Abstract
There are plenty of people suffer from limb dysfunction caused by stroke and other factors, and it is of great social significance for the rehabilitation of patients to reconstruct brain movement function and recover voluntary motor control. In the study of EEG and its network, movement has a high activation level on the central nervous system. In the study of EMG and its network, movement has a strong synergistic effect on the peripheral nervous system. Through the study of information coupling, the control feedback mechanism between these two nervous systems can be revealed. Finally, combined with the human-computer interaction rehabilitation theory and closed-loop regulation techniques, this research will help to realize the systematic and comprehensive rehabilitation for stroke hemiplegia patients.
1. Introduction
Movement has a close relationship with complex cognitive processes, which means it needs to include the synergy of motor central nervous system (CNS), motor peripheral nervous system (PNS), and other relevant systems. Bone, joint, and skeletal muscle compose the motor system. Skeletons connected in different forms are called bones, which construct the human basic form and support for muscle attachment. Under the innervated by nerves, the muscle contraction and traction attached bone and use the movable bone connection as the hub to generate the lever movement [Citation1]. However, because of the influences caused by degeneration of human functions, diseases, and external factors such as car accidents, etc., there will appear human motor function's abnormalities of different degrees and cause movement disorders. Among them, the movement disorders which caused by stroke have significant influence for patients' functional activity and quality of life [Citation2]. In other words, it will take a severe burden for both patients, families, and society [Citation3]. Therefore, the rehabilitation of limb motor function for stroke hemiplegia patients has a remarkable society significance.
The mechanism of hemiplegia rehabilitation is that achieving CNS and PNS's interaction, remodeling brain plasticity, constructing new combination relationship, and restoring autonomous motor control. The neural pathways of hemiplegia patients are fewer, which means the task-oriented repetitive functional training induces physiological and morphological plasticity after stroke through repetitive excitation or inhibition of neurotransmitters [Citation4,Citation5]. But this traditional training method have some disadvantages, on the one hand, it leaks the quantitative training plan evaluation, on the other hand, it needs involvement of a large number of medical professionals which will lead huge stress for family, society, and other aspects of hemiplegia patients [Citation6]. There still existing other routine rehabilitation therapy by means of intervention for limb dysfunction, for example, repetitive transcranial magnetic stimulation (rTMS) [Citation7], functional electrical stimulation (FES) [Citation8], etc. But these therapies hardly affect the limb function for patients recovering from a severe stroke [Citation9]. In recent years, exoskeleton robots used for limb motor function rehabilitation of hemiplegic patients have received more and more attention from all walks of life, because they developed rapidly and have begun to be commercialized [Citation10–12]. The exoskeleton rehabilitation robot reshapes the motor nervous system with the help of traditional physical human-machine interaction (HMI), which has been proven to effectively improve the rehabilitation effect of motor function in hemiplegic patients [Citation13]. Unfortunately, the mechanism of motor function recovery and nervous system remodeling in the rehabilitation process of exoskeleton robots is still unclear until now, and there is no effective theoretical basis to support the cognitive HMI mechanism of exoskeleton rehabilitation robots.
At present, research on the mechanism of motor nerves is mainly limited to the scope of a single nervous system, but the research on the information interaction and coupling relationship between between CNS and PNS is still blank. As shown in , the information interaction between the two can better reflect the synergistic relationship of the human body in the process of performing actions, reveal the deep neural mechanism related to motion more deeply, provide new technical means for motion decoding, and achieve more efficient human computer interactive control. At the same time, it also could provide innovative theories for rehabilitation technology, and establish a more reasonable new exoskeleton rehabilitation system that takes into account the information interaction pathways between the CNS and the PNS. Thereby, this paper would like to discuss a rehabilitation exoskeleton system centered on the information coupling mode of the CNS and PNS, establish a new rehabilitation theory that takes into account the internal network of a single nervous system and the information interaction mode between the two system networks, which will help realize stroke hemiplegia systematic comprehensive rehabilitation of patients from central to peripheral motor function.
2. EEG of movements
When the human body performs movements, the central nervous system of the brain firstly plans and “rehearses” the movements of the limbs, and then the central nervous system sends out the corresponding limb action control commands when it is ready, which is transmitted to the peripheral motor nerves of the limb through the spinal motor neurons, thereby triggering the relevant muscles to drive the bones to complete the corresponding movements. Therefore, the central nervous system plays an important role in controlling the execution of movements. For the mechanism of movement, we should first understand it from the level of the motor central nervous system. In limb motor tasks, researchers have found that the primary motor cortex is activated by various brain imaging techniques (e.g. fMRI, positron emission tomography, etc.) [Citation14]. Compared with other brain imaging techniques, electroencephalogram (EEG) reflects some of the electrical activity of the cerebral cortex and has the advantages of high temporal resolution and cheap price, so it is widely used to study neural activity during different movements.
2.1. Current status of EEG research on movements
Movements are mainly divided into stimulus-evoked movements induced by external stimuli and spontaneous movements caused by voluntary will. The results of the EEG study through the keypress response paradigm showed that there were significant differences between the two different movements in the movement preparation stage [Citation15]. In stimulus-evoked movements, relevant stimuli evoke components related to attention and response, while in spontaneous movements, other cortical regions are involved in the execution of spontaneous behaviors, which may be the source of intentional control by the central nervous system. Comparing patients with damaged parietal lobes with normal people, it can be found that patients with damaged parietal lobes cannot estimate the time of motor induction and preparation, which shows that the anterior auxiliary motor cortex and parietal lobe are very important for the expression of intention [Citation16]. The dorsolateral prefrontal cortex is involved in the generation and development of spontaneous movements, so when performing motor tasks, the dorsolateral prefrontal cortex is activated, resulting in increased cortical activity.
