Abstract
This study quantified and evaluated the greenhouse gas emissions of farmland soils at a watershed scale using parameters available at a regional scale. The estimation was based on field monitoring data in the Ikushunbetsu River Watershed, Hokkaido, Japan. Simple regression models were created for carbon dioxide, nitrous oxide and methane emissions associated with six major agricultural land uses, and forest as an alternative land use to farmland. An eco-balance method involves conducting an analysis on the basis of farmland surplus nitrogen (N) and global warming potential (GWP). Uncertainties associating the estimations were estimated using Mote Carlo simulation. The eco-balance is the analysis of the relationship between production and environmental load. In this study, production and environmental load were compared by changing each of the land use combinations by 10%. Farmland surplus N was lowest for soybean with 8.2 kg N ha−1 year−1, followed by paddy rice. The highest value was recorded for vegetables with 99.8 kg N ha−1 year−1. The weighted mean of total farmland based on the land use proportion was 44 ± 33.8 kg N ha−1 year−1. The calculated GWP was 7.3 Mg CO2 eq ha−1 year−1 for paddy rice and 0.1 to 6.8 Mg CO2 eq ha−1 year−1 for upland crops. The weighted mean of the total farmland area was 4.0 ± 3.4 Mg CO2 eq ha−1 year−1. The eco-balance analysis showed that there were 59 combinations out of 8008 combinations able to reduce GWP more than 6%, but less than 7%, than the value in 2005. Among them, in 30 combinations, farmland surplus N became less than the value in 2005; production was reduced compared with the value in 2005 in 27 combinations. Soybean occupied 20–80% in the seven combinations where production was increased compared with 2005, while keeping farmland surplus N below the value in 2005. The estimation of greenhouse gases in this study exhibited high uncertainty because of variability in management and errors in evaluation procedures. Quantification of the data variability is set at maximum, which comprises the measured values in this area. Based on the quantification of the uncertainty, more efficient quantification methods can be established to clarify mitigation potential. This type of quantification and comparison between production and emission enables decision makers to set some threshold values that allow a compromise between production and environmental load.
INTRODUCTION
How to quantify greenhouse gas (GHG) emissions at a regional scale is an important question concerning the mitigation of GHG emissions from agriculture. Nutrient flows at a regional scale are the sum of the management practices of all land uses in the area. In upscaling plot data to a regional scale, attention should be paid to the magnitude of the parameters influencing the target phenomenon at the desired target scale. The relative influence of an environmental parameter changes with scale (CitationLark et al. 2004). Increasing the modeling scale would be straightforward if important processes did not change with scale, if spatial variations of model parameters and inputs were easily described or insignificant, and if model parameters scaled linearly (CitationSeyfried 2003). In fact, processes do change with scale, spatial variations may dictate model results, and model parameters are often nonlinear with respect to scale (CitationKirkby et al. 1996, CitationLark et al. 2004). Process-based models require sophisticated meteorological data and site-specific information (e.g. soil type, topographic features) that are usually not available at a satisfactory spatial resolution (Citationvan Es et al. 1998). Thus, no matter how well a model represents the process of interest at a field scale, the resultant simulation will have reduced accuracy if the required parameters are of reduced accuracy. Thus, a model that is mechanistic at small scales becomes empirical at certain larger scales (CitationSeyfried 2003). A quantitative, mechanistic understanding at any scale is dependent on the extent of understanding at the next lower level (CitationWagenet 1998). By this reasoning, the potential for producing mechanistic understanding and models declines with increasing scale.
As a result of this scaling difficulty, quantification at a regional scale is often conducted with transfer functions (CitationBouma and van Lanen 1987). Two types of transfer functions have been distinguished: continuous functions and class functions. Continuous functions are based on regressions and allow direct point estimates, while class functions relate physical properties to pedogenic soil-horizon designations or soil series.
Continuous functions often used are regression models and emission factors (EFs). For example, an emission factor of nitrous oxide (N2O) emission is the proportion of this gas relative to the net amount of input (CitationIntergovernmental Panel on Climate Change 1997). This method offers the advantage of simplicity in deriving emission values for different crop types with different amounts of fertilizer. However, it is difficult to include the effects of soil and climatic variables required for an upscaling operation. Thus, the CitationIntergovernmental Panel on Climate Change (2006) suggests three steps, Tier 1, 2 and 3, in the upscaling approach. Tier 1 suggests a default emission factor for N2O (e.g. 1.25% for the net N input) for countries with insufficient inventory data. Tier 2 is recommended for countries with sufficient measurements, where country-specific EFs are used. Tier 3 is an estimation of greenhouse gases using process-based models with a spatial extension, combined with a geographical information system and remote sensing. To date, Tier 3 has only been partly prepared for restricted areas using the DAYCENT model in the USA covering 80% of arable land (CitationDel Grosso et al. 2005) or the DNDC model for regions with intensive soil survey data (CitationLi et al. 1996). Although such studies used process-based models, results revealed that the input data of climate and soil properties were roughly divided into 63 minor agricultural regions for the whole of the USA in CitationDel Grosso et al. (2005) for example.
Another approach uses lumped transfer functions that subsume lower-scale process-level complexity. This alternate approach views these transfer functions as appropriate “minimum essential element” variables at the scale in question (CitationWagenet 1998). CitationFreibauer (2003), for example, created regression models to estimate greenhouse gases from the farmlands of European Union countries. In that study, EFs were created as a function of the nitrogen input associated with mineral and organic fertilizer, the organic carbon content in topsoil soil C, and the sand content in topsoil. Compared with existing methods for large-scale inventories, these regression models allowed a better regional fit to measured values because they integrated additional driving forces of N2O emissions, such as climate, substrate availability represented by soil carbon and nitrogen, and oxygen. These simple models are promising for an analysis of the mitigation potential for regional or national stakeholders.
