ABSTRACT
Dyslexia is a neurodevelopmental difficulty affecting reading, but recent data in adults suggest that difficulties also extend to face processing. Here, we tested face processing in school children with and without dyslexia, using eye-tracking and neuropsychological tests. Children with dyslexia didn’t differ significantly from controls in face gaze patterns, face memory, or face identification accuracy. However, they were slower and more heterogeneous, with larger within-group variance than controls. Increased gaze patterns toward the eyes were associated with better face memory in controls. We discuss the possible role of experiential factors in prior research linking dyslexia and face processing differences.
Introduction
Developmental dyslexia (i.e., word-level reading difficulties not obviously caused by sensory acuity or low intellectual functioning) is linked with a range of troublesome outcomes including poor school achievement (Lyytinen, Erskine, Hämäläinen, Torppa, & Ronimus, Citation2015; Nordström, Jacobson, & Söderberg, Citation2016), and low academic self-esteem (Glazzard, Citation2010; Humphrey & Mullins, Citation2002). A large body of research has established that developmental dyslexia is commonly characterized by a phonological or phonology-to-grapheme mapping difficulty, which manifests itself in the dysfluent written word recognition and inaccurate decoding that defines the diagnosis (Lyon, Citation1995; Snowling, Hulme, & Nation, Citation2020). In this study, we examine potential processing differences of another class of visual stimuli in dyslexia – namely human faces.
The face truly holds a special status in human social perception. In a split-second, a person’s face provides important cues to his or her identity, gender, age, as well as to intentions and emotional states. Faces also hold relevant psycholinguistic information such as the direction of the other’s visual attention and speech-related articulatory gestures. The propensity to attend to faces or face-like stimuli is evident early on in development, perhaps even prior to birth (Reid et al., Citation2017), and it is presumed to be a critical skill enabling human cultural learning (Webb, Neuhaus, & Faja, Citation2017).
Eye-tracking technology is commonly used to explore the spatial dynamics of face perception. An important line of research has shown that people attend to central areas of the face, with most gaze time typically allocated to the eye region followed by the mouth or nose (e.g., Pelphrey et al., Citation2002), collectively forming a “T”-shaped pattern of fixations during perception of pictures of faces (Yarbus, Citation1967). Several studies highlight that certain gaze patterns – and more specifically, increased eye gaze – are linked with better performance on neuropsychological tests of face identification or face memory (Davis et al., Citation2017; Sekiguchi, Citation2011). Indeed, eye gaze seems to have a special relation with activation of the fusiform face area (FFA) (Hadjikhani et al., Citation2017; Morris, Pelphrey, & McCarthy, Citation2007), which is a key neural region for face perception located in the (typically right-side) inferior temporal lobe (for a review, see Kanwisher & Yovel, Citation2006). Yet, it is also well established that gaze patterns, including eye vs. mouth orientation, differ quite markedly among typically developing, healthy individuals (Gurler, Doyle, Walker, Magnotti, & Beauchamp, Citation2015) and that certain exogenous factors of the facial stimuli (e.g., type of emotion expressed or whether the person is silent or talking) also modulate gaze patterns (e.g., Åsberg Johnels et al., Citation2017; Võ, Smith, Mital, & Henderson, Citation2012).
Research on specific clinical groups has revealed important differences in face perception which might, at least partly, mediate the observed social difficulties experienced in everyday settings. Studies on individuals with diagnoses of autism (Pelphrey et al., Citation2002; Klin, Jones, Schultz, Volkmar, & Cohen, Citation2002; although see Bar-Haim, Shulman, Lamy, & Reuveni, Citation2006; Åsberg Johnels et al., Citation2017), 22q11.2 deletion syndrome (Campbell et al., Citation2010), Fragile X (Farzin, Rivera, & Hessl, Citation2009; Hong et al., Citation2019) or social anxiety disorder (Kleberg et al., Citation2017), have shown attenuated attention to the inner parts of others’ faces, especially the eyes, in these populations. In the clinical literature on face perception, a particularly striking group of individuals are those with acquired or developmental prosopagnosia, also known as “face blindness” (Kress & Daum, Citation2003). These individuals struggle with people identification and show extremely poor performance on neuropsychological tests of face memory and discrimination, which also align with aberrant scanning of the internal core features of the face, andin particular, of the eyes, during face perception (Schmalzl, Palermo, Green, Brunsdon, & Coltheart, Citation2008; Schwarzer et al., Citation2007; Sormaz, Andrews, & Young, Citation2013).
A relatively small body of recent research suggests that potential face processing alterations are also present in dyslexia, although these are most likely much subtler than in prosopagnosia. It is also possible that any eventual difficulties are contingent on certain task characteristics; in particular, during processing of facial speech movements, people with dyslexia might differ in patterns of face perception because of underlying phonological impairments (Rüsseler, Gerth, Heldmann, & Münte, Citation2015). But even paradigms involving non-speaking faces have revealed differences in face processing in dyslexia. Using fMRI, Monzalvo, Fluss, Billard, Dehaene, and Dehaene-Lambertz (Citation2012) reported reduced activation in the right FFA during perception of faces in children with dyslexia compared with skilled readers. Using a battery of neuropsychological tests, Sigurdardottir, Ívarsson, Kristinsdóttir, and Kristjánsson (Citation2015) showed that face memory performance was lower in adult participants with dyslexia than in age- and IQ-matched controls. Similarly, Gabay, Dundas, Plaut, and Behrmann (Citation2017) found that university students with dyslexia matched faces more slowly and discriminated between similar faces more poorly than controls.
