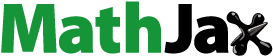
Abstract
Background
The motor imagery (MI) paradigm is widely used in active brain-computer interfaces (BCIs), but its effectiveness is hindered by accuracy limitations and individual variability. Tactile stimulation has been proposed as a potential method to enhance MI performance; however, integrating such stimulation in real-time MI tasks remains challenging due to the spontaneous nature of MI.
Objective
This study aimed to investigate the real-time effects of bilateral vibration tactile stimulation on the performance of left and right hand MI paradigms using a controlled trial with healthy subjects.
Methods
Fourteen healthy subjects participated in MI tasks under two conditions: without vibration stimulation and with bilateral vibration stimulation. Brain activation patterns and decoding accuracy of MI-BCI were compared and analyzed between these two conditions.
Results
The experimental findings demonstrated that vibration stimulation effectively activated the sensorimotor cortex and enhanced the event-related desynchronization (ERD) phenomenon induced by MI. Although bilateral vibration stimulation did not directly improve decoding accuracy of left and right hand MI, it notably peaked approximately 2 seconds post-stimulation, resulting in an approximate 7% increase in accuracy compared to baseline MI.
Conclusion
This study systematically explored the phased impact of continuous vibration stimulation on MI performance. The findings discuss potential optimization strategies for integrating tactile feedback into MI-based BCIs, thus laying groundwork for future research in tactile-enhanced MI brain-controlled systems.
1. Introduction
The motor imagery (MI) paradigm is a classic active brain-computer interface (BCI) paradigm [Citation1]. It allows users to induce mu and beta rhythms of event-related desynchronization (ERD) and synchronization (ERS) phenomena in the primary motor area of the brain through imagining limb movements without external stimulation and muscle action output [Citation2, Citation3]. Therefore, combined with the non-invasive characteristics of EEG signals, makes the MI-BCI highly promising for applications in fields such as neural rehabilitation and robot control [Citation4, Citation5]. As an active BCI that users initiate spontaneously, most individuals can master the use of MI-BCI after training. However, approximately 15–30% of users fail to utilize MI-BCI effectively, a condition referred to as “BCI illiteracy” [Citation6, Citation7]. These individuals often struggle to induce smooth ERD activation in the sensorimotor brain region, resulting in an inability to generate classifiable imagination patterns. Furthermore, the MI paradigm necessitates subjects to undergo extensive training to achieve proficient control. The imagery patterns and activation conditions vary across individuals, greatly limiting the practical application of MI-BCI [Citation8].
To address these challenges, scholars have been working on improving the decoding rate and universality of the MI paradigm. The specific methods include improving signal processing algorithms to filter out noise and extract more useful information [Citation9]; proposing new feature extraction, channel selection, and classification algorithms to improve decoding rates [Citation10]; integrating multiple signals (including EMG, fNIRS, etc.) or multiple sensory stimuli to design hybrid brain-computer interfaces to assist in improving the overall performance of the MI paradigm [Citation11–14]. Among them, the method of adding sensory stimulation has received widespread attention in recent years. For instance, Allison et al. proposed a mixed BCI that combines SSVEP with MI to enhance BCI versatility [Citation15].
The combination of tactile stimulation and MI paradigm has shown promising early results. Simple tactile stimulation can activate the user’s sensory cortex, and users can modulate this activation by focusing on tactile stimulation on different limbs. Tactile stimulation techniques include electrical stimulation, vibration stimulation, and kinesthetic stimulation, among others. Gomez et al. [Citation16] used a robotic arm to move the imagined arm and closed the sensory-motor loop by providing kinesthetic feedback, which boosted the decoding accuracy of MI. Yi et al. [Citation17] combined electrical stimulation with MI BCI. Using the feature of SSSEP induced by electrical stimulation, their classification accuracy rate increased by about 14% compared to MI. In contrast, vibration stimulation, as a convenient, inexpensive, and highly acceptable tactile stimulation method, may have greater potential in combination with the MI paradigm. Yao et al. [Citation18] combined the MI paradigm with the selective perception paradigm of bilateral vibration stimulation, which effectively reduced the BCI illiteracy rate. Shu et al. [Citation19] and Ahn et al. [Citation20] also found that vibration stimulation can improve the overall performance of MI, especially in enhancing the activation of the motor-sensory cortex and improving decoding accuracy. Zhang et al. used real-time prediction of brain phase information to apply tactile stimulation in a closed-loop manner, which improved the overall performance of hand MI [Citation21]. However, research on combining vibration tactile stimulation with the MI paradigm is still in its early stages, with many studies yielding preliminary results that require deeper exploration of specific issues such as the optimal combination of stimulation methods and parameters for optimal MI performance.
