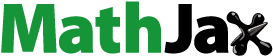
Abstract
Objective
In neuropsychological assessment, predicting the level of premorbid functioning is useful in ascertaining the presence or absence of cognitive decline post‐injury. This study set out to develop regression equations using the National Adult Reading Test (NART) in association with demographic factors to predict the Wechsler Adult Intelligence Scale—Fourth Edition (WAIS‐IV) full‐scale intelligence quotient (FSIQ) and its index scores in a cognitively healthy Australian sample, and to compare the accuracy of these regression equation with the Test of Premorbid Functioning (TOPF) in a clinical sample.
Method
The 111 cognitively healthy male and female participants aged 18–65-years completed a battery of tests, including the WAIS‐IV, NART, and TOPF. The accuracy of the premorbid IQ estimates was then examined on 22 neurologically impaired participants.
Results
The regression equation using NART errors explained 39.1% of the variance in WAIS‐IV FSIQ. When age, sex, and education were added to the model, the variance increased to 46.6%. Both the regression equations using NART errors and the TOPF significantly over‐estimated IQ in the clinical sample (p < .001), whereas the regression equation combining errors and demographics, and the TOPF with demographic correction, did not.
Conclusion
Estimating premorbid functioning is an important aspect of neuropsychological assessment. This paper developed a series of regression equations using the NART which are suitable for the Australian context.
Key words:
What is already known about this topic
Tests of premorbid functioning are useful in determining the baseline level of cognitive function.
Regression equations have been developed and combined with demographic data in the attempt to increase the predictive accuracy of these tests.
However, there is currently no equation for predicting IQ on the WAIS‐IV that has been standardised on an Australian sample.
What this topic adds
The present study developed two regression equations using an Australian sample, and updated the NART‐2 to predict WAIS‐IV FSIQ scores.
It also compared the premorbid IQ estimates produced by the NART, NART‐2, WTAR, and TOPF with WAIS‐IV FSIQ scores.
The ability of premorbid functioning tests to predict premorbid WAIS‐IV index scores was also investigated.
Neuropsychological assessments are used to examine the extent of cognitive decline post brain‐injury (Franzen, Burgess, & Smith‐Seemiller, Citation1997; Lezak, Howieson, Bigler, & Tranel, Citation2012). As the current cognitive performance of an injured client can be affected by the injury, it is useful to develop an estimate of their ability preceding an injury with which current performance can be compared (Babcock, Citation1930; Lezak et al., Citation2012; McFie, Citation1975; Wechsler, Citation1958). Current ability is often measured using tests such as the Wechsler Adult Intelligence Scale—Fourth Edition (WAIS‐IV; Wechsler, Citation2008). Ideally, pre‐injury measures of cognitive functioning should be employed as a comparison, to quantify the extent of cognitive decline post injury; however this information is rarely available (Baade & Schoenberg, Citation2004; Barker‐Collo, Thomas, Riddick, & de Jager, Citation2011). As a result, several measures have been developed in the attempt to predict pre‐injury levels of functioning.
The National Adult Reading Test (NART; Nelson, Citation1982), the restandardised NART (NART‐2; Nelson & Willison, Citation1991), the Wechsler Test of Adult Reading (WTAR; Wechsler, Citation2001), and the Test of Premorbid Functioning (TOPF; PsychCorp, Citation2009) are tasks commonly used to predict premorbid intelligence quotient (IQ). These measures use reading tests employing words with irregular grapheme‐to‐phoneme translation to estimate premorbid functioning.
These tests are based on several premises: (1) reading level is highly correlated with intelligence, and as intelligent people have a more advanced education, and thus more exposure to irregular words, they therefore perform better (Franzen et al., Citation1997). (2) The reading of irregular words is more resistant to cognitive decline than is the reading of regular words (Franzen et al., Citation1997); and (3) reading irregular words relies upon prior (i.e., pre‐injury) knowledge and minimises demands on current cognitive ability (Willshire, Kinsella, & Prior, Citation1991), and are thus less sensitive to the effects of injury.
Other methods for estimating premorbid cognitive functioning include the use of demographic variables alone (Barona, Reynolds, & Chastain, Citation1984; Reynolds & Gutkin, Citation1979; Schoenberg, Lange, Brickell, & Saklofske, Citation2007; Vanderploeg, Schinka, Baum, Tremont, & Mittenberg, Citation1998), using previous academic achievement scores (Baade & Schoenberg, Citation2004), and using ‘hold’ tests, which often use subtests from tests of current ability, including the Wechsler Intelligence scales (Larrabee, Largen, & Levin, Citation1985; Vanderploeg & Schinka, Citation1995). Although a variety of techniques are available, there is as yet no ‘gold standard’ for predicting premorbid functioning (Powell, Brossart, & Reynolds, Citation2003; Schoenberg, Scott, Ruwe, Patton, & Adams, Citation2004), with each method having both strengths and weaknesses depending on the context in which they are employed (i.e., patient samples and cultural populations) (Patterson, Graham, & Hodges, Citation1994; PsychCorp, Citation2001). For example, it is recommended by the administration manual that the WTAR not be administered to individuals with reading difficulties, developmental language disorders, paediatric brain insult, aphasia, alexia, and those with non‐English speaking backgrounds (PsychCorp, Citation2001).