The data on face processing alterations in dyslexia is far from consistent, however. Importantly, some studies have reported null findings with regard to potential differences in face processing in dyslexia (e.g., Smith-Spark & Moore, Citation2009). Nelson and Warrington (Citation1980) found no evidence for poorer delayed recognition memory for faces in children with dyslexia (n = 51) compared with skilled reading controls (n = 28). Furthermore, Liberman, Mann, Shankweiler, and Werfelman (Citation1982) explored memory performance across different classes of visual stimuli and found that poorly reading second-graders were less skilled than good readers at remembering words, whereas this was not the case for pictures of faces. However, a closer look at the participants in this seminal study shows that the “poor reading” group included children with age-typical reading skills, but who, as a group, scored lower in reading than the above-average-performing control group. In Kühn, Gerlach, Andersen, Poulsen, and Starrfelt (Citation2021), no face recognition problems were found at the group-level in a group of adolescent dyslexic readers. Uniquely, however, that study utilized norm-referenced neuropsychological tests of face perception which revealed a possibly larger-than-expected proportion of individuals with dyslexia showing face processing problems. Likewise, another recent study (Sigurdardottir, Hjartarson, Gudmundsson, & Kristjánsson, Citation2019) explored the individual differences in face processing, rather than only the mean diagnostic group performance, among dyslexic adults. They found that much of the difference between participants with and without dyslexia in face processing skill – at the group level – was driven by non-university educated adults, possibly suggesting that reduced print exposure and reading experience over time also comprises visual expertise in non-reading (e.g., face) perception. Another important perspective pointing toward the independence between reading and face processing alterations comes from research showing that individuals with prosopagnosia often perform normal on word reading tasks (for a review, see Robotham & Starrfelt, Citation2017). Taken together, important knowledge gaps exist with regard to the presence, severity, and universality of any face processing alterations in dyslexia.
In the present study, we apply a broad and unique take on the issue of face processing in children with and without dyslexia. Using a battery of neuropsychological tests, we compare children with and without dyslexia, on face perception (identification) and face memory performance. We also move beyond identification of mean performance, to present individual profiles of performance scores (c.f., Kühn et al., Citation2021; Sigurdardottir et al., Citation2019) in order to better understand the frequency and severity of any difficulties, acknowledging that face processing difficulties are unlikely to be universal in dyslexia. For the first time, we also collect eye-tracking data during face perception in order to examine whether there might be differences between dyslexic and control children in spontaneous face scanning patterns. We consistently examine perception of non-speaking faces without any demands on visual speech processing, in order not to conflate and confound well-established phonological problems with potential face processing alterations. We specifically aim to compare the two groups regarding the allocation of visual attention to inner core parts of the face (eyes and mouth). Finally, we explore the associations between eye gaze patterns during face perception and the performance in neuropsychological test of face processing.
Method
Ethics
All procedures were conducted in accordance with the Declaration of Helsinki (1964) and were approved by the local ethical committee (1090–17). Written consent from parents and verbal assent of child participants was obtained prior to testing.
Participants
Forty-six children between the ages of 9 and 13 years participated in the study. Of these, 3 children were excluded from the final sample: one child had newly arrived in the country (Sweden) and did not have adequate command of the spoken language; one child refused to continue participation; during data collection of one child, there were technical difficulties with the eye tracker. The final study sample comprised of 43 individuals of which 18 (9 boys) belonged to the ‘specific reading disorder/dyslexia’ group while 25 (8 boys) were part of the community comparison group.
Children in the dyslexia group (DYS) had been assessed by expert clinicians who identified word reading and phonological impairments, but not general language disorder, according to the ICD-10 (WHO, Citation1992). In Sweden, besides the ICD definition, the phonological model of dyslexia is widely embraced among diagnosticians (see in particular, International Dyslexia Association (cf., Lyon, Citation1995)). In general, as part of their prior diagnostic assessment, visual, and hearing impairments as well as any neurological abnormalities that would otherwise interfere with reading were ruled out at the time of the diagnosis and confirmed with parental questionnaires at intake. Of the children in the dyslexia group, 15 were recruited from the same speech-language pathology clinic where they received their diagnosis, another 3 children received their diagnosis by another clinician, including in one case by the first author.
Ten-to-twelve year old children in the comparison group (CON) were recruited from local elementary schools and were found to match the dyslexia group on listening comprehension, mean age and gender.
Parent questionnaires were used to identify any formal diagnosis, hearing, and sensory difficulties as well as those students who spoke another language at home. The Strengths and Difficulties Questionnaire (SDQ; Muris, Meesters, & van den Berg, Citation2003) was used to screen for comorbid neurodevelopmental and mental difficulties, which have been found to have a higher prevalence rate in dyslexia (Brimo et al., Citation2021; Russell, Ryder, Norwich, & Ford, Citation2015). We did not exclude children scoring high on the SDQ to maintain the representative nature of the sample. In addition, we included SDQ scores in the analysis in order to examine if and if so, how comorbid symptoms may relate to the variables of interest (e.g., Brimo et al., Citation2021; Hulme & Snowling, Citation2013).
Psychoeducational measures collected for subject characterization
Word reading
Children’s single-word reading accuracy and fluency (efficiency) were assessed using a Swedish adaptation of the Test of Word Reading Efficiency (TOWRE) called LäSt (Elwér, Fridolfsson, Samuelsson, & Wiklund, Citation2016). The ability to phonemically decode nonsensical words (nonword subscale) and the ability to quickly recognize familiar words (word subscale) are separately assessed. During the test, children in both study groups were asked twice to read out loud as many single nonwords and words as possible within a 45 second timeframe. Final scores are either raw scores or age adjusted stanine (normative mean = 5, SD = 2). Test-retest reliability = .97 in the manual on both subtests (Elwér et al., Citation2016).
Phonological processing
Phonological processing was assessed in all children using the phonological processing subtest within the language domain of the NEPSY Assessment (NEPSY; Korkman, Kirk, & Kemp, Citation1997). In this two-part assessment, children are asked to identify morphemes and phonemes from words and then to create a new word by either omitting (for example, omit ‘/dum/’ in the word ‘dumhet’) or substituting phonological elements of the word for another (for example, in the word ‘flicka’ substitute the ‘/fl/’ sound for ‘/br/’ sound). Low score on this assessment signifies reduced phonological awareness and processing. Age adjusted z-scores were calculated based on normative means and SD reported in the manual.