It is generally assumed that motor tasks cause activation in the contralateral motor cortex, but not in the ipsilateral motor cortex. Specifically, movement will produce activation of the contralateral sensorimotor cortex in the mu (8–12 Hz) and beta (13–30 Hz) frequency bands, which is the event-related desynchronization (ERD) phenomenon of mu and beta rhythms, and at the same time, the ipsilateral sensorimotor cortex will be inhibited, which is the event-related resynchronization (ERS) phenomenon of mu and beta rhythms [Citation17,Citation18]. However, an event-related potential study with a simple one-handed movement (voluntary movement, key-pressing movement) paradigm found that not only the contralateral motor cortex was activated, but also the ipsilateral motor cortex was involved, and the activation of the ipsilateral motor cortex related to motor tasks or performance [Citation19]. Numerous studies have shown that the complexity of motor tasks is related to the activation of the ipsilateral motor cortex, and the more difficult the motor task, the higher the activation level in the ipsilateral motor cortex, without significant changes in the contralateral motor cortex [Citation20]. In addition, a large number of EEG studies have found activation of motor readiness potentials in spontaneous movements [Citation21], but not in stimulus-evoked movements [Citation22].
2.2. EEG network
The execution of upper limb movements often requires the participation of multiple brain regions, and the mutual cooperation between these brain regions is the basis for effectively completing motor tasks. The interaction between brain regions can be quantitatively described by complex network analysis methods. When performing upper limb movement tasks, the EEG network mainly involves brain regions such as the premotor area, sensorimotor and subcortical structures (putamen, thalamus, and cerebellum) .
Different motor-related tasks have different EEG networks in brain regions, which can be demonstrated from . is a quantitative meta-analysis of motor imagery (MI), motor observation, and motor execution to assess differences in brain network activation for these three motor-related tasks [Citation23]. Among them, the brain regions recruited by MI were mainly two premotor cortices extending dorsally and ventrally across the supplementary motor area (SMA). Motor observation identified the network with the largest total volume of brain region activation across the three motor-related tasks, which essentially covered brain regions recruited by MI. Motor execution identified the network with the smallest total volume of brain region activation across the three motor-related tasks, recruiting small regions of the sensorimotor and premotor cortex. Thus, the three motor-related tasks recruited similar cortical networks but differed in the size of the main activated brain areas.
Figure 2. Differences in brain activation for three motor-related tasks (motor imagery, motor observation, and motor execution).

The different styles and intensities of movement corresponds the different feedback forms of brain networks. Through the research of active connection difference in movement relevant cortex networks, the experts have found that the contralateral motor cortex is strongly coupled to the premotor interval of the hemisphere, and movements show high laterality [Citation24,Citation25]. Investigating brain network differences in motor execution through brain-computer interfaces(BCI), the researchers found that the ability of the left hand to perform tasks is manifested in the brain network by the connectivity properties of bilateral motor areas, especially in the motor areas that conduct from left to right [Citation26,Citation27]. When performing bimanual task states, brain network connectivity can be modulated by motor task execution, and network activity in prefrontal cortex regions is positively correlated with motor performance [Citation28,Citation29]. There are other researches focus on motion execution network mechanism about rehabilitation of patients with motor impairment, which show that enhanced network functional connectivity between the ipsilateral cerebellum and motor cortex in task-state motor executive network features in stroke patients, and improved functional network integration is associated with recovery of motor executive ability [Citation30,Citation31].
However, these current studies mainly focus on the effect of upper limb movements on the function (static) and causal aspects of the EEG network. In turn, there are few related studies on the effect of EEG network on upper limb movements. For example, most researchers are more interested in the EEG activity before and after individual’s long-term movement strength training [Citation32–37], but rarely point out the positive effect of EEG network on the improvement of motor function. Therefore, it is necessary to use the EEG network as an indicator of the health status of the upper limb movement strength to study the information processing potential of the EEG network on the upper limb movement.
3. EMG of movements
The CNS of the brain transmits motor control information to peripheral motor nerves through spinal motor neurons and controls the musculoskeletal system to perform corresponding actions. The muscles of the limb are connected to the bones by tendon tissue, and when they contract, they can create a movement around the joints. Often a simple movement is also completed by co-activation of multiple sets of limb muscle groups. Some muscles contraction and traction to accomplish the intended purpose of the movement, while other muscles cooperate. Some muscles in the antagonistic position are moderately relaxed at this time and maintain a certain degree of tension, so as to make the movement smooth and accurate, which we could say that play a complementary role. Electromyography (EMG) is an important electrophysiological signal generated by muscle activation during exercise and contains a large amount of nerve information related to limb movement. Although the signal is not directly recorded from individual neuron, EMG signal contains information about the drive of the nerve to the muscle, i.e. the firing sequence of the motor neuron [Citation38]. EMG is a complex signal controlled by neuro system, which is depended on muscle anatomy and physiology. shows the process of acquiring EMG signal and the decomposition to achieve the Motor Unit Action Potentials (MUAPs), where the EMG signal is the MUAP sequence that shows the muscle response to neural stimulation [Citation39].
3.1. Current status of EMG research on movements
A major application of EMG is that using its features to identify motion intentions. Therefore, EMG is widely used to control auxiliary robots, rehabilitation equipment, or neural prosthetic systems [Citation40]. Based on EMG signal characteristics, there still a study identifying all rasping, flexion, and extension of the middle, ring, and little fingers as a whole, using the time-domain feature extraction method as the input of the neural network classifier, 12 types of finger flexion and extension movements can be decoded, and the accuracy rate can exceed 98% [Citation41]. After they conducted a pairwise classification study of 15 types of finger movements, the average accuracy of each pair of finger movements for all subjects was greater than 95% [Citation42]. Then they used the method of wavelet transform to reduce feature set dimension on raw EMG signal, used principal component analysis and sequential forward selection to filter features, the classification results showed that the recognition of hand movements was almost 100% successful [Citation43].