Based on these foundations, this study delves into the impact of bilateral vibration tactile stimulation on MI tasks involving both the left and right hands. Prior research has shown that vibration stimulation applied to the same side as imagined movements can enhance activation of the contralateral sensory-motor cortex and improve MI performance. However, due to the spontaneous nature of user imagination, it is not possible to apply stimulation only to the imagery side. At the same time, there is no existing research that has found that bilateral tactile stimulation can enhance the decoding rate of MI paradigms, which may be due to different application times or sensory delays.
The main contributions of this article include: To gain a deeper understanding of the effects of bilateral vibration tactile stimulation on left and right hand motor imagery tasks, we designed a controlled experiment incorporating two conditions: simple motor imagery without vibration stimulation (PMI) and motor imagery with continuous vibration stimulation (VT). By extending the duration of the motor imagery execution, we aimed to investigate the influence of vibration stimulation on long-term imagery. We used event-related spectral perturbation (ERSP), phase locking value (PLV), and other methods to compare the oscillatory patterns, time-frequency characteristics, and functional connectivity levels of EEG under different tasks. Furthermore, we discussed temporal changes in brain states. By applying the common spatial pattern (CSP) algorithm for feature extraction and linear discriminant analysis (LDA) for classification, we determined changes in MI classification rates across various time periods.
2. Method
2.1. Subjects
Fourteen healthy subjects (10 males and 4 females, aged 24–30 years) participated in the experiment, they were all right-handed. All participants were naive to EEG experiments and were free from known neurophysiological and musculoskeletal disorders. They exhibited normal tactile sensitivity and normal or corrected to normal vision. Prior to the experiment, each subject was thoroughly briefed on the experimental protocol and signed the informed consent form. To prevent psychological bias during the MI tasks, the subjects were unaware of the timing and purpose of the vibration stimulation. This study strictly adhered to the Helsinki Declaration and received ethical approval from the Ethics Committee of the First Affiliated Hospital of Nanjing Medical University (2020-SR-362).
2.2. EEG recording and tactile stimulation
We used a 64-channel active electrode system (ActiCAP Systems, BrainProducts GmbH, Germany) to acquire continuous EEG signals. depicts the electrode arrangement, where the red electrodes represent the active channels. All the channels were referenced to channel FCz (blue), and channel FPz (green) served as the ground. The sampling frequency was set at 1000 Hz, and the impedance of electrodes was kept below 10kΩ during the recordings. To minimize interference, signals were filtered by an analog bandwidth filter (0.1–100 Hz) and a notch filter (50 Hz). Before offline analysis, artifacts caused by eye movements were removed using the automatic artifact removal (AAR) algorithm based on the SOBI algorithm [Citation22] from the EEGLAB toolbox [Citation23].
In this study, piezoelectric tactors were utilized to provide vibration stimulation, offering the benefits of rapid response, low noise, and high precision. For the experiment, two piezo tactors were securely attached to the middle of the subject’s left and right wrists using medical tape. Recognizing that the sensitivity of skin mechanoreceptors to stimulus frequency varies [Citation24], we used a signal of 200 Hz sinusoidal carrier frequency modulated by 23 Hz sinusoidal frequency to drive the tactors. This signal was generated using Matlab software and amplified via an audio amplifier to activate the tactors for vibration. This signal was designed to stimulate both Pacinian corpuscles and Meissner corpuscles. Before commencing the formal experiment, subjects underwent a pre-experiment to familiarize themselves with various vibrations at various amplitudes. They were asked to select the intensity that they could distinctly perceive without disrupting their ability to perform the motor imagery task. This selected intensity served as the parameter for the main experiment.