In considering mitigation of one environmental impact, care must be taken that no other impact is increased. There are several attempts to create standardized indicators. The CitationOrganisation for Economic Co-Operation and Development (2001) suggested agri-environmental indicators setting agricultural production in a broad context by considering economic forces (e.g. farm production, employment), societal preferences (e.g. rural viability), environmental processes (e.g. interaction of agriculture with biophysical conditions) and land use changes (e.g. agricultural land use). An assessment of all these indicators is difficult, and there have been efforts to simplify multiple indicators. For example, CitationEckert et al. (2000) normalized parameters such as soil fertility, physical soil protection, pesticide use, landscape and species diversity, and energy balances into values ranging from 1 to 12, where 1 was defined as the optimum and 6 the critical threshold value. All indicators are then summarized with one index, called KUL. In industry, the widely used Eco-indicator 99 defined the “environment” in terms of damage to human health, ecosystem quality and resources (CitationGoedkoop et al. 2000). The distance-to-target approach used in Eco-indicator 95 has been changed to a damage function approach in Eco-indicator 99. One Eco-indicator point is equivalent to one-thousandth of the yearly environmental load of one average European inhabitant, taking into account the lifetime of a given substrate (total environmental load in Europe divided by the number of the inhabitants and multiplied by 1000). For nutrient flow in agroecosystems, a more comprehensive indexing is often used, such as nutrient use efficiency (CitationMoll et al. 1982) or the flow index (CitationFinn 1980).
However, uniting different categories into one index often reduces information, especially trade-off relations among the individual indicators. Weighting of indicators is subjective, and different aggregation methods can produce different results (CitationGeier 2000). Results presented orally are the most transparent, but are time consuming (CitationGeier 2000). An optical illustration of results can be given using a radar graph (CitationHaas et al. 2001), although the upper limit of the graph must be set subjectively. There is also criticism concerning the application of life-cycle approaches in an assessment of agricultural production systems on the environment in terms of effect potentials because they disregard the spatial dimension and setting (CitationHaag and Kaupenjohann 2001). These obstacles conflict with the need for a holistic analysis of a production system. An evaluation method is required that can provide decision criteria and insights into how environmental impacts and production can be balanced. The analysis of the relation between production and environmental load has been defined as “eco-balance” (CitationKimura and Hatano 2006). The eco-balance approach allows an analysis of the trade-off relationship between production and environmental impact for various impacts. In CitationKimura and Hatano's (2006) study, historical changes in nitrogen flows of a municipality from 1912 to 2002 were analyzed to evaluate the relationship between production and environmental pollution. The eco-balance analysis explored the direction of change and provided detailed analysis of the change in subsystems in an effort to analyze the driving forces behind changes in production and environmental loads.
The objective of the present study was to quantify GHG emissions from farmlands at a regional scale and give an example of how monitoring data can be scaled up using parameters available at a regional scale. A model was created by parameterization of monitoring data in this region. Uncertainty associated in the evaluation was quantified. Mitigation scenarios of GHG emissions from farmlands were analyzed using an eco-balance analysis (CitationKimura 2005). The indicators used in this study were global warming potential (GWP), production and farmland surplus nitrogen (N).
MATERIALS AND METHODS
Study site
The study was conducted at the Ikushunbetsu River watershed in central Hokkaido (N43°14′ E141°57′, ). The annual mean temperature is 7.4°C, the mean precipitation is 1,154 mm and the deepest snow depth is 1.2 m (CitationSapporo Distinct Meteorological Observatory 2006). The population in 2005 of Mikasa City, which covers almost the whole watershed, was 11,924 (CitationMikasa City 2005). Among them, 710 people (148 households) participate in agricultural activities (CitationMikasa City 2005).
According to a ground survey conducted in July 2005, the total farmland area was 1,473 ha.Global warming potential
Upland fields
The GWP was estimated through carbon dioxide (CO2) equivalence: 1 g of methane (CH4) equates to 23 g of CO2, and 1 g of nitrous oxide (N2O) is equal to 296 g of CO2 (CitationIntergovernmental Panel on Climate Change 2001).
Carbon dioxide flux was measured as the difference between carbon (C) input and output in the field. Carbon input is the sum of net primary production (NPP) and C in manure, and output is represented by the sum of soil organic C decomposition, CH4 emission and C in harvested products. The amount of applied manure, NPP and C in harvested products were obtained from inquiries with farmers. Estimations of manure C were based on these inquiries, and a C:N ratio of 10 was assumed. Soil organic C decomposition of upland fields was assumed to be equal to the CO2 fluxes at bare soils and was estimated using an equation obtained from field measurements in this region in 2003 (CitationMu et al. 2005). Only values before the soil freezing period were used. The variability of the regression line was expressed by the standard deviation of the residual (SDr):
where yi is the measured value, Yi the estimated value and n the number of data. This equation was used for the following equations.
where the average 5 cm soil temperature (°C) is the measured temperature while measuring the CO2 flux at each site, and TN and TC are the soil total N and total C concentration at 0–20 cm depth, respectively. The soil temperature at 5 cm of the watershed was estimated using air temperature from the nearest meteorological station (CitationSapporo Distinct Meteorological Observatory 2006). Based on measurements at the monitoring sites in this region (CitationMu et al. 2005), except during the soil-freezing period, the soil temperature was estimated as follows:
Nitrous oxide flux of an upland field was estimated on the basis of the following assumption (CitationToma et al. 2007):
where EFF is the emission factor for chemical fertilizer, EFO the emission factor for applied organic matter including crop residuals and N2O from soil organic matter decomposition is the background N2O emission from soil without any N fertilization. There was a positive correlation between EFF and mean annual air temperature (P < 0.01), and between EFO and the mean annual relative humidity (CitationToma et al. 2007). Based on 30-year-average meteorological data from the CitationSapporo Distinct Meteorological Observatory (2006), the average and standard deviation (SD) values for EFF and EFO were 1.85 ± 1.57% and 1.96 ± 3.82%, respectively. Background N2O emission from soil was estimated based on the relationship between soil mineral N pool and N2O emission (CitationMu et al. 2005). The soil mineral N pool was estimated based on the CO2 from soil organic matter decomposition, which was assumed to have a C:N ratio of 12:
The cumulative CH4 flux of an upland field was not considered in this study because they ranged from −0.8 kg C ha−1 to 0 kg C ha−1 and their contribution was only 0 to –0.28% of the total GWP (CitationMu et al. 2006).
