Abstract
In psychosomatic medicine, a harmonious brain–body interaction is an important cornerstone of physical health. The modern brain–computer interface (BCI), an interaction between the brain and abiotic devices, provides the benefits for people with the help of powerful computers. Our newly proposed term brain–apparatus communication (BAC), acknowledges the unique value of the above two interactions and further describes their interdependence; how this interdependence permits a better understanding of physical and psychological health and promotes the harmonious coexistence of people with the environment is worth exploring. This perspective article provides a general review of the three types of interactions and discusses the possible future trends.
1. Introduction
Upon the fertilization of an egg, the brain and various organs develop under the guidance of the genetic code, establishing an inextricable connection between the brain and the body. The early stages of this interaction occur in the womb. Upon birth, new-borns are faced with a vast variety of auditory, visual, and tactile stimulation from the environment that is delivered to the brain by a variety of sensory organs, leading to rapid brain development and quickly establishing the way that the brain perceives the external environment. Since both of these interactions (i.e., brain–body and brain–environment interactions) are centered on the brain, they may be collectively referred to as brain–apparatus communication (BAC) [Citation1]; this apparatus can be an internal organ (BAC-1) or an external device/environment (BAC-2). Psychosomatic medicine was first established ∼200 years ago, with an increased understanding of the BAC-1 benefitting patients; since the mid-1960s, various BAC-2 styles have been complemented with various artificial machines, especially computers, creating a brain–computer interface (BCI). The emergence of BCIs has brought good news for unlocking locked-in patients and facilitated neural rehabilitation.
Moreover, BAC-1 and BAC-2 are essential and inseparable in daily life. For example, when people play basketball, BAC-2 reflects the interactions of the brain with the basketball and team members, while BAC-1 reflects the interactions of the brain with the muscles, heart, lungs, etc. High-intensity video games provide another example; they involve the interaction between the brain and the video game as well as the interaction between the brain and many organs. In particular, this case involves simultaneous and intense internal and external interactions; we refer to it as BAC-3. In the following sections of this article, we first briefly introduce psychosomatic medicine and the BCI to provide historical background for BAC-1 and BAC-2, respectively. Next, we introduce the concept of BAC and additional examples. Finally, we discuss the importance and uniqueness of BAC for the diversity of brains across species. We suggest that promoting efficient BAC-3, which includes BAC-1 and BAC-2, will benefit human health and well-being and even inspired the effective future avenues to extend our ability.
2. Psychosomatic medicine: concept and history
In traditional Chinese medicine, “The Emperor’s Eighty-One Difficulties” 1900 years ago, recorded four methods for diagnosing various diseases including brain disorders: “looking, listening, asking, and touching.” “Looking” refers to observing the patient’s complexion, “listening” refers to hearing the sound, coughing, and breathing, “asking” refers to inquiring about their symptoms, and “touching” refers to noting their vital signs (e.g., their heart rate). Thus, even this far in the past, the concept that the body and the mind were united was understood and utilized to treat patients. In 1818, Heinroth introduced the term “psychosomatic,” yet the conceptual roots of psychosomatic medicine extend back to ancient Greece. After modern psychosomatic medicine was established as a medical discipline, it rapidly developed, passing through various stages. The central concept of psychosomatic medicine is that the brain and body are integral to all human functions; practitioners assess the psychological factors that affect individual vulnerability as well as the course and outcome of the illness and apply psychological therapies to treat physical illness. Psychosomatic medicine seeks to explain how immaterial events, such as behavioral or psychological responses to psychosocial stimuli, are transformed into physical changes, such as anatomical, enzymatic, autonomic, or endocrine responses. Additionally, this discipline attempts to elucidate the role of the central nervous system (CNS) in the control and regulation of endocrine and neural processes.
illustrates some of the representative research in the history of psychosomatic medicine. This groundwork was laid in the 1910s–1920s when Cannon investigated bodily changes accompanied by specific emotions [Citation2,Citation3] and proposed the Cannon–Bard theory. This theory emphasizes the role of the thalamic and hypothalamic centers in organizing the emotional responses to stimuli, marking a shift from the prior James–Lange theory to the study of central brain mechanisms underlying emotions [Citation4–8]; it was an important precursor to the following studies. Based on the Cannon–Bard theory, Papez proposed the mechanism of emotion, and Maclean suggested the presence of a relationship between psychosomatic disease and the “visceral brain” [Citation9,Citation10]. Alexander also identified emotional tension as a significant cause of physical illness [Citation11,Citation12], and the psychosomatic relationship between personality characteristics and life situations were described [Citation13]. The interaction between stress and physical disease, especially cardiovascular disorders, has been elucidated [Citation14,Citation15]. Thus, researchers gradually proposed a unified concept of health and disease. Engel further provided a method of interviewing subjects and assessing psychological data, developing a multifactorial model of illness that was assumed to result from interactions of the cellular, tissue, organismic, interpersonal, and environmental levels [Citation16–18]. In this stage (1930s–1960s), the focus shifted from the occurrence of disease to its context.
Figure 1. Representative studies during the development of the field of psychosomatic medicine. In the early twentieth century, Cannon’s laboratory studied the physiology of emotions for two decades; with the support and expansion of Bard’s studies, they proposed the Cannon–Bard theory that linked emotions and physiological responses. The Cannon–Bard theory was an important precursor for studies, such as Papez’s “A proposed mechanism of emotion.” In the mid-twentieth century (from the 1940s to the 1960s), the psychosomatic perspective identified different kinds of psychological factors in illness, and physiological changes were discussed. After the 1960s, researchers in the field proposed and discussed psychiatric connotations, psychosomatic theory, behavioral therapy, and clinical criteria. In the past two decades, the psychosomatic medicine framework has broadened to include numerous psychiatric and physical systems, and its measurements and clinical practices for treatment-related care come increasingly to the fore.
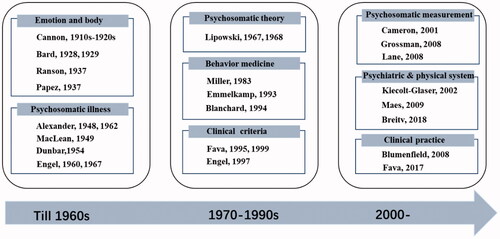
After the 1960s, the development of consultation-liaison (C-L) psychiatry provided an impetus to psychosomatic research worldwide and enhanced the psychiatric connotation of the field. During this stage, the preliminary application of psychosomatic medicine in consultation psychiatry was established [Citation19–21]. Additionally, behavioral medicine grew and was increasingly practiced. The risks of certain behaviors for the development of disease were emphasized [Citation22,Citation23] as well as the importance of family factors in the development and maintenance of severe psychosomatic problems in children [Citation24,Citation25]. Stress, trauma, and hostility were also identified as important factors in psychosomatic processes [Citation26–28]. Moreover, researchers proposed diagnostic criteria for psychosomatic syndromes [Citation29,Citation30] and outlined the relationships with current psychiatric nosology [Citation31,Citation32]. In this stage, behavioral therapy was on the rise, as it demonstrated high potential for treatment options [Citation33–36].