2.3. Experimental procedure
In this study, subjects were comfortably seated in an armchair with their eyes positioned approximately one meter from the display screen. Their hands were placed at ease on the armrests, with their wrists facing upward. The experimental setup is shown in . The experimental protocol was developed using Matlab software, and visual stimuli were delivered via Psychtoolbox [Citation25]. The experiment consisted of two sessions: a pure motor imagery (PMI) session without vibration and a motor imagery with continuous vibration tactile stimulation (VT) session. The subjects completed these two sessions in sequence, with each session containing two runs and each run containing 40 trials. illustrates the timeline of a single trial in both sessions.
Figure 3. Experimental procedure of the two sessions. Each session contains two runs, and each run consists of 40 trials.
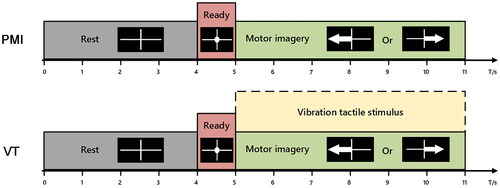
During the PMI task, a white cross was displayed on the screen for 4 s, indicating to the subject that they could relax. Then, a white circle was displayed in the center of the cross for one second, indicating that the subject should begin the motor imagery task. After that, a white arrow pointing left or right was displayed on the screen for 5th–11th second, indicating that the subject should imagine performing a left or right hand movement for the next 6 s. Finally, a white cross was displayed again on the screen to begin the next trial. The VT task had the same time course as the PMI task, except that vibration stimulation was continuously applied to both wrists of the subject during the MI task.
Each run consisted of 20 trials of left hand motor imagery and 20 trials of right hand motor imagery, performed in random order. Each trial lasted for 11 s, resulting in a total run time of approximately 7 min. Subjects were given a 5–10 min break between runs. To prevent placebo effects, the piezoelectric actuators remained attached to their wrists throughout the experiment. Additionally, as all subjects were naive to BCI experiments, they performed 20 trials of actual movement and 20 trials of MI before the start of the experiment to ensure consistency in their MI performance.
2.4. Algorithms and analysis methods
We used custom-built MATLAB scripts and EEGLAB toolbox to analyze EEG data offline. To evaluate the mean spectral power changes in time-frequency and spatial domains, we choosed event-related spectral perturbation (ERSP) and ERS/ERD method [Citation26]. Because the MI paradigm mainly affects rhythmic activity in the 8–30 Hz frequency band, in order to better analyze the impact of vibration tactile stimulation on the MI paradigm, this study mainly analyzes the alpha (8–12 Hz) and beta (13–30 Hz) band activity of the corresponding channels in the sensory-motor brain region, typical related channels include C3, C4, CP3, and CP4. The ERSP of n trials was calculated by the Equation 1:
where n is the number of trials and
denotes the spectral estimation of the kth trial at frequency
and time
The ERSP was computed in EEGLAB by calculating a short-time Fourier transform (STFT) every 200 ms with a Hanning-tapper. The reference interval was the 3rd–4th second of each trial (1 s before the white circle appeared). The topographical distributions of ERSP were computed by averaging the ERSP values of all electrodes within the specific frequency bands at specific times.
To quantify the functional connectivity between different brain regions, we used the phase-locking value (PLV) which can measuring the phase synchronization information between pairs of signals [Citation27, Citation28]. It is calculated over the N-sample window as follows:
where N represents the sample amount of each signal.
stands for the instantaneous phase difference between each pair of EEG channels
on time window
The instantaneous phase is calculated by the Hilbert transform. The PLV ranges from 0–1, with 1 denoting complete phase synchronization and 0 denoting that the signals are completely desynchronized.
About the classification performance, we used a common spatial pattern (CSP) algorithm for feature extraction and linear discriminative analysis (LDA) as a classifier. All 63 channels of data are used for classification. We filter the raw data to 8–30Hz and extract data from different time periods for classification to explore the classification performance of different tasks. A 10-fold cross-validation strategy was used in the classification for statistical analysis.