A soil map from the Basic Survey on Soil Fertility (CitationHokkaido Central Agricultural Experiment Station 1971) was used to calculate the soil organic matter decomposition rate for each soil series.
A land use map was created from a field survey in 2005. The mean soil organic matter decomposition rate for each land use type was calculated according to the proportion of soil series in each land use.
Paddy rice fields
The CH4 flux for a paddy rice field showed a high correlation with the applied amount of straw (CitationNaser 2006) and was represented by the following equation:
A similar relationship was also found for CO2 emission and N2O emission from paddy field soil:
In this study, the amount of rice straw was obtained by inquiries with farmers.
Farmland surplus nitrogen
Farmland surplus N was defined as the difference between the N input to the farmland and the sum of the output and losses from the farmland. Nitrogen input is the sum of chemical N fertilizer, N in manure, N through deposition and irrigation and biological N2 fixation. The output from the farmland is the N in harvested productions: losses are denitrification, ammonia (NH3) volatilization and N2O emission from farmland. The amount of fertilizer N, manure N and yield was calculated following inquiries with farmers conducted from 2002 to 2004 (). The amount of residue was calculated according to the proportion of harvested product to the byproduct (CitationMatsumoto 2000). The proportions were 1.428, 1.388, 1.396, 0.478, 0.598 and 0.444 for paddy rice, wheat, soybean, onion, vegetable and grassland, respectively. Ammonia volatilization was assumed to be 36% of the ammonia in the manure, which was 10% for cattle and pig (CitationBrentrup et al. 2000), and 8% of the total N for chemical fertilizer (CitationBouwman et al. 1997). Only cattle and pig manure were used in this region.
Biological N2 fixation was set to 30 kg N ha−1 year−1 for paddy rice (CitationKyaw et al. 2005) and 5 kg N ha−1 year−1
Table 1 Investigated land use management numbers
Activity data
The amount of chemical fertilizer, amendment of organic matter, yield and rice straw residuals were obtained through inquiries with farmers conducted from 2002 to 2004 for the years 2002 and 2003 (). The total number of inquiries in 2002/2003 was 22 and in 2004 the number was 57. In addition, 45 records from the extension office (private communication) were used for the year 2002. The total farm numbers were 188 in 2000 and 148 in 2005 (CitationMikasa City 2005). Among them 113 farms had paddy fields and 153 farms had uplands in 2000, while the numbers were 102 and 113 in 2005, respectively. Thus, 16–18% of the paddy rice farmers of the region and 28–38% of the upland farmers were investigated in this study.
Land uses were mapped by a ground survey conducted in July 2005. In 2005, the total farmland area was 1,473 ha (). The major land use was rice (424 ha), wheat (254 ha), soybean (43 ha), onion (337 ha), vegetable (161 ha) and grassland (253 ha). According to the results of the basic survey on soil fertility (CitationHokkaido Central Agricultural Experiment Station 1971), there were 14 soil series in the watershed. The soils with total carbon (TC) and total nitrogen content (TN) reported in this report were used to estimate soil organic matter decomposition (EquationEqn 2) and associated background N2O (EquationEqn 5). The TN and TC values exceeded the measured values of 16.0_28.7 g C kg−1 and 1.4_2.5 g N kg−1, respectively, in seven soil series. This accounts for 19% of upland
Table 2 Area and proportion of the major land use types of farmland in the Ikushunbetsu river basin in 2005
Eco-balance analysis
Land use combinations were analyzed for major farmland land uses (rice, wheat, soybean, onion, vegetables and grassland). It was assumed that all land uses could be cultivated regardless of soil type and geographical conditions. Forest was added to the farmland land uses as an alternative to cultivation. The GWP of forest was calculated using a net primary production (NPP) of 3,065 kg C ha−1 year−1 (CitationTakahashi et al. 1999) and CH4 absorption of –4.8 kg C ha−1 year−1 (CitationMorishita et al. 2004). Net ecosystem production (NEP) was calculated using the relationship between NEP and NPP (NEP = 0.705 × NPP – 425 (kg C ha−1 year−1)) as described by CitationHatano (2002). Thus, the GWP of forest became –6.5 Mg CO2 eq ha−1 year−1, while surplus N and production was 0 kg N ha−1 year−1. As land use combinations can exist in infinite combinations, combinations were analyzed by changing the proportion of each land use from 0 to 100% in 10% intervals. By changing the proportion of the seven land uses by 10% there were 8008 combinations. Farmland surplus N and N in production were calculated for all possible land use combinations.
Uncertainty analysis
The uncertainty of the calculated N flow components was evaluated using Monte Carlo simulation. In Monte Carlo simulation, variables of a given output ym are calculated as a function of variables of the model inputs xk (k = 1, . . . , m). The distribution of probabilities of each model input data xk was specified. For the activity data and inventory data with sufficient numbers (n > 5), the distribution of probabilities was created by fitting the data to the distribution of probabilities using Levenberg–Marquardt optimization (CitationPalisade Corporation 2002). When the normal distribution was not rejected by an Anderson–Darling or Kolmogorov–Smirnov fitting test at α = 0.05, the distribution of probabilities was assumed to follow the normal distribution. When the normal distribution was rejected, another distribution form (e.g. exponential, lognormal, gamma, peasonV) was chosen. When no distribution of probabilities form fitted the sample data, or if the available data number was below five, a β-subjective distribution was chosen. A β-distribution can be generated only by mean, median, maximum and minimum values. In the case of only one available datum value, median and mean were made equivalent, while the maximum and minimum were assumed to be 1.5 and 0.5-fold the mean, respectively. The probability densities for output variables were calculated from input variables using a frequency distribution derived from 10,000 runs of a Latin Hypercube Simulation (@Risk 4.5, Palisade, NY, USA). The results are exhibited as SD.
