Abstract
Practices that introduce systematic bias are common in most scientific disciplines, including toxicology. Selective reporting of results and publication bias are two of the most prevalent sources of bias and lead to unreliable scientific claims. Preregistration and Registered Reports are recent developments that aim to counteract systematic bias and allow other scientists to transparently evaluate how severely a claim has been tested. We review metascientific research confirming that preregistration and Registered Reports achieve their goals, and have additional benefits, such as improving the quality of studies. We then reflect on criticisms of preregistration. Beyond the valid concern that the mere presence of a preregistration may be mindlessly used as a proxy for high quality, we identify conflicting viewpoints, several misunderstandings, and a general lack of empirical support for the criticisms that have been raised. We conclude with general recommendations to increase the quality and practice of preregistration.
“A man once pointed to a small target chalked upon a door, the target having a bullet hole through the centre of it, and surprised some spectators by declaring that he had fired that shot from an old fowling piece at a distance of a hundred yards. His statement was true enough, but he suppressed a rather important fact. The shot had really been aimed in a general way at the barn door, and had hit it; the target was afterwards chalked round the spot where the bullet struck. A deception analogous to this is, I think, often practiced unconsciously in other matters.”
—Venn, 1866, p. 259.
For as long as data have been used to support scientific claims, people have tried to selectively present them in line with what they wish to be true. Francis Bacon observed in Novum Organum (1620) that: “Once a human intellect has adopted an opinion (either as something it likes or as something generally accepted), it draws everything else in to confirm and support it“. In his book ‘On the Decline of Science in England: And on Some of its Causes’ from 1830, Charles Babbage lamented scientists engaging in hoaxing and forging (i.e., data fabrication), and trimming and cooking (i.e., flexibly analyzing data to get the results they want). Many scientists, operating within the reward structures of the scientific enterprise, want specific theories or facts to be true, or prefer some results over others (Barber Citation1961; Mitroff Citation1974), which can introduce systematic bias in the research process. Popper (Citation1962, 64) referred to this desire as dogmatic thinking: “we expect regularities everywhere and attempt to find them even where there are none; events which do not yield to these attempts we are inclined to treat as a kind of ‘background noise’; and we stick to our expectations even when they are inadequate and we ought to accept defeat”. While all methodological procedures in science have some probability of generating erroneous claims, systematic bias non-transparently increases this probability.
At the same time, the expectations and desires of scientists form an important source of motivation to pursue scientific discoveries, as even Popper admitted (Citation1962, 420): “The dogmatic attitude of sticking to a theory as long as possible is of considerable significance. Without it we could never find out what is in a theory—we should give the theory up before we had a real opportunity of finding out its strength”. To prevent systematic bias from influencing the claims scientists make in their pursuit of new discoveries, several methodological procedures have been developed that hold bias in check. One of those methodological procedures is to severely test claims (Lakens Citation2019; Mayo Citation2018; Popper Citation1959). Performing a severe test means that scientists design studies that have a high probability to corroborate a prediction when it is right, and a high probability to falsify a prediction when it is wrong. If scientists selectively present test results in line with their predictions, and disregard results not in line with their predictions, they are chipping away at the severity of their tests by introducing systematic bias in the claims they make.
In this manuscript we will provide a state-of-the-art overview of two related solutions to reduce the negative impact of systematic bias on scientific claims: preregistration and Registered Reports. Preregistration aims to make the claim-making process more transparent. It enables peers to evaluate whether and to which extent claims were impacted by bias. Registered Reports also reduce publication bias, mitigating current problems where published results are not representative of all studies performed. Both practices help scientists to move towards a scientific practice where claims are severely tested: The tests we perform will prove us right when we are right, and wrong when we are wrong. We discuss when and why researchers should implement preregistration and Registered Reports with the aim to make our science more efficient and reliable.
Systematic bias
Systematic bias can occur throughout the research process, for example when deciding which alternative theories to test, which measures to use, which statistical tests to report (i.e., selective reporting), or which studies to submit to scientific journals (i.e., publication bias). For example, researchers might plan to perform a two-sided test to analyse the effect of fish oil as a protecting factor against air pollutants. Upon observing a p value of 0.08 they decide to perform a one-sided test to get a p value below 0.05. Opportunistically switching from two-sided to one-sided tests doubles the Type 1 error rate (the long-run probability of claiming there is an effect when there is no effect in the population) from 5% to 10%. This is one example of a research practice that reduces the severity of statistical tests, as it decreases the probability of falsifying a prediction that is wrong. A prediction is severely tested if there is a low probability that the result will support a wrong prediction or fail to support a correct prediction. The severity of a test can be impacted by theoretical decisions (e.g., making a vague or unfalsifiable prediction), methodological decisions (e.g., using an invalid measure), and statistical decisions (e.g., inflating Type 1 error rates).
In the context of opportunistically switching from a two-sided to a one-sided test, Bakan (Citation1966, 431) suggested creating a “central registry in which one registers one’s decision to run a one- or two-tailed test before collecting the data”. This idea is now known as preregistration, and its goal is to improve the ability of peers to criticize all choices related to statistical tests. Historically, it has been difficult for peers to criticize the decisions that researchers make when performing a study because a transparent overview of their decisions was lacking. This means it was difficult to critically examine the possible impact of systematic bias on the claims made.
Evaluating the presence of systematic bias
Preregistration and Registered Reports have the goal to make decisions more transparent, especially if those decisions might be biased by the data and the statistical results. Depending on how preregistration is organised, this practice can have additional benefits, such as making studies known to potential participants or other scientists, and preventing publication bias (Dickersin and Rennie Citation2003). We define preregistration as a complete description of all information related to planned analyses (including the experimental design, measures, data preprocessing, and when statistical tests will corroborate or falsify predictions) that is demonstrably created without access to the data that will be analysed. There are subtle but important differences in how different proponents of preregistration justify its use (Uygun-Tunç, Tunç, and Eper Citation2021). Our view is based on the idea that the goal of preregistration is to allow peers to transparently evaluate the presence of systematic bias that might reduce the severity of the test (Lakens Citation2019). The problem with the sharpshooter fallacy – where the target is drawn after seeing where the bullet has hit the barn door – is that there is no way for the procedure to reveal that the shooter was a bad shot. The shooter might be an actual sharpshooter, but the methodological procedure used is not able to rule out the possibility that they are not. The claim of the sharpshooter might be true, but it has not been severely tested.
Systematic bias can be introduced more or less intentionally. Scientists can opportunistically engage in practices that increase the probability of confirming their predictions at the expense of being proven wrong. John, Loewenstein, and Prelec (Citation2012) used the term ‘questionable research practices’ to describe the opportunistic misuse of flexibility in the data collection and analysis to be able to make certain scientific claims, without disclosing that these practices were used. However, as many of these practices are not at all questionable, but simply bad research practices, we refer to them as practices that introduce systematic bias. Examples include creating the hypothesis after the results are known (HARKing, Kerr Citation1998), performing multiple hypothesis tests without transparently reporting all results, or optional stopping (i.e., repeatedly analysing data as they come in without correcting the alpha level for multiple comparisons (Lakens Citation2014)). However, not all bias is introduced intentionally. Researchers might engage in practices without realising they are introducing bias, or if sufficient time passes between hypothesis generation and data analysis, researchers might simply forget what they predicted after observing an interesting pattern in their data (Nosek et al. Citation2018). Although the focus in preregistration is often on false positive results, systematic bias can also increase the probability of not finding support for a prediction if this is the desired outcome, thus increasing the probability of false negative results (i.e., Type 2 errors). Systematic bias does not only affect the error rates of scientific claims, but also estimates based on the data, such as effect sizes (Hedges Citation1984).
The easiest way to allow peers to evaluate the possible presence of systematic bias is to preregister the procedure and statistical analysis plan before the data are generated. It is also possible to preregister a planned analysis when performing secondary data analysis, where data have already been collected by someone else (e.g., https://bigcitieshealthdata.org/ or https://healthdata.gov/). Here, researchers will need to demonstrate that they did not have access to the data when they completed the preregistration, or alternatively, that they did not analyse the data even if they had access to it (Mertens and Krypotos Citation2019). Some researchers have suggested that the term preregistration can also be used when researchers share a time-stamped statistical analysis plan of a dataset that they have access to, and therefore could have used to develop their analysis plan (Weston et al. Citation2019). The argument is that scientists always need to trust their peers to some extent, so we might as well trust them when they declare that they did not use the data to create their analysis plan. Although it is true that in practice trust always plays a role in science (Hardwig Citation1991), we do not consider a time-stamped analysis plan a valid preregistration unless authors can demonstrate they created the analysis plan without access to the data. When data already exist, preregistration is only possible when access to the data are restricted. An example is the UK Biobank where researchers need to apply for access to the data, and it is in principle possible to confirm data were not accessed before the preregistration was created. The more important it is that data provenance (when and where data were created) is trustworthy, the more researchers need to rely on electronic data capture systems, data notary services (Kleinaki et al. Citation2018), or independent observers that audit the data collection.
Our definition restricts the use of the term ‘preregistration’ to a narrower subset of situations than is conventional. We believe this is warranted because current practice has already started to highlight the difficulty of a less restrictive definition of preregistration. Furthermore, the definition of preregistration has already become more restrictive over time. For example, the American Psychological Association (APA) implements badges for articles that have preregistered studies. They distinguish a ‘preregistration’ badge from a ‘preregistration+’ badge, where the latter includes an analysis plan. Currently, the convention has already changed to only treat a study as preregistered when it contains an analysis plan, and Evidence-Based Toxicology also expects preregistrations to include an analysis plan (Mellor, Corker, and Whaley Citation2024). Therefore, only the ‘preregistration+’ badge signals what most researchers would nowadays consider a preregistration.
Our definition restricts the use of preregistration to those studies where researchers can demonstrate that they did not have access to the data they will use to test their predictions. The APA already acknowledges that preregistrations based on existing data differ meaningfully from those created without access to the data. Whenever data already exist ‘the notation DE (Data Exist) will be added to the badge, indicating that registration postdates realization of the outcomes but predates analysis’ (American Psychological Association Citation2023). Similarly, Peer Community In – Registered Reports (PCI-RR), a platform that publishes peer-reviews of preprints, acknowledges distinct levels of bias-control depending on whether authors had access to the data when they preregistered or not (Peer Community In – Registered Reports Citationn.d.). They distinguish level 6 (where data do not yet exist) and level 5 (where data exist in an access-controlled data repository and researchers can demonstrate they did not access the data) from lower levels of bias control where authors cannot demonstrate they did not have access to the data when preregistering. Following our definition, preregistration should enable peers to transparently evaluate the severity of a test, which is not possible if the data were known to the researchers when they created their analysis plan. The strict definition of preregistration that we propose might deviate from current conventions, but this comes at the benefit of greater conceptual clarity and less confusion about how preregistration should be implemented in practice. Finally, we strongly feel that scientific practices should be justified based on principles grounded in a philosophy of science.
We propose that the ‘open lab notebook’ terminology is more appropriate for studies where data already exist. When implementing an open lab notebook researchers can log – in real time – when they first accessed data and whether and how access to the data informed their analysis plan. Preregistration and an open lab notebook approach also differ in how they control the probability of misleading claims. Preregistration focusses on severe tests through statistical error control. Open lab notebooks focus on robustness analyses, sensitivity analyses, and triangulation to provide support for claims (Munafò and Smith Citation2018). We recommend that researchers who have preregistered still maintain an open lab notebook. There can be good reasons why researchers need to deviate from their preregistration (Lakens Citation2024). When the main claims in a paper are based on analyses that deviated from the preregistration after seeing the data, the study should no longer be considered preregistered, but as an open lab notebook study.
How biased is the scientific literature?
It is practically impossible to empirically establish how much systematic bias has inflated error rates in published articles (Lakens Citation2015; Ofosu and Posner Citation2023). To quantify the inflation of Type 1 or 2 error rates, we would need to know not just which decisions researchers made while analyzing their data, but also which decisions they would have made if their analyses had yielded different results. Given the inherent uncertainty about how biased the scientific literature is, it is important to reflect on the question whether the amount of bias in the scientific literature is substantial enough to warrant the widespread implementation of preregistration.