Listening comprehension
Children’s listening comprehension was assessed using the text comprehension subtest from the Swedish translation of the Clinical Evaluation of the Language Fundamentals – IV, CELF-4 (Semel, Wiig, & Secord, Citation2013), which is an instrument used for identifying and diagnosing disorders in language performance. Children listen to short stories (read by the experimenter) and answer short literal and inferential comprehension questions. Test–retest reliability in the manual is between .70 and .90. The scores are reported to be age-adjusted scaled scores (normative mean = 10; SD = 3) according to procedures in the manual.
Neurodevelopmental comorbidity
Parents of all participants completed the Swedish translation of the Strengths and Difficulties Questionnaire (SDQ) in order to identify psychopathological/neurodevelopmental symptoms in children and adolescents (Goodman, Citation1997). The total difficulty score has shown good psychometric properties (Cronbach’s α = 0.80, test–retest stability is 0.70, and concurrent validity with other measures is between r = 0.67–0.73 (Muris et al., Citation2003)). SDQ data are available for 39/43 children.
Neuropsychological measures of face perception skills
Along with the above-mentioned measures, participants performed two neuropsychological tasks to evaluate face perception skills: Memory for Faces (Immediate and Delayed) and a speeded visual face perception task.
Memory for faces
Memory for faces was assessed using a subscale of the Memory and Learning domain of the NEPSY Assessment (NEPSY; Korkman et al., Citation1997). In this assessment, all children were presented with series of faces in a booklet which they had to look at for at least 5 seconds. In the immediate version, they were asked to point out the previously viewed face out of the three presented at a time. In the delayed version, children had to select a previously seen face after a 30-min delay but otherwise in the same manner. The age corrected (z-score) combined scores from the subtests, with a low score signifying poorer face memory.
Speeded face identification
The Visual Attention subtest of the NEPSY Assessment measures ‘the speed and accuracy with which the child is able to focus selectively on and maintain attention to visual targets presented within an array’ (Korkman et al., Citation1997, pg. 119). The subset includes two tasks. In the first, which serves as a control task in our study, the child is presented with a visual array of different objects and is asked to identify and cross out all the images of one category (cats) within the array. In the second task, which we call the Speeded Face Identification task, the child is presented with two drawn target faces at the top of the page and is asked to cross out identical-to-target faces from the array of different faces below. The challenging aspect of this task is the similarity between the targets and the array faces. The raw score on this task is calculated as the number of correctly matched-to-target faces (and cats in the control task) as well as the time it took to complete the assignment. In the present study, raw scores (rather than standardized scores) for accuracy (number of correctly matched objects) and time it took to complete the task were calculated separately for the “cat” control task and the Speeded Face Identification task since there are no separate norms on the two subtasks (i.e., during standardized administration and interpretation, the two subtests are combined).
Eye-tracking of spontaneous face gaze patterns
In addition to the neuropsychological measures of face perception skills, eye gaze patterns during observation of a human face were recorded. Gaze measures were collected using a mobile Tobii X2-30 eye tracker (Tobii Technology Inc., Danderyd, Sweden), which records near-infrared reflections of both eyes at 30 Hz as the subject watched a 17-inch (33.7 × 27 cm) laptop monitor. Following 9-point calibration procedure, all participants were presented with a 10-s video depicting a silent female actor with a direct gaze and natural expression. Participants were simply told to view the presented video. Video recording, rather than a still photograph, was used in order to allow natural movements, such as eye blinks, to occur even though the actor was otherwise non-moving. The stimulus and data collection were conducted in the context of a larger project, parts of which focused on visual speech (Galazka, Hadjikhani, Sundqvist & Åsberg Johnels, Citation2021). Stimulus presentation and gaze recording were collected using iMotions software (iMotions A/S, Copenhagen, Denmark).
Data analysis and statistical analysis
Data files from iMotions were processed to determine fixation durations occurring within specified areas of interest for each participant using the Timestudio software (Version 3.19; Nyström, Falck-Ytter, & Gredebäck, Citation2016), a MATLAB-based open access analysis tool which allows for analysis of dynamic stimuli such as the one used here. To track gaze patterns, areas of interest (AOIs) around the face and its internal core structures (mouth and eyes) were defined manually. The area of interest (AOI) around the face (Face AOI) was an elliptical shape encompassing the speakers face from the top of her forehead, excluding her hair, to the bottom of her chin, measuring 7.54 horizontal by 8.26 vertical visual degrees (440 × 480 pixels). The Mouth AOI was a rectangle measuring 3.43 horizontal by 1.72 vertical visual degrees (200 × 100 pixels). Eyes AOI was a rectangle measuring 7.36 horizontal by 2.38 vertical visual degrees (440 × 100 pixels). See .
The dependent measure for the spontaneous face gaze tracking was the proportion of looking time at the Eyes AOI and Mouth AOI divided by the total fixations at the Face AOI, such that:
(Face AOI + Mouth AOI)/Face AOI. Fixations to the face were calculated as the proportion of fixations within the Face AOI relative to the Screen AOI (32 × 18.4 visual degrees; 1920 × 1080 pixels), such that Face AOI/Screen AOI.
Statistical analysis was performed in JASP (version 0.14.1; [JASP Team, 2019; jasp-stats.org]) an open-source statistical software program. Data visualization were performed with RStudio version 1.2.5033 (R Foundations for Statistical Computing), using dplyr (Wickham, François, Henry, & Müller, Citation2019a), ggplot2 (Wickham et al., Citation2019b) and ggstatsplot (Patil, Citation2018) packages. Bayes estimations were performed using default priors (Cauchy r = 0.707) based on data augmentation algorithm with 5 chains of 10000 iterations.
Due to unequal variances and non-normality as well as to assess the robustness of the results, we report results of the Mann-Whitney U test to compare group differences. Since we are interested in similarities as well as differences between groups, we also report Bayes factors expressing the probability of the observed data, given null hypothesis relative to alternative (i.e., values larger than 1 are in favor of the null hypothesis). In the present case, the null hypothesis indicates no difference between DYS and CON groups, while the alternative hypothesis states that the two groups differ. Finally, in order to explore whether individual cases differed from others in the group, we conducted Crawford & Howell’s (Citation1998) t-tests.