RESULTS
Farmland nitrogen balance
The N balances of the six major land uses are shown in . The N input was above 200 kg N ha−1 year−1 for
Table 3 Calculated nitrogen balance per unit area (kg N ha−1) of farmland for major land uses
Uncertainty was expressed as SD. The SD of the sum of input, and sum of output and loss exceeded those of each flow components. In cases where one flow component showed an extremely high value, the SD of the sum equaled that extreme value. This was the case for the sum of the input for wheat, onion and vegetable. The highest SD was the amount of input for vegetables with 183.6 kg N ha−1 year−1, which also had the highest mean value. The SD of farmland surplus N ranged from 32.6 kg N ha−1 year−1 for paddy rice to 85.2 kg N ha−1 year−1 for vegetables. Those values were similar to the SD of the sum of input, and sum of output and loss except for vegetables. The SD of the weighted mean showed small values compared to the individual land uses for the sum of input, sum of output and loss as well as farmland surplus, which were 27.7 kg N ha−1 year−1, 33.2 kg N ha−1 year−1 and 33.8 kg N ha−1 year−1, respectively.
Greenhouse gas emissions and global warming potential
According to data based on our inquiries with farmers, the remaining rice straw in the fields was 421 ± 638 kg C ha−1 year−1 (average ± SD). The greenhouse gas fluxes were divided into emissions dependent on soil type and management, such that soil organic matter decomposition in upland soil and N2O emission from non-fertilizer bare soil were classified as soil-type-dependent emissions, while all emissions from paddy rice fields and N2O emission derived from chemical fertilizer, manure and residue were classified as management-dependent emission. Negative values indicate absorption, while positive values indicate emission. Except for paddy rice fields, management-dependent CO2 fluxes were negative, while soil-type-dependent CO2 fluxes were positive (). The sum of both was negative with –387 kg C ha−1 year−1 for wheat because of its high amount of residue and –212 kg C ha−1 year−1 for grassland because of the C input from the manure. The other land uses showed positive values, ranging from 272 to 1230 kg C ha−1 year−1. Methane emission from paddy rice was estimated to be 188 kg C ha−1 year−1. Nitrous oxide showed high dependence on management. The lowest management dependent N2O flux value was found for paddy rice with 1.1 kg N ha−1 year−1, the highest for onion with 5.3 kg N ha−1 year−1. Soil-type-dependent N2O ranged from 0.1 to 0.2 kg N ha−1 year−1.
The uncertainty of CO2, CH4 and N2O showed similar trends to the summarizing of the flows for the nitrogen balance in . The SD of the sum of CO2 and N2O reflected the SD of the component with the higher
Table 4 Greenhouse gas emissions from major land uses
Figure 2 Global warming potential (GWP) of the major six farmland land uses and the weighted mean of the watershed. Numbers in the figures are the sum of GWP for each land use. Values in parentheses are the standard error.
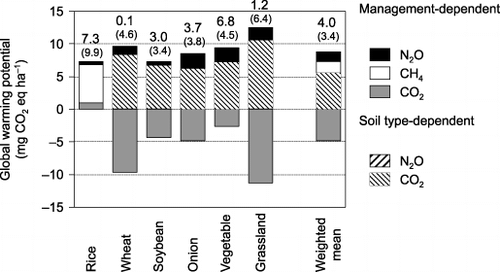
shows the contribution of CO2, CH4 and N2O to GWP of the total farmland area expressed through CO2 equivalents (CitationIntergovernmental Panel on Climate Change 2001). Methane represented 79% of the GWP in the case of paddy rice, while CO2 ranged from 81 to 95% of the absolute amount of GWP (sum of all absolute GWP fluxes) for upland land uses. Soil-type-dependant emissions were equal or higher than the amount of management dependent absorption (amount of residue and manure). Nitrous oxide contributed 6–19% of the absolute amount of GWP. As a result, rice and vegetables had the highest total amount of GWP with values of 7.3 and 6.8 Mg CO2 eq ha−1 year−1, respectively.
The average for total farmland in the Ikushunbetsu watershed was 4.0 Mg CO2 eq ha−1 year−1. In the absolute, GWP CO2 contributed to 76.3%, while methane contributed to 12.1% and N2O 11.6%, for the calculated total GWP, nitrous oxide, CH4 and CO2 emissions represented 40%, 41% and 19%, respectively. Management-dependent fluxes contributed to 58.8% of the weighted mean.
Eco-balance analysis
The possible values of GWP and farmland surplus N values under the present management methods by changing the land use proportion, ranged from –6.5 to 7.3 CO2 eq ha−1 year−1 and from 0 to 87.4 kg N ha−1 year−1, respectively (). Global warming potential and farmland surplus N can only be found within the zone shown in gray () using the present management methods.
Figure 3 Range in global warming potential (GWP) and farmland surplus N as a proportion of land use changes at 10% intervals. The black circle represents the land use proportion at 2003 and the white circles represent the 100% proportion of a single land use type. Error bars indicate standard deviation.
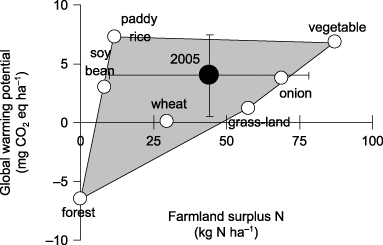
DISCUSSION
Uncertainties
Uncertainties associated with these estimations are of concern. Uncertainty can be caused by: (1) individual farmer-dependent managements and/or microsite-dependent variability, (2) estimation errors by using different estimation methods and standard deviations accompanying parameterization procedures. In the first case they are not uncertainties but existing ranges; however in the second case they are failures that need to be reduced. In this study, the two origins of uncertainties were not distinguished, hence, the evaluation resulted in a high uncertainty for the sum of flows as well as for farmland surplus N for individual land uses (,). Assumed variables for values derived from the literature, such as NH3 volatilization or the residual ratio, were set to 1.5 and 0.5-fold the mean, which might be too high. However, this value of the assumed variables is necessary to prevent over confidence (CitationEllis et al. 2000).
The uncertainties were expressed as SD, not coefficient of variance (CV), because subtracting calculations leads to extremely high CVs if the calculated values became low. For example, the SD of the farmland surplus N of soybeans was 51.9, while the CV became 634%. Thus, ideally both relative and absolute uncertainties need to be considered to avoid misunderstandings (Citationde Vries et al. 2003). In the following discussion, we also use the CV to compare our results with previous studies.