In the past two decades, psychosomatic medicine has continued to advance, providing new effective strategies and measurements for clinical practice [Citation37–40]. Psychosomatic medicine has linked psychiatric and physical systems and provided extensions into new areas. Various studies have concluded that immune modulation by psychosocial stressors or interventions is a core mechanism underlying a diverse set of diseases [Citation41]. Inflammatory, oxidative, and nitrosative stress pathways may be the genuine organic cause of chronic fatigue and psychosomatic disorders [Citation42]. How the brain-gut interactions work and the links of the gut microbiome with neurodevelopment and depression suggest another psychosomatic pathway of major importance [Citation43,Citation44]. Today, the field of psychosomatic medicine is more scientifically rigorous, diverse, and treatment-related than ever before. Regarding depression and anxiety, which are highly prevalent in patients with cardiovascular disease, the effectiveness of cognitive behavioral therapy has been evaluated in internal medicine care [Citation45]. Additionally, clinical practice guidelines have been refined and provided for clinical applications [Citation46–48]. In the past few years, the use of C-L psychiatry in psychosomatic medicine was recommended. Because C-L psychiatry addresses wider linkages between psychiatry and other medical professions, the integrating care in psychiatry has made the liaison role more important than ever.
Currently, the environment and human lifestyle are experiencing profound changes with advances in information science, intelligence science, environmental science, and biological science [Citation49]. Psychosomatic medicine provides a microcosm perspective on mind–body interactions in disease and health; it also provides a basis for maintaining, improving, and enhancing physiological functions in all aspects of human activities.
3. Brain–computer interface: history and progress
Humans have evolved basic ways to interact with the natural environment. For people with severe motor disabilities, BCI/brain–machine interaction (BMI) may provide a bidirectional link between the brain and external devices that assist with retrieving motor function and communication. Historically, BCI was suggested to have potential applications for neural rehabilitation or assistive devices that could be controlled directly by the brain without muscle engagement [Citation50–58]. Currently, BCI serves as an important interconnection between the brain and devices and has several applications.
Electroencephalography (EEG) is the main technology adopted for BCI. EEG-based BCI dates back to the mid-1960s, with examples, such as the brain-controlled lamp developed by Edmond Dewan [Citation59]. In 1973, Vidal coined the term “brain–computer interface” [Citation60], and in the following 50 years, BCI gradually became an epicenter of scientific interest. Many well-known BCI systems have been developed based on different types of EEG signals. Fetz published the first paper on invasive operant conditioning of cortical spike trains in animals [Citation61]. Subsequently, one of the most famous BCIs was developed based on slow cortical potential (SCP) recording by non-invasive EEG [Citation62] and was used for self-regulation and external control in 1979 [Citation63–66]. EEG rhythms, used for very early forms of EEG feedback, have brought out important BCI paradigms of self-regulation [Citation67–71]. In 1991, Wolpaw et al. achieved cursor control using the sensorimotor rhythm (SMR); recently, SMR as well as its evolved event-related desynchronization and synchronization features have been widely applied for realizing BCI systems [Citation51,Citation52,Citation72–74]. At the same time, many studies have been conducted on the use of event-related potentials (ERPs) and evoked potentials (EPs) that indicate brain responses to external and internal stimulation, such as the P300, steady-state visual evoked potential (SSVEP), and motion-onset visual evoked potential (mVEP) [Citation75–77]. Farwell and Donchin were the first to implement a P300-based BCI for spelling, and subsequently, numerous methods of optimally presenting stimuli and extracting single-trial responses were developed [Citation75,Citation78]. SSVEP is evoked by a rapid serial visual presentation [Citation77]; SSVEP-BCI has been an important mode because it does not require training, has more reliable classification characteristics, and achieves a high spelling speed [Citation79–86].
With technological advancements in acquiring brain signals, BCIs in the twenty-first century has become increasingly diverse. In 2007, a BCI system based on functional near-infrared spectroscopy (fNIRS), was developed and controlled by blood flow in the brain [Citation87]. Additionally, a neurofeedback system based on functional magnetic resonance imaging (fMRI) and a BCI system based on magnetoencephalography (MEG) were also implemented [Citation88,Citation89]. The combination of BCI with VR generated a new BCI mode [Citation90,Citation91]. A BCI system for multiperson cooperative control, i.e., multibrain BCI, was also constructed, and the first brain-to-brain interface was introduced to solve the three-person collaborative problem [Citation92,Citation93]. Many new BCI paradigms have emerged along other dimensions, including different kinds of cognitive and affective BCIs [Citation94–102]. Developing BCI paradigms that evolve from motion to mood, cognition, and from active to reactive or passive paradigms would provide more BCI applications [Citation99,Citation100,Citation103–108]. In addition, the flourishing technologies for acquiring and decoding brain signals at different scales also provide new directions for BCI. For instance, it has become possible to decode visual information from fMRI signals and synthesize speech from brain cortical activity, restoring spoken communication by applying deep learning technology [Citation109–111].
The history of BCI illustrates that the core technology of BCI is the specific paradigm, which includes the brain signals, what problems to address, and what solutions are adopted. A new paradigm may offer a new domain that attracts sufficient technological support for realization, including decoding, encoding, transmission, data collection, data processing, feature estimation, and pattern recognition, as well as new applications. In the future, more consideration should be given to the real needs of humans and the development of eco-friendly paradigms, followed by attention to supporting technologies necessary to implement these paradigms. BCI is an interactive pathway between the brain and the outside world and can provide unique avenues to understand and explore the brain function. illustrates the main developments in BCIs since 1973. Early BCIs mainly focused on motor function, but recent BCIs are expected to provide non-drug personalized treatments for the millions of patients suffering from neurological and mental disorders worldwide [Citation112].
4. Brain–apparatus communication
We physically experience and interact with the world, driving the expression of intelligent behaviors [Citation39,Citation113]. Stimuli act upon integrated physiological systems—the CNS, peripheral nervous system, endocrine system, immune system, etc.—to initiate a network of responses, thus giving rise to mind–body/body–mind interactions. Psychosomatic research, discussed above, focuses on mind–body interactions. Body–mind interactions include fields, such as embodied cognition, which emphasizes the deep importance of the physiological status on cognitive processes [Citation114,Citation115], and the study of somatopsychic disorders, which are mental disorders caused or exacerbated by somatic disorders. In particular, endocrine disorders, tumors, autoimmune disorders, and infections are associated with psychiatric symptoms [Citation116,Citation117]. In contrast, BCIs focus on the interaction between the brain and external devices. These two kinds of interactions seem to be clearly distinguished. In fact, our brain is constantly in relationship with the body’s organs and also interacts with the environment simultaneously. To encompass all these phenomena, we coined the terms “brain–apparatus communication” in 2010 and Bacomics (BAC + omics) in 2020, aiming to construct a unified framework that integrates the brain, body, and external environment [Citation1,Citation118]. As noted above, BAC can be divided into three different types of interactions: brain–body interactions (BAC-1), brain–external environment/device interactions (BAC-2), and the fusion of these two types of interactions (BAC-3) ().