Except identifying the movement, EMG can reflect the activation and control mechanism of PNS on action execution. Especially after the human body is damaged due to motor function, EMG can effectively reflect these injury mechanisms and has a very good guiding role for the rehabilitation of motor function. EMG signals have been widely used in the fields of lesion localization, rehabilitation treatment, and prevention of human motor function-related diseases [Citation44]. However, there still a lot problems to be solved when using single channel EMG signal for rehabilitation of motor function-related diseases. On the one hand, the EMG signal is easily affected by factors such as muscle fatigue, EMG delay, and muscle movement crosstalk [Citation45], which brings great challenges to the development of control technology based on EMG signals in rehabilitation therapy. For example, the muscle disturbance caused by the involuntary movements (shaking) of a common disease such as essential tremor, and the elderly group with a higher proportion of patients with motor function-related diseases are more susceptible to muscle fatigue, etc. These situations will produce seriously polluted EMG signal data for the main treatment methods and control technologies for the rehabilitation of such diseases [Citation46]. On the other hand, some patients with movement disorders (such as severe paralysis) may not be able to generate enough EMG signals for pathological analysis and movement control [Citation47]. In addition, some movement disorders also have great limitations to draw conclusions from EMG signals, such as the analysis of idiopathic neck pain cases, the distinction of essential tremor types, etc. [Citation48]. As a consequence, the multi-channel acquisition has become a breakthrough in decoding the co-activation mechanism of peripheral nerves during patient movement because it alleviates the shortcomings that a single channel cannot collect enough EMG signals or is affected by severe interference.
3.2. EMG networks
It is generally recognized that many degrees of freedom of the musculoskeletal system do not allow for simple one-to-one communication during specific motor tasks, on the contrary, muscles are coupled and controlled together [Citation49]. Coupling between muscles (whether mechanical or neural) reduces the number of effective degrees of freedom and thus the number of potential modes of movement, which helps to reduce the motion control complexity [Citation50]. The electrophysiological researches have showed that a wide variety of natural movements can be produced only through the combination of several coherent sets of muscle activity patterns (or muscle synergy). Among them, some modes have existed from born and won't change during the development, others are learned. Spinal cord circuits are composed of upper and lower motor neurons that generate basic neurons which coordinate multiple muscles to drive movement in concert [Citation51]. These spinal cord circuits could code co-movement output to transfer the commands for multi-joint movements to spinal cord circuit patterns coordinated by multiple muscle groups [Citation52,Citation53]. Utilizing multiple sets of effective muscle activity to joint control of robotic equipment is very helpful to improve application technology in many fields [Citation54], like solving medical issues for people with disabilities [Citation55]. This is very important to implement this program in therapeutic recovery techniques.
Same as EEG analyzation, network theory, a totally new perspective for the modular organization of the musculoskeletal system, has aroused many attention in recent EMG researches. Bassett et al. a scholar of EEG network research first introduced the network concept into the analysis of the musculoskeletal system and constructed the corresponding whole-body structural network by mapping the source and terminal of the muscle [Citation56]. However, there are few studies on muscle functional connectivity networks. For this reason, it is very necessary to characterize the relationship between muscle blocks, and pay more attention to the network model research of different fine movements to describe the cooperative working mode between different muscle blocks.
4. EEG-EMG coupling of movements
In previous studies on motor mechanisms, researchers pay more attention to the information interaction patterns within the CNS and peripheral motor nerves, but less attention to the relationship between information transmission and feedback between the two nervous systems. According the development of collection technology, there has been an increasing number of researched reporting cortical muscle synchronization between the brain and muscles observed in the human limb motor system [Citation57].
4.1. Static coupling
The most important indicator of the phenomenon of cortical muscle synchronization is the coherence static coupling analysis between the brain and the muscle. The research shows that there is a discrete shift in coherence synchronization frequency from 25 Hz to 40 Hz, that is, the change from Beta rhythm to low Gamma rhythm is a weak to strong contraction [Citation58]. Corticomuscular coherence between EEG and tibialis anterior EMG in humans during walking has been shown to exist in a localized region near the motor cortex of the leg, where they have the greatest coupling in the region of electrode Cz. When in walking, it is meaningful that appearing this coherence between the cerebral cortex and muscle groups occurs in the 24–40 Hz frequency band [Citation59]. Further research also shows that cortical-muscle coherent synchronization consistency in the Beta range is directly related to specific motor parameters [Citation60]. Compared to the single EMG feature, the classification accuracy of the coherent coherence static coupling of the cerebral cortex EEG and muscle group EMG features can also be significantly improved (the accuracy of some research results is 9.83% and 6.06%, respectively) [Citation61]. The analysis of coherence based on fast Fourier transform (magnitude and phase, etc.) or autoregressive model has been increasingly used to study the relationship between EEG rhythms or EMG signals from muscle contractions and EEG or brain magnetic oscillations [Citation62,Citation63], these studies have revealed that the electrophysiological signals collected by electrode sensors have great coherence in the Beta frequency band. In this highly coherent frequency range, linear correlations can often be detected, allowing estimation of the transit time of EEG-EMG static coherence coupling, which is useful for diagnostic determination of cortical source localization such as myoclonus.
These existing studies mainly focus on the static coupling relationship between local brain regions and local muscle blocks. As two nervous systems closely related to movement, there should be a complex information coupling relationship between the two systems as a whole, but there is still a lack of reports on this research. Therefore, further measure the dynamic coupling relationship between the CNS and the PNS from a global perspective is necessary.
4.2. Dynamic coupling
Dynamic coupling is usually the direct or indirect effect of one nervous system on another at different time periods. For periodic movement, it is more intuitive to consider the temporal modulation of coherence within each movement cycle, and the EEG-EMG coherence can generally be quantified by the volume above the significance threshold on the frequency-time plane. As shown in , the link between EEG and EMG is time-varying rather than increasing in a static and constant manner during exercise execution [Citation64]. Corticomuscular coupling of a complete movement often requires continuous time course information, this is why understanding the more detailed time course is important to gain insight into the EEG-EMG coupling mechanism [Citation65].
Figure 4. Illustration of the volume of EEG-EMG coherence above the threshold of significance on the frequency-time plane.