Results
Subject characteristics
Subject characteristics are presented in . As expected, the two groups differed significantly on the measures of word-reading efficiency (LäSt; words and nonwords subscales) and phonological processing, with the DYS group scoring characteristically low while the CON group scored very close to normative levels. There was also a significant difference in the SDQ total scores, with parents of DYS children reporting higher behavioral problems compared to the parents of children in the CON group. By contrast, the two groups were well matched on age and, importantly, on listening comprehension, with both groups, on average scoring within the age-adequate range according to population norms.
Table 1. Subject characteristics of the two study groups
Neuropsychological findings of face processing
shows a plot of individual and group performances together with statistical analysis (p-based/frequentist and Bayes estimations) for the Memory of Faces task. The results indicate that CON group (Median = 1.185) scored slightly higher on the memory for faces task than the DYS group (Median = 0.762), although the difference was not significant UMann-Whitney = 162.0, p = .123, r = −0.280. The Bayesian analysis yielded BF01 = 1.265, indicating that present sample data are approximately 1.3 times more likely to have occurred under null (no difference) than alternative hypothesis (difference), therefore showing weak evidence for the presence of either a difference or similarity between groups. For parameter estimations, δ of the resulting posterior distribution equals −0.386 with a central 95% credible interval (CI) ranging from – 0.994 to 0.160. Inspecting the individual scores in shows a great variation in performance on the face memory task in the DYS group. In particular, we note that three individuals in the DYS group clearly struggle on the task, with scores more than one standard deviation (i.e., z-scores < 1.0) than the normative mean, whereas this was not the case for anyone in the CON group. Conversely, however, it is clear that some individuals with dyslexia excel on the task, with one participant scoring more than two standard deviations above the normative mean on the task.
Figure 2. The normalized scores from the memory for faces (NEPSY) DYS and CON groups. The figure includes frequentist and Bayesian statistics.
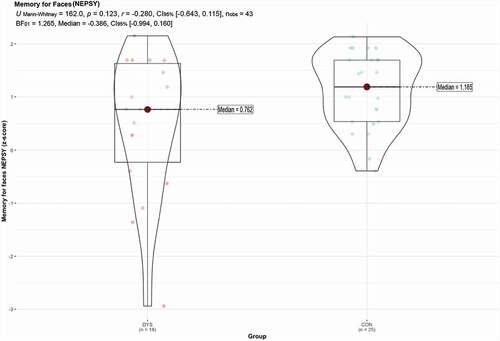
specifies statistical and Bayes estimations for the Speeded Face Identification task. The results showed that DYS children are not statistically less accurate in correctly matching faces to target (Median = 15.0) compared to the CON children (Median = 17.0), p = .399, with BF01 = 2.54 in favor of the null hypothesis. DYS children were, however, slower in completing the task (Median = 119.0 sec) than CON children (Median = 91.0 sec), p = .025, and with BF01 = 0.7 in favor of the alternative hypothesis. There were no significant group differences on the control task, in which they searched for cats, either in accuracy (U = 209, p = .635, r = −0.071, CI[−0.401, 0.275] or time to completion (U = 277.5, p = .199, r = 0.233 CI[−0.116, 0.531]). Acknowledging that there are some differences in task structure and complexity (e.g., identifying two versus one targets), this result suggested that the group difference on the speed of face identification is specific to faces.
Figure 3. Distribution graphs of total number correct and time in the Speeded face identification task in DYS and CON groups with frequentist and Bayesian statistics.
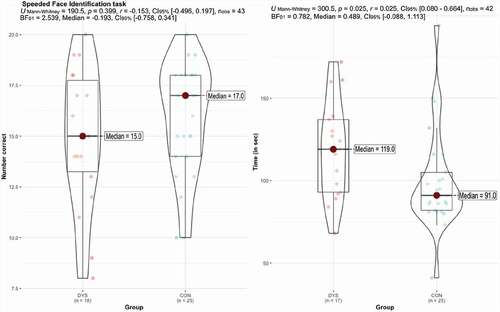
The Bayesian analysis confirmed these findings with accuracy measure yielding BF01 = 2.7, δ = −0.109, 95% CI [- 0.743, .491] and time to completion yielded BF01 = 1.9, δ = 0.27, 95% CI [- 0.268, .862]. However, again the variation within both groups is noteworthy, so even if the DYS group scored somewhat more poorly on the speeded aspect of this face processing task, many individuals scored well within the range of the CON group as can be seen on the right side of .
Eye-tracking of spontaneous face gaze patterns
Results from the eye-tracking measures indicate no significant group differences in the proportion of fixation duration at the face in general (p = .166) nor specific facial features, namely the eyes (p = .567) and the mouth (p = .980) with Bayes estimates BF01 = 1.95, BF01 = 2.9, and BF01 = 3.2, respectively, in clear favor of the null hypothesis (). Overall, the results show prioritized attention to the inner face (median > 90% in both groups). Approximately half of the total gaze time was devoted to the eye region of the person, but with notable individual differences in both groups.
Correlations
For the CON group, fixations to the eye region during face perception were associated with better neuropsychological test performance on the Memory for Faces task (rs = .409, p = .042) but not on the Speeded Face Identification task (accuracy, p = .775 and speed, p = .331). Neither the amount of fixations to the mouth (all ps >.2) nor face in general (ps >.06) correlated with the neuropsychological face processing measures.
For the DYS group, there were no significant associations between the amount of time spent fixating on the eyes and the neuropsychological face processing measures (all ps >.4). Neither the amount of fixating on the mouth nor the face in general correlated with any of the neuropsychological face processing measures in the DYS group, with the possible exception of an inverse relationship between mouth gazing and the time used to complete Speeded Face Identification task (rs = −.446, p = .074). The direction of that non-significant trend correlation means that the more they looked at the mouth, the faster they were at completing the face identification task.
In regard to neurodevelopmental comorbidity, SDQ scores did not correlate with either neuropsychological or eye-tracking-based face processing measures in either group (all ps > .16).