Figure 3. The concept of BAC. The interaction between the brain and body (BAC-1) is represented inside the green circle, the interaction between the brain and external equipment/environment (BAC-2) is represented in the outside circle, and simultaneous internal and external interaction (BAC-3) is indicated by the overlap.
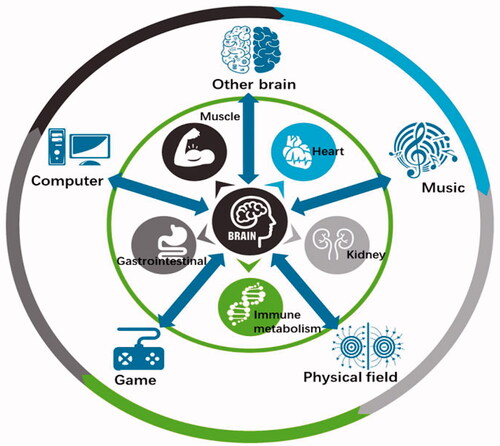
The integration of the brain and body exemplified in BAC-1 constitutes the physiological basis of human growth and development and is related to psychosomatic medicine. Network physiology has been suggested to bridge integrated physiological systems and subsystems [Citation119]. In particular, the interaction between the brain and heart has attracted increasing attention, facilitating the diagnosis and treatment of cardiovascular and cerebrovascular diseases [Citation120–124] as well as the development of new methodologies to study brain function and heartbeat dynamics [Citation125,Citation126]. provides a schematic diagram of the bidirectional brain–viscera interaction. The brain–gut axis theory proposes that gut microbes are key players in neurodevelopment and neurodegenerative diseases and may modulate the occurrence and development of neuropsychiatric diseases [Citation127–133]. The brain is also closely related to the activities of other organs, such as the muscles, stomach, lungs, liver, and kidneys [Citation134–140]. BAC-1 also highlights the interdependence of the brain and immune systems in neuroimmunology and neuropsychiatric diseases [Citation141], and different kinds of body–brain crosstalk, such as physical activity and dance, can benefit brain health [Citation142–145]. Interactions between the brain and the body, which involve the integration of multiple central and peripheral systems, will lead to novel therapeutic options [Citation146]; this active field of research offers unique opportunities to draw new theories blurring the border between cognition, emotion, and consciousness, as well as between the mind and body.
BAC-2 includes a wide range of interactions between the brain and non-biological apparatus, enabling the parsing of brain information, treatment of impaired brain, and construction of new output channels, thus realizing direct communication between the brain and external devices, related to the above concepts of BCI/BMI. presents one example, showing the neurorehabilitation of a child with cerebral palsy via a motor imagery BCI. Recently, closed-loop BCIs and more advanced BCIs have been developed. Closed-loop stimulation, which can simultaneously detect brain activity and trigger neurostimulation, is a promising technology for clinical practice [Citation147–149]. Transcranial magnetic stimulation (TMS) and transcranial electrical stimulation (tES), common neuromodulation techniques, influence the excitability or inhibition of brain regions and circuits, non-invasively modulating sensory perception and cognitive ability; they have provided important options for clinical intervention and cognitive augmentation [Citation150–154]. Additionally, real-time functional connectivity-informed neurofeedback based on fMRI has been developed; it allows self-regulation of subcortical–cortical circuits and represents a potential strategy to decrease anxiety [Citation155]. There are many comprehensive reviews or books on BCI/BMI [Citation51,Citation79,Citation156–166]. We have identified four major trends in the development of BCIs. First, BCIs have developed from the original electricity-based paradigms to integrate electricity, magnetism, sound, light, metabolism, and other inputs and outputs. Second, BCI hardware has shifted toward more flexible, wireless, miniaturized, higher-throughput, and lower-power devices. Third, BCI application scenarios have gradually expanded from rehabilitation of motor function to intervention and treatment of neurological/mental disorders, as well as to enhance perception and cognitive abilities. Fourth, BCIs have been combined with artificial intelligence, big data, digital medicine, and cloud technology, progressing from interaction to intelligent encoding/decoding and intelligent integration.
BAC-3 represents a fusion of BAC-1 and BAC-2, i.e., a fused intelligence. On the one hand, humans can enhance their intelligence and the acquisition of skills (BAC-1) depending on the communication between the brain and external objects (BAC-2); on the other hand, BAC-2 can promote the full integration of natural pathways in the human body (BAC-1). For example, controlling over a “third hand” can complement the natural movement channel, benefitting multitasking [Citation167]. In addition to the vast majority of research that focused on the brain, interfaces with peripheral nerves and organs that monitor and modulate peripheral organs have emerged as new options in the diagnosis and treatment of neurological, metabolic, and immune disorders [Citation168]. Although BAC-2 provides a promising means of controlling artificial actuators, the best solution for restoring sensation to a prosthetic limb may be the full integration of the artificial actuators into the user’s self-image [Citation169]. Therefore, in recent years, some studies have integrated the existing visual, sensory, and motor pathways of the body to restore sensory perception, improve motor ability, and control residual limbs by applying physical stimulation to the central or peripheral pathways [Citation170,Citation171]. Moreover, BAC-3 can incorporate brain-like intelligence (BAC-1) into BCIs (BAC-2), facilitating the development of artificial intelligence. Combining the neuromorphology of the brain with deep learning may lead to brain-like network models with extensive connectivity, hierarchical organization of structure and function, and time-dependent neuronal and synaptic functions, which would provide new avenues for the development of artificial intelligence and brain–apparatus intelligence (BAI) models. Other interactive approaches, such as music, video games (), computer-based training, and acupuncture, play important roles in physical and mental well-being by eliciting physiological responses in the body. For example, computer-based cognitive training has become a powerful option with the generalized beneficial effects across a large range of cognitive functions [Citation172]. The affective game, an emerging modulation approach, involves a wide range of physiological interactions and bidirectional brain-game interactions [Citation173]. Low-intensity electroacupuncture with acupoint specificity activates the vagal–adrenal axis, thus suppressing systemic inflammation and providing a neuroanatomical basis for the selection of acupoints in stimulating specific autonomic pathways [Citation174].