The SD of the weighted mean was lower than that recorded for individual land uses. Extreme values cancel each other out, which reduces the range of a weighted mean. This was also found in the study by Citationde Vries et al. (2003), who estimated the uncertainties associated in the N budget of The Netherlands. The calculated uncertainty for the N flows of the whole Netherlands (expressed as CV) ranged from 15 to 47%, while the uncertainty of individual flow ranged up to 108% (Citationde Vries et al. 2003). The range of CV in the present study is from 11 to 131%, while the individual flow ranged from 2 to 204%, except for surplus N, which can exhibit very high CV values because of its small absolute values.
The approach used by CitationFreibauer (2003) was a considerable improvement over the CitationIntergovernmental Panel on Climate Change (1997) method. The regression models used by CitationFreibauer (2003) had a mean standard error of 30–100%, while the estimated uncertainty of CitationIntergovernmental Panel on Climate Change (1997) ranged from 70 to 80% (CitationLim et al. 1999) for arable soils. The larger uncertainty in the study of CitationFreibauer (2003) resulted from the widely scattered measured data underlying the regression model, and this was also true in the present study. The estimation of N2O was based on EquationEq. 4, where N2O emission came from three individual sources, applied chemical fertilizer, organic matter and organic matter including crop residuals, and soil organic matter decomposition. The first two sources were estimated by EF, which was only management dependant, while the latter was estimated by CO2 fluxes at bare soils. This estimation method contains high variability, thus, the CV of this study of the weighted mean of GWP was 86%.
The estimation of greenhouse gases in this study exhibited high uncertainty because of variability in management and errors in evaluation procedures. The quantification of the data variability is set at maximum, which comprises the measured values in this area. Based on this uncertainty quantification, more efficient quantification methods can be established to clarify mitigation potential.
Estimation of greenhouse gas emissions for a regional scale
Simple models reflecting site-specific differences are needed to quantify greenhouse gases and analyze the mitigation potential for a region. Lumped parameters seem to subsume too much process-level information, but are actually useful for the integration of temporal and spatial variability (CitationGroffman and Tiedje 1989). Field-based information from plot research needs to be generalized to the region level and the results of annual field data need to be evaluated for time periods that are adequate for climate-based inferences (typically > 30 years). A critical step is to develop models commensurate with the upscaling objectives in terms of the required precision, spatial scale and data availability (Citationvan Es et al. 1998).
In the present study, several attempts were made to meet this goal. The methods should be more sophisticated, but they provide a good demonstration of how to handle field data in the context of a regional scale. The uncertainty of this kind of evaluation is still high, which was discussed in the previous section. Greenhouse gas emissions from different fields were considered in terms of annual accumulation, rather than daily or hourly fluxes. This approach rounds up the temporal variability of gases, especially N2O but also CO2 and CH4 fluxes, which are known to have daily fluctuations as a result of temperature and moisture changes.
Nitrous oxide is known to exhibit great spatial and temporal variability (CitationFlessa et al. 1995, CitationSmith and Dobbie 2001), which makes it difficult to predict. CitationGroffman and Tiedje (1989) found that it is easier to estimate the cumulative amount rather than daily fluxes. In the present study, generalization for spatial differences was conducted using a regression analysis (EquationEq. 5). A similar spatial generalization should be used for the EFs for chemical fertilizer and organic fertilizer. Those values are only generalized for a temporal scale (average of 30 years) and attempts must be made to extend them spatially. Spatial variability was generalized for background emission, but its contribution (0.1–0.2 kg N ha−1 year−1) was considerably lower than the default background emission from the CitationIntergovernmental Panel on Climate Change (1997) of 1 kg N ha−1 year−1. The background emission in an onion field within the same watershed ranged from 2.01–12.1 kg N ha−1 year−1 (CitationToma et al. 2007). In that field, N2O emission could be estimated in the same way, using EquationEqn 4; however, with different transfer functions. More studies are required for generalization and inclusion of hot spots of N2O background emissions. Several studies indicate that N2O emissions are highly related to the soil nitrogen pool (e.g. CitationAulakh et al. 2000) and underlie the applicability of this parameter, although further validation is required before making any generalizations.
The paddy rice field exhibited the simplest relationship in terms of GHG emission. The functional unit was reduced to the applied amount of straw (EquationEqs 6,Equation7,Equation8). As the research area lies in central Hokkaido, in which there is usually no midterm drainage of fields, CH4 emission is mostly sensitive to the amount of applied straw (CitationNaser et al. 2007). The amount of readily mineralizable carbon is one of the main factors affecting CH4 emission from flooded soils (CitationYagi and Minami 1990). Unlike the study of CitationYagi and Minami (1990), different soil types showed the same dependence to the amount of applied straw, which might be because of the lower temperature and the absence of midterm drainage practices in Hokkaido in comparison with other regions in Japan (CitationNaser et al. 2007).
Calculation of global warming potential
The calculated GWP was 7.3 Mg CO2 eq ha−1 year−1 for paddy rice and 0.1–6.8 Mg CO2 eq ha−1 year−1 for upland crops (). The average of the whole area was 4.0 Mg CO2 eq ha−1 year−1. This is in the order of previous studies: 0.7–25 Mg CO2 eq ha−1 year−1 for upland crops in Europe and an average value of 2 Mg CO2 eq ha−1 year−1 for European Union countries (CitationFreibauer 2003). Although soil respiration is rarely considered for GHG emissions from farmland soil, except where there was a land use change, there is evidence that continuous cultivation reduces the carbon content even in mineral soils, and at quite high values in the case of organic soils (CitationBellamy et al. 2005). Soil respiration must be taken into account for estimations to provide real mitigation of GHG emission. Cultivation practices, such as non-tillage should also be taken into account, and the way the residue is incorporated must be evaluated (CitationKoga et al. 2006). The fate of manure is also important. Methane emissions from paddy rice fields contributed 26% of the total GWP of the farmland area in this study. The average emission rate of 188 kg C ha−1 y−1 in this study was higher than the average of continuously flooded rice fields without organic amendment, which was 125 kg C ha−1 y−1 in Japan (CitationYan et al. 2005). A reduction in the application of rice straw has a high potential for lowering the GWP of farmland in regions with paddy rice fields.
