Abstract
Global climate change is one of the most important issues of contemporary environmental safety. A scientific consensus is forming that the emissions of greenhouse gases, including carbon dioxide, nitrous oxide and methane, from anthropogenic activities may play a key role in elevating the global temperatures. Quantifying soil greenhouse gas emissions is an essential task for understanding the atmospheric impacts of anthropogenic activities in terrestrial ecosystems. In most soils, production or consumption of the three major greenhouse gases is regulated by interactions among soil redox potential, carbon source and electron acceptors. Two classical formulas, the Nernst equation and the Michaelis–Menten equation, describe the microorganism-mediated redox reactions from aspects of thermodynamics and reaction kinetics, respectively. The two equations are functions of a series of environmental factors (e.g. temperature, moisture, pH, Eh) that are regulated by a few ecological drivers, such as climate, soil properties, vegetation and anthropogenic activity. Given the complexity of greenhouse gas production in soils, process-based models are required to interpret, integrate and predict the intricate relationships among the gas emissions, the environmental factors and the ecological drivers. This paper reviews the scientific basis underlying the modeling of greenhouse gas emissions from terrestrial soils. A case study is reported to demonstrate how a biogeochemical model can be used to predict the impacts of alternative management practices on greenhouse gas emissions from rice paddies.
INTRODUCTION
Global climate change is becoming a hot issue in contemporary science as well as politics. There is a long-lasting debate about the cause of the climate change: anthropogenic activity versus the natural cycle (e.g. CitationCrowley 2000; CitationCuffey 2004; CitationDamon and Kunen 1976). However, driven by the rapidly accumulating observations worldwide, a scientific consensus is forming that the contemporary climate change (e.g. temperature increase) is mainly caused by anthropogenic emissions of the greenhouse gases, including carbon dioxide (CO2), nitrous oxide (N2O) and methane (CH4) (CitationCrutzen et al. 1979; CitationIntergovernmental Panel on Climate Change 2001). Soil plays an important role as a source or sink of greenhouse gases in almost all terrestrial ecosystems. Quantifying the impacts of mitigating strategies on soil greenhouse gas (GHG) emissions is a core task for identifying the best management practices in agriculture or forestry. This paper provides a review of the scientific basis and modeling approach for quantifying soil GHG emissions from terrestrial soils.
GREENHOUSE GAS PRODUCTION DRIVEN BY MICROBIAL ACTIVITY
Soil microorganisms play a key role in the production and consumption of CO2, N2O and CH4 in most terrestrial ecosystems. The microbes survive and gain energy by breaking the carbon (C) bonds of dissolved organic compounds. To accomplish this process, electrons must be transferred from the dissolved organic carbon (DOC) to electron acceptors. Among the oxidants commonly existing in soils, oxygen (O2) possesses the lowest Gibbs free energy and, hence, is the first candidate electron receiver. During the process of electron transfer, the ionized oxygen will be combined with the dissociated C to form CO2 in the microbial cells. Under aerobic conditions, most soil microbes can use oxygen as an electron acceptor and release CO2 into the atmosphere. This process leads to decomposition, a reaction that dominates the losses of soil organic carbon (SOC). Soil aeration status sensitively depends on soil physical conditions. For example, during a rainfall or irrigation event, the top layers of the soil can be saturated by water that will block the diffusion of atmospheric O2 into the soil profile. While the soil microbes continuously consume the O2 left in the soil pores, the soil O2 partial pressure will drop rapidly. The depletion of O2 will depress a wide range of decomposers, but will stimulate a group of special microbes, denitrifiers in the soil. The denitrifiers are capable of using nitrate (NO− 3), an oxidative form of nitrogen, as an electron acceptor. By receiving electrons, NO− 3 will become nitrite (NO− 2). Nitrite can be further reduced to nitric oxide (NO), nitrous oxide (N2O) and finally dinitrogen (N2). During the sequential reactions of denitrification, if the intermediate product N2O can escape from the anaerobic micro-sites before it has been further reduced, a net emission of N2O will occur. If a soil has been placed under anaerobic conditions for a long time (e.g. several days), the major oxidants, such as O2, nitrate, manganese (Mn4+), iron (Fe3+) and sulfate, will be depleted by the decomposers, denitrifiers, manganese bacteria, iron bacteria and sulfur bacteria, respectively. In this case, methanogens will be activated to use hydrogen as an electron acceptor that will result in CH4 production (). The above-described mechanisms have been observed by many researchers in their field and laboratory studies worldwide (e.g. CitationCappenberg 1974; CitationDe Groot et al. 1994; CitationGambrell and Patrick 1978; CitationJakobsen et al. 1981; CitationLoveley and Phillips 1987; CitationTakai and Kamura 1966).