At one end of the spectrum, it is possible that systematic bias plays no role at all. In this context researchers are likely to use standardized measures and they cannot opportunistically manipulate the number of obtained observations; data preprocessing pipelines are identical across studies, and there is no flexibility in how the data are analyzed because, for example, the theoretical hypothesis is so precise that it corresponds to only one statistical hypothesis. In such research areas, researchers can easily demonstrate the absence of systematic bias in the literature through meta-analytic bias-detection techniques (Carter et al. Citation2019), and by conducting high-powered replication studies which should yield a high success rate. At the other end of the spectrum, it is possible that a research community examines an effect that does not exist but still generates a large number of false positives purely due to systematic bias. Most research areas will fall somewhere between these two extremes.
Over the last decade, the field of metascience has examined whether scientists engage in research practices that introduce systematic bias. We identified 18 surveys across a range of scientific disciplines that examined a similar set of questions, which revealed that many researchers admit engaging in practices that reduce the severity with which scientific claims have been tested. An example of such practices is data peeking and optional stopping, where researchers collect data, analyze the results, and if the desired results are not observed, collect additional data. Unlike sequential analysis, where the Type 1 error rate is carefully controlled as incoming data are analyzed (Lakens Citation2014; Wassmer and Brannath Citation2016), data peeking and optional stopping inflate the Type 1 error rate. Another example of a practice that inflates error rates is when researchers perform multiple variations of the same hypothesis test, for example by excluding some datapoints, or adding covariates (Lenz and Sahn Citation2021), until the test is statistically significant, and selectively report those test that support their predictions. As a final example, some scientists admit engaging in the practice known as hypothesizing after results are known, or HARKing (Kerr Citation1998), where they pretend data are used to test a hypothesis, while in reality the data were used to generate the hypothesis. Unfortunately toxicology research is not immune to these and other biased practices, as was already highlighted about 15 years ago by Wandall, Hansson, and Rudén (Citation2007). What all these practices have in common is that they reduce the severity of statistical tests. By only reporting tests that support predictions, researchers can make it look like there is clear support for a theory, when in reality this claim does not follow from all tests that are performed. Even though these problems have been pointed out for a long time (Babbage Citation1830), scientists are often not aware of the problematic nature of these practices, and sometimes they are even informally trained to engage in these practices without disclosing them. Worryingly, sometimes peer reviewers or editors ask authors to perform these practices (Köhler et al. Citation2020; Ross and Heggestad Citation2020).
Researchers indicate that they only use these practices in a subset of all studies they perform (Fiedler and Schwarz Citation2016; Isbell et al. Citation2022). In the largest study to date, the self-admitted percentage of times researchers used these practices was approximately three times lower than the percentage of researchers who engaged in a practice at least once (Schneider et al. Citation2023). summarizes the prevalence of researchers admitting to conducting research practices that reduce the severity of tests (Agnoli et al. Citation2017; Artino, Driessen, and Maggio Citation2019; B. N. Bakker et al. Citation2021; Brachem et al. Citation2022; Chin et al. Citation2021; Fiedler and Schwarz Citation2016; Fraser et al. Citation2018; Garcia-Garzon et al. Citation2022; Isbell et al. Citation2022; Janke, Daumiller, and Rudert Citation2019; John, Loewenstein, and Prelec Citation2012; Latan et al. Citation2021; Makel et al. Citation2021; Moran et al. Citation2022; Motyl et al. Citation2017; Rabelo et al. Citation2020; Schneider et al. Citation2023; Swift et al. Citation2022). It is important to point out that the percentages presented here do not directly translate into the percentage of researchers who are engaging in these practices. This is due to the inherent potential for desirable response and survey bias, low response rates across studies, a difficulty of comparing self-admission rates and estimates of the prevalence among colleagues, diverse populations, differences in the questionnaires used, variations in scoring methods (dichotomous yes/no responses, Likert, percentages), and variability in how participants interpreted the questions (Motyl et al. Citation2017). Even though it is difficult to empirically quantify the impact of these practices on knowledge generation, there are strong indications that these practices can lead to unreliable scientific claims in some research areas (e.g., Vohs et al. Citation2021). More information on the selected studies, as well as the data and code used to generate , is available in the Supplementary Material at: https://doi.org/10.17605/osf.io/xcu4m.
The costs and benefits of preregistration
The research required to accurately quantify the costs and benefits of preregistration will likely never be conducted. Yet, this is not something to worry about because the reason to preregister hypothesis tests is based on a normative argument, not on a cost-benefit analysis. Although several papers discuss the costs and benefits of preregistration (Logg and Dorison Citation2021; Sarafoglou et al. Citation2022; Simmons, Nelson, and Simonsohn Citation2021), it is impossible to provide a realistic cost-benefit analysis of introducing the practice of preregistering hypothesis tests in a scientific discipline. There is too much uncertainty about how scientists analyse their data when they do not preregister to quantify the inflated Type 1 error rate. Crucial parameters, such as the percentage of studies that examine true hypotheses, whether we can detect practices that create systematic bias, and how successful self-correction in science is, are unknown (Kerr Citation1998). Furthermore, it is difficult to know how to weigh costs and benefits of preregistration. For example, researchers report that a perceived disadvantage of preregistration is that it increases the project duration, but they also indicate it leads to a more carefully thought through research hypothesis, experimental design, and statistical analysis (Sarafoglou et al. Citation2022; Toth et al. Citation2021). The effect of this trade-off on the careers of scientists (e.g., the number and impact of scientific papers that are published) is difficult to quantify. An additional complexity is that the best implementation of preregistration is within the Registered Report publication format (as discussed below), which entails additional benefits that cannot be disentangled from preregistration in practice.
In the end, researchers should not preregister because it has become fashionable, is perceived as virtuous, or because the benefits are greater than the costs, but because it helps them to achieve what they believe is the goal of science. Preregistration is a methodological procedure that, given a specific philosophy of science (i.e., Popper’s methodological falsificationism), improves one part of the research process – the evaluation of the test severity of hypothesis tests. Both preregistration and Registered Reports are practices that are aligned with methodological falsificationism in the sense that they formalize procedures that make it harder for researchers to prove their hypotheses right when they are actually wrong. This increases the falsifiability of predictions. From a methodological falsificationist philosophy of science, the role of hypothesis testing is to critically evaluate the predictive success of models. Therefore, researchers who test hypotheses from a methodological falsificationist approach to science should preregister their studies if they want a science that has intersubjectively established severely tested claims. By preregistering hypothesis tests, their predictive success can be evaluated by peers because the severity of the hypothesis test is transparently communicated. This will not necessarily remove systematic bias, but it will make it visible to peers, who can now criticize decisions researchers have made (for a real-life example, see this interaction by Flournoy, et al. (Citation2021) and Ellwood-Lowe, Foushee, and Srinivasan (Citation2021)).
From a methodological falsificationist philosophy there is no logically coherent reason to preregister non-hypothesis testing studies such as exploratory research (Dirnagl Citation2020), secondary data analysis when researchers already had access to the data (Weston et al. Citation2019), or qualitative research (Haven and Van Grootel Citation2019). It is beneficial to carefully plan studies, but study planning can be done without subsequently creating a time-stamped document that contains a preregistered statistical analysis plan. It is also beneficial to carefully log all deviations from a plan as the research progresses, but this can be done in an open lab notebook. Features of platforms such as GitLab or GitHub (Scroggie et al. Citation2023) and Zenodo (Schapira et al. Citation2019) make them ideal tools to host these open lab notebooks. These platforms allow users to track all the information about who did what and when, thereby allowing full transparency and real-time sharing of any decisions made during the research process. There is a risk that conceptually stretching (Sartori Citation1970) the concept of preregistration to non-hypothesis testing research (instead of using more appropriate terminology such as study planning or open lab notebooks) will create confusion and hinder the uptake of preregistration where it should be implemented. Although we do not believe preregistration of exploratory research, secondary data analysis when researchers have access to the data, or qualitative research is necessary, we strongly recommend careful study planning and open lab notebooks for all research. Our strict interpretation of the goal of preregistration is based on the belief that preregistration should not become a mindless ritual, but should be justified from a set of basic principles. Researchers aiming to prevent systematic bias in qualitative research, exploratory studies, or secondary data analysis need to rely on other tools than preregistration, such as robustness checks, sensitivity analyses, triangulation, open lab notebooks, blind analyses, and independent direct replication.
Publication bias
A second major source of bias in the scientific literature is publication bias (Dickersin, Min, and Meinert Citation1992; Ensinck and Lakens Citation2023; Franco, Malhotra, and Simonovits Citation2014; Greenwald Citation1975), which typically means that significant results are more likely to be published than non-significant results. Publication bias also affect the discipline of Toxicology, where the percentage of positive results increased from approximately 50 to 90% in the period 1991–2007 (Fanelli Citation2012). However, for some research questions it could be that non-significant results are the desired outcome, and therefore they are subjected to publication bias. For example, some stakeholders may want to show that lead levels in drinking water are so small that the water is safe to drink (i.e., there is no negative effect on people’s health), even though lead is an established neurotoxicant.
One consequence of publication bias is that effect size estimates based on the published literature are systematically biased (Hedges Citation1984). The removal of non-significant results inflates effect sizes. Non-significant results will have effect size estimates closer to zero, and if meta-analysts have no access to these smaller effect sizes, the meta-analytic effect size estimate will be upwardly biased. In the worst-case scenario, all effects in the literature are Type 1 errors, and the true effect size is zero. Sterling (Citation1959, 30) already noted how publication bias means that the number of false positives in the literature may be much larger than 5% due to selective publication: “Significant results published in these fields are seldom verified by independent replication. The possibility thus arises that the literature of such a field consists in substantial part of false conclusions resulting from errors of the first kind in statistical tests of significance”. This is referred to as the false positive report probability, or the probability that a scientific claim is false in a literature that suffers from publication bias (Wacholder et al. Citation2004). Publication bias and systematic bias are especially problematic when combined. In the worst-case scenario, the scientific literature is filled with dozens of Type 1 errors, while an unbiased large scale replication study yields an effect size estimate of zero (for an example, see Vohs et al. Citation2021). Publication bias arises both because researchers do not submit non-significant results for publication (Ensinck and Lakens Citation2023; Franco, Malhotra, and Simonovits Citation2014; Greenwald Citation1975), and because reviewers and editors prefer to accept significant results for publication (Mahoney Citation1977). Despite the fact that publication bias is widespread in academia, the general public believes it is immoral (Bottesini, Rhemtulla, and Vazire Citation2022; Pickett and Roche Citation2017).
Statistical methods exist to detect publication bias, but we can never fully correct for publication bias and retrieve an unbiased effect size from a biased literature (Carter et al. Citation2019). Publication bias techniques can at most, and under certain conditions, identify the presence of bias in the literature, and calculate what the effect size might be if a specific model of systematic bias is at play in a literature. If a literature has been tainted by publication bias only new unbiased studies can provide accurate effect size estimates. From a frequentist error-statistical approach to statistical inferences (Mayo Citation2018; Neyman and Pearson Citation1928), which has the goal to make scientific claims based on tests that control Type 1 and 2 error rates in the long run, researchers need access to all tests of a prediction to be able to evaluate whether the percentage of corroborated predictions approaches that of the Type 1 or the Type 2 error rate.
Study registries
To prevent publication bias, some fields have developed study registries (Laine et al. Citation2007). A study registry is a public searchable database of studies that are performed with a description of the goal of the study, the effect of interest, and the design and method. The initial goal of registries was to make it easier for patients to take part in clinical trials (Dickersin and Rennie Citation2003). In these registries, a description of the study and contact information was provided so that patients who might benefit from new treatments would be able to find the trials. Study registries can also make planned studies known to fellow researchers, which can prevent duplication of work and can increase collaboration in science. One example of a trial registry is clinicaltrials.gov, which was established in 1997, and promoted by the Food and Drug Administration (FDA). Initially, the uptake was slow because the use of a registry was voluntary. However, two triggering events increased the use of trial registries (Dickersin and Rennie Citation2012). First, Glaxo-SmithKline was sued in 2004 because the company neglected to share the results of trials that revealed harmful effects of an antidepressant (Lemmens Citation2004). Second, the International Committee of Medical Journal Editors announced in 2004 that preregistration would be a requirement for publication for all clinical trials, and wrote: “the ICMJE requires, and recommends, that all medical journal editors require registration of clinical trials in a public trials registry at or before the time of first patient enrollment as a condition of consideration for publication”. Subsequent regulations and policies of the National Institutes of Health (NIH) provided an important impetus for the widespread adoption of trial registration (Zarin et al. Citation2019).