Figure 6. Infrared imaging of an individual playing a video game. The game loop includes psychological and physiological (e.g., cognitive, emotion, and action) inputs and context-based feedback, as well as a wide range of physiological interactions (e.g., of the brain, heart rate, breathing, muscles, metabolism, and skin temperature).
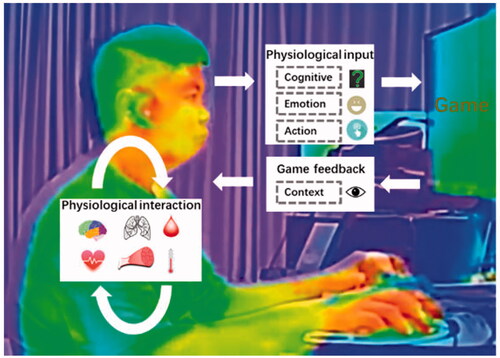
5. Diverse brains and brain–apparatus communication
Worldwide, brains exhibit a diverse array of anatomical and functional adaptations. For example, jellyfish do not have a centralized nervous system, instead possessing dispersed neurons. The brain of earthworms is small and mainly guides movement by sensing light rather than controlling the muscles. Therefore, if the brain of an earthworm is removed, it is difficult to determine changes in behavior. Caenorhabditis elegans has 302 neurons that determine its behaviors, such as chemotaxis and thermotaxis. Cockroaches have two “brains,” divided into a forebrain and a hindbrain, with one in the tail; thus, if their heads are removed, cockroaches can survive for another week. Dolphins exhibit unihemispheric sleep, resting the left or right hemispheres of their brains for ∼2 h at a time. Octopuses are very intelligent sea creatures with nine brains (one in their head and one in each tentacle), constructing brain networks. Moreover, sponges, which have no brains or nerve cells, have existed for hundreds of millions of years. Sponges are thought to have possessed nerve cells deep in their evolutionary history but to have subsequently abandoned them due to lack of use: sponges merely rest on the seabed and filter out food that flows past them. Starfish, which have eyes at the end of their arms, can detect differences in illumination, perceiving light and darkness, and thus neatly avoid predators, but they have no brain or CNS; they belong to the group of organisms that apparently lost their “brains” over the course of evolution.
Rich and varied brain patterns have been observed, with differences depending on the interactions between the brain and internal organs as well as between the brain and the living environment. Over the course of evolution, human brains became larger and much more complex, which is the key biological adaptation that allowed us to cope with natural disasters and the challenges faced by other species, and reach the top of the food chain. However, in the face of rapidly changing environments, including more complex artificial technology and the new environment of space and the deep sea, the rate of evolution of the human brain seems insufficient; brain evolution is limited by various biological aspects including physiology, biochemistry, information transmission efficiency, energy supply and delivery of abundant nutrients. That is, evolution alone is unable to meet the developing needs of humans in the real world. In this sense, the emergence of BCI can be considered an attempt to break through biological constraints, as linking computers with the brain can address both internal and external challenges.
The diversity of the biological brain determines the vividness of creatures in the biological world. Currently, BAC-1 is undoubtedly the primary and critical focus, but it is possible that brains may one day develop that solely rely on BAC-2. When the brain and external devices eliminate biological limitations, a “superman” may truly be achieved. Although this is possible in principle for a cyborg with pure BAC-2, it may take a long time and poses many ethical issues. Furthermore, if we relax the constraint of biology and assume the interaction is solely between the external environment and the “virtual brain or digital twin brain” [Citation175], a pure robot world could eventually emerge. If this robot exhibits the capacity for natural evolution, it may eventually come to rule the world. However, are there any creatures with brains but no bodies? Cosmologists assume that the low-entropy universe could give rise to many low-entropy consciousnesses, i.e., Boltzmann brains. These brains are freed from the constraints of biological organisms and may be infinitely more common than humans [Citation176].
Finally, we provide speculations on the evolution of BAC as illustrated by the five evolutionary models in . Model 1 represents a body without a brain; Model 2 represents an organism with brain–body interactions (BAC-1); Model 3 represents the coordination of the brain, body, and external objects (BAC-3 = BAC-1*BAC-2), as is currently possible; Model 4 represents the brain of an intelligent robot, such as cyborg (BAC-2); and Model 5 represents a highly intelligent agent alone (i.e., only the brain). Currently, we are at the most exciting stage of BAC evolution: Model 3. The beautiful world is all around us, and we can interact with it. Therefore, we should cherish it.
Author contributions
D.Y. conceived and designed this paper. Y.Q., Y.Z., and D.Y. wrote the paper.
Disclosure statement
No potential conflict of interest was reported by the author(s).
Additional information
Funding
References
- Yao D, Zhang Y, Liu T, et al. Bacomics: a comprehensive cross area originating in the studies of various brain-apparatus conversations. Cogn Neurodyn. 2020;14(4):425–442.
- Cannon WB. Bodily changes in pain, hunger, fear and rage. New York (NY); London: D. Appleton and Company; 1920.
- Cannon WB. The interrelations of emotions as suggested by recent physiological researches. Am J Psychol. 1914;25(2):256–282.
- Bard P. A diencephalic mechanism for the expression of rage with special reference to the sympathetic nervous system. Am J Physiol. 1928;84(3):490–515.
- Bard P. The central representation of the sympathetic system: as indicated by certain physiologic observations. Arch NeurPsych. 1929;22(2):230–246.
- Cannon WB. The James-Lange theory of emotions: a critical examination and an alternative theory. Am J Psychol. 1927;39(1/4):106–124.
- Cannon WB. The mechanism of emotional disturbance of bodily functions. N Engl J Med. 1928;198(17):877–884.
- Cannon WB. Again the James-Lange and the thalamic theories of emotion. Psychol Rev. 1931;38(4):281–295.
- MacLean PD. Psychosomatic disease and the “visceral brain”; recent developments bearing on the Papez theory of emotion. Psychosom Med. 1949;11(6):338–353.
- Papez JW. A proposed mechanism of emotion. Arch NeurPsych. 1937;38(4):725–743.
- Alexander F. The development of psychosomatic medicine. Psychosom Med. 1962;24(1):13–24.
- Alexander FE, French TME, editors. Studies in psychosomatic medicine; an approach to the cause and treatment of vegetative disturbances. Santa Fe (NM): Ronald Press; 1948.
- Dunbar HF. Emotions and bodily changes: a survey of literature on psychosomatic interrelationships. New York (NY): Columbia University Press; 1954.
- Wolff HG. Life stress and cardiovascular disorders. Circulation. 1950;1(2):187–203.
- Wolff HG. Stress and disease. Springfield (IL): Charles C. Thomas; 1953.
- Engel GL. A unified concept of health and disease. Perspect Biol Med. 1960;3:459–485.
- Engel GL. The concept of psychosomatic disorder. J Psychosom Res. 1967;11(1):3–9.