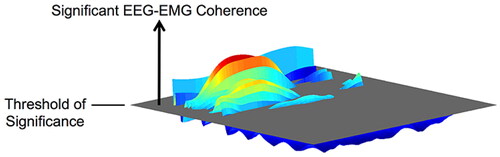
An important indicator for examining whether dynamic regulation at the cortical-muscle level reflects cortical-muscle mechanisms is also cortical-muscle coherence consistency. By segmenting the EEG-EMG static coupling consistency method into time series, the EEG-EMG dynamic coupling characteristics can be obtained. Neuroimaging researched have shown that in the process of tapping the fingers of the limbs with the rhythm, even if there is no obvious movement, the motor areas of the brain and the muscle groups corresponding to the movement are still activated with the rhythm of the movement [Citation66]. Moreover, dynamic amplitude modulation of EEG neural oscillations underpinning sensorimotor Beta rhythm mechanisms consistent with motor EMG rhythms [Citation67]. Previous researches have shown, correlation between EMG signals and EEG signals in the brain’s primary motor cortex (M1) during isometric limb contractions in Beta rhythms is a hallmark of motor control [Citation68]. Conditioned by singular audio stimulation, this cortical-muscle coherence increased within a few hundred milliseconds after stimulation, suggesting that the motor system is continuously and automatically activated in response to changes in the surrounding environment [Citation69]. But this subjective time series division cannot reflect the changes in the subtle time precision of EEG-EMG coupling information during complex limb tasks all the time.
Therefore, there existing a need to construct methods to interactively analyze the dynamic information transfer of a series of fine time divisions. Models can be built to describe how EEG and EMG interact based on neurophysiology and sound inferences. The dynamic causality model (DCM) is based on the neural cluster model and known information, making multiple assumptions about possible network connections, and then using Bayesian to filter the hypothesis connections and finally select the best model [Citation70]. DCM has been successful used in EEG-related causal analysis and applications, but there are also defects in that there are too many network nodes to solve effectively [Citation71]. Granger causality (GC) based on data-driven technology uses past information to predict the outcome, then the first signal can be used as the source of the second signal [Citation72]. With the development of GC method, GC has been applied from the time domain to the frequency domain, from binary to multivariate. The Directed Transfer Function (DTF) and Partial Directed Coherence (PDC) developed from the GC method can be applied to research for the coupling of EEG and EMG. Recent researches have further developed dynamic network analysis methods with transient significance, such as Adaptive Directed Transfer Function (ADTF) and time-varying multivariate analysis techniques, etc. [Citation73], which are critical to the relationship between the CNS and PNS. The coupling between them has potential application value.
In order to develop the coupling information interaction relationship of EEG-EMG multimodal signals, it is necessary to study the dynamic causal relationship between the two signals at different stages of action, analyze the dynamic information flow between the two systems as a whole, reveal the top-down time-varying control between the CNS and the PNS, as well as the bottom-up real-time feedback mechanism of the PNS to the CNS.
5. Human-machine interaction research on movement
It is usually to improves blood flow and metabolism in areas connected to nerve fibers for patients with stroke and loss of motor function to recover their motor function. The above progress will construct a new neural network to replace the old network which was impaired or dysfunctional, and make the neural system have a long-term plastic change [Citation74]. The relevant neural rehabilitation studies have shown that exercise rehabilitation training can remodel the injured CNS, such as neuronal circuit remodeling, enhancement of synaptic plasticity, etc. [Citation75]. Above them, the neural rehabilitation guided by HMI rehabilitation training is especially critical for the treatment of limb movement injuries [Citation76]. In summary, the research about HM's closed-loop regulation to mechanisms of functional recovery between remodeling the central nervous system and peripheral nervous system is urgent.
5.1. Rehabilitation theory of human-machine interaction
HMI means an interactive way that human and machine complete a definite task about information exchange. Especially, the exoskeleton robot combined with BCI is considered to be a new HMI technology means for effective rehabilitation of stroke hemiplegia patients. The basic principle is using BCI to assess the state of the somatosensory cortex from the cerebral cortex and respond patients' intention to move autonomously, which aims to be achieved by robotically performing electrical muscle stimulation or motor-assisted voluntary movement, making it possible to reproduce the loops of the somatosensory and motor systems, i.e. the control and feedback of movement, and to expect remodeling of the central nervous system [Citation77]. In 2008, Buch et al. used magnetoencephalography to measure sensorimotor rhythm (SMR) and established a device system for driving finger extension based on the measurement results [Citation78]. The patients whose SMR have a significant difference could continue using this BCI, which shows that plasticity of brain activity can be guided by motor assistance with BCI. Pfurtscheller et al. also used BCI to conduct SMR training for hemiplegic stroke patients and developed a system that can change the movements displayed on the computer screen according to the change in SMR [Citation79]. Their days of training have clearly shown that reduced SMR occurred in movement on the paralyzed side, and EEG classification accuracy at rest and movement could reach 70–80%, which also has shown the possibility of BCI in guiding neurofeedback plasticity of brain activity. BCI can effectively improve limb function according to the research by Daly et al. [Citation77], and relevant researches also prove that combining BCI with conventional physical therapy could effectively improve limb function for hemiplegia patients [Citation80]. In addition, a BCI system for assisting finger extension movements constructed by Ushiba et al. [Citation81], which based on the amount of SMR variation induced by motor intention and SMR reduction in motor intention in chronic stroke hemiplegia patients. Donati et al. integrated BCI, exoskeleton robot, and virtual reality to train hemiplegic patients for two years. The results showed that the system can not only allow patients to walk and memory movement through BCI, but also create multiple activities through exoskeleton robot and virtual reality, enabling hemiplegic patients to obtain a more realistic sensation during training, and enhanced the activation of the main motor area and somatosensory area of the brain of hemiplegic patients, thereby expanding the range of brain activity [Citation82].
The current rehabilitation systems provide feedback control, evaluation indicators from local indicators within an individual neural system such as SMR, muscle tone, etc. However, there is a lack of research on the plastic repair of the information coupling mode within and between the two nervous systems, between the patient and the exoskeleton robot during the rehabilitation process. It is truly a need to have more deeper research on motor neural decoding, signal source localization, and other neural mechanisms when we focus on validating the impact of BCI exoskeletons on rehabilitation.
