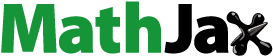
Abstract
Abstract: The purpose of this paper is to examine the determinants of health insurance, healthcare utilisation, healthcare expenditure, and health status simultaneously. The study used secondary data from the Ghana Socioeconomic Panel Survey. Health insurance, healthcare utilisation, healthcare expenditure, and health status were estimated simultaneously using the conditional mixed process (CMP) framework. The CMP corrects for likely heterogeneity and sample selection bias. The results revealed a significant association between insurance, healthcare utilisation, healthcare expenditure, and health status, implying that regressing these models apart would yield bias and inconsistent estimates making CMP estimates superior to single estimations. The results discovered that gender, age, education, obesity, physical activity, wealth index, household size, dependency ratio, formal-sector work, and savings significantly drive NHIS enrolment. Gender, age, education, obesity, chronic illness, physical activity, wealth index, risky behaviour, and type of illness influenced individuals’ visits to a health facility. The determinants of out-of-pocket payments were gender, chronic illness, wealth index, risky behaviour, distance to a health facility, and fever and diarrhoea sufferers. Finally, drivers of self-assessed health were gender, age, education, chronic illness, physical activity, wealth index, risky behaviour, fever, cold/cough and diarrhoea.
1. Introduction
Healthcare decision-making process by nature could be complicated. In most cases, both patients and doctors are involved in the decision-making process. For instance, first the patient will have to decide whether to visit a health care provider or not when ill and thereafter both the patient and the doctor will jointly decide what treatment the patient should have. This situation makes such health care decisions interrelated. Previous studies dealt with this two-part decision-making process by using the two-part model as suggested by the RAND Health Insurance Experiment (Manning et al., Citation1987). The RAND experiment likened plans of differing benefit generosity instead of comparing insured and uninsured. Another shortcoming of the RAND experiment is that it focused on the effects of cost-sharing on healthcare utilisation and health outcome rather than looking at expansion of social health insurance (Newhouse, Citation1993). The two-part model has been used extensively in the literature (Ekman, Citation2007; Nguyen et al., Citation2011). In a recent study, Woldemichael et al. (Citation2019) used an extended two-part model to model the insurance enrolment decision, the decision to spend and the conditional amount of spending simultaneously. To the best of our knowledge, no study has addressed the whole process of insurance choice, healthcare utilisation, healthcare expenditure and health status simultaneously. This study aims to fill this gap.
Interrelationships can be seen in insurance, healthcare utilisation, healthcare expenditure and health status. Insurance coverage can be included in our model mainly because it may influence a patient and doctor’s joint decision about treatment procedures or choices. For instance, insured persons are more likely to accept and undergo more complex medical procedures than their uninsured counterparts. Added to this, there could be an adverse selection problem because less healthy individuals are more likely to purchase insurance. Therefore, this study proposed a model that examines the determinants of health insurance, healthcare utilisation, healthcare expenditure and health status and accounts for their interrelationship simultaneously. The appropriate econometric technique proposed here is the Conditional Mixed Process (CMP) model as suggested by Roodman (Citation2011).
This paper contributes to the current body of empirical literature by accounting for the interrelated nature of healthcare decisions, namely health insurance, healthcare utilisation, healthcare expenditure, and health status. One important issue that emerges from the literature on health insurance, healthcare utilisation, healthcare expenditure and health status is the fact that no attempt, to the best of our knowledge, has been made to model these four thematic areas in a systematic way. Studies often look at the causal effects of health insurance on healthcare utilisation, healthcare expenditure or other outcomes (e.g., Ekman, Citation2007). Therefore, it is critical to explore how these four thematic areas interact when seen as a whole. This study attempts to fill this gap by jointly modelling health insurance, healthcare utilisation, healthcare expenditure, and health status within the CMP framework, thereby making a methodological contribution. Four structural models, namely health insurance, healthcare utilisation, healthcare spending, and health status models, are taken into account in this study. Models for health insurance and healthcare utilisation are discrete: a person is either insured or not, and they have either visited a health facility or not. Healthcare expenditure is a continuous model, while health status is an ordered model. The health insurance and healthcare utilisation models can be estimated using binary choice models (probit or logit). Whereas healthcare expenditure and health status models can be estimated using ordinary least squares and ordered (ordered probit and logit) models, respectively. It is feasible to model these structural models as standalone, using single equation estimation techniques. This study, however, opted for modelling these using a systems approach. The application of the CMP to simultaneously modelled healthcare decisions is a novelty. A unique feature of this modelling framework is that the individual equations may be binary, categorical, ordered, censored or interval-based measures rather than a continuous dependent variable (the classical regression). The CMP technique addresses sample selection bias and endogeneity and provides efficient estimates.