3. Result
The average ERSP topographic distribution across all subjects is displayed in , which illustrates the ERSP changes in the alpha band and beta band during the initial 5 s of imagined movements in both tasks. As evident in the PMI task, when subjects envisioned left or right hand movements, ERD was observed in the contralateral primary motor cortex, particularly within the alpha band. Although activation in the beta band was relatively weaker, a contralateral activation trend persisted. In contrast, the brain activation in the VT task was significantly stronger than that in the PMI task. In the alpha band, the ERD degree and range of activation in the sensorimotor cortex were deeper and broader. When imagining right hand movements, significant bilateral activation of the sensorimotor cortex was observed. In the beta band, stronger desynchronization than that in the PMI task was also evident. However, the range of activation was relatively concentrated and not as extensive as that in the alpha band.
Figure 4. Grand-averaged spatial distributions of ERSP patterns of all subjects for each class and frequency band. Each row displays the ERSP changes during the first 5 s after imagery begins.
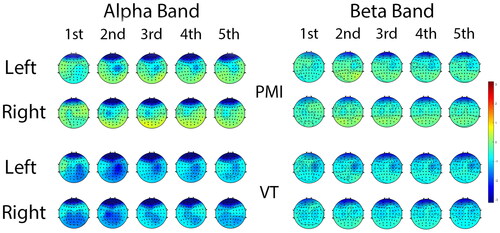
To gain a deeper understanding of the impact of vibration stimulation on the activation levels of motor function areas, we selected two representative channels, C3 and C4, to calculate the ERD extrema during the execution of all motor imagery tasks in both types of tasks. presents the distribution of ERD extrema across different tasks and frequency bands. As evident in the figure, the inclusion of tactile stimulation generally results in a reduction of ERD extrema. Notably, both left- and right-hand imagery tasks produce more significantly reduces in the C4 channel, particularly within the alpha frequency band.
Figure 5. The peak ERD values over the C3 and C4 channels for two sessions for all subjects. Where * represents p < 0.05.
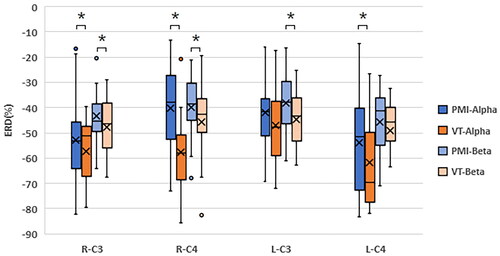
presents the average PLV values across all channels under various frequency bands over time. As shown in the figure, in both the theta and alpha bands, regardless of whether vibration stimulation was applied or not, there was a notable increase in PLV values following the commencement of the MI task that persisted until its conclusion. This suggests that the execution of imagination tasks enhances the functional connectivity level of certain brain regions. In the PMI task, there was not much difference in PLV changes between left and right hand MI. In the theta band, the VT task generated stronger functional connectivity than the PMI task during MI, and this enhancement was more significant in the alpha band, especially during left hand imagery with vibration stimulation, where this enhancement was most significant 2 s after the MI task began. In the beta band, it is evident that the mean PLV of the VT task consistently exceeded that of the PMI task, regardless of whether the subject was engaging in MI or not. This indicates that the somatosensory stimulation-induced activation and functional connectivity enhancement in the sensory cortex are primarily concentrated in the beta band, and this activation likely has a sustained impact.
The alterations in time-frequency characteristics and functional connectivity levels may impact classification outcomes. In this study, a sliding time window of 2 s was utilized to assess the changes in classification accuracy over the course of imagination. presents the individual decoding rates of the subjects for two types of tasks, along with the average values and standard deviations for all subjects. illustrates the changes in the average decoding accuracy over time for all subjects under the two different tasks. As shown in the figure, within the initial 2 s of imagery, there was minimal disparity in classification accuracy between the two tasks. The average classification rate for the PMI task exceeded that of the VT task in the first three windows. However, the classification accuracy of the VT task exhibited an upward trend as the imagery progressed, peaking at the 7th time window. Notably, the classification accuracy of the VT task significantly surpassed that of the PMI task in the 7th and 8th classification time windows (p = 0.036, 0.018). This suggests that vibration tactile stimulation does not immediately enhance decoding accuracy but rather requires a certain adaptation period to significantly enhance classification results during the later stages of MI.