In general, CO2, N2O or CH4 is a byproduct of the microbial survival, which is characterized by a transfer of electrons. This type of reaction is categorized as a reduction–oxidation (or redox) reaction. In soils, the major factors controlling the redox processes are redox potential (i.e. Eh in volts), DOC concentration, and the relevant electron acceptor (e.g. O2, nitrate, Mn4+, Fe3+, sulfate or hydrogen) concentration. Soil Eh determines if a redox reaction can occur; and if it occurs, the contents of DOC and the electron acceptor will collectively determine the rate of the biogenic reaction. Any mitigation measure, if it can alter one or more of the three factors (i.e. Eh, DOC or electron acceptor), will change the emissions of CO2, N2O or CH4. Thus, it is essential for quantifying GHG emissions to understand how the impacts of Eh, DOC and the electron acceptor on greenhouse gases can be quantitatively determined.
Two classical formulas, the Nernst equation and the Michaelis–Menten equation, can be used to describe the interactions between the three driving factors and the microbial activities. The Nernst equation is a basic thermodynamic formula defining soil Eh based on concentrations of the dominant oxidants and reductants existing in the soil liquid phase (CitationStumm and Morgan 1981):
where Eh is redox potential (V), Eo is standard redox potential (V), R is gas constant, T is temperature in kelvin, n is number of electrons transferred in the redox reaction, F is Faraday constant, [O] is the concentration of the oxidant (mol L−1) and [W] is the concentration of the reductant (mol L−1).
The Michaelis–Menten equation is a widely applied formula describing the kinetics of microbial growth with dual nutrients (CitationPaul and Clark 1989):
Figure 1 In soils, CO2, N2O and CH4 are produced or consumed by microbial activities, which are regulated by the soil redox potential (Eh) and the availability of the energy source (i.e. dissolved organic carbon) and the electron acceptors (e.g. oxygen, nitrate, Mn4+, Fe3+, sulfate, hydrogen).
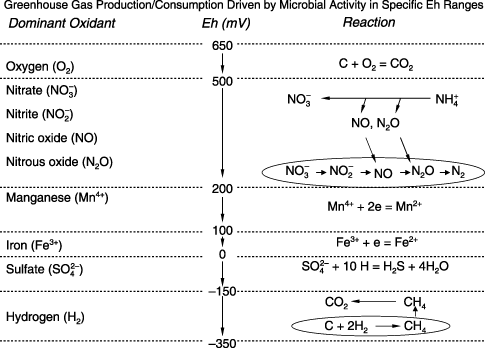
where R is reaction rate, Rmax is maximum reaction rate, DOC is concentrations of dissolved organic C, [O] is concentrations of oxidant, and Ka and Kb are half-saturation constants for substrates DOC and oxidant, respectively.
As the Nernst and Michaelis–Menten equations share a common item, oxidant concentration ([O]), the two equations can be integrated to track the feedback between Eh dynamics and the microbe-mediated redox reaction in a soil.