2. Methodology
2.1. Data
The Ghana Socioeconomic Panel Survey (GSPS) dataset forms the basis of this empirical analysis. This survey is a joint work between the Institute of Statistical, Social and Economic Research (ISSER) at the University of Ghana and the Economic Growth Centre at Yale University. The study utilized the first three waves (2009/2010, 2014/2015 and 2018/2019), comprising a total sample of 31,807 individuals. The dataset covers information on households’ demographic characteristics, assets, household health (including information on insurance status, anthropometry, activities of daily living, miscellaneous health, health in the past two weeks, health in the past 12 months before the survey), consumption module and housing characteristics, among others. During the data collection process, the survey used a two-stage stratified sample design. The first stage encompasses enumeration areas (EAs) selection, while the second stage involves a random selection of 15 households from each EA throughout the ten administrative regions of Ghana.
2.2. Theoretical model
The paper adopts the demand for health care services model based on the consumer behaviour theory. This model was first developed by Grossman (Citation1972) and modified by some health researchers such as Jewell (Citation2009) and Mwami and Oleche (Citation2017). The theory postulates that a consumer divides his income among several competing demands to maximize utility. Related to this study, a sick person’s decision to seek medical attention or not is subject to his production function and budget constraints. Thus, utility maximisation is the optimisation problem, and it is provided by the function:
Where stands for the consumer utility derives from health care services,
represents consumption goods that provide utility to an individual but have no direct effect on his health,
is for health-related goods that affect an individual’s health directly, and
represents an individual’s health status. The sick individual seeks to maximize utility subject to health production function and budget constraints. The budget constraint is expressed as:
Where stands for income,
,
as defined before and
represents personal healthcare. Prices for consumer goods, health-related goods, and individual health care are denoted by
,
, and
, respectively. The health production function is provided by:
Where stands for predisposing factors including demographic and socio-economic characteristics such as age, education level, income and gender of the sick person; health system characteristics such as distance to health care facility, cost of services, and quality of services offered; health factors such as chronic illness and self-assessed health; and enabling factors such as accessibility and insurance coverage.
The solution to the optimisation problem using Equationequations 1(1)
(1) , Equation2
(2)
(2) and 3 results in the formation of a Lagrangian function expressed as follows:
The demand function is given by equation (4), and its solution yields the optimal values of ,
and
that maximise the utility of the customer, in this case the sick person. Equation (4) can be solved to get the reduced form of the demand function for a sick person using health care services, which results in:
Where are as described before, and
represents the demand for individual health care services. The price of goods and services, income, the demographic and socioeconomic features of the sick person, the characteristics of the health system, and enabling factors are all matters that affect how much a person uses health care services. In this paper, the determinants of health insurance, healthcare utilisation, healthcare expenditure and health status are examined simultaneously.
2.3. Empirical model
The CMP model is mainly built as a seemingly unrelated regression (SUR) model and as a result permits building different models and mixing them in a multi-equation fashion (Roodman, Citation2011). In the CMP framework, individual equations need not follow the classical regressions model where the dependent variable is continuous. Also, the CMP framework may allow ordered probit estimation. The invocation of CMP allows for the specification of several equations with each using a different estimation technique and allowing each equation to differ by observation. Estimation under CMP uses the maximum likelihood technique. The basic assumption underpinning the CMP framework is the joint modelling of two or more equations which allow for cross-equation correlation of the error terms. This provides a justification for its use in this current study. A SUR model of health insurance status (), health care utilisation (
), healthcare expenditure (
) and health status in the spirit of the CMP is specified as follows:
Assuming that is observed, then the likelihood function can be specified as:
According to Roodman (Citation2011), this function is impossible to directly estimate using standard functions in statistical software. To directly estimate, we have to factor into probability distribution functions,
and
.Footnote1
Based on the foregoing, the empirical specifications of the four models are:
where ,
and
are health insurance status (i.e., NHIS enrolment), healthcare utilisation (i.e., visits to health facility), healthcare expenditure (i.e., out-of-pocket payments), and health status (i.e., self-assessed health), respectively;
is the set of variables hypothesized to influence
,
and
. These set of variables include gender (male), age, education, obesity, chronic illness, physical activity, wealth index, type of illness suffered, body mass index, risky behaviour, formal-sector work, dependency ratio, household size, savings, and distance to a health facility. Representing the respective error terms are
,
and
. Table shows the measurement of variables and their descriptive statistics.
Table 1. Variables, measurement and descriptive statistics
3. Results and discussions
The study examines the determinants of health insurance, healthcare utilisation, health expenditure and health status and their interrelationship. Variables included in the CMP estimations were carefully selected to avoid collinearity and ensure convergence. Baseline regression results are presented in the Appendix Tables A1. Table presents the CMP estimates in which issues of sample selection bias and endogeneity are addressed. The rho (ρ) parameters measure the association between our four models. The results show a significant relationship between health insurance and healthcare utilisation, health insurance and health expenditure, and healthcare utilisation and health status. These findings imply that separately estimating these models is most likely to yield bias and inconsistent estimates. Therefore, the CMP estimates are superior to single equations estimates. As a result, CMP joint estimates form the basis of the discussion of our results.