- Engel GL. The need for a new medical model: a challenge for biomedicine. Science. 1977;196(4286):129–136.
- Lipowski ZJ. Review of consultation psychiatry and psychosomatic medicine. II. Clinical aspects. Psychosom Med. 1967;29(3):201–224.
- Lipowski ZJ. Review of consultation psychiatry and psychosomatic medicine. I. General principles. Psychosom Med. 1967;29(2):153–171.
- Lipowski ZJ. Review of consultation psychiatry and psychosomatic medicine. 3. Theoretical issues. Psychosom Med. 1968;30(4):395–422.
- Krantz DS, Glass DC, Contrada R, et al. Behavior and health: the biobehavioral paradigm. In: Behavioral and social science research: a national resource: part II. Washington (DC): National Academy Press; 1982. p. 76–145.
- Miller NE. Behavioral medicine: symbiosis between laboratory and clinic. Annu Rev Psychol. 1983;34(1):1–31.
- Minuchin S, Baker L, Rosman BL, et al. A conceptual model of psychosomatic illness in children: family organization and family therapy. Arch Gen Psychiatry. 1975;32(8):1031–1038.
- Starfield B, Gross E, Wood M, et al. Psychosocial and psychosomatic diagnoses in primary care of children. Pediatrics. 1980;66(2):159–167.
- Frese M. Stress at work and psychosomatic complaints: a causal interpretation. J Appl Psychol. 1985;70(2):314–328.
- Pennebaker JW, Susman JR. Disclosure of traumas and psychosomatic processes. Soc Sci Med. 1988;26(3):327–332.
- Smith TW. Hostility and health: current status of a psychosomatic hypothesis. Health Psychol. 1992;11(3):139–150.
- Fava GA. The concept of psychosomatic disorder. Psychother Psychosom. 1992;58(1):1–12.
- Fava GA, Freyberger HJ, Bech P, et al. Diagnostic criteria for use in psychosomatic research. Psychother Psychosom. 1995;63(1):1–8.
- Engel GL. From biomedical to biopsychosocial. Being scientific in the human domain. Psychosomatics. 1997;38(6):521–528.
- Fava GA. Subclinical symptoms in mood disorders: pathophysiological and therapeutic implications. Psychol Med. 1999;29(1):47–61.
- Blanchard EB. Behavioral medicine and health psychology. New York (NY): John Wiley & Sons; 1994.
- Emmelkamp PM, Van Oppen P. Cognitive interventions in behavioral medicine. Psychother Psychosom. 1993;59(3–4):116–130.
- Fava GA. Well-being therapy: conceptual and technical issues. Psychother Psychosom. 1999;68(4):171–179.
- Hellman CJ, Budd M, Borysenko J, et al. A study of the effectiveness of two group behavioral medicine interventions for patients with psychosomatic complaints. Behav Med. 1990;16(4):165–173.
- Cameron OG. Interoception: the inside story—a model for psychosomatic processes. Psychosom Med. 2001;63(5):697–710.
- Grossman P. On measuring mindfulness in psychosomatic and psychological research. J Psychosomat Res. 2008;64(4):405–408.
- Gramann K, Gwin JT, Ferris DP, et al. Cognition in action: imaging brain/body dynamics in mobile humans. Rev Neurosci. 2011;22(6):593–608.
- Lane RD. Neural substrates of implicit and explicit emotional processes: a unifying framework for psychosomatic medicine. Psychosom Med. 2008;70(2):214–231.
- Kiecolt-Glaser JK, McGuire L, Robles TF, et al. Psychoneuroimmunology and psychosomatic medicine: back to the future. Psychosom Med. 2002;64(1):15–28.
- Maes M. Inflammatory and oxidative and nitrosative stress pathways underpinning chronic fatigue, somatization and psychosomatic symptoms. Curr Opin Psychiatry. 2009;22(1):75–83.
- Breit S, Kupferberg A, Rogler G, et al. Vagus nerve as modulator of the brain–gut axis in psychiatric and inflammatory disorders. Front Psychiatry. 2018;9:44.
- Lima-Ojeda JM, Rupprecht R, Baghai TC. “I am I and my bacterial circumstances”: linking gut microbiome, neurodevelopment, and depression. Front Psychiatry. 2017;8:153.
- Reavell J, Hopkinson M, Clarkesmith D, et al. Effectiveness of cognitive behavioral therapy for depression and anxiety in patients with cardiovascular disease: a systematic review and meta-analysis. Psychosom Med. 2018;80(8):742–753.
- Blumenfield M, Tiamson-Kassab M. Psychosomatic medicine: a practical guide. New York (NY): Lippincott Williams & Wilkins; 2008.
- Fava GA, Cosci F, Sonino N. Current psychosomatic practice. Psychother Psychosom. 2017;86(1):13–30.
- Philbrick KL, Rundell JR, Netzel PJ, et al. Clinical manual of psychosomatic medicine: a guide to consultation-liaison psychiatry. Washington (DC): American Psychiatric Pub; 2012.
- Berman MG, Kardan O, Kotabe HP, et al. The promise of environmental neuroscience. Nat Hum Behav. 2019;3(5):414–417.
- Jamil N, Belkacem AN, Ouhbi S, et al. Noninvasive electroencephalography equipment for assistive, adaptive, and rehabilitative brain-computer interfaces: a systematic literature review. Sensors. 2021;21(14):4754.
- Wolpaw JR, Birbaumer N, McFarland DJ, et al. Brain-computer interfaces for communication and control. Clin Neurophysiol. 2002;113(6):767–791.
- Wolpaw JR, McFarland DJ, Neat GW, et al. An EEG-based brain-computer interface for cursor control. Electroencephalogr Clin Neurophysiol. 1991;78(3):252–259.
- Kotchoubey B, Blankenhorn V, Fröscher W, et al. Stability of cortical self-regulation in epilepsy patients. Neuroreport. 1997;8(8):1867–1870.
- Kotchoubey B, Schleichert H, Lutzenberger W, et al. A new method for self-regulation of slow cortical potentials in a timed paradigm. Appl Psychophys Biof. 1997;22(2):77–93.
- Lebedev MA, Nicolelis MA. Brain-machine interfaces: from basic science to neuroprostheses and neurorehabilitation. Physiol Rev. 2017;97(2):767–837.
- Mudgal SK, Sharma SK, Chaturvedi J, et al. Brain computer interface advancement in neurosciences: applications and issues. Interdiscip Neurosurg. 2020;20:100694.
- Pineda JA, Allison BZ, Vankov A. The effects of self-movement, observation, and imagination on mu rhythms and readiness potentials (RP's): toward a brain-computer interface (BCI). IEEE Trans Rehabil Eng. 2000;8(2):219–222.
- Saha S, Baumert M. Intra- and inter-subject variability in EEG-based sensorimotor brain computer interface: a review. Front Comput Neurosci. 2019;13:87.