The ability of reading tests to predict premorbid IQ has been examined in both clinical and non‐clinical populations. Nebes, Martin, and Horn (Citation1984) reported no significant discrepancies between NART scores obtained by participants with Alzheimer's disease when compared to healthy controls. When compared to alternate methods of predicting premorbid IQ, the literature regarding the use of reading tests over other measures is inconsistent. For example, Bright, Jaldow, and Kopelman (Citation2002) found that premorbid IQ estimates produced by demographic variables differed more from current WAIS FSIQ scores than did the NART and NART‐2 predictions for both control and clinical groups.
Tests of premorbid functioning have also been compared with each other. Mathias, Bowden, and Barrett‐Woodbridge (Citation2007) examined the accuracy of NART‐2 and WTAR estimates of WAIS‐III FSIQ in a neurologically healthy, Australian sample and found significant discrepancies between current and predicted IQ scores. Mathias, Bowden, Bigler, and Rosenfeld (Citation2007) also found that WTAR estimates were affected in participants with traumatic brain injury (TBI).
Some studies have also examined the contribution of demographic factors on premorbid prediction. Crawford, Parker, Stewart, Besson, and De Lacy (Citation1989) for example found that the addition of age, sex, education, and occupation to the NART regression equation increased the proportion of variance explained in predicted IQ scores from 66% for NART errors alone to 73%. Watt and O'Carroll (Citation1999) also found that the addition of education to the NART‐2 regression equation increased the percentage of the variance explained in WAIS‐R FSIQ from 30% to 42%, and from 51% to 60% for verbal IQ (VIQ) when socioeconomic status, age, and education were entered into the equation. It has therefore been suggested that a combination of demographic variables in conjunction with reading error scores provides the most reliable estimate of an individual's premorbid IQ.
However, regression equations may not be useful for predicting premorbid IQ when applying these to populations on which they have not been standardised (Harnett, Godfrey, & Knight, Citation2004). The NART and NART‐2 were developed and normed in Great Britain, meaning scoring procedures were based upon British pronunciation conventions, which may not be applicable to other populations, such as those in the United States and possibly now, even in Australia. Differences may also exist in word familiarity due to cultural influences. For example, the British spelling for the word ‘jail’ is ‘gaol’, which may not be a familiar word form to American participants. Clinicians should therefore be conscious of these potential influences when making decisions regarding diagnoses, rehabilitation, and compensation.
Another issue in this context is the ‘Flynn effect’, which refers to the increase in average intelligence test scores as a function of time (Flynn, Citation1987). Flynn (Citation2009) found an average increase of 0.3 IQ points per year with different intelligence scales. The implication of this phenomenon for premorbid functioning tests is that the validity and reliability of these estimates may be affected by the test of current ability that they were developed to predict (see Norton, Watt, Gow, & Crowe, Citation2016; Watt, Gow, Norton, & Crowe, Citation2016). For example, due to the times at which the NART, NART‐2, and WTAR were developed with regard to their respective versions of the WAIS, they may overestimate premorbid IQ as measured by the WAIS‐IV by approximately 8.1, 6.2, and 3.3 points, respectively.
If tests of premorbid functioning do produce premorbid IQ estimates that are higher than those on the WAIS‐IV, this would result in an over‐inflated pre‐injury baseline and culminate in a discrepancy between observed and predicted premorbid IQ, even if no deficit were actually present. Thus, some people may be identified as impaired on this basis when in reality, this is not actually the case. Developing a regression equation using an updated standardisation sample that was designed to predict to the most recent version of the WAIS (i.e., the WAIS‐IV) would circumvent this issue.
This paper describes two studies. Study 1 set out to develop regression formulas for predicting WAIS‐IV FSIQ using NART/NART‐2 errors and demographics, standardised for use in Australia. It also examined the accuracy of NART, NART‐2, WTAR, and TOPF‐generated estimates of FSIQ. The ability of NART/NART‐2 errors to predict WAIS‐IV indices (Verbal Comprehension Index (VCI); Perceptual Reasoning Index (PRI); Processing Speed Index (PSI); and Working Memory Index (WMI)) was also examined. As the NART‐2 was originally developed for prediction of the WAIS‐R, this study updated the NART‐2 to predict WAIS‐IV FSIQ and the WAIS‐IV Indices. Similarly, the study also updated the WTAR to predict WAIS‐IV FSIQ, as it was originally developed to predict WAIS‐III FSIQ scores. Study 2 investigated the accuracy of estimates produced by the developed regression equations and the TOPF in a clinical sample of traumatically brain injured individuals. As this sample was impaired, it was predicted that significant discrepancies would exist between the predicted premorbid and current abilities.