Table 1. Classification accuracies and mean accuracies in two conditions over 14 subjects.
4. Discussion
This study delved into the impact of continuous vibration tactile stimulation on both wrists on MI performance in two hands. We evaluated subjects’ time-frequency characteristics, decoding accuracy, and functional connectivity levels, tracking their variations over time. Our findings reveal that during MI, bilateral vibration stimulation enhances contralateral sensorimotor brain region activation and improves the functional connectivity level of the alpha and beta frequency bands of the brain. However, it does not directly enhance the decoding accuracy of MI in both hands.
Chatterjee et al. [Citation29] previously reported that when vibration stimulation was applied to the same side as the imagery hand, it significantly boosts MI-BCI decoding accuracy, with a more significant improvement on the left side compared to the right. Our findings align with these results. Users appeared particularly susceptible to tactile stimulation’s influence when imagining movement of their non-dominant hand, leading to notable activation in both side sensory-motor cortices. Nevertheless, bilateral stimulation may confuse or interfere with some users, leading to decreased classification accuracy. Additionally, vibration stimulation led to notable activation in the ipsilateral sensory-motor cortex, which diminished ERD lateralization to some extent and could potentially hinder classification. Nevertheless, given the spontaneous nature of the MI paradigm, it is not feasible to apply stimulation exclusively to the imagined side in practical applications. Therefore, the findings of this study remain positive.
After analyzing the PLV results, we observed a more significant improvement in MI of the non-dominant hand when vibration stimulation was added. This could be attributed to the fact that users’ attention is drawn to the left tactile stimulation, leading to more significant activation compared to the right side. Previous studies have suggested that beta rebound likely reflects a transient state of arrest or inhibition of the motor cortex network [Citation30], which might explain the decrease in functional connectivity in the beta band during motor imagery. Interestingly, unlike the rapid enhancement of ERD and PLV, the classification accuracy began to boost about 2 s after the application of stimulation. This might be because subjects needed time to adapt to the change in imagery rhythm brought by tactile stimulation or because subjective intention needs time to stabilize. Similarly, Ahn et al. [Citation20] designed an experimental paradigm in which vibration stimulation was applied for the first three seconds of imagery and then withdrawn for the next three seconds. Their results showed that after the end of vibration stimulation, individual subjects had a continuous decrease in ERD, and the decoding accuracy also showed an upward trend. This suggests that vibration stimulation may act as a pre-stimulus, helping users produce ERD earlier and maintain it for a longer period of time, thus providing more discriminative information for MI classification. Furthermore, Wang et al. used long-term electrical stimulation-assisted training to enhance the pattern level and classification accuracy of MI-BCI [Citation31]. This demonstrates the long-term effectiveness of tactile stimulation and also highlights its potential in MI training.
presents the event-related spectral perturbation (ERSP) distribution for a typical subject (S12) in the time-frequency domain. As evident from the figure, this subject did not evoke continuous and significant ERD during the PMI tasks. However, the VT tasks exhibited significant enhancement, characterized by consistent activation in both the alpha and beta frequency bands of the sensorimotor cortex. Notably, right hand tasks displayed more lateralization advantages compared to left hand tasks. Channels CP3 and CP4, without tactile stimulation input, displayed minimal activation during PMI tasks. In contrast, VT tasks triggered significant activation in these channels, exhibiting patterns similar to channels C3 and C4. It’s worth noting that alpha band activation was primarily concentrated in somatosensory regions. Moreover, right hand imagery tasks generated distinct activation on both sides, while left hand tasks failed to evoke significant activation. This suggests that vibration stimulation aids users in focusing more on MI, rather than solely activating somatosensory cortices as a stimulus.