- Dewan EM. Communication by voluntary control of the electroencephalogram. Vol. 284. Air Force Cambridge Research Laboratories, Office of Aerospace Research, United States Air Force; 1966. p. 1–7.
- Vidal JJ. Toward direct brain-computer communication. Annu Rev Biophys Bioeng. 1973;2(1):157–180.
- Fetz EE. Operant conditioning of cortical unit activity. Science. 1969;163(3870):955–958.
- Walter WG, Cooper R, Aldridge VJ, et al. Contingent negative variation: an electric sign of sensorimotor association and expectancy in the human brain. Nature. 1964;203:380–384.
- Birbaumer N, Cohen LG. Brain-computer interfaces: communication and restoration of movement in paralysis. J Physiol. 2007;579(Pt 3):621–636.
- Birbaumer N, Ghanayim N, Hinterberger T, et al. A spelling device for the paralysed. Nature. 1999;398(6725):297–298.
- Elbert T, Rockstroh B, Lutzenberger W, et al. Biofeedback of slow cortical potentials. I. Electroencephalogr Clin Neurophysiol. 1980;48(3):293–301.
- Lutzenberger W, Elbert T, Rockstroh B, et al. The effects of self-regulation of slow cortical potentials on performance in a signal detection task. Int J Neurosci. 1979;9(3):175–183.
- Kamiya J. The first communications about operant conditioning of the EEG. J Neurother. 2011;15(1):65–73.
- Nowlis DP, Kamiya J. The control of electroencephalographic alpha rhythms through auditory feedback and the associated mental activity. Psychophysiology. 1970;6(4):476–484.
- Mehler DMA. Turning markers into targets – scoping neural circuits for motor neurofeedback training in parkinson’s disease. Brain-Apparatus Communication: A Journal of Bacomics. 2022;1(1):1–27.
- Plotkin WB. On the self-regulation of the occipital alpha rhythm: control strategies, states of consciousness, and the role of physiological feedback. J Exp Psychol Gen. 1976;105(1):66–99.
- Sterman MB, Howe RC, Macdonald LR. Facilitation of spindle-burst sleep by conditioning of electroencephalographic activity while awake. Science. 1970;167(3921):1146–1148.
- Birbaumer N. Breaking the silence: brain-computer interfaces (BCI) for communication and motor control. Psychophysiology. 2006;43(6):517–532.
- Pfurtscheller G, Graimann B, Huggins JE, et al. Spatiotemporal patterns of beta desynchronization and gamma synchronization in corticographic data during self-paced movement. Clin Neurophysiol. 2003;114(7):1226–1236.
- Wolpaw JR, McFarland DJ, Vaughan TM, et al. The Wadsworth center brain-computer interface (BCI) research and development program. IEEE Trans Neural Syst Rehabil Eng. 2003;11(2):204–207.
- Farwell LA, Donchin E. Talking off the top of your head: toward a mental prosthesis utilizing event-related brain potentials. Electroencephalogr Clin Neurophysiol. 1988;70(6):510–523.
- Guo F, Hong B, Gao X, et al. A brain–computer interface using motion-onset visual evoked potential. J Neural Eng. 2008;5(4):477–485.
- McMillan GR, Calhoun G, Middendorf M, et al., editors. Direct brain interface utilizing self-regulation of steady-state visual evoked response (SSVER). In: Proceedings of the RESNA ‘95 Annual Conference; Vancouver, BC; 1995.
- Allison BZ, Pineda JA. Effects of SOA and flash pattern manipulations on ERPs, performance, and preference: implications for a BCI system. Int J Psychophysiol. 2006;59(2):127–140.
- Abiri R, Borhani S, Sellers EW, et al. A comprehensive review of EEG-based brain-computer interface paradigms. J Neural Eng. 2019;16(1):011001.
- Jiang J, Yin E, Wang C, et al. Incorporation of dynamic stopping strategy into the high-speed SSVEP-based BCIs. J Neural Eng. 2018;15(4):046025.
- Zhang Y, Xu P, Cheng K, et al. Multivariate synchronization index for frequency recognition of SSVEP-based brain–computer interface. J Neurosci Methods. 2014;221:32–40.
- Chen X, Wang Y, Nakanishi M, et al. High-speed spelling with a noninvasive brain-computer interface. Proc Natl Acad Sci USA. 2015;112(44):E6058–E6067.
- Cheng M, Gao X, Gao S, et al. Design and implementation of a brain-computer interface with high transfer rates. IEEE Trans Biomed Eng. 2002;49(10):1181–1186.
- Chang MH, Baek HJ, Lee SM, et al. An amplitude-modulated visual stimulation for reducing eye fatigue in SSVEP-based brain-computer interfaces. Clin Neurophysiol. 2014;125(7):1380–1391.
- Vialatte FB, Maurice M, Dauwels J, et al. Steady-state visually evoked potentials: focus on essential paradigms and future perspectives. Prog Neurobiol. 2010;90(4):418–438.
- Volosyak I. SSVEP-based Bremen-BCI interface–boosting information transfer rates. J Neural Eng. 2011;8(3):036020.
- Coyle SM, Ward TE, Markham CM. Brain–computer interface using a simplified functional near-infrared spectroscopy system. J Neural Eng. 2007;4(3):219–226.
- Mellinger J, Schalk G, Braun C, et al. An MEG-based brain-computer interface (BCI). Neuroimage. 2007;36(3):581–593.
- Yoo S-S, Jolesz FA. Functional MRI for neurofeedback: feasibility study on a hand motor task. Neuroreport. 2002;13(11):1377–1381.
- Lotte F, Faller J, Guger C, et al. Combining BCI with virtual reality: towards new applications and improved BCI. In: Towards practical brain-computer interfaces. New York (NY): Springer; 2012. p. 197–220.
- Putze F. Methods and tools for using BCI with augmented and virtual reality. In: Brain art. New York (NY): Springer; 2019. p. 433–446.
- Jiang L, Stocco A, Losey DM, et al. BrainNet: a multi-person brain-to-brain interface for direct collaboration between brains. Sci Rep. 2019;9(1):6115.
- Nijholt A, Poel M, editors. Multi-brain BCI: characteristics and social interactions. In: International Conference on Augmented Cognition. New York, NY: Springer; 2016.
- Bartels J, Andreasen D, Ehirim P, et al. Neurotrophic electrode: method of assembly and implantation into human motor speech cortex. J Neurosci Methods. 2008;174(2):168–176.
- Guenther FH, Brumberg JS, Wright EJ, et al. A wireless brain-machine interface for real-time speech synthesis. PLOS One. 2009;4(12):e8218.
- Nijboer F, Furdea A, Gunst I, et al. An auditory brain-computer interface (BCI). J Neurosci Methods. 2008;167(1):43–50.