STUDY 1
Method
Participants
This paper consists of two separate studies and thus used two different samples. The demographic characteristics of both samples are presented in Table .
Table 1. Demographic characteristics of the non‐impaired and clinical samples
For Study 1, in which regression equations for predicting WAIS‐IV FSIQ were developed, individuals needed to be aged 18–65-years to participate. Exclusion criteria included a previous diagnosis of a brain disorder or neurological illnesses, or a current diagnosis of a psychiatric illness. Participation was voluntary, with participants being entered in a draw to win one of 18 $50 Coles/Myer vouchers. The final sample contained 111 participants, with females aged younger than males and completing slightly fewer years of formal education.
Materials
Wechsler Adult Intelligence Scale—Fourth Edition (WAIS‐IV)
The WAIS‐IV was used to determine current IQ. The 10 core subtests were administered and scored as prescribed in the test manual (Wechsler, Citation2008). It has excellent internal consistency and test–retest reliability (α = .97–.98, r = .98–.99).
Estimates of IQ
The NART, NART‐2, WTAR, and TOPF were administered to participants to estimate premorbid IQ. The NART has excellent reliability (α = .93, r = .96–.98) and has demonstrated validity as a measure of general intelligence (Crawford, Stewart, Parker, Besson, & Cochrane, Citation1989). The WTAR has excellent test–retest reliability (r > .90) and correlates highly with the NART (r = .78). The TOPF has excellent internal consistency (r = .92–.99) and test–retest reliability (r = .89–.95; PsychCorp, Citation2009).
The tests were administered separately but in the same manner. A laminated copy of the respective word lists was given to each participant, who were then instructed to read the words out aloud with the caution that some words might be unknown to them or might be difficult to pronounce. Slight variations in pronunciation were accepted when due to regional variability, as indicated by the pronunciation guides provided for each test. Each measure of premorbid functioning was then scored as prescribed in the individual test manuals. The Word Choice Test (PsychCorp, Citation2009) was also administered.
Procedure
The majority of the participants in the non‐impaired sample were recruited through a flyer that was displayed at La Trobe University (Bundoora campus) and community centres throughout Melbourne. Some additional participants were gained through word‐of‐mouth.
Each participant was administered the tests by a research student, and the testing took 90–120-min to complete. Prior to testing, prospective participants were sent the demographic screening questionnaire by email or post to eliminate those meeting the exclusion criteria. Participants were assessed in a one‐on‐one setting, either in a University office or in participant's homes. The tests were then administered as described above.
Cases with Word Choice Test scores of ≤43 and seven or below for WAIS‐IV Reliable Digit Span were excluded, as these scores are indicative of invalid performance (Holdnack, Millis, Larrabee, & Iverson, Citation2013). The recipients of the vouchers were selected using a random number generation program, which were awarded at the end of data collection.
Results
Prior to analyses, the data was checked for normality and linearity. No assumptions were violated. A summary of scores obtained by the non‐impaired participants on the tests of premorbid and current abilities are presented in Table .
Table 2. Obtained scores of the non‐impaired sample on tests of current and premorbid IQ
To examine the relationship between WAIS‐IV FSIQ and the premorbid functioning tests, four linear regression analyses were performed using the scores of the non‐impaired sample. Significant equations were found for the NART, F(1, 109) = 70.39, standard error of estimate (SEE) = 8.96, p < .001, NART‐2, F(1, 109) = 69.38, SEE = 8.99, p < .001, WTAR, F(1, 109) = 52.44, SEE = 9.45, p < .001, and TOPF, F(1, 109) = 36.48, SEE = 9.95, p < .001. The TOPF accounted for the least percentage of variance in WAIS‐IV FSIQ scores, explaining only 25.1%, followed by the WTAR (32.5%), NART‐2 (38.9%), and NART (39.2%).
Development of the regression equations
A linear bootstrapping regression was performed using the scores of the non‐impaired sample to develop a regression equation for predicting IQ, with WAIS‐IV FSIQ used as the dependent variable and NART/NART‐2 errors as the independent variable. This statistical method resamples from the available data to build a sampling distribution for regression modelling (Efron, Citation1979). Table provides a summary of the output.
Table 3. (a) Regression equations for predicting WAIS‐IV index scores using NART/NART‐2 errors and demographics. (b) Regression analysis of WAIS‐IV FSIQ predictors for the regression equations for the non‐clinical sample
The model was significant, F(1, 109) = 69.90, SEE = 8.97, p < .001, explaining 39.1% of variance in WAIS‐IV FSIQ. The following equation was produced:
As previous studies have shown that the addition of demographic variables can increase predictive accuracy (see Crawford, Parker, et al., Citation1989; Watt & O'Carroll, Citation1999), these variables were also entered into the model. Note, sex was denoted as ‘Male = 0’ and ‘Female = 1’, and education was defined as years of successfully completed formal education. The model remained significant, F(4, 106) = 23.15, SEE = 8.51, p < .001, explaining 46.6% of the variation in WAIS‐IV FSIQ. Both NART/NART‐2 errors and education were significant predictors (p < .001 and p = .013, respectively).