Table 2. Conditional Mixed Process estimates of health insurance, healthcare utilisation health expenditure and health status
A diagnostic assessment of our model shows that the model reasonably fits the data well, as revealed by the Wald test, which is significant at a 1 per cent level. Columns 2 and 3 present the probit estimates for the factors influencing NHIS enrolment or membership (see, Table ). We find gender, age, education, obesity, physical activity, wealth index, household size, dependency ratio, formal-sector work and savings to be significant in explaining NHIS enrolment. The male gender negatively determines NHIS enrolment at a one per cent level, meaning that males have a lower likelihood of NHIS enrolment than females. The marginal effect suggests that the probability of males enrolling in the NHIS decreases by 9.1 percentage points. Generally, males are more risk-loving and less likely to take protective measures such as health insurance purchases against future illness episodes. The present finding is consistent with the works of Sekyi et al. (Citation2015) and Ghosh and Gupta (Citation2017) but contradicts the work of Duku (Citation2018).
The age variable significantly influences NHIS membership positively. This finding supports economic theory proposing that ageing depreciates health stock which intends to make individuals purchase health insurance as a health investment to decrease the rate of depreciation and to overcome catastrophic health expenditure upon illness episodes (Grossman, Citation1972). The result also corroborates the findings of Sekyi et al. (Citation2015) and Duku (Citation2018).
Education is a positive determinant of NHIS membership. Education increases people’s knowledge, conceptual prowess, receptiveness and awareness. Therefore, highly educated individuals are better informed, increasing their enrolment likelihood. This finding is in line with previous studies (Dake, Citation2018; Duku, Citation2018; Salari et al., Citation2019; Sekyi et al., Citation2015).
The obesity variable is a positive determinant of NHIS membership at a 1 per cent level. This result means that the probability of an obese person enrolling in the NHIS increases by 2.3 percentage points. A plausible explanation for this finding is that obesity is often linked to many health risks such as diabetes, heart disease, stroke, and some types of cancer and its associated health care cost. Health insurance scheme provides a safety net in terms of financial protection against health cost, hence increased enrolment for obese persons who are likely to have higher healthcare cost than their non-obese counterparts.
The variable that captures people’s difficulty participating in physical activities/roles (i.e., physical activity) is a positive determinant of NHIS membership. The marginal effect indicates that the probability of an individual having difficulty participating in physical activities and enrolling in the scheme increases by 10.8 percentage points. By implication, many of these persons have underlying health conditions, hence would like to take up health insurance to cover future healthcare costs and improve their health status. This finding is in line with the work of Sekyi et al. (Citation2022).
Surprisingly, the wealth index is negatively associated with NHIS membership. This result suggests that as the household wealth index increases, the probability of individuals enrolling on the scheme decreases by 8.3 percentage points. A possible explanation for this finding is that wealthy persons can afford out-of-pocket payments and private health insurance, which seems more convenient. Hence, this reduces their probability of enrolling in the NHIS. This finding is in contrast with earlier studies by Dake (Citation2018), Salari et al. (Citation2019), and Nsiah-Boateng et al. (Citation2019).
Household size is a negative predictor of NHIS membership. Larger households tend to have fewer financial resources to pay the insurance premium, thereby reducing their enrolment. This finding confirms earlier studies (Kusi et al., Citation2015; Sekyi et al., Citation2015; Woldemichael et al., Citation2019). This result goes contrary to studies conducted by Sarpong et al. (Citation2010), Lu et al. (Citation2012), Dake (Citation2018), and Nsiah-Boateng et al. (Citation2019).
As expected, the formal-sector work variable positively predicts NHIS membership. The marginal effect suggests that persons from formal sector-headed households are 13.6% more likely to enrol in the NHIS. In the Ghanaian context, this finding is understandable because, as mandatory SSNIT contributors, formal-sector workers are allowed to register with the scheme without paying a premium. This result is similar to that of Wielen et al. (Citation2018), who found that older adults who live in households headed by formal sector employees were more likely to be enrolled in Ghana’s NHIS.
Savings is positively and significantly associated with NHIS membership. The marginal effect suggests that households whose heads save with banking institutions and others are 9.4% more likely to be members of NHIS. The plausible reason for this result is that savings increase households’ ability to pay a premium. Our finding agrees with Kimani et al. (Citation2012), who found that saving schemes and community-based saving groups were critical determinants of participation in public health insurance in Kenya.
The fourth and fifth columns present the probit estimates of determinants of healthcare utilisation. All the variables that entered into this model were significant. The gender variable negatively influences healthcare utilisation, suggesting that males are less likely to visit a health facility when ill than their female counterparts. The estimated marginal effect indicates that the probability of males visiting a health facility when sick decreases by 4.9 percentage points. Even though evidence suggests higher longevity for women relative to men, women tend to have a higher frequency of morbidity, leading to increased healthcare utilisation (Wingard et al., Citation1989). This finding is comparable to the work of Han-Kim and Lee (Citation2016) but contradicts the study by O’Connor (Citation2015).
Age has a significant positive effect on healthcare utilisation. Expectedly, older persons face deteriorating health conditions making them more likely to utilise care. This finding is consistent with the works of Awoke et al. (Citation2017) and Grustam et al. (Citation2020). However, this finding contradicts the works of Cameron et al. (Citation1988), Ekman (Citation2007), O’Connor (Citation2015), and Han-Kim and Lee (Citation2016), who found age to be associated with less health service utilisation.