CONSTRUCTION OF VIRTUAL ECOSYSTEMS BASED ON BIOGEOCHEMICAL CONCEPTS
Redox potential, DOC and the electron acceptor are the major, but not the only, factors determining GHG emissions from soils. For example, temperature, moisture and pH can also affect the biochemical or geochemical reactions related to soil CO2, N2O or CH4 emissions. In general, there are seven environmental factors, namely gravity, radiation, temperature, moisture, pH, Eh and substrate concentration gradient, that control GHG production/consumption in soils. Most of the environmental factors are variable in space and time and are driven by a few ecological drivers, including climate, soil properties, vegetation and anthropogenic activity. The ecological drivers, the environmental factors and the reactions compose a complex system, which governs transport and transformation of chemical elements, including C and nitrogen (N), in ecosystems. Concepts of biogeochemistry have been adopted to cope with the complex systems.
Biogeochemistry is a scientific discipline studying the relationship between life and its environment through defining, understanding and tracking the movement of chemical elements (CitationVernadsky 1944). Biogeochemistry consists of four concepts, abundance, field, coupling and cycling, which regulate the interactions between life and its environment (CitationLi 2001). Biogeochemical abundance explores the correlation between the elemental compositions of life and its inorganic environment (e.g. atmosphere, crust, soil, sea water). During the last several decades, various chemical and physical analytical methods have been used to identify the abundances of essential or trace elements in various life forms and the environment. These measurements have provided fundamental datasets for understanding the essential correlation between life and its environment through comparison of the biogeochemical abundances. Biogeochemical field is the assemblage of environmental forces or factors, including gravity, radiation, temperature, moisture, pH, Eh or redox potential, and substrate concentration gradients. These factors construct a multi-dimensional, biogeochemical field in which the transport and transformation of chemical elements occur. The biogeochemical field plays a key role in integrating various factors and processes into an entirety, such as an ecosystem. A biogeochemical field is produced by primary drivers (e.g. climate, soil, topography, vegetation and anthropogenic activity) in a specific ecosystem, and it determines all of the relevant biochemical or geochemical processes and, hence, the ecosystem evolution. Mathematically expressing the biogeochemical field is a key step to predicting the transport and transformation of chemical elements in ecosystems. Biogeochemical modeling reconstructs the dynamics of the biogeochemical fields as they continually vary in space and time. Biogeochemical coupling describes how chemical elements must act in specific couples or groups during their movement in the environment. In most terrestrial ecosystems, the processes of coupling/decoupling of chemical elements are realized through a series of biochemical or geochemical reactions, including mechanical movement, dissolution/crystallization, decomposition/combination, oxidation/reduction, adsorption/desorption, complexation/decomplexation and assimilation/dissimilation. As do all chemical reactions, each of these processes has two directions to lead to elemental coupling or decoupling in various forms. The direction and rate of each reaction is usually controlled by more than one environmental factor. Elemental coupling and decoupling through the biochemical or geochemical reactions are driven by both internal factors (e.g. atomic structure, bond energies, electronegativies) and external factors (e.g. gravity, radiation, temperature, moisture, pH, Eh, substrate concentration gradients) forming the biogeochemical field. A myriad of coupling/decoupling phenomena shape the complexity of an element's biogeochemical cycle. Theoretical analyses of thermodynamics, chemical reaction kinetics, bond energy/enthalpy and quantum chemistry have been used to predict the coupling/decoupling phenomena occurring within the biotic bodies (e.g. antagonistic and synergistic effects of the elements) or the environment (e.g. CitationHedin et al. 1998; CitationLi et al. 2000). Biogeochemical cycling represents the ultimate movement of chemical elements through transport in space and transformation in time at the interface between life and its environment, in response to the biogeochemical field and within the constraints of biogeochemical abundance and coupling. Through elemental cycling, life interacts with and shapes its environment by means of exchanges of energy, matter and information. These four concepts, biogeochemical abundance, field, coupling and cycling, are interwoven to define the scientific scope of biogeochemistry and, hence, determine its methodologies. Based on these concepts, the dynamics
Figure 2 Biogeochemical field consists of seven environmental factors and bridges between the ecological drivers (e.g. climate, soil, vegetation and anthropogenic activity) and the biochemical or geochemical reactions that determine elemental coupling, decoupling and cycling in ecosystems.
