticides – an optimal control approach. Ecol Complex. 2019;37:24–31.
- Johnson MA, Ruiz-Diaz CP, Manoukis NC, et al. Coffee berry borer (Hypothenemus hampei), a global pest of coffee: perspectives from historical and recent invasions, and future priorities. Insects. 2020;11(12):882.
- Fotso Fotso Y, Grognard F, Tsanou B, et al. Modelling and control of coffee berry borer infestation. HAL Id: hal-01871508. 2018.
- Driessche P, Watmough J. Reproduction numbers and subthreshold endemic equilibria for compartment models of disease transmission. Math Biosci. 2002;180(1-2):29–48.
- Mojeeb A, Osman E, Isaac AK. Simple mathematical model for malaria transmission. J Adv Math Comp Sci. 2017;25:1–24.
- LaSalle JP. The stability of dynamical systems. Philadelphia (PA): Society for Industrial and Applied Mathematics; 1976.
- Chitnis N, Cushing JM, Hyman JM. Bifurcation analysis of a mathematical model for malaria transmission. SIAM J Appl Math. 2006;67(1):24–45.
- Chowdhury J, Al Basir F, Takeuchi Y, et al. A mathematical model for pest management in Jatropha curcas with integrated pestcides – an optimal control approach. Ecol Complex. 2019;37:24–31.
- Al Basir F, Ray S. Impact of farming awareness based roguing, insecticide spraying and optimal control on the dynamics of mosaic disease. Ric Di Mat. 2020;69(1):1–11.
- Fleming WH, Rishel RW. Deterministic and stochastic optimal control. Vol. 1. New York: Springer Science & Business Media; 2012.
- Kumar S, Takeuchi Y. Optimal control of infectious disease: information-induced vaccination and limited treatment. Phys A: Stat Mech Appl. 2020;542:123196.
- Lukes DL. Differential equations: classical to controlled. 1982.
- Pontryagin LS. Mathematical theory of optimal processes. London (UK): Routledge; 2018.
- Lenhart S, Workman JT. Optimal control applied to biological models. London (UK): CRC Press; 2007.
- Abraha T, Al Basir F, Obsu LL, et al. Pest control using farming awareness: impact of time delays and optimal use of biopesticides. Chaos Solit Fractals. 2021;146:110869.
- Alemneh HT, Makinde OD, Theuri DM. Optimal control model and cost effectiveness analysis of maize streak virus pathogen interaction with pest invasion in maize plant. Egypt J Basic Appl Sci. 2020;7(1):180–193.