This study presents a preliminary exploration with a limited set of vibration stimulation parameters and a standardized stimulation approach. The primary objective is to investigate the temporal and activation patterns of vibration stimulation in enhancing the MI paradigm. The unique characteristics of tactile stimulation, which does not occupy the visual channel and can close the motor sensory loop, make the tactile-MI hybrid paradigm particularly suitable for rehabilitation training and the control of rehabilitation robots. The results observed in this study indicate that vibration stimulation can induce widespread activation of brain regions. This, to some extent, may contribute to expediting functional reorganization and recovery in damaged brain areas. Furthermore, the integration of vibration stimulation with rehabilitation robots holds significant potential for enhancing rehabilitation outcomes. This is attributed to the advantages of vibration stimulation, including its high safety profile, small size, and high user acceptance. Previous studies have explored the application of vibration stimulation in stroke rehabilitation training and reported improvements in patients’ tactile perception and motor function recovery [Citation32–34]. However, most of these studies have not involved the analysis of electrophysiological signals, the underlying physiological mechanisms remain unverified, and they have not been applied to active rehabilitation systems based on brain control. In future studies, we will attempt to incorporate different vibration stimulation methods, explore the effects of applying vibration stimulation to different limb positions and at different time points during imagery on the overall performance of MI, and develop a hybrid EEG control system based on tactile assistance.
5. Conclusion
In this study, we investigated the instantaneous effects of constant bilateral vibration tactile stimulation on the overall performance of the left-right hand MI paradigm. We delved into the electrophysiological signal characteristics of imagery tasks involving different limbs and the distinct response patterns to vibration stimulation. Our findings indicate that the addition of vibration stimulation significantly boosts sensorimotor cortex activation during MI and enhances functional connectivity in the brain, particularly in the alpha and beta frequency bands. Notably, we observed that bilateral vibration stimulation did not immediately enhance overall MI classification accuracy; rather, a certain duration of stimulation was important before any improvement in classification rates became apparent. The findings of this study provide valuable insights for the design of vibration tactile-assisted online motor imagery control systems in future research. Furthermore, they hold significant promise for applications in brain-controlled robotics, particularly in rehabilitation robot training systems.
Disclosure statement
No potential competing interest was reported by the authors.
This work involved human subjects in its research. Approval of all ethical and experimental procedures and protocols was granted by the Ethics Committee of the First Affiliated Hospital of Nanjing Medical University under Application No. 2020-SR-362.
Additional information
Funding
Notes on contributors
Wenbin Zhang
Wenbin Zhang received the Ph.D degree in Instrument Science and technology from Southeast University in 2023. He is currently a Postdoctoral Researcher at the Collage of Computer Science and Software Engineering, Hohai University. His research interests include brain-computer interfaces, rehabilitation robotics, and tactile perception.
Aiguo Song
Aiguo Song received the Ph.D degree in measurement and control from Southeast University in 1996. He has authored or coauthored more than 210 technical papers in the peer-refereed journals. He is currently a Professor with the School of Instrument Science and Engineering, Southeast University. His current interests concentrate on teleoperation, haptic display, Internet telerobotics, and distributed measurement systems.
Hexuan Hu
Hexuan Hu received the B.S. degree in the thermal energy engineering from South-East University, Nanjing, P. R. China, in 1998, and the M.Sc. and Ph.D. degrees in automation science, computer engineering and signal processing from the University of Science and Technology of Lille, Villeneuve-d’Ascq, France, in 2003 and 2009, respectively. He is currently the Professor in the College of Computer and Information, Hohai University, Nanjing, China, where he develops and applies models and algorithms in the fields of Artificial Intelligence, Internet of Things, Intelligent Control Theory, Deep Learning, Automated Planning, and Model Checking.
Minmin Miao
Minmin Miao received the B.Eng. degree in electronic information engineering from Jiangnan University, Wuxi, China, in 2012, the M.Sc. degree in signal and information processing from Jiangnan University, Wuxi, China, in 2015, and the Ph.D. degree in instrument science and technology from Southeast University, Nanjing, China, in 2018. He is currently an Associate Professor with the School of Information Engineering, Huzhou University, Huzhou, China. His research interests include brain-computer interface, signal processing and pattern recognition.
Baoguo Xu
Baoguo Xu received the B.S. degree in measurement and control from the China University of Mine and Technology, Xuzhou, China, in 2004, and the Ph.D. degree in measurement and control from Southeast University, Nanjing, China, in 2009. He is currently a Professor with the School of Instrument Science and Engineering, Southeast University. His current research interests include brain computer interface, natural human–robot interaction, soft robotics and rehabilitation robotics.