Finally, we explored characteristics of the three participants in the dyslexia group that scored 1 SD or more below the normative mean on the NEPSY face memory test. There was no clear shared profile of these three children regarding performance on the speeded face identification measure, their eye gaze patterns, or their SDQ scores. According to Crawford and Howell’s t-tests (Citation1998) the three cases did not significantly differ from the others in the dyslexia group on any of these measures (all ps > .1).
Discussion
The current work was motivated by recent findings showing face processing alterations in adults with dyslexia, a term used to refer to individuals with developmental word reading difficulties commonly associated with phonological impairments (e.g., Snowling et al., Citation2020). We administrated two neuropsychological tests indexing face memory and face identification, the latter of which yielded an accuracy and a speed-based performance score. Frequentist and Bayesian statistics revealed, with one exception, no – or only quite subtle – group differences when comparing children with dyslexia to age- and listening comprehension matched community controls. The one significant group difference was on the speed score, suggesting that children with dyslexia are slower at face identification. Noteworthy, however, was the very considerable individual variation within the dyslexia group on the face memory task. At a general level, this finding underscores that the “averaging artefact” is a constant threat in research on neurodevelopmental and learning disabilities, meaning that the mean score on a certain test does not necessarily well represent the performance of any one single participant (e.g., Brock, Citation2011). Scrutinizing individual profiles of performance showed that there were some children in the DYS group who scored poorly on the face processing tasks; on the norm-referenced test 3/18 (17%) performed below the normal range. Strikingly, however, several other children in the DYS group performed well above the average range, according to the norms on the face memory task and/or relative to the performance of the community controls, on all three performance scores. Hence, struggling to read does not rule out the possibility of excelling in face processing, a finding which confirms the relative developmental and processing independence of these two sets of skills (cf., Robotham & Starrfelt, Citation2017). Compared with prior research in the field, methodologically the current study is most similar to the recent one by Kühn et al. (Citation2021), which focused on a developmental (child) population rather than on (young) adults (e.g., Gabay et al., Citation2017; Sigurdardottir et al., Citation2019, Citation2015) and which also, as is our case here, moved beyond identification of mean performance to present individual profiles of neuropsychological performance scores of face processing. Overall, our results on the neuropsychological tests are strikingly similar to those presented Kühn et al.; for instance, in their study of 24 high-school students with dyslexia, the individual variation was similarly large with 4 (17%) displaying poor face processing. Compared with results in Sigurdardottir et al. (Citation2015) and Gabay et al. (Citation2017) our current sample did not display the same type of general face processing alteration. Speculatively, age might play a role in the difference to these studies. It has been shown, outside the specific context of face perception, that many cognitive deficits associated with developmental dyslexia may be a consequence of reduced and suboptimal reading experience (Huettig, Lachmann, Reis, & Petersson, Citation2018), which is likely to compound over time. Clearly, longitudinal studies would be helpful in teasing out the developmental relations between these sets of skills and the extent to which face processing alterations are dependent on age and reading experience. At the same time, we were able to identify, at group level, difficulties on the speeded aspect of the visual face identification task in the current dyslexia group, and there were individual cases who performed poorly on the face memory task as well. The question then arises as to why this might be the case. Kühn et al. argue, and we agree, that this question relates to the broader issue of why developmental problems more generally tend to co-occur at a higher than expected rate (Brimo et al., Citation2021; Gillberg, Citation2010).
We hypothesized that gaze patterns might play a role in group differences, but this was not the case. In terms of face gaze patterns, our study is the first to utilize eye-tracking technology to explore how individuals with and without dyslexia deploy visual attention during face perception. We confirmed that the participants prioritized attention to the face in general and to the eye region in particular, and less toward the mouth, as has been shown in prior research on individuals without dyslexia (e.g., Åsberg Johnels et al., Citation2017; Pelphrey et al., Citation2002). Importantly, p-based and Bayesian statistics showed that the groups with and without dyslexia were more similar than different in their gaze patterns. Thus, to the extent children with dyslexia display any face perception difficulties, these do not seem to be obviously associated with underlying gaze alterations. In this sense, the case of dyslexia might differ from other clinical groups with much more universal alterations in face perception, in conditions such as autism (Fedor et al., Citation2018) or prosopagnosia (Schmalzl et al., Citation2008; Schwarzer et al., Citation2007). For instance, Schmalzl et al. (Citation2008) reported on the successful treatment outcome for a girl with severe developmental prosopagnosia; interestingly, the remediation of her face processing difficulty was clearly associated with increased attention to the eye region during face perception. Our findings suggest that it is not obvious that such treatment regimens involving gaze pattern training will be helpful for dyslexic readers with face perception difficulties.
We found an association between spontaneous face gaze patterns and neuropsychological test scores of face processing in correlational analyses, that was present in the community controls only. A number of potential weaknesses should be acknowledged regarding the eye-tracking data collected that could affect the associations between eye-tracking and neuropsychological measures in our study. First, while our eye-tracking equipment has a strength in its low intrusiveness, the sampling resolution is too low for fine-grained temporal or spatial analyses of saccades, individual scanpaths, or for very precise gaze location identification (such as just below one eye (Peterson & Eckstein, Citation2012)). Also, eye gaze patterns were not recorded simultaneously to the neuropsychological tasks but with a separate in-house created face-stimuli. A third potential limitation that needs to be considered is the lack of information regarding the psychometric properties of the eye-tracking data on the face gaze task; while the development of such knowledge is certainly important for future eye-tracking and psychophysiological research more generally, several existing studies have pointed to there being stable individual differences in face gaze patterns among children and in clinical groups (e.g., Reimann et al., Citation2021). Even so, and as mentioned, in the community controls, we did show that increased eye gaze aligned with better face memory performance. This finding replicates a general literature in which individuals with higher face recognition, face learning, or face memory ability to direct their gaze to the eye region more frequently compared to those with lower face processing ability (Davis et al., Citation2017; Sekiguchi, Citation2011). For instance, one analysis of typically developing children and adults considered together found that performance on the face recognition was positively associated with the number of fixations made to the eyes during immediate recognition memory trials (Fedor et al., Citation2018). As of yet, however, there are surprisingly few studies exploring this association in a pure developmental (child) sample, thus our study makes a novel contribution in this regard.