The following equation was produced:
Four separate bootstrapping linear regression analyses were then performed using the non‐impaired sample to examine if NART/NART‐2 errors predicted scores for the WAIS‐IV VCI, PRI, PSI, and WMI. Table presents the developed regression equations for each index, and Table provides a summary of the regression output.
Table 4. Regression analysis of VCI, PRI, and WMI predictors for the non‐impaired sample
As seen in Table , a significant regression equation was found for VCI, with VCI as the dependent variable and NART/NART‐2 errors as the independent variable, F(1, 109) = 66.56, SEE = 8.53, p < .001. The NART/NART‐2 errors significantly predicted VCI scores, t = −8.16, p < .001, explaining 37.9% of the variance in VCI scores. Age, sex, and education were then entered into the model, which remained significant, F(4, 106) = 19.47, SEE = 8.33, p < .001. The addition of these variables increased the proportion of variance explained in VCI scores to 42.4%, with NART/NART‐2 errors (t = −7.47, p < .001) and age as significant predictors (t = −2.01, p = .024).
A significant regression equation was also found for PRI and NART/NART‐2 errors, F(1, 109) = 33.58, SEE = 10.38, p < .001. The NART/NART‐2 errors significantly predicted PRI scores, t = −5.80, p < .001, explaining 23.6% of the variance. Age, sex, and education were then entered into the model, which remained significant, F(4, 106) = 11.02, SEE = 10.11, p < .001, explaining 29.4% of the variance in PRI scores. However only NART/NART‐2 errors was a significant predictor (t = −4.78, p < .001).
A significant regression equation was found for WMI and NART/NART‐2 errors, F(1, 109) = 38.38, SEE = 11.33, p < .001. The NART/NART‐2 errors significantly predicted WMI scores, t = −6.20, p < .001, explaining 26.0% of the variance in WMI scores. Age, sex, and education were then entered into the model, which remained significant, F(4, 106) = 13.92, SEE = 10.82, p < .001, explaining 34.4% of the variance in WMI scores. The NART/NART‐2 errors (t = −4.94, p < .001) and sex (t = −2.89, p = .005) were significant predictors of WMI.
Finally, a significant regression equation was found for PSI and NART/NART‐2 errors, F(1, 109) = 5.45, SEE = 15.15, p = .021. The NART/NART‐2 errors significantly predicted PSI scores (t = −2.33, p = .021), explaining only 4.8% of the variance. Age, sex, and education were then entered into the model, which remained significant, F(4, 106) = 5.12, SEE = 14.41, p = .001, explaining 16.2% of the variance in PSI scores. Sex (t = 2.35, p = .021) and education (t = 2.25, p = .027) were significant predictors.
STUDY 2
Method
Participants
For Study 2, in which the developed equations were validated, the sample consisted of 22 clinical patients with TBI (N = 22). All of the TBI participants had closed head injuries. Duration of post‐traumatic amnesia (PTA) was calculated based on the background medical reports that were provided to the clinician conducting the assessments as well as from the patients’ self‐report and were categorised into levels of severity based upon the Diagnostic and Statistical Manual of Mental Disorders—Fifth Edition (DSM‐5; American Psychiatric Association, Citation2013) criteria. The demographics of the sample are presented in Table , and injury data for each participant is presented in Table . Unfortunately GCS and neuroradiological data were not consistently available for many of the patients in the sample, circumventing the use of these more accurate measure of injury severity. The average age of the clinical sample was older than that of the non‐impaired sample. Females also spent slightly more years in formal education than did the males.
Table 5. Injury data for the clinical sample
Materials
Study 2 used the same materials as those described in Study 1.
Procedure
Participants in the clinical sample were recruited and entered into a database from a private practice that conducted neuropsychological assessments for medicolegal, diagnostic and rehabilitation purposes. Participants were assessed by a qualified neuropsychologist, and tests were administered as described above.
Results
Comparing equation estimates versus the TOPF in a clinical sample
The WAIS‐IV FSIQ estimates produced by the regression equations developed using the non‐impaired sample with and without demographic corrections, and the TOPF with and without demographic corrections (scored using the TOPF manual; PsychCorp, Citation2009 ) were compared in a clinical sample. In clinical samples with known brain injury, premorbid FSIQ estimates should be significantly greater than actual FSIQ, due to the likelihood of the brain injury adversely affecting aspects of FSIQ. To compare the estimates, several analyses were undertaken. The data was checked for assumption violations, with none noted.
First, the clinical participants’ mean scores on the WAIS‐IV, developed regression equations, and TOPF estimates were examined (see Table ). The mean difference between WAIS‐IV FSIQ and estimates produced by the regression equations without and with demographic correction, and the TOPF without and with demographic correction were −8.21, −4.35, −3.73, and −9.86, respectively for the clinical sample.