From 2000 onwards, registries have increasingly been used to prevent bias, and regulations have become increasingly strict in terms of reporting both the primary outcome of studies before data collection, as well as updating the registry with the results after data collection is complete, although these rules are not always followed (Goldacre et al. Citation2018). As long as all researchers register their studies in a registry, and update the registry with the results, study registries can be an effective approach to combat publication bias.
Over time, study registries have increased the level of detail they require, moving in the direction of requiring a preregistration of the study design and analysis. When study registries require researchers to register a planned analysis, they combine a study registry with a preregistration (thereby also reducing opportunistic flexibility in the analysis). For example, the SPIRIT checklist (https://www.spirit-statement.org/) is essentially a template to preregister studies (Chan et al. Citation2013). So, even though initially study registries had a different goal than preregistration, in some research areas study registries have incorporated checklists that come close to a full preregistration. Therefore, study registries can increase transparency, in a similar way as preregistrations, and reduce publication bias in a similar way as Registered Reports.
Registered Reports
Another proposed solution to combat publication bias emerged in response to early metascientific studies documenting the presence of publication bias in psychology (Sterling Citation1959). An initial proposal by Rosenthal (Citation1966, 36) focused on results-blind review to reduce publication bias: “To accomplish this might require that procedures only be submitted initially for editorial review or that only the result-less section be sent to a referee or, at least, that an evaluation of the procedures be set down before the referee or editor reads the results”. Similarly, Walster and Cleary (Citation1970, 17) wrote: “There is a cardinal rule in experimental design that any decision regarding the treatment of data must be made prior to an inspection of the data. If this rule is extended to publication decisions, it follows that when an article is submitted to a journal for review, the data and the results should be withheld.” The first proposal to review a detailed study proposal and commit to publishing it before the data are collected was proposed by Johnson (Citation1975, 43) in the European Journal of Parapsychology:
“According to the philosophy of this model, the experimenter should define his problem, formulate his hypothesis, and outline his experiment, prior to commencing his study. He should write his manuscript, stating at least essential facts, before carrying out his investigation. This manuscript, in principle only lacking data in the tables, presentation of the results, and interpretation of the results, should be sent to one or more editors, and the experiment should not initiate his study until at least one of the editors has promised to publish the study, regardless of the outcome of the experiment. In this way we could avoid selective reporting. Furthermore, the experimenter will not be given the opportunity to change his hypotheses in such a way that they “fit” the outcome of the experiment”.
After the in-principle acceptance, researchers collect and/or analyze the data following the preregistered plan. Deviations are possible, but should be clearly documented, and the impact of the deviations on the severity and the validity of the test should be evaluated (Lakens Citation2024). If the deviations from the plan are substantial, the editor and reviewers might need to reevaluate the study. At the last step, the researchers submit the final manuscript for peer review. This is called Stage 2 review. Now that the manuscript includes the results and the conclusions reviewers check the extent to which the researchers followed their preregistration, evaluate the consequences of any deviations from the preregistration in terms of the severity and validity of the tests, and check whether the conclusions follow from the data. It is not possible that the manuscript is rejected after a negative result is observed, except under rare circumstances where quality checks indicate that methodological problems lead to an uninformative study. In addition to reducing publication bias and systematic bias. Registered Reports have the benefit of moving peer review forward in the research process. This makes it possible to incorporate feedback from peers in the study design, and improve any weaknesses identified by peers before the data are collected (Soderberg et al. Citation2021). Furthermore, you will know if the study will be accepted before doing most of the work (which is especially useful if the study requires substantial effort to collect the data). Similar to study registries, Register Reports combat both publication bias and systematic bias, but can be implemented for any single study, even if a field does not require all studies to be submitted to a registry.
A downside of Registered Reports is that the starting time of the data collection can’t be completely planned by the researcher, because researchers need to wait until the Stage 1 peer review is completed, and perhaps even until a revision is reviewed, before in-principle acceptance has been received. This limitation can be resolved through scheduled review. Researchers can indicate they will submit a Registered Report at a specific date. The editor will find reviewers and instruct them to schedule in the review process immediately after the scheduled submission date. This workflow (as for example implemented by Peer Community In Registered Reports) substantially speeds up the peer review process of Stage 1 reports. Note that Evidence Based Toxicology will publish Registered Reports that have been peer reviewed by PCI-RR.
The effectiveness of preregistration and Registered Reports
As preregistration and Registered Reports have become more popular across several scientific disciplines, it has become possible to metascientifically evaluate the extent to which they have improved research practices. It is difficult to provide empirical support for the hypothesis that preregistration and Registered Reports will lead to studies of higher quality. To test such a hypothesis, scientists should be randomly assigned to a control condition where studies are not preregistered, a condition where researchers are instructed to preregister all their research, and a condition where researchers have to publish all their work as a Registered Report. We would then follow the success of theories examined in each of these three conditions in an approach Meehl (Citation2004) calls cliometric metatheory by empirically examining which theories become ensconced, or sufficiently established that most scientists consider the theory as no longer in doubt. Because such a study is not feasible, causal claims about the effects of preregistration and Registered Reports on the quality of research are practically out of reach. To date, all metascientific research on the effects of Registered Reports and preregistration on the quality of research has been correlational.
When evaluating the benefits of preregistration and Registered Reports empirically, it is important to remember what the goal of preregistration and Registered Reports is to begin with. Preregistration, successfully implemented, should allow peers to transparently evaluate the severity of a test. The extent to which this benefit is achieved in practice greatly depends on how well researchers preregister. Registered Reports should, additionally, reduce publication bias. In addition to studying the benefits, it is important to examine potential negative side-effects of the practice to preregister studies.
Metascientific research has supported the prediction that due to more transparent reporting, preregistration increases the ability of peers to evaluate the severity of tests compared to non-preregistered studies (Ofosu and Posner Citation2023; Toth et al. Citation2021). Preregistration also allows peers to evaluate any deviations from the original plan such as changes to the analysis plan or preregistered hypothesis that otherwise go unnoticed (Toth et al. Citation2021; van den Akker et al. Citation2023). This improved transparency is often regarded as one of the positive aspects of preregistration (Spitzer and Mueller Citation2023; Toth et al. Citation2021) and clinical registries. For instance, Kaplan and Irvin (Citation2015) compared the proportion of clinical trials showing a benefit of the intervention before and after the imposition that clinical trials had to be registered in year 2000. They observed that before 2000, 17 of 30 trials (57%) showed a benefit of the intervention in comparison to only 2 of 25 trials (8%) after 2000. Although this result suggests that the proportion of positive results in trials declined after 2000, it is not possible to make any causal claim from the observed results as it was a retrospective observational study which only included a small number of trials (n = 55), and unmeasured confounders could have impacted the observed results.
At the same time, too often preregistrations include vague hypotheses, or completely omit important details of the methods, procedures, and analysis plan. This hinders the ability of peers to critically evaluate the preregistration and any deviations from it (Bakker et al. Citation2020, Citation2021; Brodeur et al. Citation2024; van den Akker et al. Citation2023). Despite these shortcomings in some preregistrations, it is important to point out that some studies are accompanied by a high-quality preregistration, and almost all preregistrations increase the ability of peers to evaluate the severity of tests compared to non-preregistered studies (Ofosu and Posner Citation2023; Toth et al. Citation2021). It should not be surprising that there is a long way to go before all researchers are able to complete high-quality preregistrations, as the process was also slow when registries were introduced in medical research (Qureshi, Gough, and Loudon Citation2022).
When it comes to Registered Reports, there is strong correlational evidence that more null results appear in the scientific literature compared to standard publication formats. Scheel et al. (Citation2021) showed that while 96% of traditional publications yielded significant results, only 44% of tested hypotheses in Registered Reports yielded significant results (see also Allen and Mehler Citation2019). This correlational study cannot tell us why more null results appear in the literature. One reason could be that researchers might primarily use Registered Reports when they have a high prior they will observe a null effect. A second reason could be that, with all things equal, without systematic bias much less than 96% of hypothesis tests would yield statistically significant results (Scheel et al. Citation2021). In fact, it seems plausible that researchers are less prone to p-hacking and cherry picking after obtaining “in-principle acceptance” for their Registered Reports.
A range of possible additional benefits and downsides of preregistration and Registered Reports have been examined. First, researchers have explored whether preregistered tests differ from non-preregistered tests with respect to either bias, or how often they yield significant results. The idea is that preregistration might reduce flexibility in the data analysis, but the findings are mixed. While Toth et al. (Citation2021) found that preregistered studies reported statistically significant result less often (48%) than non-preregistered studies (66%), van den Akker et al. (Citation2023) found a similar proportion of null results between preregistered and non-preregistered studies. A difference between these studies is that van den Akker coded multiple hypotheses per study of which almost half were not mentioned in the introduction or discussion, while Toth et al. (Citation2021) analyzed only hypotheses explicitly stated in the introduction and/or preregistration document. It is possible that the latter category is more at risk of systematic bias. Despite these differences, the effect – if any – seems small. In theory, knowing that peers may scrutinize one’s work should act as a safeguard against systematic bias, albeit most peers rarely read preregistrations (Spitzer and Mueller Citation2023). For instance, Brodeur et al. (Citation2024) found that preregistered randomized controlled trials with pre-specified analysis plans provided less indications of p-hacking in economics. Other studies have reported smaller effect sizes for preregistered studies than non-preregistered studies (Kvarven, Strømland, and Johannesson Citation2019; Schäfer and Schwarz Citation2019), but in both these studies the fact that most (or all) preregistered studies were replications introduces a confound that makes it impossible to draw conclusions about preregistration. As long as deviations from a preregistration are common, and many preregistrations are vague or incomplete, it is too early to be able to adequately test if preregistered studies yield less biased results.
A recent survey revealed that in economics, political science, and especially psychology, most researchers were supportive of preregistration (Ferguson et al. Citation2023). Interestingly, this study also showed that researchers underestimated how many of their peers were supportive of preregistration. Across disciplines, psychologists are more likely to preregister, with a noticeable increase over time in all disciplines (Brodeur et al. Citation2024; Ferguson et al. Citation2023; Logg and Dorison Citation2021; Simmons, Nelson, and Simonsohn Citation2021). In general, researchers are positive about preregistration, and indicate preregistration encourages them to think through the study, can improve collaboration between researchers, and signals the absence of systematic bias (Logg and Dorison Citation2021; Sarafoglou et al. Citation2022; Spitzer and Mueller Citation2023; Toth et al. Citation2021). Preregistration might provide an opportunity for researchers to identify gaps or flaws in their hypothesis, study design, or analysis plan that would otherwise have been encountered once the experiment was completed (Simmons, Nelson, and Simonsohn Citation2021). Preregistration can also serve as an opportunity to produce discussions between collaborators to refine methods and analysis plan, and ultimately, conduct a study of higher quality (Logg and Dorison Citation2021; Sarafoglou et al. Citation2022; Spitzer and Mueller Citation2023; van’t Veer and Giner-Sorolla Citation2016). Preregistered studies have also been associated with a higher prevalence of a priori power analyses (Bakker et al. Citation2020; van den Akker et al. Citation2023), sample size rationale, and clearly defined stopping rule (Toth et al. Citation2021). Although more research is needed, initial evidence suggests the quality of Registered Reports is judged to be higher than that of comparable traditional articles (Soderberg et al. Citation2021). Registered Reports had higher rigor of methods and analyses, and also showed better alignment between the question and methods, compared to manuscripts that were not Registered Reports. Although it is often argued that a downside of preregistration and Registered Reports is that they take more time than standard research (Logg and Dorison Citation2021; Sarafoglou et al. Citation2022; van’t Veer and Giner-Sorolla Citation2016), there is evidence that researchers perceive their studies as higher quality when preregistered, specifically with respect to the analysis plan, the hypotheses, and the study design (Sarafoglou et al. Citation2022). It might therefore be more accurate to state that a higher-quality study is a time-consuming task, and preregistering highlights aspects of a planned study that can be improved by investing more time.