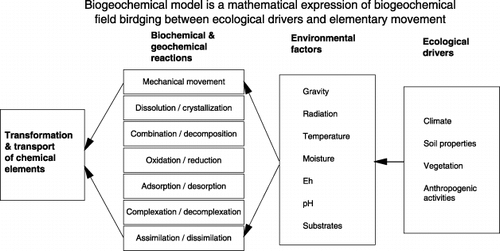
As shown in , a virtual ecosystem consists of two components as two bridges linking the ecological drivers and the environmental factors and the environmental factors and the biochemical or geochemical reactions, respectively. Scientific laws in physics, chemistry and biology as well as equations empirically generated from experiments can be incorporated in the framework to express the quantitative relationships among the linked factors or processes. Within the virtual ecosystem, any change in a single factor could cause variation in multiple factors or processes in the system. For example, a change in irrigation could simultaneously alter the soil temperature, moisture, Eh and substrate concentrations; the altered environmental factors could collectively affect dissolution/crystallization, oxidation/reduction, adsorption/desorption and other reactions that will finally change the production and consumption of CO2, N2O or CH4 in the soil. Converting the virtual ecosystem into a computable program will build a biogeochemical model to serve GHG or other ecological or environmental studies.
A BIOGEOCHEMICAL MODEL FOR SIMULATING GREENHOUSE GAS EMISSIONS
During the past 15 years with support from US federal agencies (e.g. Environmental Protection Agency (EPA), National Science Foundation (NSF), National Aeronautics and Space Administration (NASA), National Oceanic
Figure 3 A computable kinetic scheme “anaerobic balloon” was invented to link the Nernst equation to the Michaelis–Menten equation for tracking the interaction between the soil redox conditions and the microbial activities.
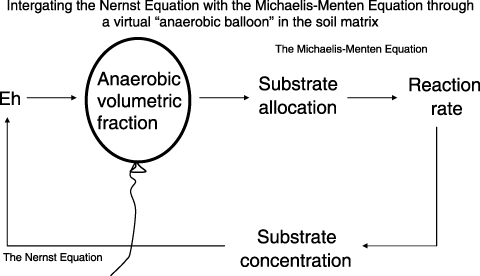
By linking the balloon scheme with other algorithms defining soil climate, crop growth and farming management practices (e.g. tillage, fertilization, manure amendment, irrigation, grazing), DNDC estimates GHG emissions across climatic zones, soil types and management regimes. During the past decade, DNDC has been independently tested by a number of researchers worldwide with promising results (CitationBrown et al. 2002; CitationButterbach-Bahl et al. 2001; CitationCai et al. 2003; CitationGrant et al. 2004; CitationJagadeesh Babu et al. 2006; CitationKesik et al. 2005; CitationKiese et al. 2005; CitationPathak et al. 2005; CitationSaggar et al. 2003; CitationSmith et al. 1997, Citation2004; CitationXu-Ri et al. 2003; CitationZhang et al. 2006). The results indicate that computer models equipped with biogeochemical processes could form a reliable tool for quantifying GHG emissions.