In conclusion, we show that children with dyslexia do not perform significantly worse than control children in face memory and in accuracy during a face recognition task, but that they seem to work more slowly on that latter task. No group differences were evidenced in eye-tracking data of spontaneous eye, mouth, and overall face gazing. We confirm previous studies indicating that increased gaze patterns toward the eyes are associated with better face recognition, but this was only seen in the community controls. We also demonstrate that the individual variations in the group of children with dyslexia are much more pronounced than those in the control group, underlining the important issue of a danger of an averaging artifact when examining individuals with neurodevelopmental issues. While some of the dyslexic children performed worse than controls, other actually excelled on the tasks, demonstrating that there is no systematic association between dyslexia and face identification or memory, or in particular between dyslexia and alterations in gaze patterns during face processing. Therefore, approaches aiming at improving the amount of time spent in the eye region may not be of any benefit in a large proportion of children with dyslexia.
Consent to participate
Written informed consent was obtained from the parents of all participants and verbal assent was obtained by the participants.
Consent to publish
No identifiable details of the minor participants or their parents, such as videos or photographs, were used in the manuscript.
Ethical approval
Swedish Ethical Review Authority (Etikprövningsmyndigheten #1090-17).
Availability of data and material
Material for the eyetracking task and code is available upon request of the corresponding authors. The conditions of our ethics approval do not permit public archiving of anonymized study data. Access will be granted to named individuals in accordance with ethical procedures governing the reuse of sensitive data. Please contact Jakob Åsberg Johnels and/or personal data representative at the University of Gothenburg.
Acknowledgments
We thank the children, parents, and the teachers who made this project possible. We would also like to extend our gratitude to our colleague Emilia Carlsson, Ph.D., for agreeing to model as the “face stimuli” in the experiment. Finally, we are grateful to our funding agencies, specifically grants from Lennart “Aktiestinsen” Israelssons stiftelse to JÅJ and the Swedish Research Council (2018-04115) to the research group.
Disclosure statement
No potential conflict of interest was reported by the author(s).
Additional information
Funding
References
- Åsberg Johnels, J., Hovey, D., Zürcher, N., Hippolyte, L., Lemonnier, E., Gillberg, C., & Hadjikhani, N. (2017). Autism and emotional face‐viewing. Autism Research, 10(5), 901–910. doi:https://doi.org/10.1002/aur.1730
- Bar-Haim, Y., Shulman, C., Lamy, D., & Reuveni, A. (2006). Attention to eyes and mouth in high-functioning children with autism. Journal of Autism and Developmental Disorders, 36(1), 131–137. doi:https://doi.org/10.1007/s10803-005-0046-1
- Brimo, K., Dinkler, L., Gillberg, C., Lichtenstein, P., Lundström, S., & Åsberg Johnels, J. (2021). The co‐occurrence of neurodevelopmental problems in dyslexia. Dyslexia, 27(3), 277–293. doi:https://doi.org/10.1002/dys.1681
- Brock, J. (2011). Commentary: Complementary approaches to the developmental cognitive neuroscience of autism—Reflections on Pelphrey et al. Journal of Child Psychology and Psychiatry, 52(6), 645–646. doi:https://doi.org/10.1111/j.1469-7610.2011.02414.x
- Campbell, L., McCabe, K., Leadbeater, K., Schall, U., Loughland, C., & Rich, D. (2010). Visual scanning of faces in 22q11. 2 deletion syndrome: Attention to the mouth or the eyes? Psychiatry Research, 177(1–2), 211–215. doi:https://doi.org/10.1016/j.psychres.2009.06.007
- Crawford, J. R., & Howell, D. C. (1998). Comparing an individual’s test score against norms derived from small samples. The Clinical Neuropsychologist, 12(4), 482–486. doi:https://doi.org/10.1076/clin.12.4.482.7241
- Davis, J., McKone, E., Zirnsak, M., Moore, T., O’Kearney, R., Apthorp, D., & Palermo, R. (2017). Social and attention‐to‐detail subclusters of autistic traits differentially predict looking at eyes and face identity recognition ability. British Journal of Psychology, 108(1), 191–219. doi:https://doi.org/10.1111/bjop.12188
- Elwér, Å., Fridolfsson, I., Samuelsson, S., & Wiklund, C. (2016). Läst- test i läsförståelse, läsning och stavning för årskurs 1–6. Stockholm, Sweden: Hogrefe.
- Farzin, F., Rivera, S. M., & Hessl, D. (2009). Brief report: visual processing of faces in individuals with fragile X syndrome: An eye tracking study. Journal of Autism and Developmental Disorders, 39(6), 946–952. doi:https://doi.org/10.1007/s10803-009-0744-1
- Fedor, J., Lynn, A., Foran, W., DiCicco-Bloom, J., Luna, B., & O’Hearn, K. (2018). Patterns of fixation during face recognition: Differences in autism across age. Autism, 22(7), 866–880. doi:https://doi.org/10.1177/1362361317714989
- Gabay, Y., Dundas, E., Plaut, D., & Behrmann, M. (2017). Atypical perceptual processing of faces in developmental dyslexia. Brain and Language, 173, 41–51. doi:https://doi.org/10.1016/j.bandl.2017.06.004
- Galazka, M. A., Hadjikhani, N., Sundqvist, M., & Åsberg Johnels, J. (2021). Facial speech processing in children with and without dyslexia. Annals of Dyslexia, 71, 501–524.