- Ferrez PW, del RMJ. Error-related EEG potentials generated during simulated brain-computer interaction. IEEE Trans Biomed Eng. 2008;55(3):923–929.
- Hunt AR, Kingstone A. Covert and overt voluntary attention: linked or independent? Brain Res Cogn Brain Res. 2003;18(1):102–105.
- Min BK, Chavarriaga R, Millan JDR. Harnessing prefrontal cognitive signals for brain-machine interfaces. Trends Biotechnol. 2017;35(7):585–597.
- Nijboer F, Morin FO, Carmien SP, et al., editors. Affective brain-computer interfaces: psychophysiological markers of emotion in healthy persons and in persons with amyotrophic lateral sclerosis. In: 2009 3rd International Conference on Affective Computing and Intelligent Interaction and Workshops. New York, NY: IEEE; 2009.
- Mühl C, Allison B, Nijholt A, et al. A survey of affective brain computer interfaces: principles, state-of-the-art, and challenges. Brain-Comput Interfaces. 2014;1(2):66–84.
- Widge AS, Dougherty DD, Moritz CT. Affective brain-computer interfaces as enabling technology for responsive psychiatric stimulation. Brain Comput Interfaces. 2014;1(2):126–136.
- Wu D, Liu Y, Yao D-Z. Listen to the brain in real time – the Chengdu brainwave music. J Electron Sci Technol. 2009;7(1):34–39.
- Wu D, Li CY, Yao DZ. Scale-free music of the brain. PLOS One. 2009;4(6):e5915.
- Garcia-Molina G, Tsoneva T, Nijholt A. Emotional brain–computer interfaces. IJAACS. 2013;6(1):9–25.
- Shanechi MM. Brain-machine interfaces from motor to mood. Nat Neurosci. 2019;22(10):1554–1564.
- Liu M, Tan J, Jiang Y, et al. Using deep learning to decode abnormal brain neural activity in MDD FROM single-trial EEG signals. Brain-Apparatus Communication: A Journal of Bacomics. 2022;1(1):28–37.
- Zander TO, Jatzev S, editors. Detecting affective covert user states with passive brain-computer interfaces. In: 2009 3rd International Conference on Affective Computing and Intelligent Interaction and Workshops. New York, NY: IEEE; 2009.
- Anumanchipalli GK, Chartier J, Chang EF. Speech synthesis from neural decoding of spoken sentences. Nature. 2019;568(7753):493–498.
- Horikawa T, Tamaki M, Miyawaki Y, et al. Neural decoding of visual imagery during sleep. Science. 2013;340(6132):639–642.
- Miyawaki Y, Uchida H, Yamashita O, et al. Visual image reconstruction from human brain activity using a combination of multiscale local image decoders. Neuron. 2008;60(5):915–929.
- Nicolelis MA. Brain-machine-brain interfaces as the foundation for the next generation of neuroprostheses. Natl Sci Rev. 2021;9:nwab206.
- Clark A. Being there: putting brain, body, and world together again. London: MIT Press; 1998.
- Wilson AD, Golonka S. Embodied cognition is not what you think it is. Front Psychol. 2013;4:58.
- Wilson M. Six views of embodied cognition. Psychon Bull Rev. 2002;9(4):625–636.
- Bransfield RC, Cook MJ, Bransfield DR. Differentiating psychosomatic, somatopsychic, multisystem illnesses and medical uncertainty. Healthcare. 2019;7(4):114.
- Hall RC, Gruzenski WP, Popkin MK. Differential diagnosis of somatopsychic disorders. Psychosomatics. 1979;20(6):381–389.
- Qin Y, Liu T, Yao D. Bacomics – a new discipline integrating brain and the outside. Sheng Wu Yi Xue Gong Cheng Xue Za Zhi. 2021;38(3):507–511.
- Ivanov PC, Liu KKL, Bartsch RP. Focus on the emerging new fields of network physiology and network medicine. New J Phys. 2016;18:100201.
- Gorelick PB, Furie KL, Iadecola C, et al. Defining optimal brain health in adults: a presidential advisory from the American Heart Association/American Stroke Association. Stroke. 2017;48(10):e284–e303.
- Mather M, Thayer JF. How heart rate variability affects emotion regulation brain networks. Curr Opin Behav Sci. 2018;19:98–104.
- Soufer R, Arrighi JA, Burg MM. Brain, behavior, mental stress, and the neurocardiac interaction. J Nucl Cardiol. 2002;9(6):650–662.
- Taggart P. Brain-heart interactions and cardiac ventricular arrhythmias. Neth Heart J. 2013;21(2):78–81.
- Taggart P, Critchley H, van Duijvendoden S, et al. Significance of neuro-cardiac control mechanisms governed by higher regions of the brain. Auton Neurosci. 2016;199:54–65.
- Candia-Rivera D, Catrambone V, Barbieri R, et al. Functional assessment of bidirectional cortical and peripheral neural control on heartbeat dynamics: a brain-heart study on thermal stress. Neuroimage. 2022;251:119023.
- Nann M, Haslacher D, Colucci A, et al. Heart rate variability predicts decline in sensorimotor rhythm control. J Neural Eng. 2021;18(4):0460b5.
- Fulling C, Dinan TG, Cryan JF. Gut microbe to brain signaling: what happens in vagus. Neuron. 2019;101(6):998–1002.
- Klimovich A, Giacomello S, Bjorklund A, et al. Prototypical pacemaker neurons interact with the resident microbiota. Proc Natl Acad Sci USA. 2020;117(30):17854–17863.
- Levinthal DJ, Strick PL. Multiple areas of the cerebral cortex influence the stomach. Proc Natl Acad Sci USA. 2020;117(23):13078–13083.
- Liang S, Wu XL, Hu X, et al. Recognizing depression from the microbiota-gut-brain axis. IJMS. 2018;19(6):1592.
- Long-Smith C, O'Riordan KJ, Clarke G, et al. Microbiota-gut-brain axis: new therapeutic opportunities. Annu Rev Pharmacol Toxicol. 2020;60:477–502.
- Needham BD, Funabashi M, Adame MD, et al. A gut-derived metabolite alters brain activity and anxiety behaviour in mice. Nature. 2022;602(7898):647–653.
- Vujic A, Krause C, Tso G, et al. Gut-brain computer interfacing (GBCI): wearable monitoring of gastric myoelectric activity. Annu Int Conf IEEE Eng Med Biol Soc. 2019;2019:5886–5889.
- Azzalini D, Rebollo I, Tallon-Baudry C. Visceral signals shape brain dynamics and cognition. Trends Cogn Sci. 2019;23(6):488–509.
- Hosang L, Canals RC, van der Flier FJ, et al. The lung microbiome regulates brain autoimmunity. Nature. 2022;603(7899):138–144.