BRINGING UNCERTAINTY UNDER CONTROL FOR UPSCALING
One of the major purposes for developing process-based models is to extend our understanding gained at specific sites to regional, national or global scales. In fact, policy decisions can only be made based on their effectiveness at regional or national scales. When a process-based model is applied to a regional scale, an issue of uncertainty will arise even when the model may have been well calibrated and validated at a site scale. The uncertainty mainly results from spatial heterogeneity of the input parameters used for the upscaling. For applying this type of model to a region, the region is routinely divided into many grid cells or polygons, with an assumption that each grid cell is uniform in all its properties. This assumption is against the fact that some of the input parameters, especially soil properties, usually vary even within small scales, such as a county or a farm. Averaging the variations of the input data may not resolve the problem because the correlation between the modeled output (e.g. GHG flux or other concerned items) and the drivers is non-linear. There are a number of measures to cope with this challenge. A relatively simple approach, the Most Sensitive Factor (MSF) method, was recently developed with DNDC applications (CitationLi et al. 1996, Citation2004). Through sensitivity tests, the most sensitive factors for a concerned output (e.g. CO2, N2O or CH4) can be identified. For example, initial SOC content has been identified as the most sensitive factor for soil N2O emissions in many case studies (CitationLi et al. 2002). During the input database construction, the most sensitive factor (e.g. SOC content) was assigned two values for each grid cell, the maximum and minimum values observed within the cell. During the model simulation, DNDC runs twice for each grid cell with the two extreme values (e.g. the maximum and minimum SOC contents) in the cell. The two runs will produce two N2O fluxes to form a range, which is assumed to be wide enough to include the “real” N2O flux with a high probability. This methodology has been verified against the Monte Carlo method, a classical tool for uncertainty tests. The results indicated that 60–90% of the Monte Carlo method induced CO2, N2O or CH4 fluxes were located within the ranges produced by the MSF method (CitationLi et al. 2004) (). By constructing the regional database with the maximum and minimum values for
soil properties or other sensitive factors, the uncertainties induced by the spatial heterogeneity of input parameters can be brought under control.A CASE STUDY ON MITIGATING GHG EMISSIONS FROM PADDY SOILS IN CHINA
It has long been known that paddy soil is an important emitter of methane. In fact, GHG emissions from paddy soils have been intensively studied to date. Water management has been investigated as one of the options for mitigation of GHG emissions (CitationKanno et al. 1997; CitationNugroho et al. 1994; CitationWassmann et al. 1993; CitationYagi et al. 1994, Citation1996, Citation1997). A study was recently carried out to upscale the impacts of change in water management on crop yield, soil C sequestration and GHG emissions for the entire rice fields of China (CitationLi et al. 2006). An evolution of water management for rice agriculture is under way in China. Continuous flooding was the dominant practice before the 1980s, which constantly kept the rice fields flooded with a water layer of approximately 5–10 cm during the rice growing season. From 1980 to 2000, the traditional practice was gradually replaced by midseason drainage, in which the rice fields were drained 2–5 times during a rice growing season. Each of the draining periods lasted for 5–10 days. The midseason drainage increased the rice yield by enhancing plant root development, depressing over-tillering and increasing mineralization of soil organic nitrogen. After 2000, a new practice, shallow flooding, emerged in rice cultivation in China. In this practice, the rice fields are marginally flooded with the soil surface barely covered by a thin water layer, even sometimes having the water table drop below the soil surface for days. This management practice saves water and improves soil aeration (CitationChen 2004). This decades-long evolution in water management raised an interesting question: how would the changes in water management affect GHG emissions from rice production in China?
The DNDC was used to answer this question. Thirty million hectares of rice paddies in China were simulated with several scenarios of alternatives, varying in management practices of water, fertilizer and rice straw. The DNDC was run with 21-year alternative management schemes for each of the ∼2,500 counties in the country. The modeled results indicate that: (1) replacing continuous flooding with midseason drainage in 1980–2000 has substantially reduced CH4 emissions from rice production in China by approximately 4 Tg CH4-C per year, (2) despite large scale adoption of midseason drainage currently, there is still a large potential for additional CH4 reductions from Chinese rice paddies of 20–60% from 2000 to 2020 by applying shallow flooding (), (3) changes in management for reducing CH4 emissions simultaneously affect soil carbon dynamics as well as N2O emissions and can thereby reorder the ranking of technical mitigation effectiveness, (4) most
Figure 5 Impacts of management alternatives on nationally averaged CH4 emissions from rice paddy fields in China from 2000 to 2020. The continuous flooding scenario produced the highest CH4 emissions. Applying midseason drainage reduced more than half of the emissions resulting from continuous flooding. Shallow flooding further reduced CH4 emissions by 200–300 kg CH4-C per ha. From 2000 to 2020, CH4 emissions slightly increased because of the soil C accumulation.