- Gillberg, C. (2010). The Essence in child psychiatry: Early symptomatic syndromes eliciting neurodevelopmental clinical examinations. Research in Developmental Disabilities, 31(6), 1543–1551. doi:https://doi.org/10.1016/j.ridd.2010.06.002
- Glazzard, J. (2010). The impact of dyslexia on pupils’ self‐esteem. Support for Learning, 25(2), 63–69. doi:https://doi.org/10.1111/j.1467-9604.2010.01442.x
- Goodman, R. (1997). The strengths and difficulties questionnaire: A research note. Journal of Child Psychology and Psychiatry, 38(5), 581–586. doi:https://doi.org/10.1111/j.1469-7610.1997.tb01545.x
- Gurler, D., Doyle, N., Walker, E., Magnotti, J., & Beauchamp, M. (2015). A link between individual differences in multisensory speech perception and eye movements. Attention, Perception & Psychophysics, 77(4), 1333–1341. doi:https://doi.org/10.3758/s13414-014-0821-1
- Hadjikhani, N., Zurcher, N. R., Lassalle, A., Hippolyte, L., Ward, N., & Johnels, J. Å. (2017). The effect of constraining eye-contact during dynamic emotional face perception—an fMRI study. Social Cognitive and Affective Neuroscience, 12(7), 1197–1207. doi:https://doi.org/10.1093/scan/nsx046
- Hong, M. P., Eckert, E. M., Pedapati, E. V., Shaffer, R. C., Dominick, K. C., Wink, L. K., … Erickson, C. A. (2019). Differentiating social preference and social anxiety phenotypes in fragile X syndrome using an eye gaze analysis: A pilot study. Journal of Neurodevelopmental Disorders, 11(1), 1–10. doi:https://doi.org/10.1186/s11689-019-9262-4
- Huettig, F., Lachmann, T., Reis, A., & Petersson, K. M. (2018). Distinguishing cause from effect–many deficits associated with developmental dyslexia may be a consequence of reduced and suboptimal reading experience. Language, Cognition and Neuroscience, 33(3), 333–350. doi:https://doi.org/10.1080/23273798.2017.1348528
- Hulme, C., & Snowling, M. J. (2013). Developmental disorders of language learning and cognition. Hoboken, New Jersey: John Wiley & Sons.
- Humphrey, N., & Mullins, P. M. (2002). Self-concept and self-esteem in developmental dyslexia. Journal of Research in Special Educational Needs, 2(2), no–no. doi:https://doi.org/10.1111/j.1471-3802.2002.00163.x
- Kanwisher, N., & Yovel, G. (2006). The fusiform face area: A cortical region specialized for the perception of faces. Philosophical Transactions of the Royal Society B: Biological Sciences, 361(1476), 2109–2128. doi:https://doi.org/10.1098/rstb.2006.1934
- Kleberg, J. L., Högström, J., Nord, M., Bölte, S., Serlachius, E., & Falck-Ytter, T. (2017). Autistic traits and symptoms of social anxiety are differentially related to attention to others’ eyes in social anxiety disorder. Journal of Autism and Developmental Disorders, 47(12), 3814–3821. doi:https://doi.org/10.1007/s10803-016-2978-z
- Klin, A., Jones, W., Schultz, R., Volkmar, F., & Cohen, D. (2002). Visual fixation patterns during viewing of naturalistic social situations as predictors of social competence in individuals with autism. Archives of General Psychiatry, 59(9), 809–816. doi:https://doi.org/10.1001/archpsyc.59.9.809
- Korkman, M., Kirk, U., & Kemp, S. (1997). NEPSY: A developmental neuropsychological assessment – Manual. San Antonio, TX: Psychological Corporation.
- Kress, T., & Daum, I. (2003). Developmental prosopagnosia: A review. Behavioural Neurology, 14(3, 4), 109–121. doi:https://doi.org/10.1155/2003/520476
- Kühn, C. D., Gerlach, C., Andersen, K. B., Poulsen, M., & Starrfelt, R. (2021). Face recognition in developmental dyslexia: Evidence for dissociation between faces and words. Cognitive Neuropsychology, 38(1), 107–115. doi:https://doi.org/10.1080/02643294.2020.1847060
- Liberman, I. Y., Mann, V. A., Shankweiler, D., & Werfelman, M. (1982). Children’s memory for recurring linguistic and nonlinguistic material in relation to reading ability. Cortex, 18(3), 367–375. doi:https://doi.org/10.1016/S0010-9452(82)80035-X
- Lyon, G. R. (1995). Toward a definition of dyslexia. Annals of Dyslexia, 45(1), 1–27. doi:https://doi.org/10.1007/BF02648210
- Lyytinen, H., Erskine, J., Hämäläinen, J., Torppa, M., & Ronimus, M. (2015). Dyslexia—early identification and prevention: Highlights from the Jyväskylä longitudinal study of dyslexia. Current Developmental Disorders Reports, 2(4), 330–338. doi:https://doi.org/10.1007/s40474-015-0067-1
- Monzalvo, K., Fluss, J., Billard, C., Dehaene, S., & Dehaene-Lambertz, G. (2012). Cortical networks for vision and language in dyslexic and normal children of variable socio-economic status. Neuroimage, 61(1), 258–274. doi:https://doi.org/10.1016/j.neuroimage.2012.02.035
- Morris, J. P., Pelphrey, K. A., & McCarthy, G. (2007). Controlled scanpath variation alters fusiform face activation. Social Cognitive and Affective Neuroscience, 2(1), 31–38. doi:https://doi.org/10.1093/scan/nsl023
- Muris, P., Meesters, C., & van den Berg, F. (2003). The strengths and difficulties questionnaire (SDQ). European Child & Adolescent Psychiatry, 12(1), 1–8. doi:https://doi.org/10.1007/s00787-003-0298-2
- Nelson, H. E., & Warrington, E. K. (1980). An investigation of memory functions in dyslexic children. British Journal of Psychology, 71(4), 487–503. doi:https://doi.org/10.1111/j.2044-8295.1980.tb01762.x
- Nordström, T., Jacobson, C., & Söderberg, P. (2016). Early word decoding ability as a longitudinal predictor of academic performance. European Journal of Psychology of Education, 31(2), 175–191. doi:https://doi.org/10.1007/s10212-015-0258-5