Education is positive and statistically significant in explaining the probability of utilizing healthcare services. This result implies that for each additional educational level attained, the likelihood of an individual visiting a health facility when ill increases by 1.5 percentage points. A probable explanation for this finding is that, generally, education creates health awareness, particularly the need to seek care when ill and its related benefits, thereby making the highly educated more likely to utilize healthcare services. This finding supports evidence from the literature (Awoke et al., Citation2017; Grustam et al., Citation2020; Masiye & Kaonga, Citation2016).
Obesity is a positive predictor of healthcare utilisation. This result implies that obesity significantly increases the likelihood of visiting a health facility when ill. The marginal effect suggests that the probability of obese persons visiting a health facility when ill increase by 2.1 percentage points. A probable explanation for this finding is that obesity is associated with many health risks leading to increased healthcare utilisation. This finding confirms an earlier study in Estonia by Vals et al. (Citation2013).
Health-related variables that capture individual health care needs, mainly chronic illness and physical activity, are positive determinants of healthcare utilisation. The marginal effects suggest that the probabilities of utilising healthcare increase by 7.5 and 7.2 percentage points for individuals exposed to chronic health problems and those who have difficulty participating in physical activities, respectively. These findings reveal that utilisation of healthcare services increased for persons with observed healthcare needs. Studies by Ekman (Citation2007) and Han-Kim and Lee (Citation2016) observed an increased probability of outpatient usage for persons with chronic illness. Our finding on physical activity is similar to a study by Grustam et al. (Citation2020), which found increased utilisation of primary care for persons with daily activities impairment.
The coefficient of wealth index is negative and statistically significant in influencing the probability of visiting a health facility. This result implies that increasing wealth is associated with a lesser likelihood of health facility visitation. A plausible explanation for this finding is that the opportunity cost of illness is high for wealthy people. Since they have the means, they invest in health-enhancing measures. These measures may include eating good nutritious foods and maintaining preventive care. All these measures may lower their susceptibility to illnesses, leading to a reduction in health facility visitation. This finding supports the work of Ta et al. (Citation2020). However, this finding contradicts the study by Awoke et al. (Citation2017), who reported increased utilisation, particularly private health facility visits for wealthy persons.
The dummy that captures risky behaviour is negatively associated with healthcare utilisation. The marginal effect suggests that individuals who engage in risky behaviour are 2.7% less likely to visit a health facility when ill. Expectedly, individuals who engage in risky behaviour (i.e., consume alcoholic beverages and/or smoke or chew tobacco) often care less about life and are relatively less conscious about their health needs, resulting in lower healthcare utilisation. Previous studies have also revealed a lower healthcare utilisation for smokers and drinkers (Rezayatmand et al., Citation2017; Vals et al., Citation2013).
All the dummies that capture the type of illness suffered are positive determinants of healthcare utilisation. These findings imply that the probability of visiting a health facility when ill increases for sufferers of either fever, cold/cough or diarrhoea relative to other illnesses. The marginal effects suggest that fever, cold/cough or diarrhoea increase the probability of visiting a health facility by 28, 23.4 and 28.2 percentage points, respectively. These results demonstrate Ghanaians’ high level of concern about their health. This mentality of seeking care when ill with delays decreases numerous health risks and associated financial costs. The results are consistent with a study by Sekyi and Domanban (Citation2012). However, our findings contradict the work of Masiye and Kaonga (Citation2016), who reported that persons who had diarrhoea reduced their probability of seeking formal healthcare but instead had a higher likelihood for self-medication.
The ordinary least squares estimates of the determinants of health expenditure are presented in the sixth column. The predictors were gender, chronic illness, wealth index, risky behaviour, and distance to a health facility. Gender is a positive determinant of out-of-pocket health expenditures, suggesting that males pay more for health services than their female counterparts. Our finding is partly supported by a study in China by Du et al. (Citation2019), who found that women spend less on inpatient care but pay more on outpatient services than men. This result contradicts the findings of Malik et al. (Citation2012) in Pakistan, Masiye and Kaonga (Citation2016) in Zambia, Zeng et al. (Citation2018) in Zimbabwe and Aregbeshola and Khan (Citation2020) in Nigeria.
Contrary to expectations, the chronic illness variable predicts out-of-pocket health expenditures negatively. This result means that individuals suffering from chronic illnesses pay significantly less out-of-pocket health expenditures than those without it. This result contradicts the observation that individuals with chronic health conditions have observed healthcare needs resulting in increased utilisation. A plausible explanation for this finding is that individuals with chronic illnesses might have lost interest in formal care and may resort to self-treatment leading to lower out-of-pocket health expenditure. This surprising result is consistent with a study in Burkina Faso by Su et al. (Citation2006), where people were less likely to spend money on chronic sickness. However, this finding is inconsistent with previous studies (Aregbeshola & Khan, Citation2020; Du et al., Citation2019; Ekman, Citation2007; Nakovics et al., Citation2020).