5.2. Closed-loop regulation of human-machine interaction
It is a two-way communication progress in HMI. Rely on various biological signals such as the wearer’s EEG signal and EMG signal to decode and express the wearer’s advanced cognitive behavioral patterns, and combine the physical signals of the human-machine system such as posture, interaction force, pressure, etc., the HMI technology could integrate and analyze the multimodal signals to establish the wearer’s intention estimation model and then combine the feedback information to the wearer to realize the closed-loop feedback path of human-machine two-way interaction. And establish a wearer-exoskeleton hybrid intelligent model to realize the cognition, cooperation, and movement of the HMI system.
There are two mainly interaction ways in exoskeleton's interaction, physical interaction, and biological information interaction. It is mainly through buttons, touch screens, and other devices that hemiplegic patients can directly input movement intentions in a switch mode, which is a physical interaction. This interaction method is relatively simple, but there is no interaction between the patient and the exoskeleton in the process from the triggering of the signal to the triggering of the next signal. Therefore, patients could not perform motion control to the exoskeleton during exercise. To achieve the real-time interaction during exercise between patients and the exoskeleton, the force sensors which can continuously obtain changes in the interaction force between the patient and the exoskeleton seems a viable option [Citation83]. Biological information interaction mainly uses EEG signals and EMG signals for motion intention recognition. Because the biological information is the signal that hemiplegia patients spontaneously develop during exercise, compared with physical interaction, this interaction is simple and natural, and the motor and perception functions of the brain can be strengthened through BCI systems such as MI [Citation84]. EMG signal interaction can be refined into trigger interaction and continuous interaction. Trigger interaction means that when the EMG signal reaches the threshold, the movement intention of the hemiplegic patient is output to the exoskeleton [Citation85]. Same as physical interaction, this type interaction could not work during exercise. Thence, using this interactive way to train is closer to the passive mode in rehabilitation training. Another continuous interaction method is extracting features for each time node in the preprocessed EMG signal. While the exoskeleton identifying this signal feature, it could turn motion intent into actual motion which accomplish the continuous extraction of patient motion intent changes [Citation86]. Therefore, this interaction could increase the information interaction in the process of exercise, so that patients with hemiplegia can carry out active mode rehabilitation training.
The mainstream BCI systems for exoskeleton are steady-state visual evoked potential (SSVEP) and MI. For these two closed-loop regulation techniques, the accuracy rate of SSVEP is generally higher, and the role of MI in promoting plastic changes in brain function is greater. SSVEP are elicited by the attentional focus on a steady pattern of a visual stimulus [Citation87]. In the SSVEP paradigm, a stimulus flickering at constant frequency is generated by LEDs or by alternating images on a computer screen. Sylvain et al. set up an SSVEP-based BCI for controlling a robotic arm exoskeleton device on a motorized wheelchair [Citation88]. SSVEP stimulation consists of 3 groups of LEDs flashing at different frequencies. They directly classified the EEG signals obtained by SSVEP based on the canonical correlation analysis (CCA) method [Citation89] and achieved good classification performance. Juan et al. used slow rhythms phase analysis of EEG to study brain activity as a function of motor state during a motor imagery-based BCI session [Citation90]. They found that cortical slow rhythms are synchronized during the active phase at the onset of motor imagery and the relaxed phase at the end of motor imagery [Citation91] and that this synchronicity, quantified by phase coherence [Citation92], is modulated by sensory feedback (which reduces it) and gradually increases with daily training. These findings suggest that motor imagery contributes to the remodeling of the cortex to control motor function. Based on MI-BCI exoskeletons combined with virtual reality, Donati et al. showed the two-way neural interaction of “human to machine” and “machine to human”, and verified the influence of two-way neural interaction on brain function in [Citation82]. Specifically, they recruited American Spinal Injury Association (ASIA) patients with grade B injuries for rehabilitation training. All patients wearing this exoskeleton rehabilitation robot scored the proprioception of the lower limb joints (0: not present, 1: present). Patients were asked to describe stimuli while performing lower extremity activities: which side of the body (right or left), which joint (hip, knee, ankle, toe), and which movement (flexion, extension, adduction, abduction). From initiation to 4 months of training, none of the patients were able to describe the mobilization of their lower extremities, nor could they distinguish which side of their body was stimulated, with all scores of 0. From month 4 to month 7, patients showed a better perception of this type of stimulation, with a partial scores of 1. After 7 months of training, the patient had fully recovered and described lower extremity activities, with all scores of 1.
Figure 5. Proposed components for the rehabilitation mechanism: direct brain control of virtual or robotic legs, the continuous stream of tactile stimulation representing the missing haptic feedback from the legs, and robotic actuators to train patients to walk. Cortical and spinal plasticity are hypothesized to change and to modulate neurological circuits in the preserved area around the lesion through motor (red) and sensory (blue) connections.
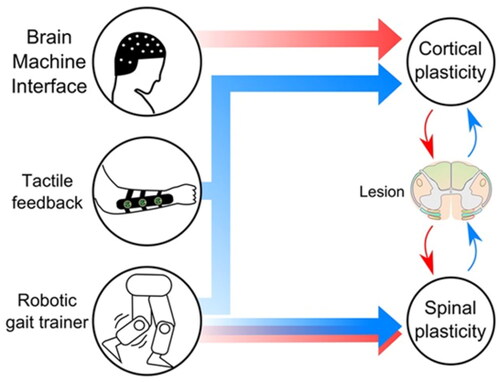
Through the control feedback relationship between different movements and the exoskeleton, it is feasible to establish a synchronous real-time online two-way closed-loop interactive system. This synchronization system is based on the high-accuracy recognition of exoskeletons, and also has high requirements for the real-time transfer efficiency of human-exoskeletons. This can also establish an asynchronous offline two-way closed-loop interactive system, which considers the delay time and latency between the human and the exoskeleton, and give compensatory feedback after full control intention to achieve a closed-loop regulation system that meets expectations. However, what most should be established is the EEG-EMG-exoskeleton multi-source information fusion system, which plays a multimodal regulation role of the inner loop and the outer loop double closed loop on HMI external level. In this way, the information interaction coupling mode at the overall level may provide more effective and objective feedback and evaluation indicators for rehabilitation, which is the base of new possible exoskeleton rehabilitation training robotic system for stroke patients.