Metascientific research has also shown that tensions exist between the idea that preregistration does not allow deviations from the prespecified plan, while researchers often need to deviate from their analysis plan (Toth et al. Citation2021; Spitzer and Mueller Citation2023). Researchers who state that their study was preregistered do not always transparently report deviations (Claesen et al. Citation2019; Ofosu and Posner Citation2023; Toth et al. Citation2021; Willroth and Atherton Citation2024). One reason might be that researchers perceive that deviations from their preregistration may result in a loss of credibility of their claims (Spitzer and Mueller Citation2023). However, editors consider certain deviations from a preregistration unproblematic, while not being transparent about deviations is evaluated negatively (Willroth and Atherton Citation2024), and not all deviations impact the severity of the test (Lakens Citation2024). However, deviating from the preregistration can increase the possibility of a Type 1 error in other cases, thereby reducing the severity of the test. For example, imagine a researcher who performs a preregistered test that yields a non-significant result. The researcher did not preregister the exclusion of outliers (even though this is best practice) but observes several outliers in their data. They decide to exclude the outliers and re-run the test. The result is now statistically significant. This non-preregistered analysis might be more valid, but the possibility that this finding is a Type 1 error has increased.
Criticism of preregistration
Preregistration has seen a considerable uptake across many scientific disciplines. Any practice that disrupts the status quo will receive criticism, even though some believe points are too often “half-baked criticisms, raising issues that have already been fully addressed” (Syed Citation2024). The criticisms on preregistration fall into three categories. First, researchers argue preregistration is not needed to improve science. If this turns out to be valid, preregistration should be abandoned, as the goal scientists have can be achieved more efficiently. Second, preregistration might have unintended consequences. This is almost certainly true, but this is only problematic if there are no subsequent interventions to address undesired side-effects. The last category of criticisms is based on misunderstandings of preregistration. Sometimes these misunderstandings are due to the fact that many researchers do not provide a clear conceptual definition of preregistration when they discuss the topic (Lakens Citation2019), which leads to unproductive discussions that generate more heat than light. Other times researchers’ understanding of preregistration continues to develop over time, and ideas about preregistration from a decade ago might have been less clearly developed than they are today. It is worth pointing out that so far there has been no published criticisms of Registered Reports, as there is widespread acknowledgement that publication bias should be prevented, and Registered Reports have the additional benefit that Stage 1 peer review can help to improve the quality of preregistrations.
Is preregistration worth the effort?
Science should resist unnecessary bureaucratization, and if preregistration would have no benefits, it will only increase the workload. Although the argument for preregistration is primarily normative, and a true cost-benefit analysis is difficult to establish, some critics have argued that preregistration is outright unnecessary. One reason preregistration would not improve science is because people can identify systematic bias when they read a scientific article even without a preregistration. As Rubin (Citation2022) wrote: “Importantly, readers are able to identify deficiencies in the quality of a theoretical rationale, as presented in a research report, even if they are misled about when that rationale was developed. Hence, although flexible theorizing may occur during HARKing, readers can identify any associated low-quality theorizing and take this into account in their estimates of relative verisimilitude”. Similarly, researchers have argued that “statistical problems become irrelevant because theories, not random selection, dictate what comparisons are necessary” (Szollosi et al. Citation2020). The idea is that theories are (or should be) so strong that tests of their predictions have no meaningful flexibility. Authors who have raised this criticism on preregistration fail to provide any real-life examples of theories that sufficiently constrain how they can be tested, nor do they provide empirical support for their hypothesis that peers can identify systematic bias. It is difficult to think of theories that dictate all data-preprocessing steps, test assumptions, and statistical models to test a prediction. One might also argue that theories that have reached this level of predictive power no longer need to be tested. It is also unclear how we develop such theories without going through a phase where theories are still vague in their predictions.
Interestingly, other criticisms of preregistration raise the exact opposite point, and consider all theories too weak to make predictions to begin with. Pham and Oh (Citation2021, 168) argued that: “rather than advocating preregistration as a means to foster more falsification-oriented, confirmatory research, it may be more realistic and productive to simply acknowledge that most consumer research is largely exploratory, thus limiting the epistemological value of traditional falsificationism.” Similarly, in economics, Olken (Citation2015, 63) argues that the lack of resources means an ideal practice of exploratory studies followed by confirmatory studies is impossible, and states that: “Such constraints mean that most of these follow-up confirmatory trials will never be done, and the “exploratory” analysis is all the community will have to go on”.
Obviously, it cannot be both true that theories are so strong that they allow us to identify systematic bias, and completely absent so that we should treat all our research as exploratory. Regrettably, these different critics of preregistration do not engage with each other’s arguments, nor do they empirically examine whether their claims hold. We believe the truth is likely in the middle: Researchers are guided by some proto-theories that they use to derive predictions, but these theories are often not strong enough to prevent systematic bias. Of course, researchers who agree with these arguments are free to not preregister, and instead (1) transparently demonstrate the severity of the test based on an argument that the theory perfectly constrains how the hypothesis is tested, or (2) acknowledge that they did not severely test any claims, and present their results as input for future tests of predictions.
Another reason preregistration would not improve science is because methodological falsificationism is not the best way to make scientific discoveries. Currently, preregistration is tied to a philosophy of science that believes it is valuable to make claims that are severely tested. This error-statistical approach to scientific knowledge generation (Mayo Citation2018) connects Neyman-Pearson hypothesis testing where the maximum Type 1 and Type 2 error rate in studies is controlled by setting an alpha level and designing studies with high statistical power to the goal of making scientific claims (Frick Citation1996; Lakens Citation2019). It should be noted that beyond frequentist statistics, several approaches to Bayesian statistics also value severity (Gelman and Shalizi Citation2013; van Dongen, Sprenger, and Wagenmakers Citation2023; Vanpaemel Citation2019). Nevertheless, researchers might reject the idea that severely tested claims are important in scientific knowledge generation.
It is understandable that researchers with different philosophies of science would not see any value in preregistration. As noted previously, if researchers believe their field is not actually testing predictions, but instead solely performs exploratory research (Olken Citation2015; Pham and Oh Citation2021), preregistration is of little use. Some researchers have hinted that scientists should rely on ‘inference to the best explanation’ or Bayesian updating of their belief in theories instead (Rubin Citation2022). However, inference to the best explanation is used to construct theories, not to test them (Haig Citation2018), and even proponents of inference to the best explanation write that “The strengths of the error-statistical approach are considerable […] and I believe that they combine to give us the most coherent philosophy of statistics currently available” (Haig Citation2020, 2).
Others have criticized preregistration for marginalizing other philosophical approaches to science, such as constructivist research (which builds on the idea that knowledge about the world is always a human and social construction), threatening ‘pluralism’ in science. For example, Bazzoli (Citation2022) argued that “constructionist epistemologies are not concerned with replicability of findings at all” and “the social reality we inhabit is not necessarily objective and measurable”. This criticism mainly raises the concern that research performed from a different philosophy of science, such as ‘social constructivism’ will be seen as less valuable, relative to preregistered studies. This might indeed happen, but we should not criticize best practices in other philosophies of science, just because we fear it will be more difficult for our preferred philosophy to become more widely accepted.
Unintended side-effects of preregistration
Every change to a complex system will have unintended side-effects. It is possible that the unintended side-effects of preregistration have such a high cost that the normative argument to preregister might be correct, but that implementing it in practice would come at too great a cost for scientific progress. Some authors are worried that preregistration might dissuade researchers from exploring their data (i.e., data-driven analyses). For example, Ellemers (Citation2013, 3) argued that “we are at risk of becoming methodological fetishists, who measure quality of science by the use of “proper” research procedures, not by the progress we make in understanding human behavior […]. This would reverse the means and the ends of doing research and stifles the creativity that is essential to the advancement of science.” Similarly, Pham and Oh (Citation2021, 68–169) noted: “Although it is often argued that preregistration does not preclude exploratory analyses, one of its key drawbacks, if not its main drawback, is that it discourages exploration by insisting on a sharp distinction between exploratory and confirmatory findings and granting evidentiary status only to confirmatory results.”
There is no empirical evidence to support this hypothesis, and one could just as easily hypothesize that preregistration will help liberate exploration and give it its proper place in the scientific literature. Höfler et al. (Citation2022) argued that honest exploration is not rewarded in scientific journals due to a longstanding pressure to produce confirmatory results. As preregistration helps researchers to distinguish planned analyses from data-driven exploratory tests, the honest and transparent reporting of exploratory analyses might increase the appreciation of exploratory research. Currently, purely exploratory research is rarely published in most research areas. Future metascientific studies should examine whether preregistration increases or decreases exploratory analyses. Cortex, which launched Registered Reports in 2014, also launched ‘Exploratory Reports’ in 2017 in response to concerns that exploratory research would be discouraged (McIntosh Citation2017). The article format has not proven very popular, with only 17 Exploratory Reports published since 2018, an average of approximately 3 a year, while Cortex publishes approximately 179 empirical articles a year (R. McIntosh, personal communication, March 21, 2024).
One of the few empirical studies examining if preregistration discourages researchers to explore all possible analyses was performed by Collins, Whillans, and John (Citation2021) who aimed to examine the behavioral and subjective consequences of performing confirmatory research (e.g., a preregistered study). From their first study Collins and colleagues concluded that “engaging in a pre-registration task impeded the discovery of an interesting but non-hypothesized result.” However, a closer look at the findings reveals no overall difference in how much researchers explored the data. There was a difference in how often participants explored one specific analysis out of seven analyses presented to participants. This analysis happened to be the one that, if performed, would reveal a surprising interaction effect. However, as participants could not know this while exploring, there does not seem to be a possible mechanism underlying the effect, and future replication studies are needed to reduce the probability that this finding was a Type 1 error. In their second study Collins and colleagues found both exploratory and confirmatory data analyses were perceived as enjoyable, but exploring data was evaluated as slightly more positively, and as slightly less scientific. The differences were small, and how enjoyable it is to perform either type of analysis is unlikely to have a noticeable effect on how many exploratory or confirmatory studies are published in the scientific literature.
A unintended side-effect of preregistration is the idea researchers might believe that they are stuck with their plan, even if they realize it is not appropriate, which has been referred to as ‘researcher commitment bias’ (Rubin & Donkin, Citation2022). Metascientific research has shown that deviations from preregistration are very common, so it seems that few researchers stick with a preregistered plan if they consider it suboptimal. It is unknown how often researchers do not deviate, when a deviation would have improved their article. The best way to mitigate this side-effect is to educate scientists about when and how they can deviate from preregistration, and to develop structured approaches to reporting deviations from preregistrations (Lakens Citation2024; Willroth and Atherton Citation2024).
A second unintended side-effect is that researchers mindlessly treat preregistration as a signal of study quality, adding a ‘superficial veneer of rigor’ (Devezer et al. Citation2021) without carefully evaluating the validity and severity of the test. It has been pointed out that non-preregistered studies can be as severe or more severe tests than non-preregistered studies (Lash and Vandenbroucke Citation2012), and it would be a bad use of preregistration to heuristically treat it as a cue of superior quality. Again, this criticism is not specific to preregistration, as scientists have raised concerns about a range of mindless heuristics to evaluate the quality of studies, such as the country of origin of the scientists, the institutions they work at, or the prestige of the author (even though there are remaining questions about the extent to which such bias exists, see Lee et al. Citation2013).