References
- Pfurtscheller G, Neuper C. Motor imagery and direct brain-computer communication. Proc IEEE. 2001;89(7):1123–1134. doi: 10.1109/5.939829.
- Pfurtscheller G, Da Silva FHL. Event-related EEG/MEG synchronization and desynchronization: basic principles. Clin Neurophysiol. 1999;110(11):1842–1857. doi: 10.1016/s1388-2457(99)00141-8.
- Pfurtscheller G. Spatiotemporal ERD/ERS patterns during voluntary movement and motor imagery. Suppl Clin Neurophysiol. 2000;53:196–198. doi: 10.1016/s1567-424x(09)70157-6.
- Keng AK, Cuntai G, Soon PK, et al. Brain-computer interface-based robotic end effector system for wrist and hand rehabilitation: results of a three-armed randomized controlled trial for chronic stroke. Front Neuroeng. 2014;7:30. doi: 10.3389/fneng.2014.00030.
- Wolpaw JR, Birbaumer N, Mcfarland DJ, et al. Brain–computer interfaces for communication and control. Clin Neurophysiol. 2002;113(6):767–791. doi: 10.1016/s1388-2457(02)00057-3.
- Guger C, Edlinger G, Harkam W, et al. How many people are able to operate an EEG-based brain-computer interface (BCI)? IEEE Trans Neural Syst Rehabil Eng. 2003;11(2):145–147. J doi: 10.1109/TNSRE.2003.814481.
- Ahn M, Cho H, Ahn S, et al. High theta and low alpha powers may be indicative of BCI-illiteracy in motor imagery. PLoS One. 2013;8(11):e80886. doi: 10.1371/journal.pone.0080886.
- Ahn M, Jun SC. Performance variation in motor imagery brain–computer interface: a brief review. J Neurosci Methods. 2015;243:103–110. doi: 10.1016/j.jneumeth.2015.01.033.
- Makeig, S., Kothe, C., Mullen, T., Bigdely-Shamlo, N.,. Evolving signal processing for brain–computer interfaces. Proc. IEEE. 2012;100(Special Centennial Issue):1567–1584. doi: 10.1109/JPROC.2012.2185009.
- Lotte F, Bougrain L, Cichocki A, et al. A review of classification algorithms for EEG-based brain-computer interfaces: a 10 year update. J Neural Eng. 2018;15(3):031005. doi: 10.1088/1741-2552/aab2f2.
- Leeb R, Sagha H, Chavarriaga R, et al. A hybrid brain–computer interface based on the fusion of electroencephalographic and electromyographic activities. J Neural Eng. 2011;8(2):025011. doi: 10.1088/1741-2560/8/2/025011.
- Pfurtscheller G, et al. The hybrid BCI. Frontiers Neurosci. 2010;4:3.
- Wang Z, Zhou Y, Chen L, et al. BCI monitor enhances electroencephalographic and cerebral hemodynamic activations during motor training. IEEE Trans Neural Syst Rehabil Eng. 2019;27(4):780–787. Apr doi: 10.1109/TNSRE.2019.2903685.
- Zeng H, et al. Closed-loop hybrid gaze brain-machine interface based robotic arm control with augmented reality feedback. Frontiers Neuro-Robotics. 2017;11:60.
- Allison BZ, Brunner C, Kaiser V, et al. Toward a hybrid brain–computer interface based on imagined movement and visual attention. J Neural Eng. 2010;7(2):26007. doi: 10.1088/1741-2560/7/2/026007.
- Gomez-Rodriguez M, Peters J, Hill J, et al. Closing the sensorimotor loop: haptic feedback facilitates decoding of motor imagery. J Neural Eng. 2011;8(3):036005. doi: 10.1088/1741-2560/8/3/036005.
- Yi W, Qiu S, Wang K, et al. Enhancing performance of a motor imagery based brain–computer interface by incorporating electrical stimulation-induced SSSEP. J Neural Eng. 2017;14(2):026002. doi: 10.1088/1741-2552/aa5559.