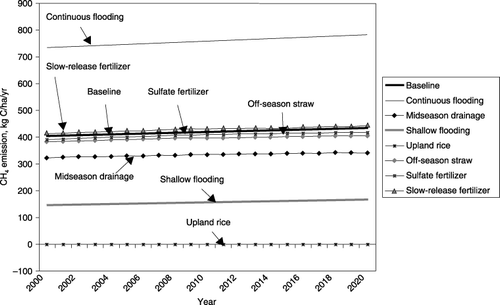
In summary, quantifying soil GHG emissions is a scientific challenge that requires capacity to extend the understanding at a microbial or soil chemical scale to a regional or global scale. Fortunately, the latest developments in biogeochemistry, spatial data acquisition and computing techniques have provided potentials to integrate the research effort across the scales in space and time. The business of biogeochemical modeling is still in the early stages, although few researchers now doubt its irreplaceable role in contemporary ecosystem studies. I hope this brief review has touched on the basic issues of biogeochemical modeling and will draw more scientists to the new development.
ACKNOWLEDGMENTS
I thank Dr Kazuyuki Yagi for his invitation and financial support that allowed me to deliver this presentation at the International Workshop on Monsoon Asia Agricultural Greenhouse Gas Emissions, 7–9 March 2006, Tsukuba, Japan. The research itself was supported by US National Science Foundation (US NSF), Environmental Protection Agency (EPA) and National Aeronautics and Space Administration (NASA) Terrestrial Ecology (NAG5-12838 and NAG5-7631), Earth Observation System (EOS)-Interdisciplinary Science (IDS) (NAG5-10135) and other relevant programs.
REFERENCES
- Crowley , TJ . 2000 . Causes of climate change over the past 1000 years . Science , 289 : 270 – 277 .
- Cuffey , KM . 2004 . Into an Ice Age . Nature , 431 : 133 – 134 .
- Damon , PE and Kunen , SM . 1976 . Global Cooling? . Science , 193 : 447 – 453 .
- Crutzen , PJ , Heidt , LE , Krasnec , JP , Pollock , WH and Seiler , W . 1979 . Biomass burning as a source of the atmospheric gases CO, H2, N2O, CH3Cl, and Cos . Nature , 282 : 253 – 256 .
- Intergovernmental Panel on Climate Change . 2001 . Climate Change 2001A Scientific BasisIntergovernmental Panel on Climate Change (IPCC) , Edited by: Houghton , JT , Ding , Y Griggs , DJ . Cambridge : Cambridge University Press .
- Cappenberg , TE . 1974 . Interrelations between sulfate-reducing and methane-producing bacteria in bottom deposits of a fresh water lake: I. Field observations . Antonie van Leeuwenhoek , 40 : 285 – 295 .
- De Groot , CJ , Vermoesen , A and van Cleemput , O . 1994 . Laboratory study of the emission of N2O and CH4from a calcareous soil . Soil Sci , 158 : 355 – 364 .
- Gambrell , RP and Patrick , WH . 1978 . “ Chemical and microbiological properties of anaerobic soils and sediments ” . In Plant Life in Anaerobic Environment , Edited by: Hook , DD and Crawford , RMM . 375 – 423 . Ann Arbor : Ann Arbor Science Publication .
- Jakobsen , P , Patrick , WH Jr and Williams , BG . 1981 . Sulfide and methane formation in soils and sediments . Soil Science , 132 : 279 – 287 .