The effect of the wealth index on out-of-pocket health expenditure is positive and statistically significant. This result means that the wealthy spend more on healthcare than the poor. Geographical inequalities in healthcare facilities and personnel distribution continue to exist in Ghana. The imbalance in the delivery of healthcare services creates access difficulties for the poor since they cannot afford it. Therefore, additional wealth increases the demand for high-quality health services resulting in higher out-of-pocket health expenditures. Our result is comparable to previous findings, such as Zeng et al. (Citation2018) in Zimbabwe and Nakovics et al. (Citation2020) in Malawi but contradicts the findings of Aregbeshola and Khan (Citation2020) in Nigeria.
The risky behaviour variable is a positive determinant of out-of-pocket health expenditure. This finding implies that individuals who engage in risky behaviour pay more when they use health care services. At first glance, the result may seem counterintuitive as we found that individuals who engage in risky behaviour are less likely to visit a health facility. The present finding suggests that though they could use less care, once they do, they pay more out-of-pocket health expenditures. This observation is likely due to their relatively less health consciousness. So whenever they demand health care, their illness may be severe, resulting in increased out-of-pocket payments. This result is the same as Rezayatmand et al. (Citation2017) and Du et al. (Citation2019).
The variable distance to a health facility is a positive determinant of out-of-pocket health expenditure. This finding suggests that the probability of incurring out-of-pocket health expenditure increases by 2.7 percentage points for every hour travelled to a health facility. This finding confirms studies by Malik et al. (Citation2012) and Masiye and Kaonga (Citation2016).
The determinants of health status measured by self-assessed health (SAH) are presented in the final column. The parameter estimates are from an ordered probit model. Of the ten variables, namely gender, age, education, chronic illness, physical activity, wealth index, risky behaviour, and dummies for fever, cold/cough and diarrhoea that significantly predict SAH, only the wealth index failed to meet our expectation. For example, the coefficient on gender is positive and statistically significant at a 1% level. This result implies that males are more likely to report good health than their female counterparts. Males are relatively more optimistic about their health relative to females. This finding corroborates previous studies such as Todorova et al. (Citation2013), Fan et al. (Citation2019), and Baidin et al. (Citation2021).
The coefficient on age is negative and statistically significant at a 1% level. This finding means that people are less likely to report being in good health as they get older. The aged are more likely to experience several health conditions simultaneously, making them rate their health low. This result corroborates earlier studies such as Fan et al. (Citation2019) in China, Sedefoğlu and Soytaş (Citation2019) in Turkey and Baidin et al. (Citation2021) in Russia. The aged are exposed to multiple health problems and illnesses linked to ageing, making them more pessimistic when self-reporting health (Bilgel & Karahasan, Citation2018; Franks et al., Citation2003).
Education is a positive determinant of SAH. The positive coefficient of education implies that individuals with higher educational attainment are more likely to report good health relative to their counterparts with lower educational attainment. Education enables individuals to have better jobs and higher wealth resulting in better access and utilisation of high-quality health care, all connected to better health reporting. This finding is consistent with previous studies (Baidin et al., Citation2021; Sedefoğlu & Soytaş, Citation2019; Todorova et al., Citation2013).
Expectedly, chronic illness and physical activity are negatively and significantly associated with the SAH. These findings mean that the likelihood of reporting good health decreases for individuals with a chronic health condition or those having difficulties participating in physical activities/roles. Individuals exposed to a chronic health condition or having physical difficulties often have several underlying health conditions making them less optimistic in describing their health status. These findings are consistent with previous studies (Asfar et al., Citation2007; Bilgel & Karahasan, Citation2018).
Contrary to expectations, the wealth index predicts SAH negatively. Individuals with a higher economic status generally self-report better health than those with lower economic levels. A plausible explanation for this outcome is that wealthy people are often prone to lifestyle diseases such as heart diseases, atherosclerosis, stroke, obesity, type 2 diabetes and the like. In the Ghanaian context, social and economic status is associated with body size. Hence, wealthy people prefer to become overweight and obese to merit their social status. All these attitudes expose the rich to lifestyle diseases, resulting in their reporting of poor health. This finding goes contrary to a study by Asfar et al. (Citation2007).
The variable controlling for individuals’ health behaviours is risky behaviour is a negative predictor of health. This result means that individuals who smoke, drink, or do both are less likely to describe their health as good. Health risks linked to smoking and drinking include heart disease, stroke, cancer, lung diseases, diabetes, cardiovascular diseases, chronic liver diseases, cancers, acute alcohol poisoning, and the like. All these health risks can make individuals who smoke and drink think less optimistic about their health. This finding compares well with previous studies such as Todorova et al. (Citation2013) and Fan et al. (Citation2019).
All the dummies that capture the type of illness suffered negatively determine SAH. These results imply that sufferers of fever, cold/cough or diarrhoea reported low values of self-assessed health status. These results are understandable because some people who had these ailments were just about getting over them or were still suffering from them during the survey’s memory period. All of these can make them less optimistic in describing their health.