- Yao L, Meng J, Zhang D, et al. Combining motor imagery with selective sensation toward a hybrid-modality BCI. IEEE Trans Biomed Eng. 2014;61(8):2304–2312. Aug doi: 10.1109/TBME.2013.2287245.
- Shu X, Chen S, Meng J, et al. Tactile stimulation improves sensorimotor rhythm-based BCI performance in stroke patients. IEEE Trans Biomed Eng. 2019;66(7):1987–1995. Jul. doi: 10.1109/TBME.2018.2882075.
- Ahn S, Ahn M, Cho H, et al. Achieving a hybrid brain–computer interface with tactile selective attention and motor imagery. J Neural Eng. 2014;11(6):066004. doi: 10.1088/1741-2560/11/6/066004.
- Zhang W, Song A, Zeng H, et al. Closed-loop phase-dependent vibration stimulation improves motor imagery-based brain-computer interface performance. Frontiers Neurosci. 2021;15:15.
- Gómez-Herrero G. Automatic artifact removal (AAR) toolbox v1.3 (release 09.12.2007) for MATLAB. Tampere Univ. Technol., Tampere, Finland. 2007;3:1–23.
- Delorme A, Makeig S. EEGLAB: An open source toolbox for analysis of single-trial EEG dynamics including independent component analysis. J Neurosci Methods. 2004;134(1):9–21. Mar doi: 10.1016/j.jneumeth.2003.10.009.
- Breitwieser C, Kaiser V, Neuper C, et al. Stability and distribution of steady-state somatosensory evoked potentials elicited by vibro-tactile stimulation. Med Biol Eng Comput. 2012;50(4):347–357. Apr doi: 10.1007/s11517-012-0877-9.
- Brainard DH. The psychophysics toolbox. Spatial Vis. 1997;10(4):433–436. doi: 10.1163/156856897X00357.
- Romain G, Arnaud D. Single-trial normalization for event-related spectral decomposition reduces sensitivity to noisy trials. Front Psychol. 2011;2. doi: 10.3389/fpsyg.2011.00236.
- Lachaux JP, Rodriguez E, Martinerie J, et al. Measuring phase synchrony in brain signals. Hum Brain Mapp. 1999;8(4):194–208. doi: 10.1002/(SICI)1097-0193(1999)8:4<194::AID-HBM4>3.0.CO;2-C.
- Gysels E, Celka P. Phase synchronization for the recognition of mental tasks in a brain-computer interface. IEEE Trans Neural Syst Rehabil Eng. 2004;12(4):406–415. doi: 10.1109/TNSRE.2004.838443.
- Chatterjee A, Aggarwal V, Ramos A, et al. A brain-computer interface with vibrotactile biofeedback for haptic information. J Neuroeng Rehabil. 2007;4(1):40. doi: 10.1186/1743-0003-4-40.
- Pfurtscheller G, Neuper C, Brunner C, et al. Beta rebound after different types of motor imagery in man. Neurosci Lett. 2005;378(3):156–159. doi: 10.1016/j.neulet.2004.12.034.
- Wang Z, He B, Zhou Y, et al. Incorporating EEG and EMG patterns to evaluate BCI-based long-term motor training. IEEE Trans Human-Mach Syst. 2022;52(4):648–657. doi: 10.1109/THMS.2022.3168425.
- Seim CE, Ritter B, Starner TE, et al. Design of a wearable vibrotactile stimulation device for individuals with upper-limb hemiparesis and spasticity. IEEE Trans Neural Syst Rehabil Eng. 2022;30:1277–1287. doi: 10.1109/TNSRE.2022.3174808.
- Seim C, Chen B, Han C, et al. Relief of post-stroke spasticity with acute vibrotactile stimulation: controlled crossover study of muscle and skin stimulus methods. Front Hum Neurosci. 2023;17:1206027. doi: 10.3389/fnhum.2023.1206027.
- Seim CE, Wolf SL, Starner TE. Wearable vibrotactile stimulation for upper extremity rehabilitation in chronic stroke: clinical feasibility trial using the VTS Glove. Journal of Neuro Engineering and Rehabilitation. 2021;18:1–11. doi: 10.1186/s12984-021-00813-7.