Table 6. Descriptive statistics of index scores produced by the WAIS‐IV, regression equations, and the TOPF with and without demographic correction for the clinical sample
A repeated measures analysis of variance (ANOVA) was performed to determine if these differences were significant. The assumption of sphericity had been violated; therefore degrees of freedom were corrected using Greenhouse–Geisser estimates. Significant discrepancies existed between scores on the WAIS‐IV and premorbid functioning estimates, F(2.02, 42.31) = 12.10, p < .001, .
Post hoc Bonferroni adjusted comparisons revealed that the average actual WAIS‐IV FSIQ of the clinical group was 82.00, which was significantly less than predicted premorbid FSIQ yielded by the regression equation using NART/NART‐2 errors (M = 90.21) and TOPF with demographic correction (M = 91.86). Thus predicted premorbid FSIQ was significantly higher than current FSIQ, indicating a decline in cognitive functioning. No significant differences were evident for the other estimates (p > .05). It should be noted that FSIQ is a composite measure includes both aspects of neuropsychological functioning which are susceptible to injury as well as those which are reported not to be. Thus, in clinical populations, judicious interpretation of premorbid estimates must be made on the basis of clinical judgment.
The authors also investigated if the predictions produced by the premorbid measures differed from the general population FSIQ mean of 100 (SD = 15) using four separate one‐sample t‐tests. Estimates produced by each premorbid IQ measures significantly differed from the general population FSIQ mean (regression equation: t(21) = 29.53, p < .001; regression equation with demographic correction: t(21) = t(21) = 27.53, p < .001; TOPF: t(21) = 27.78, p < .001; TOPF with demographic correction: t(21) = 41.13, p < .001).
The final analyses examined the accuracy of WAIS‐IV index score estimates for the clinical sample produced by the premorbid functioning tests. The TOPF equative model was used to calculate WAIS‐IV index predictions (PsychCorp, Citation2009). Actual and predicted scores obtained by the clinical sample are also presented in Table .
The difference between actual and predicted WAIS‐IV index scores were compared, and four separate repeated measures ANOVAs were performed to determine if the differences were significant. In all analyses, the assumption of sphericity was violated, thus the degrees of freedom were corrected using Greenhouse–Geisser estimates.
The mean difference between WAIS‐IV indices and estimates produced by the regression equations with and without demographics, the TOPF, and the TOPF with demographic correction are displayed in Table . For VCI, the ANOVA showed that the scores differed significantly, F(2.01, 42.26) = 9.01, p = .001, , with post hoc Bonferroni corrected comparisons revealing that premorbid VCI scores yielded by the NART/NART‐2 algorithm with demographics significantly differed from the average actual WAIS‐IV VCI score (p = .04). Thus this equation produced premorbid VCI estimates that were significantly lower than current VCI scores. No significant differences were evident for the other estimates (p > .05).
For PRI, the results of the ANOVA showed that actual and estimated PRI scores significantly differed, F(1.69, 35.43) = 11.96, p < .001, . Post hoc Bonferroni corrected comparisons revealed significant differences between the PRI and estimates for the TOPF with demographic correction (p < .001). Thus this measure produced premorbid PRI scores that were significantly higher than current PRI scores, indicating a decline in non‐verbal reasoning abilities. No significant differences existed for the other scores (p > .05).
For WMI, the ANOVA showed that actual and estimated WMI scores significantly differed, F(1.79, 37.54) = 7.53 p = .002, . Post hoc Bonferroni corrected comparisons revealed that scores differed significantly between WAIS‐IV WMI and the estimates produced by the TOPF with demographic correction (p = .005). Thus this measure produced predicted premorbid WMI scores that were significantly higher than current WMI scores, indicating a decline in cognitive functioning. No significant difference was evident between WAIS‐IV WMI and the other measures (p > .05).
Finally, for PSI, the results of the ANOVA showed that actual and estimated PSI scores significantly differed, F(1.92, 40.23) = 27.14, p < .001, . Post hoc Bonferroni comparisons revealed that scores differed significantly between WAIS‐IV PSI and the estimates produced by the regression equation using NART/NART‐2 errors (p < .001), the regression equation with demographic correction (p = .002), and the TOPF with demographic correction (p < .001). Thus these measures produced premorbid PSI scores that were significantly higher than current scores, indicating a decline in cognitive functioning. No significant difference was evident between WAIS‐IV PSI and the TOPF (p > .05).
DISCUSSION
This study developed regression equations for predicting premorbid WAIS‐IV FSIQ using NART/NART‐2 errors and demographics in a neurologically healthy Australian sample. The premorbid FSIQ estimates from the NART, NART‐2, WTAR, and TOPF were compared with current WAIS‐IV FSIQ scores. The TOPF explained the least percentage of variation in WAIS‐IV FSIQ at only 25.1%, followed by the WTAR, NART‐2, and NART (32.5%, 38.9%, and 39.2%, respectively). These values are lower than those reported by Mathias, Bowden, and Barrett‐Woodbridge (Citation2007), who found that the NART explained 44% of variance in WAIS‐III FSIQ scores in an Australian sample. Studies performed elsewhere have also reported differing values, ranging from 42–49% in New Zealand (Barker‐Collo et al., Citation2008; Starkey & Halliday, Citation2011), to 66% in the UK (Crawford, Parker, et al., Citation1989).