Disclosure statement
No potential conflict of interest was reported by the author(s).
Additional information
Funding
References
- De Groote F, Falisse A. Perspective on musculoskeletal modelling and predictive simulations of human movement to assess the neuromechanics of gait. Proceedings of the royal society. Proc Biol Sci. 2021;288(1946):20202432.
- Katan M, Luft A. Global burden of stroke. Semin Neurol. 2018;38(2):208–211.
- Mozaffarian D, Benjamin EJ, Go AS, et al. Heart disease and stroke statistics—2015 update: a report from the American Heart Association. Circulation. 2015;131(4):e29–e322.
- Nudo RJ, Wise BM, SiFuentes F, et al. Neural substrates for the effects of rehabilitative training on motor recovery after ischemic infarct. Science. 1996;272(5269):1791–1794.
- Hanlon RE. Motor learning following unilateral stroke. Arch Phys Med Rehabil. 1996;77(8):811–815.
- Li S, Wu K, Meng Q, et al. Research progress on intelligent assessment system for upper limb function of stroke patients. Sheng Wu Yi Xue Gong Cheng Xue Za Zhi. 2022;39(3):620–626.
- Kirton A, Chen R, Friefeld S, et al. Contralesional repetitive transcranial magnetic stimulation for chronic hemiparesis in subcortical paediatric stroke: a randomised trial. Lancet Neurol. 2008;7(6):507–513.
- Eraifej J, Clark W, France B, et al. Effectiveness of upper limb functional electrical stimulation after stroke for the improvement of activities of daily living and motor function: a systematic review and meta-analysis. Syst Rev. 2017;6(1):1–21.
- VAdS J, MdS S, NMdS R, et al. Combining proprioceptive neuromuscular facilitation and virtual reality for improving sensorimotor function in stroke survivors: a randomized clinical trial. J Cent Nerv Syst Dis. 2019;11:1179573519863826.
- He C, Xiong C-H, Chen Z-J, et al. Preliminary assessment of a postural synergy-based exoskeleton for post-stroke upper limb rehabilitation. IEEE Trans Neural Syst Rehabil Eng. 2021;29:1795–1805.
- Wu Q, Wang X, Chen B, et al. Development of a minimal-intervention-based admittance control strategy for upper extremity rehabilitation exoskeleton. IEEE Trans Syst Man Cybern Syst. 2017;48(6):1005–1016.
- Bao G, Pan L, Fang H, et al. Academic review and perspectives on robotic exoskeletons. IEEE Trans Neural Syst Rehabil Eng. 2019;27(11):2294–2304.
- Iwamoto Y, Imura T, Suzukawa T, et al. Combination of exoskeletal upper limb robot and occupational therapy improve activities of daily living function in acute stroke patients. J Stroke Cerebrovasc Dis. 2019;28(7):2018–2025.
- Wijk BCM, Van Beek PJ, Daffertshofer A, et al. Differential modulations of ipsilateral and contralateral beta (de)synchronization during unimanual force production. Eur J Neurosci. 2012;36(1):2088–2097.
- Waszak F, Wascher E, Keller P, et al. Intention-based and stimulus-based mechanisms in action selection. Exp Brain Res. 2005;162(3):346–356.
- Sirigu A, Daprati ES, Giraux P, et al. Altered awareness of voluntary action after damage to the parietal cortex. Nat Neurosci. 2004;7(1):80–84.
- Gourab K, Schmit BD. Changes in movement-related β-band EEG signals in human spinal cord injury. Clin Neurophysiol. 2010;121(12):2017–2023.
- Bechtold L, Ghio M, Lange J, et al. Event-related desynchronization of mu and beta oscillations during the processing of novel tool names. Brain Lang. 2018;177:44–55.
- Bai O, Mari Z, Vorbach S, et al. Asymmetric spatiotemporal patterns of event-related desynchronization preceding voluntary sequential finger movements: a high-resolution EEG study. Clin Neurophysiol. 2005;116(5):1213–1221.
- Burle B, van den Wildenberg WPM, Spieser L, et al. Preventing (impulsive) errors: electrophysiological evidence for online inhibitory control over incorrect responses. Psychophysiology. 2016;53(7):1008–1019.
- Zhang L, Zhang R, Yao D, et al. Differences in intersubject early readiness potentials between voluntary and instructed actions. Front Psychol. 2020;11:529821.
- Zhang L, Ren H, Zhang R, et al. Time-estimation process could cause the disappearence of readiness potential. Cogn Neurodyn. 2022;16(5):1003–1011.
- Hardwick RM, Caspers S, Eickhoff SB, et al. Neural correlates of action: comparing meta-analyses of imagery, observation, and execution. Neurosci Biobehav Rev. 2018;94:31–44.
- Volz LJ, Eickhoff SB, Pool EM, et al. Differential modulation of motor network connectivity during movements of the upper and lower limbs. Neuroimage. 2015;119:44–53.
- Calvert GHM, Carson RG. Neural mechanisms mediating cross education: with additional considerations for the ageing brain. Neurosci Biobehav Rev. 2022;132:260–288.
- Bauer R, Fels M, Vukelic M, et al. Bridging the gap between motor imagery and motor execution with a brain-robot interface. Neuroimage. 2015;108:319–327.
- Khademi F, Naros G, Nicksirat A, et al. Rewiring cortico-muscular control in the healthy and poststroke human brain with proprioceptive β-Band neurofeedback. J Neurosci. 2022;42(36):6861–6877.
- Monteiro TSK, Adab HZ. Age-related differences in network flexibility and segregation at rest and during motor performance. Neuroimage. 2019;194:93–104.
- Jiang R, Scheinost D, Zuo N, et al. A neuroimaging signature of cognitive aging from whole‐brain functional connectivity. Adv Sci. 2022;9(24):2201621.