4. Conclusion
This study was part of the author’s PhD study spanning from 2018 to 2021. The study was unique in that it looked at the determinants of health insurance, healthcare utilisation, health expenditure and health status and their interrelationship concurrently. The study employed the CMP estimation technique to correct for possible heterogeneity and sample selection bias. The results revealed a significant association between these indicators. By implication, these findings suggest that estimating these models alone would yield bias and inconsistent estimates, thus making the CMP estimates more superior to single equations estimates. The results further revealed that gender, age, education, obesity, physical activity, wealth index, household size, dependency ratio, formal-sector work, and savings were significant determinants of NHIS enrolment. Factors that influence visits to a health facility were gender, age, education, obesity, chronic illness, physical activity, wealth index, risky behaviour, and type of illness suffered. The drivers of out-of-pocket payments were gender, chronic illness, wealth index, risky behaviour, distance to a health facility, fever and diarrhoea. Finally, predictors of self-assessed health were gender, age, education, chronic illness, physical activity, wealth index, risky behaviour, and dummies for sufferers of fever, cold/cough and diarrhoea.
These findings have some relevant policy implications. The study recommends enhanced public education due to the positive effect of education on health insurance enrolment and healthcare utilisation. Even though enrolment in NHIS has increased over the years, for Ghana to achieve universal health coverage as enshrined in the Sustainable Development Goals, concerted efforts are needed to educate the populace about why they should enrol in the scheme. Continuous education should be embarked upon by organisations such as the Information Services Department (ISD), National Commission on Civic Education (NCCE), and the national broadcaster that is Ghana Broadcasting Corporation (GBC) to encourage many households to enrol in the scheme. Moreover, the various public relations officers in the districts should intensify public education and sensitisation drives on the scheme’s benefits, particularly in rural areas.
Table A1. Baseline regression results
Table
Acknowledgements
The author is grateful to both the Economic Growth Centre at Yale University and the Institute of Statistical, Social, and Economic Research (ISSER) at the University of Ghana for the permission to use the Ghana Socioeconomic Panel Survey dataset.
Disclosure statement
No potential conflict of interest was reported by the author(s).
Additional information
Funding
Notes on contributors
Samuel Sekyi
Samuel Sekyi graduated from the University of South Africa with a PhD in Economics. He is a Senior Lecturer at the Department of Economics, Simon Diedong Dombo University of Business and Integrated Development Studies, Ghana. His main areas of expertise and interest are Microeconometrics, health economics, development economics and agricultural economics. His recent research assessed the contextual dynamics of Ghana’s National Health Insurance Scheme, where he provided rigorous methodological alternatives to healthcare utilisation, financial protection and health status analyses. This current study makes a valuable contribution to the body of knowledge in healthcare decisions by jointly modelling health insurance, healthcare utilisation, healthcare expenditure and health status within the Conditional Mixed Process framework.
Notes
1. See details in Roodman (Citation2011) as the paper extensively treats how to do this.
References
- Aregbeshola, B. S., & Khan, S. M. (2020). Out-of-pocket health-care spending and its determinants among households in Nigeria: A national study. Journal of Public Health (Germany), 29(4), 931–16. https://doi.org/10.1007/s10389-020-01199-x
- Asfar, T., Ahmad, B., Rastam, S., Mulloli, T. P., Ward, K. D., & Maziak, W. (2007). Self-rated health and its determinants among adults in Syria: A model from the Middle East. BMC Public Health, 7(177), 1–9. https://doi.org/10.1186/1471-2458-7-177
- Awoke, M. A., Negin, J., Moller, J., Farell, P., Yawson, A. E., Biritwum, R. B., & Kowal, P. (2017). Predictors of public and private healthcare utilization and associated health system responsiveness among older adults in Ghana. Global Health Action, 10(1), 1301723. https://doi.org/10.1080/16549716.2017.1301723
- Baidin, V., Gerry, C. J., & Kaneva, M. (2021). How self-rated is self-rated health? Exploring the role of individual and institutional factors in reporting heterogeneity in Russia. Social Indicators Research, 155(2), 675–696. https://doi.org/10.1007/s11205-020-02604-4
- Bilgel, F., & Karahasan, B. C. (2018). Self-rated health and primary care utilization: Is selection into healthcare endogenously determined? The Economic Research Forum; The Economic Research Forum).