It was surprising that the TOPF accounted for the least amount of variance in FSIQ, as it was specifically developed to predict to the WAIS‐IV, and, as a result would be expected to produce the most accurate estimates. This is concerning, as clinicians are often encouraged or required to use the most recently developed premorbid functioning test (i.e., the TOPF) in conjunction with its corresponding test of current ability (i.e., the WAIS‐IV) to avoid potential influences such as the Flynn effect (see Norton et al., Citation2016; Watt et al., Citation2016).
Two regression equations for predicting premorbid IQ as measured by the WAIS‐IV were developed in this study. The equation using only NART/NART‐2 errors to predict premorbid WAIS‐IV FSIQ barely improved predictive accuracy. However, the addition of age, sex, and education to the model significantly increased the proportion of variance explained in WAIS‐IV FSIQ scores from 39.1% to 46.6%. These improvements are similar in size to those noted by Watt and O'Carroll (Citation1999), who observed that the addition of education increased the proportion of variance explained in WAIS‐R FSIQ from 30% for NART‐2 errors to 42%. Crawford, Parker, et al. (Citation1989) also found that the addition of age, sex, education, and occupation increased the predictive accuracy of WAIS FSIQ from 66% for NART errors to 73%, an equivalent level to that found in this study. However, Schoenberg, Scott, Duff, and Adams (Citation2002) found that the Oklahoma Premorbid Intelligence Estimate‐3 (OPIE‐3; Schoenberg et al., Citation2002) algorithms developed in their study provided a greater range of FSIQ estimates and smaller standard errors than did the WTAR and the North American Adult Reading Test (NAART; Blair & Spreen, Citation1989).
This study also examined the ability of NART/NART‐2 errors to predict WAIS‐IV index scores. The errors explained the highest proportion of variance for the VCI at 37.9%, which was expected as this index relies upon verbal abilities much like the tests of premorbid IQ, followed by the WMI at 26% and the PRI at 23.6%, with the smallest proportion for PSI at 4.8%. These values are significantly lower that those reported by Crawford, Stewart, et al. (Citation1989), who found that the NART regression equation predicted 72% and 33% of the variance in WAIS VIQ and Performance IQ. A New Zealand regression formula using NART errors to estimate premorbid IQ also explained the highest proportion of variance in VIQ at 45.4%, followed by the PIQ at 22.8% (Barker‐Collo et al., Citation2011).
The accuracy of estimates produced by the developed regression equations and the TOPF with and without demographic correction was also investigated in a small clinical sample. Premorbid FSIQ estimates produced by the equation using NART/NART‐2 errors and the TOPF with demographic correction were significantly higher than current FSIQ scores, demonstrating cognitive decline. These data suggest that these algorithms yielded accurate estimates of premorbid FSIQ for the clinical sample. However, no significant differences were noted between actual and estimated WAIS‐IV FSIQ scores produced by the regression equation with demographics and the TOPF. These findings are particularly concerning, as it indicates that the TOPF may be failing to provide accurate estimates of premorbid FSIQ, despite this measure being co‐normed with the WAIS‐IV. The accuracy of the premorbid estimates was also compared to the general population FSIQ mean, with all estimates differing significantly.
Lastly, the accuracy of predicted WAIS‐IV index score estimates was examined in a clinical sample. The estimates produced by the regression equation with demographic correction significantly differed from actual VCI scores. However actual VCI scores were significantly higher than predicted premorbid VCI scores. No significant differences were noted between actual and predicted WAIS‐IV VCI scores for the other measures. These findings are consistent with the premises behind the development of premorbid functioning measures: (1) The reading of irregular words is more resistant to cognitive decline than is the reading of regular words (Franzen et al., Citation1997); and (2) reading irregular words relies upon prior (i.e., pre‐injury) knowledge and minimises demands on current cognitive ability (Willshire et al., Citation1991), and are thus less sensitive to the effects of injury. Premorbid PRI and WMI estimates produced by the TOPF with demographics differed significantly from actual PRI and WMI scores, indicating the presence of cognitive decline. No significant differences were noted between actual and estimated WAIS‐IV PRI and WMI scores for the other measures. Finally, premorbid PSI differed significantly from actual WAIS‐IV PSI scores by all measures, aside from the TOPF.
Limitations and future directions
There were several limitations of this study. While the second regression formula developed in this study included demographics as potential moderating factors, the sample on which it was developed was generally young and well‐educated. The mean age of the non‐clinical sample was 32.19-years, with the majority of participants aged 18–29-years, with only two aged over 60. The average number of years of education was also relatively high, with a mean of 15.18-years.
The size of the clinical sample was relatively small, consisting of only 22 participants. Future studies should aim to recruit participants from varying locations to obtain participants with a wider age range and years spent in formal education. A further limitation of this study was the administration procedure, in which all participants completed the tests in the same order. As the NART, WTAR, and TOPF contain some overlapping words, participants may have experienced practice effects, which may have affected performance. In future, studies should counterbalance the order of administration to circumvent this effect.