Validation of the results is difficult or impossible at large scales. One way of checking the result is to test the basic assumption behind the extrapolation (CitationWagenet 1998). For example, CitationGroffman et al. (1992) measured denitrification enzyme activity as a proxy for the annual denitrification N flux at a large number of sites across a region to determine if the relationship between denitrification and the land characteristics of soil texture and drainage was robust at the regional scale. Key assumptions of the regression model used in the present study must be further checked within the watershed.
Eco-balance analysis
The eco-balance analysis based on land use changes provided a rough illustration of the range of environmental impacts in this region (). In fact, soil types influence the applicability of land use types. In the study area, land uses such as paddy rice fields are mainly distribute in lower areas with a soil type consisting of Fluvisols (CitationKimura 2005). A more detailed analysis for specific land use types may improve the results, by considering specific thresholds of relevant spatial characteristics, as conducted by CitationKlöcking et al. (2003) with respect to hydrological impact studies. In the present study, the weighted mean of the soil type for each land use was used for the Eco-Balance. A more complex analysis of different management methods in different soil types can show the specific optimization potential of the watershed.
The analysis showed that GWP could be reduced by more than 6% in 6209 out of all 8008 combinations (). The amount of production even increased for certain land use combinations. The main potential for reduction was by reducing paddy rice fields and increasing forest areas. Carbon dioxide uptake was observed as forest areas increased, which was accompanied by a decline in production. This is quite natural, but the illustration provided in this study allows the establishment of a certain target value. The quantification of production and emission enables decision makers to set some threshold values, allowing a compromise between production and environmental load. In the case of GHG emissions, if production is maintained at the present level, emissions inevitably occur, although our analysis showed that production could still be maintained by reducing GWP by 6%. The target value of 6% was set according to the Kyoto Protocol, but this value is tentative and any target value can be set to consider ideal land-use combinations. A similar analysis can be conducted for different management scenarios. In such cases, the proportion of different management methods can be gradually changed rather than changing the land use type.
The basic purpose of this analysis was to compare environmental loads. The indicators were not united into one single index. In studies involving agricultural life-cycle assessment, the results can be expressed in different units, such as a farm, area, livestock or product (CitationHaas et al. 2000). This unit is termed the functional unit. However, the results of the analysis depend on the functional unit. For example, the study by CitationHaas et al. (2000) showed that the organic agricultural system had the smallest CO2 emission in comparison to intensive and extensive systems when the farm, area and livestock were used as the functional unit. When product was chosen as the functional unit, the extensive system had the lowest emission in comparison to intensive and organic systems. These researchers concluded that a product-related functional unit might be appropriate for environmental impacts that contribute on a global scale, while production efficiency combined with minimizing negative environmental impacts might be relevant for a national point of view.
In the present study, the target of the analysis was the optimization of the total farmland area of the watershed. Thus, the unit production per area was used to compare the amount in production and the environmental load. This should also be chosen for other indicators if the target area is the total watershed. Deciding which land use to choose must be based on setting a threshold value that determines the desired reduction in GHG emissions, the acceptable farmland surplus N and the tolerable reduction in yield. The target for environmental load must be decided on the basis of ecological conditions of the area, and the reduction in yield must be analyzed by considering the economic income of the farmers. Further studies are required to set these targets.
Creation of a single index makes it easier to compare complex systems. Some examples are given by the Eco-Indicator 99 (CitationGoedkoop et al. 2000) and KUL (CitationEckert et al. 2000) methods. The Eco-Indicator 99 method provides options that allow users to create their own weighting using a mixing triangle with human health, ecosystem quality and energy resources at each edge of the triangle. Human health may be given a weight of 50%, ecosystem quality 40% and energy resources 10%. However, the normalization of the individual indicator units is still subjective, and the unification to a single index reduces information. In the present study, weighing is not applied and the results are illustrated visually. This analysis is a relative analysis of the present situation, and the combination of land uses allows decision makers to understand the weak points of the present management. Global warming potential can be reduced by increasing the proportion of forest, wheat, grass, soybean and onion, while farmland surplus N can be reduced by increasing forest, soybean, paddy rice and wheat. To reduce both environmental impacts, an increase in the proportion of forest, soybean and wheat represents a promising strategy. These land uses may be favorable in set-aside programs. In contrast, such an approach clearly shows the need for management changes in land uses like paddy fields and vegetables.
Conclusions
This study showed how GHG emissions can be quantified at a regional scale. To quantify GHG emissions at a regional scale, the process must be represented by parameters available at large scales. Parameterization of monitoring data seems to provide an important link between point measurement and detailed process-based models. The quantification enabled a mitigation analysis using an eco-balance method. Many mitigation strategies were found; however, GHG emission occurs at a certain degree if production is maintained. Future interpretation and parameterization are needed to develop the estimation model and to apply it to other regions. The results of the quantification must be validated and the uncertainty needs to be reduced. The integration of monitoring, regional activity data and GIS maps will be a key in future analyses.
ACKNOWLEDGMENTS
This work was financially supported by the Global Environmental Research Program of the Ministry of the Environment of Japan (No. S-2). This paper was presented at the International Workshop on Monsoon Asia Agricultural Greenhouse Gas Emissions (MAGE-WS), 7–9 March 2006, Tsukuba, Japan.
REFERENCES
- Aulakh , MS , Khera , TS and Doran , JW . 2000 . Mineralization and denitrification in upland, nearly saturated and flooded subtropical soil II. Effect of organic manures varying in N content and C:N ratio . Biol. Fertil. Soils , 31 : 168 – 174 .
- Bellamy , PH , Loveland , PJ , Bradley , RI , Lark , RM and Kirk , GJD . 2005 . Carbon losses from all soils across England and Wales 1978–2003 . Nature , 437 : 245 – 248 .