- Cheng L, Wu Z, Sun J, et al. Reorganization of motor execution networks during Sub-Acute phase after stroke. IEEE Trans Neural Syst Rehabil Eng. 2015;23(4):713–723.
- Wang Y, Luo J, Guo Y, et al. Changes in EEG brain connectivity caused by short-term bci neurofeedback-rehabilitation training: a case study. Front Hum Neurosci. 2021;15:345.
- Spring JN, Tomescu MI, Barral J. A single-bout of endurance exercise modulates EEG microstates temporal features. Brain Topogr. 2017;30(4):461–472.
- Zhang R, Yao D, Valdés-Sosa PA, et al. Efficient resting-state EEG network facilitates motor imagery performance. J Neural Eng. 2015;12(6):066024.
- To XV, Nasrallah FA. A roadmap of brain recovery in a mouse model of concussion: insights from neuroimaging. Acta Neuropathol Commun. 2021;9(1):1–20.
- Cottone C, Porcaro C, Cancelli A, et al. Neuronal electrical ongoing activity as a signature of cortical areas. Brain Struct Funct. 2017;222(5):2115–2126.
- Nijhuis P, Keller PE, Nozaradan S, et al. Dynamic modulation of cortico-muscular coupling during real and imagined sensorimotor synchronisation. Neuroimage. 2021;238:118209.
- Bernardi NF, Van Vugt FT, Valle-Mena RR, et al. Error-related persistence of motor activity in resting-state networks. J Cogn Neurosci. 2018;30(12):1883–1901.
- Farina D, Jiang N, Rehbaum H, et al. The extraction of neural information from the surface EMG for the control of upper-limb prostheses: emerging avenues and challenges. IEEE Trans Neural Syst Rehabil Eng. 2014;22(4):797–809.
- De Luca CJ, Adam A, Wotiz R, et al. Decomposition of surface EMG signals. J Neurophysiol. 2006;96(3):1646–1657.
- Phinyomark A, Phukpattaranont P, Limsakul C. A review of control methods for electric power wheelchairs based on electromyography signals with special emphasis on pattern recognition. IETE Tech Rev. 2011;28(4):316–326.
- Tenore F, Ramos A, Fahmy A, et al. editors. Towards the control of individual fingers of a prosthetic hand using surface EMG signals. International Conference of the IEEE Engineering in Medicine & Biology Society; 2007 Aug 22–26; Lyon, France.
- Khushaba RN, Kodagoda S, editors. Electromyogram (EMG) feature reduction using mutual components analysis for multifunction prosthetic fingers control. International Conference on Control Automation Robotics & Vision; 2012 Dec 05–07; Guangzhou, China.
- Sahin U, Sahin F. editors. Pattern recognition with surface EMG signal based wavelet transformation. IEEE International Conference on Systems; 2012 Oct 14–17; Seoul, Korea (South).
- Andreas S, Markus Due J, Lars Louis A, et al. EMG evaluation of hip adduction exercises for soccer players: implications for exercise selection in prevention and treatment of groin injuries. Br J Sports Med. 2014;48(14):1108–1114.
- Danna-Dos SA, Poston B, Jesunathadas M, et al. Influence of fatigue on hand muscle coordination and EMG-EMG coherence during three-digit grasping. J Neurophysiol. 2010;104(6):3576–3587.
- Conchola EC, Thompson BJ, Smith DB. Effects of neuromuscular fatigue on the electromechanical delay of the leg extensors and flexors in young and old men. Eur J Appl Physiol. 2015;52(5):844–851.
- Lalitharatne TDT, Hayashi Y. Towards hybrid EEG-EMG-based control approaches to be used in bio-robotics applications: current status, challenges and future directions. Paladyn, J Behav Robot. 2013;4(2):147–154.
- Castelein B, Cools A, Bostyn E, et al. Analysis of scapular muscle EMG activity in patients with idiopathic neck pain: a systematic review. J Electromyogr Kinesiol. 2015;25(2):371–386.
- Bernstein N. The coordination and regulation of movements. Oxford: Pergamon; 1967.
- Turvey MT. Coordination. Am Psychol. 1990;45:938–953.
- Takei T, Confais J, Tomatsu S, et al. Neural basis for hand muscle synergies in the primate spinal cord. Proc Natl Acad Sci USA. 2017;114(32):8643–8648.
- Levine AJ, Hinckley CA, Hilde KL, et al. Identification of a cellular node for motor control pathways. Nat Neurosci. 2014;17(4):586–593.
- Pierrot-Deseilligny E, Burke D. The circuitry of the human spinal cord: its role in motor control and movement disorders. Cambridge (UK): Cambridge University Press; 2005.
- Jair L, Loaiza NA. Evolución Y tendencias EN EL desarrollo DE prótesis DE mano. DYNA. 2011;78(169):191–200.
- Song X, Van De Ven SS, Liu L, et al. Activities of daily living-based rehabilitation system for arm and hand motor function retraining after stroke. IEEE Trans Neural Syst Rehabil Eng. 2022;30:621–631.
- Murphy AC, Muldoon SF, Baker D, et al. Structure, function, and control of the musculoskeletal network. PLOS Biol. 2018;16(1):e2002811.
- Gross JT, Salenius S, Hari R, et al. Corticomuscular synchronization during isometric muscle contraction in humans as revealed by magnetoencephalography. J Physiol. 2000;527(3):623–631.
- Brown P. Cortical drives to human muscle: the piper and related rhythms. Prog Neurobiol. 2000;60(1):97–108.
- Petersen TH, Willerslev-Olsen M, Conway BA, et al. The motor cortex drives the muscles during walking in human subjects. J Physiol. 2012;590(10):2443–2452.
- Kilner JM, Baker SN, Salenius S, et al. Human cortical muscle coherence is directly related to specific motor parameters. J Neurosci. 2000;20(23):8838.