- Loveley , DR and Phillips , EJP . 1987 . Competitive mechanism for inhibition of sulfate reduction and methane production in the zone of ferric iron reduction in sediments . ApplEnvironMicrobiol , 53 : 2636 – 2641 .
- Takai , Y and Kamura , T . 1966 . The mechanism of reduction in waterlogged paddy soil . Folia Microbiol , 11 : 303 – 313 .
- Stumm , W and Morgan , JJ . 1981 . “ Oxidation and Reduction ” . In Aquatic Chemistry: An Introduction Emphasizing Chemical Equilibria in Natural Waters , 2nd edn , Edited by: Stumm , W and Morgan , JJ . 418 – 503 . New York : John Wiley & Sons .
- Paul , EA , Clark , FE , Paul , EA and Clark , FE . 1989 . “ Dynamics of residue decomposition and soil organic matter turnover ” . In Soil Microbiology and Biochemistry , 2nd edn , 157 – 166 . San Diego : Academic Press .
- Vernadsky , VI . 1944 . Problems of Biogeochemistry, the Fundamental Matter-Energy Difference Between the Living and the Inert Natural Bodies of the Biosphere , Edited by: Vernadsky , George and Hutchinson] , G.E. New Haven : Connecticut Academy of Arts and Sciences .
- Li , C . 2001 . Biogeochemical concepts and methodologies: Development of the DNDC model . Quaternary Sci. , 21 : 89 – 99 . (in Chinese)
- Hedin , LO , von Fischer , JC , Ostrom , NE , Kennedy , BP , Brown , MG and Robertson , GP . 1998 . Thermodynamic constraints on nitrogen transformations and other biogeochemical processes at soil-stream interfaces . Ecology , 79 : 684 – 703 .
- Li , C , Aber , J , Stange , F , Butterbach-Bahl , K and Papen , H . 2000 . A process-oriented model of N2O and NO emissions from forest soils: 1, Model development . JGeophysRes , 105 : 4369 – 4384 .
- Li , C , Frolking , S and Frolking , T . 1992 . A model of nitrous oxide evolution from soil driven by rainfall events: 1. Model structure and sensitivity . J. Geophys. Res. , 97 : 9759 – 9776 .
- Li , C , Frolking , S and Harriss , R . 1994 . Modeling carbon biogeochemistry in agricultural soils . Global Biogeochem. Cycles , 8 : 237 – 254 .
- Li , C , Narayanan , V and Harriss , R . 1996 . Model estimates of nitrous oxide emissions from agricultural lands in the United States . Global Biogeochem. Cycles , 10 : 297 – 306 .
- Brown , L , Syed , B Jarvis , SC . 2002 . Development and application of a mechanistic model to estimate emission of nitrous oxide from UK agriculture . Atmos. Environ. , 36 : 917 – 928 .
- Butterbach-Bahl , K , Stange , F , Papen , H and Li , C . 2001 . Regional inventory of nitric oxide and nitrous oxide emissions for forest soils of southeast Germany using the biogeochemical model PnET-N-DNDC . J. Geophys. Res. , 106 ( D24 ) : 34155 – 34166 .
- Cai , Z , Sawamoto , T Li , C . 2003 . Field validation of the DNDC model for greenhouse gas emission in East Asian cropping systems . Global Biogeochem. Cycles , 17
- Grant , B , Smith , WN , Desjardins , R , Lemkc , R and Li , C . 2004 . Estimated N2O and CO2emissions as influenced by agricultural practices in Canada . Climate Change , 65 : 315 – 332 .
- Jagadeesh Babu , Y , Li , C , Frolking , S , Nayak , DR and Adhya , TK . 2006 . Field validation of DNDC model for methane and nitrous oxide emissions from rice-based production systems of India . Nutr. Cycling Agroecosyst. , 74 : 157 – 174 .
- Kesik , M , Ambus , P Baritz , P . 2005 . Inventories of N2O and NO emissions from European forest soils . Biogeosciences , 2 : 353 – 375 .