On the other hand, preregistration seems to increase trust in claims that researchers make (Alister et al. Citation2021; Conry-Murray, Mcconnon, and Bower Citation2022; Field et al. Citation2020; Spitzer and Mueller Citation2023), but the effect is very small. Researchers indicate that preregistered studies are perceived to signal the absence of systematic bias (Logg and Dorison Citation2021; Sarafoglou et al. Citation2022). At the same time, metascientific research has shown that preregistrations are often of low quality (M. Bakker et al. Citation2020), and hypothesis tests from preregistrations are still selectively reported (Ensinck and Lakens Citation2023; van den Akker, van Assen, Enting, et al. Citation2023). One way to prevent the unintended side-effect of mindlessly using preregistration as a sign of quality is to make it easier to evaluate how severe the preregistered tests were, and to determine whether preregistered hypothesis tests are correctly reported in the article (Eijk et al. Citation2024; Lakens and DeBruine Citation2021; Van Lissa et al. Citation2021). Another solution is to educate researchers about the fact that preregistration in itself does not automatically make a study better than a non-preregistered study (Lakens Citation2019; Mayo Citation2018), which will hopefully reduce the extent to which researchers use preregistration as a “lazy heuristic for research quality” (Pham and Oh Citation2021). A third solution is to implement preregistration within Registered Reports, where the Stage 1 review provides an opportunity for peer reviewers to criticize the theory, study design, and analysis plan of the Registered Report.
Misunderstandings about preregistration
Some researchers outright misunderstand the goal of preregistration and believe it intends to reduce exploration. For example, McDermott (Citation2022, 57) argued that “science should not discourage exploration. Yet that is exactly what preregistration entails and demands” despite the dozens of papers explaining why this statement is wrong, as preregistration only requires transparently communicating which analyses were preregistered, and which are data-driven (Höfler et al. Citation2022; Nosek and Lakens Citation2014; Wagenmakers et al. Citation2012). As Simons (Citation2018) reminded us: “Note that preregistration does not preclude a complete and careful evaluation of the data and evidence; exploration is the engine of discovery and the source of new hypotheses even if it does not support confirmatory hypothesis tests”.
Some researchers confuse how severely a claim has been tested with the probability that a theory is true. For example, when Szollosi and Donkin (Citation2021) wrote “Presumably, we are to be more skeptical of theories that have been adjusted, and more confident in theories whose predictions were borne out in an experiment” they presumed too much. Preregistration only aims to make the severity of the test transparent. How much confidence we should have in theories that have been adjusted is a complex question (Lakatos Citation1978), because the verisimilitude of a theory is based on many factors unrelated to systematic bias, such as the validity of the test (Vazire, Schiavone, and Bottesini Citation2022). Severely tested claims are in practice likely correlated with the verisimilitude of a theory, especially in a scientific system where systematic bias is present, but a corroborated preregistered test is not necessarily closer to the truth (Lakens Citation2019), and deviations can increase the validity of an inference, despite lowering the severity with which a claim has been tested (Lakens Citation2024).
Some researchers believe the goal of preregistration is to improve theorizing. For example, Szollosi et al. (Citation2020) wrote: “Our critique is focused on the use of preregistration as an attempt to improve scientific reasoning and theory development.” However, the goal of preregistration is not to improve scientific reasoning or theory development. One could argue that having access to more severely tested claims facilitates theory development, but that depends on the theory. One reason we promote a rigorous definition of preregistration, grounded in a methodological-falsificationist philosophy of science, is to prevent these kinds of misunderstandings.
Some researchers have argued that “preregistration is offered as a solution to the problem of using data more than once,” such as double dipping or data-peeking (Devezer et al. Citation2021). However, this is not an accurate description of the problem that preregistration aims to address. Data can be used more than once, for example in sequential analysis where data are collected, analyzed, and if a non-significant result is observed, data collection is continued, and all data (including the data used to make the decision to continue data collection) is analyzed at the second analysis (Lakens Citation2014; Proschan, Lan, and Wittes Citation2006; Wassmer and Brannath Citation2016). Sequential analyses can be used as severe tests of predictions as long as the Type 1 error rate is controlled. Instead, preregistration solves the problem that peers cannot transparently evaluate the severity of a test when there is no information about the extent to which the data influence when and how often the data was analyzed.
Preregistration in practice
represents the workflow promoted in previous sections, to present when and how researchers should adopt preregistration and Registered Reports. As an example, researchers might be interested in studying the toxicity of microplastics in mice. They develop their research question and start an open lab notebook to document the research process. The open lab notebook increases transparency about decisions made during the research process, and can indicate what was known about the data (e.g., for secondary data analysis) or explain how data-driven decisions were made (e.g., during non-confirmatory analyses). The researchers can then choose to design a study and preregister their hypotheses and statistical analysis plan on a platform such as the Open Science Framework. This preregistration allows peers to evaluate the severity with which claims were tested and makes the opportunistic use of flexibility in how claims are tested (and the accompanying increase in Type 1 or Type 2 error rates) transparent. Researchers can subsequently choose to submit their preregistration as a Registered Report to prevent publication bias. If they decide to follow the Registered Report publication route and have a strict timeline, they can optionally use the possibility to schedule Stage 1 peer review (for example at PCI-RR). After peer review and receiving in-principle acceptance they collect and analyze the data. For preregistered studies researchers should clearly distinguish preregistered analyses from non-preregistered analyses. Deviations from the analysis plan are possible but should be transparently reported, and researchers should reflect on the trade-off between the reduced test severity and the increased test validity. For non-preregistered analyses researchers can summarize notes in the open lab notebook and reflect on where bias might have influenced their decisions.
Figure 2. The publication process for preregistered studies, Registered Reports, and non-preregistered studies. The gray area represents the procedure for analyses that cannot or have not been preregistered.
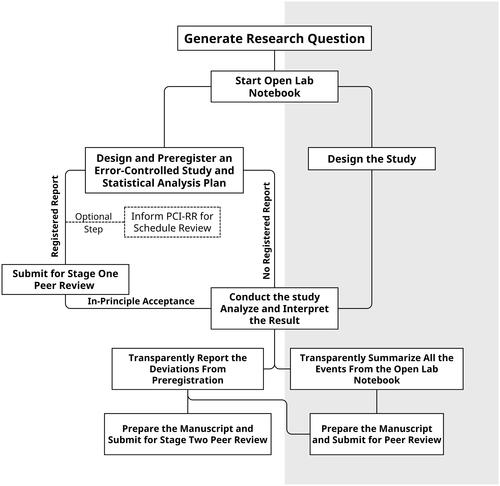
Researchers then submit their manuscript. For Registered Reports, authors will receive Stage 2 review, which only checks if the preregistered plan was followed, there were no unexpected events that threaten the validity of the study, and the conclusions follow from the data. For preregistered studies and traditional manuscripts the standard peer review process takes place, and there is no protection against publication bias.
It is useful to keep the following recommendations in mind when implementing preregistration. To allow peers to evaluate the severity of a test, the first recommendation is to make the preregistration document as detailed as possible (Waldron and Allen Citation2022). It is important to acknowledge that preregistrations can lack sufficient detail either because researchers do not yet know how they will test their predictions, or because the verbal descriptions are too vague.
The second recommendation is for researchers who plan exploratory research, qualitative studies, or secondary data analysis when the data was accessible beforehand to use the best tools to increase transparency. As we have recommended, an open lab notebook approach is better suited than a preregistration in these categories of research questions. Researchers should prevent premature tests of hypotheses, when their actual research questions best fit an exploratory approach (Höfler et al. Citation2022; Scheel et al. Citation2021). Empirical research can be used to generate theories, instead of testing theories, in line with Van Fraassen’s idea that “experimentation is the continuation of theory construction by other means” (Van Fraassen Citation1980, 77). A severe test of a prediction often requires tests of auxiliary hypotheses concerning the effect of measures and manipulations (Uygun-Tunç and Tunç Citation2023), as well as descriptive data to inform researchers about how to preprocess data and which test assumptions are met. Sometimes only a direct replication of an exploratory study can be preregistered in sufficient detail. Researchers can prevent incomplete preregistrations by following preregistration templates developed for their specific research area (Mellor, Corker, and Whaley Citation2024). Researchers can also use other tools more suited to these types of research, such as open lab notebooks.
To prevent vague preregistrations, the third recommendation is that researchers preregister the analysis code that reads in and processes the raw data, performs all preregistered tests, and specifies how the results of the tests will be interpreted. Regrettably, a recent estimate suggests that only slightly more than a quarter of the researchers who preregister include analysis code (Silverstein et al. Citation2024). When preregistering analysis code researchers can either write code based on a simulated dataset, or on data from a pilot study. An even higher bar to prevent ambiguity is to make sure a preregistration is machine readable, where the evaluation of hypothesis tests (i.e., whether they corroborate or falsify predictions) can be performed automatically based on the analysis code and the data (Lakens and DeBruine Citation2021). In a clear preregistration of a hypothesis test, all components of a hypothesis test (the analysis, the way results will be compared to criteria, and how results will be evaluated in terms of corroborating of falsifying a prediction) will be clearly specified. By preregistering the analysis code, all steps in the data analysis are clearly communicated, including assumption checks, exclusion of outliers, and the exact analysis to be run (including any parameters that need to be specified for the test).
Lastly, deviations from preregistration are sometimes unavoidable. Although some researchers manage to report a study that was carried out exactly as planned in the preregistration, in practice deviations are common (Claesen et al. Citation2019; Toth et al. Citation2021; van den Akker et al. Citation2023; Willroth and Atherton Citation2024). Metascientific research has revealed several reasons to deviate from a preregistration, which largely fall into five categories (Lakens Citation2024): (1) unforeseen events, (2) errors in the preregistration, (3) missing information, (4) violations of untested assumptions, and (5) the falsification of auxiliary hypotheses. Depending on the amount of flexibility researchers have when deviating from a preregistration (e.g., due to unforeseen events), deviations might have no meaningful impact on the severity of tests. In other cases, for example when correcting errors, or when assumptions are not met, deviations can reduce the severity of a test, but increase the validity of the inference (Shadish, Cook, and Campbell Citation2001). As invalid inferences have little scientific value, it can be necessary to deviate from a preregistration, even if this comes at the expense of the severity of the test. It is recommended that any form of deviation, including descriptions of when and where they occurred, are clearly reported. Additionally, the justification for each deviation, as well as an evaluation of its impact on the severity of the test, should be stated (Lakens Citation2024).
Conclusion
Preregistration, study registries and Registered Reports are important tools to improve scientific practice and increase transparency. These tools make it easier for peers to verify research, which is one of the goals of open science according to the Society of Environmental Toxicology and Chemistry (Declaration on Open Science Citation2020). In theory, there is no reason to be concerned about a lack of transparency as long as scientists are perfectly unbiased. Keynes (Citation1921) famously wrote: “The peculiar virtue of prediction or predesignation is altogether imaginary. The number of instances examined and the analogy between them are the essential points, and the question as to whether a particular hypothesis happens to be propounded before or after their examination is quite irrelevant”. This often-cited passage is followed by an important caveat, when he adds: “The opposite view, which the unreliability of some statisticians has brought into existence,—that it is a positive advantage to approach statistical evidence without preconceptions based on general grounds, because the temptation to ‘cook’ the evidence will prove otherwise to be irresistible,—has no logical basis and need only be considered when the impartiality of an investigator is in doubt”. This viewpoint was echoed more recently by Vandenbroucke (Citation2010): “One thread in the arguments favoring registration of observational studies is that conclusions from data that bear on a “prespecified hypothesis” are stronger than if the hypothesis was not specified before seeing the data. Although seemingly intuitive, logicians have a hard time proving this; the explanation a scientist arrives at might be exactly the same, whether thought of before or after seeing the data, so what’s the difference?”.
There is indeed no logical basis to treat a hypothesis that is created before or after looking at data any differently. But given the overwhelming empirical evidence of bias in the scientific literature there is a social epistemological basis to distinguish claims inspired by data from claims tested on data. After all, it seems that human beings are not impartial, and without preconceptions. For this reason, the current approach to science is to implement methodological procedures that allow peers to transparently evaluate whether decisions introduce bias. Preregistration is such a methodological procedure and, when implemented well, makes it possible to criticize decisions related to the statistical analyses perceived to reduce the severity of tests. Registered Reports, in addition, have the benefit of reducing publication bias. Metascientific research reveals both practices achieve their goals, although continued attention to both the practical implementation, as well as the philosophical grounding are required to fully achieve its benefits.
Authors’ contributions
Conceptualization: DL. Writing and editing: DL, CM, RS, MD.
Supplementary_Materials (1).docx
Download MS Word (29.8 KB)Disclosure statement
The authors declare no competing interests.