- Cameron, A. C., Trivedi, P. K., Milne, F., & Piggott, J. (1988). A microeconometric model of the demand for health care and health insurance in Australia. The Review of Economic Studies, 55(1), 85. https://doi.org/10.2307/2297531
- Dake, F. A. A. (2018). Examining equity in health insurance coverage: An analysis of Ghana’s national health insurance scheme. International Journal for Equity in Health, 17(1), 1–10. https://doi.org/10.1186/s12939-018-0793-1
- Duku, S. K. O. (2018). Differences in the determinants of health insurance enrolment among working-age adults in two regions in Ghana. BMC Health Services Research, 18(384). https://doi.org/10.1186/s12913-018-3192-9
- Du, J., Yang, X., Chen, M., & Wang, Z. (2019). Socioeconomic determinants of out-of-pocket pharmaceutical expenditure among middle-aged and elderly adults based on the China Health and Retirement Longitudinal Survey. BMJ Open, 9(7), 1–13. https://doi.org/10.1136/bmjopen-2018-024936
- Ekman, B. (2007). The impact of health insurance on outpatient utilization and expenditure: Evidence from one middle-income country using national household survey data. Health Research Policy and Systems, 5(6). https://doi.org/10.1186/1478-4505-5-6
- Fan, H., Yan, Q., Coyte, P. C., & Yu, W. (2019). Does public health insurance coverage lead to better health outcomes? Evidence from Chinese adults. INQUIRY: The Journal of Health Care Organization, Provision, and Financing, 56, 1–10. https://doi.org/10.1177/0046958019842000
- Franks, P., Gold, M. R., & Fiscella, K. (2003). Sociodemographics, self-rated health, and mortality in the US. Social Science & Medicine, 56(12), 2505–2514. https://doi.org/10.1016/S0277-9536(02)00281-2
- Ghosh, S., & Gupta, N. D. (2017). Targeting and effects of Rashtriya Swasthya Bima Yojana on access to care and financial protection. Economic and Political Weekly, 52(4), 61–70. https://www.epw.in/journal/2017/4/special-articles/targeting-and-effects-rashtriya-swasthya-bima-yojana-access-care-and
- Grossman, M. (1972). On the concept of health capital and the demand for health. Journal of Political Economy, 80(2), 223–255. https://doi.org/10.1086/259880
- Grustam, A., Vranes, A. J., Soldatovic, I., Stojicic, P., & Andersen, Z. J. (2020). Factors associated with utilization of primary and specialist healthcare services by elderly cardiovascular patients in the Republic of Serbia: A cross-sectional study from the national health survey 2013. International Journal of Environmental Research and Public Health, 17(7), 1–14. https://doi.org/10.3390/ijerph17072602
- Han-Kim, K., & Lee, M. (2016). Factors associated with health services utilization between the years 2010 and 2012 in Korea: Using Andersen’s Behavioral model. Osong Public Health and Research Perspectives, 7(1), 18–25. https://doi.org/10.1016/j.phrp.2015.11.007
- Jewell, R. T. (2009). Demand for prenatal health care in South America. Applied Economics, 41(4), 469–479. https://doi.org/10.1080/00036840701604396
- Kimani, J. K., Ettarh, R., Kyobutungi, C., Mberu, B., & Muindi, K. (2012). Determinants for participation in a public health insurance program among residents of urban slums in Nairobi, Kenya: Results from a cross-sectional survey. BMC Health Services Research, 12(1), 1–11. https://doi.org/10.1186/1472-6963-12-66
- Kusi, A., Enemark, U., Hansen, K. S., & Asante, F. A. (2015). Refusal to enrol in Ghana’s national health insurance scheme: Is affordability the problem? International Journal for Equity in Health, 14(1), 1–14. https://doi.org/10.1186/s12939-014-0130-2
- Lu, C., Chin, B., Lewandowski, J. L., Basinga, P., Hirschhorn, L. R., Hill, K., Murray, M., & Binagwaho, A. (2012). Towards universal health coverage: An evaluation of Rwanda Mutuelles in its first eight years. PLoS ONE, 7(6). https://doi.org/10.1371/journal.pone.0039282
- Malik, A. M., Syed, S. I. A., & Hammarström, A. (2012). Socio-economic determinants of household out-of-pocket payments on healthcare in Pakistan. International Journal for Equity in Health, 11(15), 1–7. https://doi.org/10.1186/1475-9276-11-1
- Manning, W., Newhouse, J., Duan, N., Keeler, E., Leibowitz, A., & Marquis, M. (1987). Health insurance and the demand for medical care: Evidence from a randomized experiment. American Economic Review, 77(3), 251–277. https://www.jstor.org/stable/1804094
- Masiye, F., & Kaonga, O. (2016). Determinants of healthcare utilisation and out-of-pocket payments in the context of free public primary healthcare in Zambia. International Journal of Health Policy and Management, 5(12), 693–703. https://doi.org/10.15171/ijhpm.2016.65
- Mwami, M. N., & Oleche, M. O. (2017). Determinants of utilization of health care services in Kenya. International Journal of Academic Research in Business and Social Sciences, 7(10), 132–156. https://doi.org/10.6007/ijarbss/v7-i10/3367
- Nakovics, M. I., Brenner, S., Bongololo, G., Chinkhumba, J., Kalmus, O., Leppert, G., & De Allegri, M. (2020). Determinants of healthcare seeking and out-of-pocket expenditures in a ‘free’ healthcare system: Evidence from rural Malawi. Health Economics Review, 10(1), 1–12. https://doi.org/10.1186/s13561-020-00271-2