- Bouma , J and van Lanen , HAJ . 1987 . “ Transfer functions and threshold values: from soil characteristics to land qualities. Workshop on Quantified Land Evaluation Process ” . In International Institute for Aerosphere Survey and Earth Science , Edited by: Beek , KJ . Vol 6 , 106 – 110 . Enschede, , The Netherlands : ITC Publ. .
- Bouwman , AF , Lee , DS , Asman , WAH , Dentener , FJ , van der Hoek , KW and Olivier , JGJ . 1997 . A global high-resolution emission inventory for ammonia . Global Biogeochem. Cycl , 11 : 561 – 587 .
- Brentrup , F , Küstres , J , Lammel , J and Kuhlmann , H . 2000 . Methods to estimate on-field nitrogen emissions from crop production as an input to LCA studies in the agricultural sector . Int. J. LCA , 5 : 349 – 357 .
- De Vries , W , Kros , J , Oenema , O and de Klein , J . 2003 . Uncertainties in the fate of nitrogen II: A quantitative assessment of the uncertainties in major nitrogen fluxes in the Netherlands . Nutr. Cycl. Agroecosyst , 66 : 71 – 102 .
- Del Grosso , SJ , Mosier , AR , Parton , WJ and Ojima , DS . 2005 . DAYCENT model analysis of past and contemporary soil N2O and net greenhouse gas flux for major crops in the USA . Soil Tillage Res , 83 : 9 – 24 .
- Eckert , H , Breitschuh , G and Sauerbeck , DR . 2000 . Criteria and standards for sustainable agriculture (KUL) . J. Plant Nutr. Soil Sci , 163 : 337 – 351 .
- Ellis , EC , Li , RG , Yang , LZ and Cheng , X . 2000 . Long-term change in village-scale ecosystems in China using landscape and statistical methods . Ecol. Appl , 10 : 1057 – 1073 .
- van Es , HM , DeGaetano , AT and Wilks , DS . 1998 . Space–time upscaling of plot-based research information: frost tillage . Nutr. Cycl. Agroecosys , 50 : 85 – 90 .
- Finn , JT . 1980 . Flow analysis of models of the Hubbard Brook ecosystem . Ecol , 61 : 562 – 571 .
- Flessa , H , Dörsch , P and Beese , F . 1995 . Seasonal variation of N2O and CH4fluxes in differently managed arable soils in southern Germany . J. Geophys. Res , 100 : 23115 – 23124 .
- Freibauer , A . 2003 . Regionalised inventory of biogenic greenhouse gas emissions from European agriculture . Europ. J. Agron , 19 : 135 – 160 .
- Geier , U . 2000 . Anwendung der Ökobilanz-Methode in der landwirtschaft-dargestellt am Beispiel einer Prozeß-Ökobilanz konventioneller und organischer Bewirtschaftung Köster, , Berlin (PhD thesis)
- Goedkoop , M , Effting , S and Collignon , M . 2000 . The Eco-indicator 99: a damage oriented method for Life Cycle Impact Assessment Manual for Designers PRé Consultants Amersfoort, , The Netherlands Available from URL: http://www.pre.nl/eco-indicator99/ei99-reports.htm
- Groffman , PM and Tiedje , JM . 1989 . Denitrification in north temperate forest soils: Relationships between denitrification and environmental factors at the landscape scale . Soil. Biol. Biochem , 21 : 621 – 626 .
- Groffman , PM , Tiedje , JM , Mokma , DL and Simkins , S . 1992 . Regional scale estimates of denitrification in north temperate forest soils . Landscape Ecol , 7 : 45 – 53 .
- Haag , D and Kaupenjohann , M . 2001 . Landscape fate of nitrate fluxes and emissions in Central Europe. A critical review of concepts, data, and models for transport and retention . Agric. Ecosyst. Environ , 86 : 1 – 21 .
- Haas , G , Wetterich , F and Geier , U . 2000 . Life Cycle Assessment Framework in Framework in Agriculture on the Farm Level . Int. J. LCA , 5 : 345 – 348 .
- Haas , G , Wetterich , F and Köpcke , U . 2001 . Comparing intensive, extensified and organic grassland farming in southern Germany by process life cycle assessment . Agric. Ecosyst. Environ , 83 : 43 – 54 .
- Hatano , R . 2002 . “ Modeling of carbon cycle under the influence of soil-plan interaction ” . In Prediction of Environmental Load – From Monitoring to Modeling , Edited by: Hasegawa , S , Hatano , R and Okazaki , M . 175 – 192 . Tokyo : Hakuyusya . (in Japanese)
- Hokkaido Central Agricultural Experiment Station . 1971 . Results of the Basic Survey on Soil Fertility Edited by: Mori , T . 1 – 019 . Hokkaido (in Japanese)
- Intergovernmental Panel on Climate Change . 1997 . “ Agriculture ” . In IPCC Guidelines for National Greenhouse Gas Inventories. Workbook , Paris : Intergovernmental Panel on Climate Change . Available from URL:http://www.ipcc-nggip.iges.or.jp/public/gl/invs5.htm
- Intergovernmental Panel on Climate Change . 2001 . Climate Change 2001: The Scientific Basis , Edited by: Houghton , JT , Ding , Y , Griggs , DJ , Noguer , M , van der Linden , PJ and Xiaosu , D . Cambridge : Cambridge University Press .
- Intergovernmental Panel on Climate Change 2006 2006 IPCC Guidelines for National Greenhouse Gas Inventories Volume 4 Agriculture, Forestry and Other Land UseAvailable from URL: http://www.ipcc-nggip.iges.or.jp/public/2006gl/vol4.htm
- Kimura , SD . 2005 . Creation of an eco-balance model to assess environmental risks caused by nitrogen load in a basin-agroecosystem , Hokkaido : Hokkaido University Graduate School of Agriculture . (PhD thesis)
- Kimura , SD and Hatano , R . 2007 . An eco-balance approach to the evaluation of historical changes in nitrogen loads at a regional scale . Agric. Sys , 94 : 165 – 176 .