Data availability statement
The data and code used to generate is available from: https://doi.org/10.17605/osf.io/xcu4m. Supplementary materials for is available from: https://osf.io/sxg7q
Additional information
Funding
References
- Agnoli, F., J. M. Wicherts, C. L. S. Veldkamp, P. Albiero, and R. Cubelli. 2017. “Questionable Research Practices among Italian Research Psychologists.” PloS One 12 (3): e0172792. https://doi.org/10.1371/journal.pone.0172792
- Alister, M., R. Vickers-Jones, D. K. Sewell, and T. Ballard. 2021. “How Do We Choose Our Giants? Perceptions of Replicability in Psychological Science.” Advances in Methods and Practices in Psychological Science 4 (2): 251524592110181. https://doi.org/10.1177/25152459211018199
- Allen, C., and D. M. A. Mehler. 2019. “Open Science Challenges, Benefits and Tips in Early Career and Beyond.” PLoS Biology 17 (5): e3000246. https://doi.org/10.1371/journal.pbio.3000246
- American Psychological Association. 2023. Open Science Badges. https://www.apa.org/pubs/journals/resources/open-science-badges
- Artino, A. R., E. W. Driessen, and L. A. Maggio. 2019. “Ethical Shades of Gray: International Frequency of Scientific Misconduct and Questionable Research Practices in Health Professions Education.” Academic Medicine: journal of the Association of American Medical Colleges 94 (1): 76–84. https://doi.org/10.1097/ACM.0000000000002412
- Babbage, C. 1830. Reflections on the Decline of Science in England: And on Some of Its Causes. London: B. Fellowes.
- Bakan, D. 1966. “The Test of Significance in Psychological Research.” Psychological Bulletin 66 (6): 423–437. https://doi.org/10.1037/h0020412
- Bakker, B. N., J. Kokil, T. Dörr, N. Fasching, and Y. Lelkes. 2021. “Questionable and Open Research Practices: Attitudes and Perceptions among Quantitative Communication Researchers.” Journal of Communication 71 (5): 715–738. https://doi.org/10.1093/joc/jqab031
- Bakker, M., C. L. S. Veldkamp, M. A. L. M. van Assen, E. A. V. Crompvoets, H. H. Ong, B. A. Nosek, C. K. Soderberg, D. Mellor, and J. M. Wicherts. 2020. “Ensuring the Quality and Specificity of Preregistrations.” PLoS Biology 18 (12): e3000937. https://doi.org/10.1371/journal.pbio.3000937
- Barber, B. 1961. “Resistance by Scientists to Scientific Discovery.” Science (New York, N.Y.) 134 (3479): 596–602. https://doi.org/10.1126/science.134.3479.596
- Bazzoli, A. 2022. “Open Science and Epistemic Pluralism: A Tale of Many Perils and Some Opportunities.” Industrial and Organizational Psychology 15 (4): 525–528. https://doi.org/10.1017/iop.2022.67
- Bottesini, J. G., M. Rhemtulla, and S. Vazire. 2022. “What Do Participants Think of Our Research Practices? An Examination of Behavioural Psychology Participants’ Preferences.” Royal Society Open Science 9 (4): 200048. https://doi.org/10.1098/rsos.200048
- Brachem, J., M. Frank, T. Kvetnaya, L. F. F. Schramm, and L. Volz. 2022. “Replikationskrise, p-Hacking Und Open Science.” Psychologische Rundschau 73 (1): 1–17. https://doi.org/10.1026/0033-3042/a000562
- Brodeur, A., N. M. Cook, J. S. Hartley, and A. Heyes. 2024. Do Pre-Registration and Pre-Analysis Plans Reduce p-Hacking and Publication Bias? Evidence from 15,992 Test Statistics and Suggestions for Improvement (Working Paper 101). I4R Discussion Paper Series. https://www.econstor.eu/handle/10419/281136
- Carter, E. C., F. D. Schönbrodt, W. M. Gervais, and J. Hilgard. 2019. “Correcting for Bias in Psychology: A Comparison of Meta-Analytic Methods.” Advances in Methods and Practices in Psychological Science 2 (2): 115–144. https://doi.org/10.1177/2515245919847196
- Chambers, C. D. 2013. “Registered Reports: A New Publishing Initiative at Cortex.” Cortex: A Journal Devoted to the Study of the Nervous System and Behavior 49 (3): 609–610. https://doi.org/10.1016/j.cortex.2012.12.016
- Chambers, C. D., and L. Tzavella. 2022. “The Past, Present and Future of Registered Reports.” Nature Human Behaviour 6 (1): 29–42. https://doi.org/10.1038/s41562-021-01193-7
- Chan, A.-W., J. M. Tetzlaff, P. C. Gøtzsche, D. G. Altman, H. Mann, J. A. Berlin, K. Dickersin, et al. 2013. “SPIRIT 2013 Explanation and Elaboration: Guidance for Protocols of Clinical Trials.” BMJ (Clinical Research ed.) 346 (jan08 15): e7586–e7586. https://doi.org/10.1136/bmj.e7586
- Chin, J. M., J. T. Pickett, S. Vazire, and A. O. Holcombe. 2021. “Questionable Research Practices and Open Science in Quantitative Criminology.” Journal of Quantitative Criminology 39 (1): 21–51. https://doi.org/10.1007/s10940-021-09525-6
- Claesen, A., S. L. B. T. Gomes, F. Tuerlinckx, and Vanpaemel Wolf. 2019. “Preregistration: Comparing Dream to Reality.” [Preprint]. PsyArXiv. https://doi.org/10.31234/osf.io/d8wex
- Collins, H. K., A. V. Whillans, and L. K. John. 2021. “Joy and Rigor in Behavioral Science.” Organizational Behavior and Human Decision Processes 164: 179–191. https://doi.org/10.1016/j.obhdp.2021.03.002
- Conry-Murray, C., A. McConnon, and M. Bower. 2022. “The Effect of Preregistration and P-Value Patterns on Trust in Psychology and Biology Research.” Collabra: Psychology 8 (1): 36306. https://doi.org/10.1525/collabra.36306
- Declaration on Open Science. 2020. https://www.setac.org/resource/declaration-on-open-science.html
- Devezer, B., D. J. Navarro, J. Vandekerckhove, and E. Ozge Buzbas. 2021. “The Case for Formal Methodology in Scientific Reform.” Royal Society Open Science 8 (3): 200805. https://doi.org/10.1098/rsos.200805
- Dickersin, K., Y. I. Min, and C. L. Meinert. 1992. “Factors Influencing Publication of Research Results. Follow-up of Applications Submitted to Two Institutional Review Boards.” JAMA 267 (3): 374–378. https://doi.org/10.1001/jama.1992.03480210049019
- Dickersin, K., and D. Rennie. 2003. “Registering Clinical Trials.” JAMA 290 (4): 516–523. https://doi.org/10.1001/jama.290.4.516
- Dickersin, K., and D. Rennie. 2012. “The Evolution of Trial Registries and Their Use to Assess the Clinical Trial Enterprise.” JAMA 307 (17): 1861–1864. https://doi.org/10.1001/jama.2012.4230
- Dirnagl, U. 2020. “Preregistration of Exploratory Research: Learning from the Golden Age of Discovery.” PLoS Biology 18 (3): e3000690. https://doi.org/10.1371/journal.pbio.3000690
- Eijk, N., van Jiao, H, and Peters, G.-J. 2024. Making Preregistration Accessible: An R Package to Make Machine-Readable Preregistrations and Create New Preregistration Forms. https://doi.org/10.31234/osf.io/qfjy7
- Ellemers, N. 2013. “Connecting the Dots: Mobilizing Theory to Reveal the Big Picture in Social Psychology (and Why we Should Do This): The Big Picture in Social Psychology.” European Journal of Social Psychology 43 (1): 1–8. https://doi.org/10.1002/ejsp.1932
- Ellwood-Lowe, M. E., R. Foushee, and M. Srinivasan. 2021. Response to Flournoy (2021): Reflections on the Benefits and Challenges of Communicating the Results of a Pre-Registered Study. https://doi.org/10.31234/osf.io/wfe4r
- Ensinck, E., and D. Lakens. 2023. An Inception Cohort Study Quantifying How Many Registered Studies Are Published. PsyArXiv. https://doi.org/10.31234/osf.io/5hkjz
- Fanelli, D. 2012. “Negative Results Are Disappearing from Most Disciplines and Countries.” Scientometrics 90 (3): 891–904. https://doi.org/10.1007/s11192-011-0494-7
- Ferguson, J., R. Littman, G. Christensen, E. L. Paluck, N. Swanson, Z. Wang, E. Miguel, D. Birke, and J.-H. Pezzuto. 2023. “Survey of Open Science Practices and Attitudes in the Social Sciences.” Nature Communications 14 (1): 5401. https://doi.org/10.1038/s41467-023-41111-1
- Fiedler, K., and N. Schwarz. 2016. “Questionable Research Practices Revisited.” Social Psychological and Personality Science 7 (1): 45–52. https://doi.org/10.1177/1948550615612150
- Field, S. M., E.-J. Wagenmakers, H. A. L. Kiers, R. Hoekstra, A. F. Ernst, and D. van Ravenzwaaij. 2020. “The Effect of Preregistration on Trust in Empirical Research Findings: Results of a Registered Report.” Royal Society Open Science 7 (4): 181351. https://doi.org/10.1098/rsos.181351
- Flournoy, J. 2021. A Comment on Ellwood-Lowe et al. (2020/2021): Presentation and Discussion of Results Should Take the Lead from the Preregistration. https://doi.org/10.31234/osf.io/23qsw
- Franco, A., N. Malhotra, and G. Simonovits. 2014. “Publication Bias in the Social Sciences: Unlocking the File Drawer.” Science (New York, N.Y.) 345 (6203): 1502–1505. https://doi.org/10.1126/SCIENCE.1255484
- Fraser, H., T. Parker, S. Nakagawa, A. Barnett, and F. Fidler. 2018. “Questionable Research Practices in Ecology and Evolution.” PloS One 13 (7): e0200303. https://doi.org/10.1371/journal.pone.0200303
- Frick, R. W. 1996. “The Appropriate Use of Null Hypothesis Testing.” Psychological Methods 1 (4): 379–390. https://doi.org/10.1037/1082-989X.1.4.379
- Garcia-Garzon, E., A. Angulo-Brunet, O. Lecuona, J. R. Barrada, and G. Corradi. 2022. “Exploring COVID-19 Research Credibility among Spanish Scientists.” Current Psychology 43: 1–12. https://doi.org/10.1007/s12144-022-02797-6
- Gelman, A., and C. R. Shalizi. 2013. “Philosophy and the Practice of Bayesian Statistics.” British Journal of Mathematical and Statistical Psychology 66 (1): 8–38. https://doi.org/10/f4k2h4
- Goldacre, B., N. J. DeVito, C. Heneghan, F. Irving, S. Bacon, J. Fleminger, and H. Curtis. 2018. “Compliance with Requirement to Report Results on the EU Clinical Trials Register: Cohort Study and Web Resource.” BMJ (Clinical Research ed.) 362: k3218. https://doi.org/10.1136/bmj.k3218
- Greenwald, A. G. 1975. “Consequences of Prejudice against the Null Hypothesis.” Psychological Bulletin 82 (1): 1–20. https://doi.org/10.1037/h0076157
- Haig, B. D. 2018. “An Abductive Theory of Scientific Method.” In Method Matters in Psychology, 35–64. Springer. https://link.springer.com/book/10.1007/978-3-030-01051-5#bibliographic-information
- Haig, B. D. 2020. “What Can Psychology’s Statistics Reformers Learn from the Error-Statistical Perspective?” Methods in Psychology 2: 100020. https://doi.org/10.1016/j.metip.2020.100020
- Hardwig, J. 1991. “The Role of Trust in Knowledge.” Journal of Philosophy 88 (12): 693–708. https://doi.org/10.2307/2027007
- Haven, T. L., and L. Van Grootel. 2019. “Preregistering Qualitative Research.” Accountability in Research 26 (3): 229–244. https://doi.org/10.1080/08989621.2019.1580147
- Hedges, L. V. 1984. “Estimation of Effect Size under Nonrandom Sampling: The Effects of Censoring Studies Yielding Statistically Insignificant Mean Differences.” Journal of Educational Statistics 9 (1): 61–85. https://doi.org/10.3102/10769986009001061
- Höfler, M., S. Scherbaum, P. Kanske, B. McDonald, and R. Miller. 2022. “Means to Valuable Exploration: I. The Blending of Confirmation and Exploration and How to Resolve It.” Meta-Psychology 6. https://doi.org/10.15626/MP.2021.2837
- Isbell, D. R., D. Brown, M. Chen, D. J. Derrick, R. Ghanem, M. N. G. Arvizu, E. Schnur, M. Zhang, and L. Plonsky. 2022. “Misconduct and Questionable Research Practices: The Ethics of Quantitative Data Handling and Reporting in Applied Linguistics.” Modern Language Journal 106 (1): 172–195. https://doi.org/10.1111/modl.12760
- Janke, S., M. Daumiller, and S. C. Rudert. 2019. “Dark Pathways to Achievement in Science: Researchers’ Achievement Goals Predict Engagement in Questionable Research Practices.” Social Psychological and Personality Science 10 (6): 783–791. https://doi.org/10.1177/1948550618790227
- John, L. K., G. Loewenstein, and D. Prelec. 2012. “Measuring the Prevalence of Questionable Research Practices with Incentives for Truth Telling.” Psychological Science 23 (5): 524–532. https://doi.org/10.1177/0956797611430953
- Johnson, M. 1975. “Models of Control and Control of Bias.” European Journal of Parapsychology 1: 36–44.