- Li J, Qi H, Gu Y, et al. editors. Towards single trial decoding of cortical-muscular activities. IEEE International Conference on Advanced Intelligent Mechatronics; 2017 July 03–07; Munich, Germany.
- Teija S, Forss N, Salenius S, et al. Oscillatory cortical drive to isometrically contracting muscle in Unverricht-Lundborg type progressive myoclonus epilepsy (ULD). Clin Neurophysiol. 2002;113(12):1973–1979.
- Panzica F, Canafoglia L, Franceschetti S, et al. Movement-activated myoclonus in genetically defined progressive myoclonic epilepsies: EEG–EMG relationship estimated using autoregressive models. Clin Neurophysiol. 2003;114(6):1041–1052.
- Yoshida T, Masani K, Zabjek K, et al. Dynamic increase in corticomuscular coherence during bilateral, cyclical ankle movements. Front Hum Neurosci. 2017;11:155.
- Yang Q, Siemionow V, Yao W, et al. Single-Trial EEG-EMG coherence analysis reveals muscle Fatigue-Related progressive alterations in corticomuscular coupling. IEEE Trans Neural Syst Rehab Eng. 2010;18(2):97–106.
- Chen JL, Penhune VB, Zatorre RJ. Listening to musical rhythms recruits motor regions of the brain. Cereb Cortex. 2008;18(12):2844–2854.
- Fujioka T, Ross B, Trainor LJ. Beta-band oscillations represent auditory beat and its metrical hierarchy in perception and imagery. J Neurosci. 2015;35(45):15187–15198.
- Bourguignon M, Piitulainen H, Smeds E, et al. MEG insight into the spectral dynamics underlying steady isometric muscle contraction. J Neurosci. 2017;37(43):10421–10437.
- Piitulainen H, Bourguignon M, Smeds E, et al. Phasic stabilization of motor output after auditory and visual distractors. Hum Brain Mapp. 2015;36(12):5168–5182.
- Friston KJ, Harrison L, Penny W. Dynamic causal modelling. Neuroimage. 2003;19(4):1273–1302.
- Moran RJ, Stephan KE, Seidenbecher T, et al. Dynamic causal models of steady-state responses. Neuroimage. 2009;44(3):796–811.
- Friston KJ, Moran R, Seth AK. Analysing connectivity with granger causality and dynamic causal modelling. Curr Opin Neurobiol. 2013;23(2):172–178.
- Li FL, Chen B, Li H, et al. The time-varying networks in P300: a task-evoked EEG study. IEEE Trans Neural Syst Rehabil Eng. 2016;24(7):725–733.
- Citri A, Malenka RC. Synaptic plasticity: multiple forms, functions, and mechanisms. Neuropsychopharmacology. 2008;33(1):18–41.
- Dimyan MA, Cohen LG. Neuroplasticity in the context of motor rehabilitation after stroke. Nat Rev Neurol. 2011;7(2):76–85.
- Biernaskie J, Corbett D. Enriched rehabilitative training promotes improved forelimb motor function and enhanced dendritic growth after focal ischemic injury. J Neurosci. 2001;21(14):5272–5280.
- Daly JJ, Wolpaw JR. Brain-computer interfaces in neurological rehabilitation. Lancet Neurol. 2008;7(11):1032–1043.
- Buch E, Weber C, Cohen LG, et al. Think to move: a neuromagnetic brain-computer interface (BCI) system for chronic stroke. Stroke. 2008;39(3):910–917.
- Pfurtscheller G, Mllerputz GR, Scherer R, et al. Rehabilitation with brain-computer interface systems. Computer. 2008;41(10):58–65.
- Broetz D, Braun C, Weber C, et al. Combination of brain-computer interface training and goal-directed physical therapy in chronic stroke: a case report. Neurorehabil Neural Repair. 2010;24(7):674–679.
- Ushiba J, Morishita A, Maeda T, editors. A task-oriented brain-computer interface rehabilitation system for patients with stroke hemiplegia. International Conference on Wireless Communications; 2014 May 11–14; Aalborg, Denmark.
- Donati ARC, Shokur S, Morya E, et al. Long-Term training with a brain-machine interface-Based gait protocol induces partial neurological recovery in paraplegic patients. Sci Rep. 2016;6:30383.
- Simon AM, Gillespie RB, Ferris DP. Symmetry-based resistance as a novel means of lower limb rehabilitation. J Biomech. 2007;40(6):1286–1292.
- Ono T, Tomita Y, Inose M, et al. Multimodal sensory feedback associated with motor attempts alters BOLD responses to paralyzed hand movement in chronic stroke patients. Brain Topography. 2015;28(2):340–351.
- Kiguchi K, Rahman MH, Sasaki M, et al. Development of a 3DOF mobile exoskeleton robot for human upper-limb motion assist. Rob Auton Syst. 2008;56(8):678–691.
- Lenzi T, Rossi SMM, De Vitiello N, et al. Intention-based EMG control for powered exoskeletons. IEEE Trans Biomed Eng. 2012;59(8):2180–2190.
- Chen Y, Yang C, Ye X, et al. Implementing a calibration-free SSVEP-based BCI system with 160 targets. J Neural Eng. 2021;18(4):046094.
- Chevallier S, Kalunga EK, Barthélemy Q, et al. Review of riemannian distances and divergences, applied to SSVEP-based BCI. Neuroinformatics. 2021;19(1):93–106.
- Zhuang X, Yang Z, Cordes D. A technical review of canonical correlation analysis for neuroscience applications. Hum Brain Mapp. 2020;41(13):3807–3833.
- Barios JA, Ezquerro S, Bertomeu-Motos A, et al. Synchronization of slow cortical rhythms during motor imagery-based brain–machine interface control. Int J Neural Syst. 2019;29(05):1850045.
- Mushtaq M, Marshall L, Bazhenov M, et al. Differential thalamocortical interactions in slow and fast spindle generation: a computational model. PLOS One. 2022;17(12):e0277772.
- López ME, Pusil S, Pereda E, et al. Dynamic low frequency EEG phase synchronization patterns during proactive control of task switching. Neuroimage. 2019;186:70–82.