CONCLUSION
While it was found that the Australian‐derived regression formulas were able to predict a large proportion of the variance in WAIS‐IV FSIQ, the equation using NART/NART‐2 errors significantly over‐estimated premorbid IQ. What was also concerning was that the TOPF also significantly over‐estimated premorbid IQ, and accounted for the least proportion of variance in WAIS‐IV FSIQ in the non‐clinical sample. As the addition of demographic variables into the regression equation increased predictive accuracy, it is recommended that a combined approach for predicting premorbid IQ be used.
ACKNOWLEDGEMENT
The authors would like to thank Bennie Gow and Kate Norton for their assistance in the data collection.
REFERENCES
- American Psychiatric Association (2013). Diagnostic and statistical manual of mental disorders. (5th ed.). Arlington, VA: American Psychiatric Publishing.
- Baade, L., & Schoenberg, M. (2004). A proposed method to estimate premorbid intelligence utilizing group achievement measures from school records. Archives of Clinical Neuropsychology, 19, 227–243.
- Babcock, H. (1930). An experiment in the measurement of mental deterioration. Archives of Psychology, 18, 5–10.
- Barker‐collo, S., Bartle, H., Clarke, A., van Toledo, A., Vykopel, H., & Willetts, A. (2008). Accuracy of the National Adult Reading Test and Spot the Word estimates of premorbid intelligence in a non‐clinical New Zealand sample. New Zealand Journal of Psychology, 37(3), 53–61.
- Barker‐collo, S., Thomas, K., Riddick, E., & de Jager, A. (2011). A New Zealand regression formula for premorbid estimation using the National Adult Reading Test. New Zealand Journal of Psychology, 40(2), 47–55.
- Barona, A., Reynolds, C., & Chastain, R. (1984). A demographically based index of premorbid intelligence for the WAIS‐R. Journal of Consulting and Clinical Psychology, 52(5), 885–887.
- Blair, J., & Spreen, O. (1989). Predicting premorbid IQ: A revision of the National Adult Reading Test. The Clinical Neuropsychologist, 3, 129–136.
- Bright, P., Jaldow, E., & Kopelman, M. (2002). The National Adult Reading Test as a measure of premorbid intelligence: A comparison with estimates derived from demographic variables. Journal of the International Neuropsychological Society, 8(6), 847–854. https://doi.org/10.1017.S1355617702860131.
- Crawford, J., Parker, D., Stewart, L., Besson, J., & De lacy, G. (1989). Prediction of WAIS IQ with the National Adult Reading Test: Cross‐validation and extension. British Journal of Clinical Psychology, 28(3), 267–273. https://doi.org/10.1111/j.2044-8260.1989.tb01376.x
- Crawford, J., Stewart, L., Parker, D., Besson, J., & Cochrane, R. (1989). Estimation of premorbid intelligence: Combining psychometric and demographic approaches improved predictive accuracy. Personality and Individual Differences, 10(7), 793–796. https://doi.org/10.1016/0191-8869(89)90126-8
- Efron, B. (1979). Bootstrap methods: Another look at the jackknife. The Annals of Statistics, 7(1), 1–26.
- Flynn, J. (1987). Massive IQ gains in 14 nations: What IQ tests really measure. Psychological Bulletin, 101, 171–191.
- Flynn, J. (2009). The WAIS‐III and WAIS‐IV: Daubert motions favor the certainly false over the approximately true. Applied Neuropsychology, 16, 98–104. https://doi.org/10.1080/09084280902864360
- Franzen, M., Burgess, E., & Smith‐seemiller, L. (1997). Methods of estimating premorbid functioning. Archives of Clinical Neuropsychology, 12(8), 711–738. https://doi.org/10.1093/arclin/12.8.711
- Harnett, M., Godfrey, H., & Knight, R. (2004). Regression equations for predicting premorbid performance on executive test measures by persons with traumatic brain injuries. New Zealand Journal of Psychology, 33(2), 78–87.
- Holdnack, J., Millis, S., Larrabee, G., & Iverson, G. (2013). Chapter 7—Assessing Performance Validity with the ACS. In J.Holdnack, L.Drozdick, L.Weiss, & G.Iverson (Eds.), WAIS‐IV, WMS‐IV, and ACS. (pp. 331–365). San Diego, CA: Academic Press.
- Larrabee, G., Largen, J., & Levin, H. (1985). Sensitivity of age‐decline resistant (“hold”) WAIS subtests to Alzheimer's disease. Journal of Clinical and Experimental Neuropsychology, 7(5), 497–504.
- Lezak, M., Howieson, D., Bigler, E., & Tranel, D. (2012). Neuropsychological assessment. (5th ed.). New York: Oxford University Press.