- Kaplan, R. M., and V. L. Irvin. 2015. “Likelihood of Null Effects of Large NHLBI Clinical Trials Has Increased over Time.” PloS One 10 (8): e0132382. https://doi.org/10.1371/journal.pone.0132382
- Kerr, N. L. 1998. “HARKing: Hypothesizing After the Results Are Known.” Personality and Social Psychology Review: An Official Journal of the Society for Personality and Social Psychology, Inc 2 (3): 196–217. https://doi.org/10.1207/s15327957pspr0203_4
- Keynes, J. M. 1921. A Treatise on Probability. Cambrige: Cambridge University Press.
- Kleinaki, A.-S., P. Mytis-Gkometh, G. Drosatos, P. S. Efraimidis, and E. Kaldoudi. 2018. “A Blockchain-Based Notarization Service for Biomedical Knowledge Retrieval.” Computational and Structural Biotechnology Journal 16: 288–297. https://doi.org/10.1016/j.csbj.2018.08.002
- Köhler, T., M. G. González-Morales, G. C. Banks, E. H. O’Boyle, J. A. Allen, R. Sinha, S. E. Woo, and L. M. V. Gulick. 2020. “Supporting Robust, Rigorous, and Reliable Reviewing as the Cornerstone of Our Profession: Introducing a Competency Framework for Peer Review.” Industrial and Organizational Psychology 13 (1): 1–27. https://doi.org/10.1017/iop.2019.121
- Kvarven, A., E. Strømland, and M. Johannesson. 2019. “Comparing Meta-Analyses and Preregistered Multiple-Laboratory Replication Projects.” Nature Human Behaviour 4 (4): 423–434. https://doi.org/10.1038/s41562-019-0787-z
- Laine, C., R. Horton, C. D. DeAngelis, J. M. Drazen, F. A. Frizelle, F. Godlee, C. Haug, et al. 2007. “Clinical Trial Registration—Looking Back and Moving Ahead.” New England Journal of Medicine 356 (26): 2734–2736. https://doi.org/10.1056/NEJMe078110
- Lakatos, I. 1978. The Methodology of Scientific Research Programmes: Volume 1: Philosophical Papers. Cambridge: Cambridge University Press.
- Lakens, D. 2014. “Performing High-Powered Studies Efficiently with Sequential Analyses.” European Journal of Social Psychology 44 (7): 701–710. https://doi.org/10.1002/ejsp.2023
- Lakens, D. 2015. “On the Challenges of Drawing Conclusions from p-Values Just below 0.05.” PeerJ. 3: e1142. https://doi.org/10.7717/peerj.1142
- Lakens, D. 2019. “The Value of Preregistration for Psychological Science: A Conceptual Analysis.” Japanese Psychological Review 62 (3): 221–230. https://doi.org/10.24602/sjpr.62.3_221
- Lakens, D. 2024. “When and How to Deviate From a Preregistration.” Collabra: Psychology 10 (1): 117094. https://doi.org/10.1525/collabra.117094
- Lakens, D., and L. M. DeBruine. 2021. “Improving Transparency, Falsifiability, and Rigor by Making Hypothesis Tests Machine-Readable.” Advances in Methods and Practices in Psychological Science 4 (2): 251524592097094. https://doi.org/10.1177/2515245920970949
- Lash, T. L., and J. P. Vandenbroucke. 2012. “Commentary: Should Preregistration of Epidemiologic Study Protocols Become Compulsory? Reflections and a Counterproposal.” Epidemiology (Cambridge, Mass) 23 (2): 184–188. https://doi.org/10.1097/EDE.0b013e318245c05b
- Latan, H., C. J. Chiappetta Jabbour, A. B. Lopes de Sousa Jabbour, and M. Ali. 2021. “Crossing the Red Line? Empirical Evidence and Useful Recommendations on Questionable Research Practices among Business Scholars.” Journal of Business Ethics 184 (3): 549–569. – https://doi.org/10.1007/s10551-021-04961-7
- Lee, C. J., C. R. Sugimoto, G. Zhang, and B. Cronin. 2013. “Bias in Peer Review.” Journal of the American Society for Information Science and Technology 64 (1): 2–17. https://doi.org/10.1002/asi.22784
- Lemmens, T. 2004. “Leopards in the Temple: Restoring Scientific Integrity to the Commercialized Research Scene.” Journal of Law, Medicine & Ethics: Journal of the American Society of Law, Medicine & Ethics 32 (4): 641–657. https://doi.org/10.1111/j.1748-720x.2004.tb01969.x
- Lenz, G. S., and A. Sahn. 2021. “Achieving Statistical Significance with Control Variables and Without Transparency.” Political Analysis 29 (3): 356–369. https://doi.org/10.1017/pan.2020.31
- Logg, J. M., and C. A. Dorison. 2021. “Pre-Registration: Weighing Costs and Benefits for Researchers.” Organizational Behavior and Human Decision Processes 167: 18–27. https://doi.org/10.1016/j.obhdp.2021.05.006
- Mahoney, M. J. 1977. “Publication Prejudices: An Experimental Study of Confirmatory Bias in the Peer Review System.” Cognitive Therapy and Research 1 (2): 161–175. https://doi.org/10.1007/BF01173636
- Makel, M. C., J. Hodges, B. G. Cook, and J. A. Plucker. 2021. “Both Questionable and Open Research Practices Are Prevalent in Education Research.” Educational Researcher 50 (8): 493–504. https://doi.org/10.3102/0013189X211001356
- Mayo, D. G. 2018. Statistical Inference as Severe Testing: How to Get beyond the Statistics Wars. Cambridge: Cambridge University Press.
- McDermott, R. 2022. “Breaking Free: How Preregistration Hurts Scholars and Science.” Politics and the Life Sciences: The Journal of the Association for Politics and the Life Sciences 41 (1): 55–59. https://doi.org/10.1017/pls.2022.4
- McIntosh, R. D. 2017. “Exploratory Reports: A New Article Type for Cortex.” Cortex: A Journal Devoted to the Study of the Nervous System and Behavior 96: A1–A4. https://doi.org/10.1016/j.cortex.2017.07.014
- Meehl, P. E. 2004. “Cliometric Metatheory III: Peircean Consensus, Verisimilitude and Asymptotic Method.” British Journal for the Philosophy of Science 55 (4): 615–643. https://doi.org/10.1093/bjps/55.4.615
- Mellor, D., K. S. Corker, and P. Whaley. 2024. “Preregistration Templates as a New Addition to the Evidence-Based Toxicology Toolbox.” Evidence-Based Toxicology 2 (1): 2314303. https://doi.org/10.1080/2833373X.2024.2314303
- Mertens, G., and A.-M. Krypotos. 2019. “Preregistration of Analyses of Preexisting Data.” Psychologica Belgica 59 (1): 338–352. https://doi.org/10.5334/pb.493
- Mitroff, I. I. 1974. “Norms and Counter-Norms in a Select Group of the Apollo Moon Scientists: A Case Study of the Ambivalence of Scientists.” American Sociological Review 39 (4): 579–595. https://doi.org/10.2307/2094423
- Moran, C., A. Richard, K. Wilson, R. Twomey, and A. Coroiu. 2022. “I Know It’s Bad, but I Have Been Pressured into It: Questionable Research Practices among Psychology Students in Canada.” Canadian Psychology/Psychologie Canadienne 64 (1): 12–24. https://doi.org/10.1037/cap0000326
- Motyl, M., A. P. Demos, T. S. Carsel, B. E. Hanson, Z. J. Melton, A. B. Mueller, J. P. Prims, et al. 2017. “The State of Social and Personality Science: Rotten to the Core, Not so Bad, Getting Better, or Getting Worse?” Journal of Personality and Social Psychology 113 (1): 34–58. https://doi.org/10.1037/pspa0000084
- Munafò, M. R., and G. D. Smith. 2018. “Robust Research Needs Many Lines of Evidence.” Nature 553 (7689): 399–401. https://doi.org/10.1038/d41586-018-01023-3
- Neyman, J., and E. S. Pearson. 1928. “On the Use and Interpretation of Certain Test Criteria for Purposes of Statistical Inference: Part I.” Biometrika 20A (1–2): 175–240. https://doi.org/10.1093/biomet/20A.1-2.175
- Nosek, B. A., C. R. Ebersole, A. C. DeHaven, and D. T. Mellor. 2018. “The Preregistration Revolution.” Proceedings of the National Academy of Sciences of the United States of America 115 (11): 2600–2606. https://doi.org/10.1073/pnas.1708274114
- Nosek, B. A., and D. Lakens. 2014. “Registered Reports: A Method to Increase the Credibility of Published Results.” Social Psychology 45 (3): 137–141. https://doi.org/10.1027/1864-9335/a000192
- Ofosu, G. K., and D. N. Posner. 2023. “Pre-Analysis Plans: An Early Stocktaking.” Perspectives on Politics 21 (1): 174–190. https://doi.org/10.1017/S1537592721000931
- Olken, B. A. 2015. “Promises and Perils of Pre-Analysis Plans.” Journal of Economic Perspectives 29 (3): 61–80. https://doi.org/10.1257/jep.29.3.61
- Peer Community In – Registered Reports. n.d. Guide for Authors. https://rr.peercommunityin.org/help/guide_for_authors
- Pham, M. T., and T. T. Oh. 2021. “Preregistration Is Neither Sufficient nor Necessary for Good Science.” Journal of Consumer Psychology 31 (1): 163–176. https://doi.org/10.1002/jcpy.1209
- Pickett, J. T., and S. P. Roche. 2017. “Questionable, Objectionable or Criminal? Public Opinion on Data Fraud and Selective Reporting in Science.” Science and Engineering Ethics 24 (1): 151–171. https://doi.org/10.1007/s11948-017-9886-2
- Popper, K. R. 1959. The Logic of Scientific Discovery. London: Routledge.
- Popper, K. R. 1962. Conjectures and Refutations: The Growth of Scientific Knowledge. London: Routledge.
- Proschan, M. A., K. K. G. Lan, and J. T. Wittes. 2006. Statistical Monitoring of Clinical Trials: A Unified Approach. New York: Springer.
- Qureshi, R., A. Gough, and K. Loudon. 2022. “The SPIRIT Checklist—Lessons from the Experience of SPIRIT Protocol Editors.” Trials 23 (1): 359. https://doi.org/10.1186/s13063-022-06316-7
- Rabelo, A. L. A., J. E. M. Farias, M. M. Sarmet, T. C. R. Joaquim, R. C. Hoersting, L. Victorino, J. G. N. Modesto, and R. Pilati. 2020. “Questionable Research Practices among Brazilian Psychological Researchers: Results from a Replication Study and an International Comparison.” International Journal of Psychology: Journal International de Psychologie 55 (4): 674–683. https://doi.org/10.1002/ijop.12632
- Rosenthal, R. 1966. Experimenter Effects in Behavioral Research. New York: Appleton-Century-Crofts.