- Newhouse, J. P. (1993). Free for all? Lessons from the Rand Health Insurance Experiment. Harvard University Press. https://doi.org/10.7249/CB199
- Nguyen, H. T. H., Rajkotia, Y., & Wang, H. (2011). The financial protection effect of Ghana National Health Insurance Scheme: Evidence from a study in two rural districts. International Journal for Equity in Health, 10(4), 4. https://doi.org/10.1186/1475-9276-10-4
- Nsiah-Boateng, E., Prah, J. R., & Nonvignon, J. (2019). Is enrolment in the national health insurance scheme in Ghana pro-poor? Evidence from the Ghana Living Standards Survey. BMJ Open, 9(7), 1–8. https://doi.org/10.1136/bmjopen-2019-029419
- O’Connor, G. E. (2015). The impact of insurance coverage on consumer utilization of health services: An exploratory study. International Journal of Bank Marketing, 33(3), 276–297. https://doi.org/10.1108/IJBM-05-2014-0061
- Rezayatmand, R., Pavlova, M., & Groot, W. (2017). Patient payment and unhealthy behavior: A comparison across European countries. BioMed Research International, 2017, 1–13. https://doi.org/10.1155/2017/2615105
- Roodman, D. (2011). Fitting fully observed recursive mixed-process models with cmp. The Stata Journal, 11(2), 159–206. https://doi.org/10.1177/1536867X1101100202
- Salari, P., Akweongo, P., Aikins, M., & Tediosi, F. (2019). Determinants of health insurance enrolment in Ghana: Evidence from three national household surveys. Health Policy and Planning, 34(8), 582–594. https://doi.org/10.1093/heapol/czz079
- Sarpong, N., Loag, W., Fobil, J., Meyer, C. G., Schwarz, N. G., Schwarz, N. G., & Schwarz, N. G. (2010). National health insurance coverage and socio-economic status in a rural district of Ghana. Tropical Medicine and International Health, 15(2), 191–197. https://doi.org/10.1111/j.1365-3156.2009.02439.x
- Sedefoğlu, G., & Soytaş, M. A. (2019). Self-reported health status: A microeconometric analysis for Turkey. Metody Ilościowe W Badaniach Ekonomicznych, 19(4), 440–451. https://orcid.org/10000-0002-7012-184X
- Sekyi, S., Aglobitse, P. B., & Addai-Asante, J. (2015). Enrolment on health insurance scheme in Ghana: Evidence from Mfantseman Municipality. Ghana Journal of Development Studies, 12(1), 53–70. https://doi.org/10.4314/gjds.v12i1-2.4
- Sekyi, S., & Domanban, P. B. (2012). The effects of health insurance on outpatient utilization and healthcare expenditure in Ghana. International Journal of Humanities and Social Science, 2(10), 40–49. https://citeseerx.ist.psu.edu/viewdoc/download?
- Sekyi, S., Domanban, P. B., & Agbenyo, F. (2022). Exploring heterogeneity of national health insurance scheme enrolment among persons in the informal sector. International Journal of Health Planning and Management. Advance online publication https://doi.org/10.1002/hpm.3557.
- Su, T. T., Pokhrel, S., Gbangou, A., & Flessa, S. (2006). Determinants of household health expenditure on western institutional health care. European Journal of Health Economics, 7(3), 199–207. https://doi.org/10.1007/s10198-006-0354-1
- Ta, Y., Zhu, Y., & Fu, H. (2020). Trends in access to health services, financial protection and satisfaction between 2010 and 2016: Has China achieved the goals of its health system reform? Social Science & Medicine, 245, 112715. https://doi.org/10.1016/j.socscimed.2019.112715
- Todorova, I. L. G., Tucker, K. L., Jimenez, M. P., Lincoln, A. K., Arevalo, S., & Falcón, L. M. (2013). Determinants of self-rated health and the role of acculturation: Implications for health inequalities. Ethnicity & Health, 18(6), 563–585. https://doi.org/10.1080/13557858.2013.771147
- Vals, K., Kiivet, R. A., & Leinsalu, M. (2013). Alcohol consumption, smoking and overweight as a burden for health care services utilization: A cross-sectional study in Estonia. BMC Public Health, 13(772). https://doi.org/10.1186/1471-2458-13-772
- Wielen, N., Van Der, Channon, A. A., & Falkingham, J. (2018). Universal health coverage in the context of population ageing: What determines health insurance enrolment in rural Ghana? BMC Public Health, 18(657), 1–13. https://doi.org/10.1186/s12889-018-5534-2
- Wingard, D. L., Cohn, B. A., Kaplan, G. A., Cirillo, P. M., & Cohen, R. D. (1989). Sex differentials in morbidity and mortality risks examined by age and cause in the same cohort. American Journal of Epidemiology, 130(3), 601–610. https://doi.org/10.1093/oxfordjournals.aje.a115374
- Woldemichael, A., Gurara, D., & Shimeles, A. (2019). The impact of community based health insurance schemes on out-of-pocket healthcare spending: Evidence from Rwanda. In IMF Working Papers WP/19/38 (WP/19/38; Strategy, Policy and Review Department). https://doi.org/10.5089/9781484398074.001
- Zeng, W., Lannes, L., & Mutasa, R. (2018). Utilization of health care and burden of out-of-pocket health expenditure in Zimbabwe: Results from a national household survey. Health Systems and Reform, 4(4), 300–312. https://doi.org/10.1080/23288604.2018.1513264