- Nyström, P., Falck-Ytter, T., & Gredebäck, G. (2016). The TimeStudio project: An open source scientific workflow system for the behavioral and brain sciences. Behavior Research Methods, 48(2), 542–552. doi:https://doi.org/10.3758/s13428-015-0616-x
- Patil, I. (2018). ggstatsplot: ‘ggplot2’ based plots with statistical details. CRAN. Retrieved https://cran.r-project.org/web/packages/ggstatsplot/index.html
- Pelphrey, K. A., Sasson, N. J., Reznick, J. S., Paul, G., Goldman, B. D., & Piven, J. (2002). Visual scanning of faces in autism. Journal of Autism and Developmental Disorders, 32(4), 249–261. doi:https://doi.org/10.1023/A:1016374617369
- Peterson, M. F., & Eckstein, M. P. (2012). Looking just below the eyes is optimal across face recognition tasks. Proceedings of the National Academy of Sciences, 109(48), E3314–E3323. doi:https://doi.org/10.1073/pnas.1214269109
- Reid, V. M., Dunn, K., Young, R. J., Amu, J., Donovan, T., & Reissland, N. (2017). The human fetus preferentially engages with face-like visual stimuli. Current Biology, 27(12), 1825–1828. doi:https://doi.org/10.1016/j.cub.2017.05.044
- Reimann, G. E., Walsh, C., Csumitta, K. D., McClure, P., Pereira, F., Martin, A., & Ramot, M. (2021). Gauging facial feature viewing preference as a stable individual trait in autism spectrum disorder. Autism Research, 14(8), 1670–1683. doi:https://doi.org/10.1002/aur.2540
- Robotham, R. J., & Starrfelt, R. (2017). Face and word recognition can be selectively affected by brain injury or developmental disorders. Frontiers in Psychology, 8, 1547. doi:https://doi.org/10.3389/fpsyg.2017.01547
- Rüsseler, J., Gerth, I., Heldmann, M., & Münte, T. F. (2015). Audiovisual perception of natural speech is impaired in adult dyslexics: An ERP study. Neuroscience, 287, 55–65. doi:https://doi.org/10.1016/j.neuroscience.2014.12.023
- Russell, G., Ryder, D., Norwich, B., & Ford, T. (2015). Behavioural difficulties that co-occur with specific word reading difficulties: A UK Population- based cohort study. Dyslexia, 21(2), 123–141. doi:https://doi.org/10.1002/dys.1496
- Schmalzl, L., Palermo, R., Green, M., Brunsdon, R., & Coltheart, M. (2008). Training of familiar face recognition and visual scan paths for faces in a child with congenital prosopagnosia. Cognitive Neuropsychology, 25(5), 704–729. doi:https://doi.org/10.1080/02643290802299350
- Schwarzer, G., Huber, S., Grueter, M., Grueter, T., Groß, C., Hipfel, M., & Kennerknecht, I. (2007). Gaze behaviour in hereditary prosopagnosia. Psychological Research, 71(5), 583–590. doi:https://doi.org/10.1007/s00426-006-0068-0
- Sekiguchi, T. (2011). Individual differences in face memory and eye fixation patterns during face learning. Acta Psychologica, 137(1), 1–9. doi:https://doi.org/10.1016/j.actpsy.2011.01.014
- Semel, E., Wiig, E. H., & Secord, W. A. (2013). Clinical evaluation of language fundamentals, fourth edition (CELF-4), Swedish version. Stockholm, Sweden: Pearson Assessment.
- Sigurdardottir, H. M., Hjartarson, K. H., Gudmundsson, G. L., & Kristjánsson, Á. (2019). Own-race and other-race face recognition problems without visual expertise problems in dyslexic readers. Vision Research, 158, 146–156. doi:https://doi.org/10.1016/j.visres.2019.02.010
- Sigurdardottir, H. M., Ívarsson, E., Kristinsdóttir, K., & Kristjánsson, Á. (2015). Impaired recognition of faces and objects in dyslexia: Evidence for ventral stream dysfunction? Neuropsychology, 29(5), 739. doi:https://doi.org/10.1037/neu0000188
- Smith-Spark, J. H., & Moore, V. (2009). The representation and processing of familiar faces in dyslexia: Differences in age of acquisition effects. Dyslexia, 15(2), 129–146. doi:https://doi.org/10.1002/dys.365
- Snowling, M. J., Hulme, C., & Nation, K. (2020). Defining and understanding dyslexia: Past, present and future. Oxford Review of Education, 46(4), 501–513. doi:https://doi.org/10.1080/03054985.2020.1765756
- Sormaz, M., Andrews, T., & Young, A. (2013). Contrast negation supports the importance of the eye region for holistic representations of facial identity. Journal of Vision, 13(9), 97-97. doi:https://doi.org/10.1167/13.9.97
- Võ, M. L. H., Smith, T. J., Mital, P. K., & Henderson, J. M. (2012). Do the eyes really have it? Dynamic allocation of attention when viewing moving faces. Journal of Vision, 12(13), 3-3. doi:https://doi.org/10.1167/12.13.3
- Webb, S. J., Neuhaus, E., & Faja, S. (2017). Face perception and learning in autism spectrum disorders. Quarterly Journal of Experimental Psychology, 70(5), 970–986. doi:https://doi.org/10.1080/17470218.2016.1151059
- Wickham, H., Chang, W., Henry, L., Pedersen, T. L., Takahashi, K., Wilke, C., & Woo, K. (2019b). ggplot2: Create elegant data visualisations using the grammar of graphics. Retrieved https://CRAN.R-project.org/package=ggplot2.
- Wickham, H., François, R., Henry, L., & Müller, K. (2019a). dplyr: A grammar of data manipulation. Retrieved https://CRAN.R-project.org/package=dplyr.
- World Health Organization. (1992). The ICD-10 classification of mental and behavioural disorders: Clinical descriptions and diagnostic guidelines. Geneva, Switzerland: Author. https://apps.who.int/iris/handle/10665/37958
- Yarbus, A. L. (1967). Eye movements during perception of complex objects A.L. Yarbus . In Eye movements and vision (pp. 171–211). Boston, MA: Springer.