- Davenport A. The brain and the kidney–organ cross talk and interactions. Blood Purif. 2008;26(6):526–536.
- Hu Y, Ji G, Li G, et al. Brain connectivity, and hormonal and behavioral correlates of sustained weight loss in obese patients after laparoscopic sleeve gastrectomy. Cereb Cortex. 2021;31(2):1284–1295.
- Li HC, Cao WF, Zhang XX, et al. BOLD-fMRI reveals the association between renal oxygenation and functional connectivity in the aging brain. Neuroimage. 2019;186:510–517.
- Mrozek S, Constantin JM, Geeraerts T. Brain-lung crosstalk: implications for neurocritical care patients. World J Crit Care Med. 2015;4(3):163–178.
- Rebollo I, Devauchelle AD, Beranger B, et al. Stomach-brain synchrony reveals a novel, delayed-connectivity resting-state network in humans. Elife. 2018;7:33321.
- Schiller M, Ben-Shaanan TL, Rolls A. Neuronal regulation of immunity: why, how and where? Nat Rev Immunol. 2021;21(1):20–36.
- Karpati FJ, Giacosa C, Foster NE, et al. Dance and the brain: a review. Ann N Y Acad Sci. 2015;1337:140–146.
- Zhang X, Zhang S, Lu B, et al. Dynamic corticomuscular multi-regional modulations during finger movement revealed by time-varying network analysis. J Neural Eng. 2022;19(3):036014.
- Gujing L, Hui H, Xin L, et al. Increased insular connectivity and enhanced empathic ability associated with dance/music training. Neural Plast. 2019;2019:9693109.
- Pedersen BK. Physical activity and muscle-brain crosstalk. Nat Rev Endocrinol. 2019;15(7):383–392.
- Cathomas F, Murrough JW, Nestler EJ, et al. Neurobiology of resilience: interface between mind and body. Biol Psychiatry. 2019;86(6):410–420.
- Lozano AM, Lipsman N, Bergman H, et al. Deep brain stimulation: current challenges and future directions. Nat Rev Neurol. 2019;15(3):148–160.
- Scangos KW, Khambhati AN, Daly PM, et al. Closed-loop neuromodulation in an individual with treatment-resistant depression. Nat Med. 2021;27(10):1696–1700.
- Sun FT, Morrell MJ. Closed-loop neurostimulation: the clinical experience. Neurotherapeutics. 2014;11(3):553–563.
- Filmer HL, Dux PE, Mattingley JB. Applications of transcranial direct current stimulation for understanding brain function. Trends Neurosci. 2014;37(12):742–753.
- Polania R, Nitsche MA, Ruff CC. Studying and modifying brain function with non-invasive brain stimulation. Nat Neurosci. 2018;21(2):174–187.
- Reinhart RMG, Nguyen JA. Working memory revived in older adults by synchronizing rhythmic brain circuits. Nat Neurosci. 2019;22(5):820–827.
- Reis J, Swayne OB, Vandermeeren Y, et al. Contribution of transcranial magnetic stimulation to the understanding of cortical mechanisms involved in motor control. J Physiol. 2008;586(2):325–351.
- Thut G, Miniussi C. New insights into rhythmic brain activity from TMS–EEG studies. Trends Cogn Sci. 2009;13(4):182–189.
- Zhao Z, Yao S, Li K, et al. Real-time functional connectivity-informed neurofeedback of amygdala-frontal pathways reduces anxiety. Psychother Psychosom. 2019;88(1):5–15.
- Chaudhary U, Birbaumer N, Ramos-Murguialday A. Brain-computer interfaces for communication and rehabilitation. Nat Rev Neurol. 2016;12(9):513–525.
- He B, Yuan H, Meng J, et al. Brain–computer interfaces. In: Neural engineering. New York (NY): Springer; 2020. p. 131–183.
- Kawala-Sterniuk A, Browarska N, Al-Bakri A, et al. Summary of over fifty years with brain-computer interfaces – a review. Brain Sci. 2021;11(1):43.
- Mak JN, Wolpaw JR. Clinical applications of brain-computer interfaces: current state and future prospects. IEEE Rev Biomed Eng. 2009;2:187–199.
- McFarland DJ, Daly J, Boulay C, et al. Therapeutic applications of BCI technologies. Brain Comput Interfaces (Abingdon). 2017;47(1–2):37–52.
- McFarland DJ, Wolpaw JR. Brain-computer interfaces for communication and control. Commun ACM. 2011;54(5):60–66.
- Moxon KA, Foffani G. Brain-machine interfaces beyond neuroprosthetics. Neuron. 2015;86(1):55–67.
- Nicolas-Alonso LF, Gomez-Gil J. Brain computer interfaces, a review. Sensors. 2012;12(2):1211–1279.
- Schalk G, Leuthardt EC. Brain-computer interfaces using electrocorticographic signals. IEEE Rev Biomed Eng. 2011;4:140–154.
- Tan DS, Nijholt A. Brain-computer interfaces. London: Springer-Verlag London Limited; 2010.
- Yadav D, Yadav S, Veer K. A comprehensive assessment of brain computer interfaces: recent trends and challenges. J Neurosci Methods. 2020;346:108918.
- Penaloza CI, Nishio S. BMI control of a third arm for multitasking. Sci Robot. 2018;3(20):aat1228.
- Shahriari D, Rosenfeld D, Anikeeva P. Emerging frontier of peripheral nerve and organ interfaces. Neuron. 2020;108(2):270–285.
- Pisotta I, Ionta S. Are we the robots?: Man-machine integration. In: Emerging theory and practice in neuroprosthetics. London: IGI Global; 2014. p. 81–100.
- Andersen RA, Kellis S, Klaes C, et al. Toward more versatile and intuitive cortical brain-machine interfaces. Curr Biol. 2014;24(18):R885–R897.
- Bouton CE, Shaikhouni A, Annetta NV, et al. Restoring cortical control of functional movement in a human with quadriplegia. Nature. 2016;533(7602):247–250.
- Kendrick KM, Yao D. Can computer-based cognitive therapy become a front-line option for prevention and treatment of mental disorders? AJP. 2017;174(4):303–304.
- Robinson R, Wiley K, Rezaeivahdati A, et al., editors. “Let’s get physiological, physiological!” A systematic review of affective gaming. In: Proceedings of the Annual Symposium on Computer-Human Interaction in Play; 2020.
- Liu S, Wang Z, Su Y, et al. A neuroanatomical basis for electroacupuncture to drive the vagal-adrenal axis. Nature. 2021;598(7882):641–645.
- Zhang G, Cui Y, Zhang Y, et al. Computational exploration of dynamic mechanisms of steady state visual evoked potentials at the whole brain level. Neuroimage. 2021;237:118166.
- Davenport M, Olum KD. Are there Boltzmann brains in the vacuum. arXiv Preprint 2010:arXiv:10080808.