- Kiese , R , Li , C , Hilbert , D , Papen , H and Butterbach-Bahl , K . 2005 . Regional application of PnET-N-DNDC for estimating the N2O source strength of tropical rainforests in the Wet Tropics of Australia . Global Change Biology , 11 : 128 – 144 .
- Pathak , H , Li , C and Wassmann , R . 2005 . Greenhouse gas emissions from Indian rice fields: calibration and upscaling using the DNDC model . Biogeosciences , 2 : 113 – 123 .
- Smith , P , Smith , JU Powlson , DS . 1997 . A comparison of the performance of nine soil organic matter models using datasets from seven long-term experiments . Geoderma , 81 : 153 – 225 .
- Saggar , S , Andrew , RM , Tate , KR , Hedley , CB and Townsend , JA . Simulation of nitrous oxide emissions from New Zealand dairy-grazed pastures and its mitigation strategies . Proc. of the 3rd International Methane and Nitrous Oxide Mitigation Conference . November 17–21 2003 , Beijing, China. pp. 461 – 468 .
- Xu-Ri , X , Wang , M and Wang , Y . 2003 . Using a modified DNDC model to estimate N2O fluxes from semi-arid grassland in China . Soil Biol. Biochem. , 35 : 615 – 620 .
- Zhang , F , Li , C , Wang , Z and Wu , H . 2006 . Modeling impacts of management alternatives on soil carbon storage of farmland in Northwest China . Biogeosci. , 3 : 451 – 466 .
- Li , C , Mosier , A Wassmann , R . 2004 . Modeling greenhouse gas emissions from rice-based production systems: sensitivity and upscaling . Global Biogeochem. Cycles , 18 : GB1043
- Kanno , T , Miura , Y , Tsuruta , H and Minami , K . 1997 . Methane emission from rice paddy fields in all of Japanese prefecture . Nutr. Cycling Agroecosyst. , 49 : 147 – 151 .
- Nugroho , S , Lumbanraja , J , Suprapto , H and Ardjasa , WS . 1994 . Effect of intermittent irrigation on methane emission from an Indonesian paddy field . Soil SciPlant Nutr , 40 : 609 – 615 .
- Yagi , K , Chairoj , P , Tsruta , H , Cholitkul , W and Minami , K . 1994 . Methane emission from rice paddy fields in the central plain of Thailand . Soil SciPlant Nutr , 40 : 29 – 37 .
- Yagi , K , Tsuruta , H , Kanda , K and Minami , K . 1996 . Effect of water management on methane emission from a Japanese rice paddy field: Automated methane monitoring . Global Biogeochem. Cycles , 10 : 255 – 268 .
- Yagi , K , Tsuruta , H and Minami , K . 1997 . Possible options for mitigating methane emission from rice cultivation . Nutr. Cycling Agroecosyst. , 49 : 213 – 220 .
- Li , C , Salas , W , DeAngelo , B and Rose , S . 2006 . Assessing alternatives for mitigating net greenhouse gas emissions and increasing yields from rice production in China over the next 20 years . J. Environ. Qual. , 35 : 1554 – 1565 .
- Chen , WF . 2004 . Techniques for Rice Production in North China , Beijing : Chinese Agricultural Press . (in Chinese)
- Li , C , Our , J Frolking , S . 2002 . Reduced methane emissions from large scale changes in water management in China's rice paddies during 1980–2000 . Geophys. Res. Letters , 29 : 20
- Smith , WN , Grant , RL , Desjardins , R , Lemke , R and Li , C . 2004 . Estimates of the interannual variations of N2O emissions from agricultural soils in Canada . Nutr. Cycling Agroecosyst. , 68 : 37 – 45 .
- Wassmann , R , Papen , H and Rennenberg , H . 1993 . Methane emission from rice paddies and possible mitigation strategies . Chemosphere , 26 : 201 – 217 .