- Ross, R., and E. D. Heggestad. 2020. “An Empirical Exploration of Reviewers’ and Editors’ Roles Fostering High Quality Research during Peer Review.” Industrial and Organizational Psychology 13 (1): 32–36. https://doi.org/10.1017/iop.2020.16
- Rubin, M. 2022. “The Costs of HARKing.” British Journal for the Philosophy of Science 73 (2): 535–560. https://doi.org/10.1093/bjps/axz050
- Rubin, M., and C. Donkin. 2022. “Exploratory Hypothesis Tests Can Be More Compelling than Confirmatory Hypothesis Tests.” Philosophical Psychology:1–29. https://doi.org/10.1080/09515089.2022.2113771
- Sarafoglou, A., M. Kovacs, B. Bakos, E.-J. Wagenmakers, and B. Aczel. 2022. “A Survey on How Preregistration Affects the Research Workflow: Better Science but More Work.” Royal Society Open Science 9 (7): 211997. https://doi.org/10.1098/rsos.211997
- Sartori, G. 1970. “Concept Misformation in Comparative Politics.” American Political Science Review 64 (4): 1033–1053. https://doi.org/10.2307/1958356
- Schäfer, T., and M. A. Schwarz. 2019. “The Meaningfulness of Effect Sizes in Psychological Research: Differences Between Sub-Disciplines and the Impact of Potential Biases.” Frontiers in Psychology 10: 813. https://doi.org/10.3389/fpsyg.2019.00813
- Schapira, M., T. O. L. N. Consortium, and R. J. Harding, Open Lab Notebook Consortium. 2019. “Open Laboratory Notebooks: Good for Science, Good for Society, Good for Scientists.” F1000Research. (8 (87): 87. https://doi.org/10.12688/f1000research.17710.2
- Scheel, A. M., L. Tiokhin, P. M. Isager, and D. Lakens. 2021. “Why Hypothesis Testers Should Spend Less Time Testing Hypotheses.” Perspectives on Psychological Science: a Journal of the Association for Psychological Science 16 (4): 744–755. https://doi.org/10.1177/1745691620966795
- Schneider, J. W., N. Allum, J. P. Andersen, M. B. Petersen, N. Mejlgaard, and R. Zachariae. 2023. Is Something Rotten in the State of Denmark? Cross-National Evidence for Widespread Involvement but Not Systematic Use of Questionable Research Practices across All Fields of Research. https://doi.org/10.31222/osf.io/r6j3z
- Scroggie, K. R., K. J. Burrell-Sander, P. J. Rutledge, and A. Motion. 2023. “GitHub as an Open Electronic Laboratory Notebook for Real-Time Sharing of Knowledge and Collaboration.” Digital Discovery 2 (4): 1188–1196. https://doi.org/10.1039/D3DD00032J
- Shadish, W. R., T. D. Cook, and D. T. Campbell. 2001. Experimental and Quasi-Experimental Designs for Generalized Causal Inference. Boston: Houghton Mifflin.
- Silverstein, P., C. R. Pennington, P. Branney, D. B. O’Connor, E. Lawlor, E. O’Brien, and D. Lynott. 2024. “A Registered Report Survey of Open Research Practices in Psychology Departments in the UK and Ireland.” British Journal of Psychology (London, England: 1953):1–36. https://doi.org/10.1111/bjop.12700
- Simmons, J. P., L. D. Nelson, and U. Simonsohn. 2021. “Pre-Registration: Why and How.” Journal of Consumer Psychology 31 (1): 151–162. https://doi.org/10.1002/jcpy.1208
- Simons, D. J. 2018. “Introducing Advances in Methods and Practices in Psychological Science.” Advances in Methods and Practices in Psychological Science 1 (1): 3–6. https://doi.org/10.1177/2515245918757424
- Soderberg, C. K., T. M. Errington, S. R. Schiavone, J. Bottesini, F. S. Thorn, S. Vazire, K. M. Esterling, and B. A. Nosek. 2021. “Initial Evidence of Research Quality of Registered Reports Compared with the Standard Publishing Model.” Nature Human Behaviour 5 (8): 990–997. https://doi.org/10.1038/s41562-021-01142-4
- Spitzer, L., and S. Mueller. 2023. “Registered Report: Survey on Attitudes and Experiences regarding Preregistration in Psychological Research.” PloS One 18 (3): e0281086. https://doi.org/10.1371/journal.pone.0281086
- Sterling, T. D. 1959. “Publication Decisions and Their Possible Effects on Inferences Drawn from Tests of Significance—Or Vice Versa.” Journal of the American Statistical Association 54 (285): 30–34. https://doi.org/10.2307/2282137
- Swift, J. K., C. D. Christopherson, M. O. Bird, A. Zöld, and J. Goode. 2022. “Questionable Research Practices among Faculty and Students in APA-Accredited Clinical and Counseling Psychology Doctoral Programs.” Training and Education in Professional Psychology 16 (3): 299–305. https://doi.org/10.1037/tep0000322
- Syed, M. 2024. Preregistration: More Promises than Pitfalls [Substack newsletter]. Get Syeducated. https://web.archive.org/web/20240315201654/https://getsyeducated.substack.com/p/preregistration-more-promises-than
- Szollosi, A., and C. Donkin. 2021. “Arrested Theory Development: The Misguided Distinction Between Exploratory and Confirmatory Research.” Perspectives on Psychological Science: a Journal of the Association for Psychological Science 16 (4): 717–724. https://doi.org/10.1177/1745691620966796
- Szollosi, A., D. Kellen, D. J. Navarro, R. Shiffrin, I. van Rooij, T. Van Zandt, and C. Donkin. 2020. “Is Preregistration Worthwhile?” Trends in Cognitive Sciences 24 (2): 94–95. https://doi.org/10.1016/j.tics.2019.11.009
- Toth, A. A., G. C. Banks, D. Mellor, E. H. O’Boyle, A. Dickson, D. J. Davis, A. DeHaven, J. Bochantin, and J. Borns. 2021. “Study Preregistration: An Evaluation of a Method for Transparent Reporting.” Journal of Business and Psychology 36 (4): 553–571. https://doi.org/10.1007/s10869-020-09695-3
- Uygun-Tunç, D., M. N. Tunç, and Z. B. Eper. 2021. Is Open Science Neoliberal? https://doi.org/10.31234/osf.io/ft8dc
- Uygun-Tunç, D., and M. N. Tunç. 2023. “A Falsificationist Treatment of Auxiliary Hypotheses in Social and Behavioral Sciences: Systematic Replications Framework.” Meta-Psychology 7. https://doi.org/10.15626/MP.2021.2756
- van den Akker, O. R., M. A. L. M. van Assen, M. Bakker, M. Elsherif, T. K. Wong, and J. M. Wicherts. 2023. “Preregistration in Practice: A Comparison of Preregistered and Non-Preregistered Studies in Psychology.” Behavior Research Methods. https://doi.org/10.3758/s13428-023-02277-0
- van den Akker, O. R., M. A. L. M. van Assen, M. Enting, M. de Jonge, H. H. Ong, F. Rüffer, M. Schoenmakers, A. H. Stoevenbelt, J. M. Wicherts, and M. Bakker. 2023. “Selective Hypothesis Reporting in Psychology: Comparing Preregistrations and Corresponding Publications.” Advances in Methods and Practices in Psychological Science 6 (3): 25152459231187988. https://doi.org/10.1177/25152459231187988
- van Dongen, N., J. Sprenger, and E.-J. Wagenmakers. 2023. “A Bayesian Perspective on Severity: Risky Predictions and Specific Hypotheses.” Psychometric Bulletin & Review 30:516–533.
- Van Fraassen, B. C. 1980. The Scientific Image. Oxford: Oxford University Press.
- Van Lissa, C. J., A. M. Brandmaier, L. Brinkman, A.-L. Lamprecht, A. Peikert, M. E. Struiksma, and B. M. I. Vreede. 2021. “WORCS: A Workflow for Open Reproducible Code in Science.” Data Science 4 (1): 29–49. https://doi.org/10.3233/DS-210031
- Vandenbroucke, J. P. 2010. “Preregistration of Epidemiologic Studies: An Ill-Founded Mix of Ideas.” Epidemiology (Cambridge, Mass) 21 (5): 619–620. https://doi.org/10.1097/EDE.0b013e3181e942b8
- Vanpaemel, W. 2019. “The Really Risky Registered Modeling Report: Incentivizing Strong Tests and HONEST Modeling in Cognitive Science.” Computational Brain & Behavior 2 (3–4): 218–222. https://doi.org/10.1007/s42113-019-00056-9
- van’t Veer, Anna Elisabeth, and Roger Giner-Sorolla. 2016. “Pre-Registration in Social Psychology—A Discussion and Suggested Template.” Journal of Experimental Social Psychology 67: 2–12. https://doi.org/10.1016/j.jesp.2016.03.004
- Vazire, S., S. R. Schiavone, and J. G. Bottesini. 2022. “Credibility Beyond Replicability: Improving the Four Validities in Psychological Science.” Current Directions in Psychological Science 31 (2): 162–168. https://doi.org/10.1177/09637214211067779
- Vohs, K. D., B. J. Schmeichel, S. Lohmann, Q. F. Gronau, A. J. Finley, S. E. Ainsworth, J. L. Alquist, et al. 2021. “A Multisite Preregistered Paradigmatic Test of the Ego-Depletion Effect.” Psychological Science 32 (10): 1566–1581. https://doi.org/10.1177/0956797621989733
- Wacholder, S., S. Chanock, M. Garcia-Closas, L. El Ghormli, and N. Rothman. 2004. “Assessing the Probability That a Positive Report is False: An Approach for Molecular Epidemiology Studies.” Journal of the National Cancer Institute 96 (6): 434–442. https://doi.org/10.1093/jnci/djh075
- Wagenmakers, E.-J., R. Wetzels, D. Borsboom, H. L. van der Maas, and R. A. Kievit. 2012. “An Agenda for Purely Confirmatory Research.” Perspectives on Psychological Science: A Journal of the Association for Psychological Science 7 (6): 632–638. https://doi.org/10.1177/1745691612463078
- Waldron, S., and C. Allen. 2022. “Not All Pre-Registrations Are Equal.” Neuropsychopharmacology: Official Publication of the American College of Neuropsychopharmacology 47 (13): 2181–2183. https://doi.org/10.1038/s41386-022-01418-x
- Walster, G., and T. Cleary. 1970. “A Proposal for a New Editorial Policy in the Social Sciences.” American Statistician 24 (2): 16–19. https://doi.org/10.1080/00031305.1970.10478884
- Wandall, B., S. O. Hansson, and C. Rudén. 2007. “Bias in Toxicology.” Archives of Toxicology 81 (9): 605–617. https://doi.org/10.1007/s00204-007-0194-5
- Wassmer, G., and W. Brannath. 2016. Group Sequential and Confirmatory Adaptive Designs in Clinical Trials. Cham: Springer International Publishing. https://doi.org/10.1007/978-3-319-32562-0
- Weston, S. J., S. J. Ritchie, J. M. Rohrer, and A. K. Przybylski. 2019. “Recommendations for Increasing the Transparency of Analysis of Preexisting Data Sets.” Advances in Methods and Practices in Psychological Science 2 (3): 214–227. https://doi.org/10.1177/2515245919848684
- Willroth, E. C., and O. E. Atherton. 2024. “Best Laid Plans: A Guide to Reporting Preregistration Deviations.” Advances in Methods and Practices in Psychological Science 7 (1). https://doi.org/10.31234/osf.io/dwx69
- Zarin, D. A., K. M. Fain, H. D. Dobbins, T. Tse, and R. J. Williams. 2019. “10-Year Update on Study Results Submitted to ClinicalTrials.gov.” New England Journal of Medicine 381 (20): 1966–1974. https://doi.org/10.1056/NEJMsr1907644