- Mathias, J., Bowden, S., & Barrett‐woodbridge, M. (2007). Accuracy of the Wechsler Test of Adult Reading (WTAR) and National Adult Reading Test (NART) when estimating IQ in a healthy Australian Sample. Australian Psychologist, 42(1), 49–56. https://doi.org/10.1080/00050060600827599
- Mathias, J., Bowden, S., Bigler, E., & Rosenfeld, J. (2007). Is performance on the Wechsler Test of Adult Reading affected by traumatic brain injury? British Journal of Clinical Psychology, 46(4), 457–466. https://doi.org/10.1348/014466507X190197
- Mcfie, J. (1975). Assessment of organic intellectual impairment. New York: Academic Press.
- Nebes, R., Martin, D., & Horn, L. (1984). Sparing of semantic memory in Alzheimer's disease. Journal of Abnormal Psychology, 93(3), 321–330. https://doi.org/10.1037/0021-843X.93.3.321
- Nelson, H. (1982). The National Adult Reading Test (NART): Test manual. Windsor, UK: NFER‐Nelson.
- Nelson, H., & Willison, J. (1991). National Adult Reading Test (NART). (2nd ed.). Berkshire, Great Britain: NFER‐Nelson.
- Norton, K., Watt, S., Gow, B., & Crowe, S. F. (2016). Are tests of premorbid functioning subject to the Flynn effect? Australian Psychologist, 51(5), 374–379. https://doi.org/10.1111/ap.12235
- Patterson, K., Graham, N., & Hodges, J. (1994). Reading in dementia of the Alzheimer's type: A preserved ability? Neuropsychology, 8(3), 395–407.
- Powell, B., Brossart, D., & Reynolds, C. (2003). Evaluation of the accuracy of two regression‐based methods for estimating premorbid IQ. Archives of Clinical Neuropsychology, 18, 277–292.
- PsychCorp (2001). Wechsler Test of Adult Reading manual. San Antoniou, TX: The Psychological Corporation.
- PsychCorp (2009). Advanced Clinical Solutions for WAIS‐IV and WMS‐IV Administration and Scoring manual. San Antoniou, TX: The Psychological Corporation.
- Reynolds, C., & Gutkin, T. (1979). Predicting the premorbid intellectual status of children using demographic data. Clinical Neuropsychologist, 1(2), 36–38.
- Schoenberg, M., Lange, R., Brickell, T., & Saklofske, D. (2007). Estimating premorbid general cognitive functioning for children and adolescents using the American Wechsler Intelligence Scale for Children—Fourth Edition: Demographic and current performance approaches. Journal of Child Neurology, 22(4), 379–388.
- Schoenberg, M., Scott, J., Duff, K., & Adams, R. (2002). Estimation of WAIS‐III intelligence from combined performance and demographic variables: Development of the OPIE‐3. The Clinical Neuropsychologist, 16(4), 426–438.
- Schoenberg, M., Scott, J., Ruwe, W., Patton, D., & Adams, R. (2004). Assumptions that underlie predicting premorbid IQ: A comment on the “Evaluation of the accuracy of two regression‐based methods for estimating premorbid IQ”. Archives of Clinical Neuropsychology, 19, 1103–1106.
- Starkey, N., & Halliday, T. (2011). Development of the New Zealand Adult Reading Test (NZART): Preliminary findings. New Zealand Journal of Psychology, 40(3), 129–141.
- Vanderploeg, R., & Schinka, J. (1995). Predicting WAIS‐R IQ premorbid ability: Combining subtest performance and demographic variable predictors. Archives of Clinical Neuropsychology, 10(3), 225–239.
- Vanderploeg, R., Schinka, J., Baum, K., Tremont, G., & Mittenberg, W. (1998). WISC‐III premorbid prediction strategies: Demographic and best performance approaches. Psychological Assessment, 10(3), 277–284.
- Watt, K., & O'carroll, R. (1999). Evaluating methods for estimating premorbid intellectual ability in closed head injury. Journal of Neurology, Neurosurgery and Psychiatry, 66(4), 474–479. https://doi.org/10.1136/jnnp.66.4.474
- Watt, S., Gow, B., Norton, K., & Crowe, S. F. (2016). Investigating discrepancies between predicted and observed Wechsler Adult Intelligence Scale—Version IV Full Scale Intelligence Quotient scores in a non‐clinical sample. Australian Psychologist, 51(5), 380–388. https://doi.org/10.1111/ap.12239
- Wechsler, D. (1958). The measurement and appraisal of adult intelligence. (4th ed.). Baltimore: Williams & Wilkins.
- Wechsler, D. (2001). Wechsler Test of Adult Reading. San Antonio, TX: The Psychological Corporation.
- Wechsler, D. (2008). Wechsler Adult Intelligence Scale. (4th ed.). San Antonio, TX: The Psychological Corporation.
- Willshire, D., Kinsella, G., & Prior, M. (1991). Estimating WAIS‐R IQ from the National Adult Reading Test: A cross‐validation. Journal of Clinical and Experimental Neuropsychology, 13, 204–216. https://doi.org/10.1080/01688639108401038