- Kirkby , MJ , Imeson , AC , Bergkamp , G and Cammeraat , LH . 1996 . Scaling up processes and models from the field plot to the watershed and regional areas . J. Soil Water Conserv , 51 : 391 – 396 .
- Klöcking , B , Stroeob , B , Knoblauch , S , Maier , U , Pfuetzner , B and Gericke , A . 2003 . Development and allocation of land-use scenarios in agriculture for hydrological impact studies . Physics Chem. Earth , 28 : 1311 – 1321 .
- Koga , N , Sawamoto , T and Tsuruta , H . 2006 . Life cycle inventory-based analysis of greenhouse gas emissions from arable land farming systems in Hokkaido, northern Japan . Soil Sci. Plant Nutr , 52 : 564 – 574 .
- Kyaw , MK , Toyoda , K , Okazaki , M , Motobayashi , T and Tanaka , H . 2005 . A case study of nitrogen balance in a paddy field planted with whole crop rice (Oryza sativa cv. Kusahonami) during two rice-growing seasons . Biol. Fertili. Soils , 42 : 72 – 82 .
- Lark , RM , Milne , AE , Addiscott , TM , Goulding , KWT , Webster , CP and O’Flaherty , S . 2004 . Scale- and location-dependent correlation of nitrous oxide emissions with soil properties: an analysis using wavelets . Europ. J. Soil Sci , 55 : 611 – 627 .
- Li , CS , Narayanan , V and Harriss , RC . 1996 . Model estimates of nitrous oxide emissions from agricultural lands in the United States . Global Biogeochem. Cycl , 10 : 297 – 306 .
- Lim , B , Boileau , P Bonduki , Y . 1999 . Improving the quality of national greenhouse gas inventories . Environ. Sci. Policy , 2 : 335 – 346 .
- Matsumoto , N . 2000 . Development of estimation method and evaluation of nitrogen flow in regional areas . Bull. Natl. Inst. Agro-Environ. Sci , 18 : 81 – 152 . (in Japanese)
- Mikasa City . 2005 . Statistics Reports for Mikasa City , Japan : Mikasa City . (in Japanese)
- Moll , RH , Kamprath , EJ and Jackson , WA . 1982 . Analysis and interpretation of factors which contribute to efficiency of nitrogen utilization . Agron. J , 74 : 562 – 564 .
- Morishita , T , Hatano , R , Nagata , O , Sakai , K , Koide , T and Nakahara , O . 2004 . Effect of nitrogen deposition on CH4uptake in forest soils in Hokkaido, Japan . Soil Sci. Plant Nutr , 50 : 1187 – 1194 .
- Mu , ZJ , Kimura , SD and Hatano , R . Contributions of individual greenhouse gases from upland cropping systems in Central Hokkaido to the net global warming potentials . Proc. of the 3rd International Nitrogen Conference, Nanjing, China, October 2004 . Edited by: Zhu , Z , Minami , K and Xing , G . pp. 707 – 714 . Beijing : Science Press USA .
- Mu , ZJ , Kimura , SD and Hatano , R . 2006 . Estimation of global warming potential from upland cropping systems in central Hokkaido, Japan . Soil Sci. Plant Nutr , 52 : 371 – 377 .
- Naser , HM . 2006 . Evaluating the status of greenhouse gas budgets of paddy fields in central Hokkaido , Japan : Hokkaido University Graduate School of Agriculture, Hokkaido University . (PhD thesis)
- Naser , HM , Nagata , O , Tamura , S and Hatano , R . 2007 . Methane emissions from five paddy fields with different amounts of rice straw application in central Hokkaido, Japan . Soil Sci. Plant Nutr , 53 : 95 – 101 .
- Nishimune , A , Konno , T , Saito , G and Fujita , I . 1983 . The relationship between nitrogen fixation and grain yields of legumes on the main upland soils in Tokachi, Hokkaido . Res. Bull. Hokkaido Natl. Agric. Exp. Stn , 137 : 81 – 106 . (in Japanese)
- Organisation for Economic Co-Operation and Development . 2001 . Environmental Indicators For Agriculture. Methods and Results , Vol 3 , Paris : Organisation for Economic Co-Operation and Development .
- Pakrou , N and Dillon , P . 2000 . Key process of the nitrogen cycle in an irrigated and non-irrigated grazed pasture . Plant Soil , 224 : 231 – 250 .
- Palisade Corporation . 2002 . Guide to Using @RISK version 4.5 , Newfield : Palisade Corporation .
- Resource Council, Science and Technology Agency . 1982 . Standard Tables of Food Composition in Japan , vol 87 , 37 – 291 . Tokyo : Printing Bureau, Ministry of Finance . (in Japanese)
- Von Rheinbaben , W . 1990 . Nitrogen losses from agricultural soils through denitrification – A critical evaluation . Z. Pflanzenernaehr. Bodenk , 153 : 157 – 166 .
- Saika , M . 1990 . “ Grass values as nutrition ” . In Grassland Science , Edited by: Okubo , T . 157 – 166 . Tokyo : Buneido . (in Japanese)
- Sapporo District Meteorological Observatory2006Digital reading room. Day and annual climate data at Iwamizawa weather station.] Available from URL: http://www.data.kishou.go.jp/(in Japanese)
- Seyfried , MS . 2003 . “ Incorporation of remote sensing data in an upscaled soil water model ” . In Scaling Methods in Soil Physics , Edited by: Pachepsky , Y , Radcliff , DE and Selim , HM . 309 – 345 . Boca Raton : CRC press .
- Smith , KA and Dobbie , KE . 2001 . The impact of sampling frequency and sampling times on chamber-based measurements of N2O emissions from fertilized soils . Global Change Biol , 7 : 933 – 945 .
- Takahashi , K , Yoshida , K Suzuki , M . 1999 . Stand biomass, net production and canopy structure in a secondary deciduous broad-leaved forest, Northern Japan . Res. Bull. Hokkaido Univ. Forests , 56 : 70 – 85 .
- Toma , Y , Kimura , SD , Hirose , Y , Kusa , K and Hatano , R . 2007 . Variation of emission factor of N2O derived from chemical N fertilizer and organic matter – A case study of onion fields in Mikasa, Hokkaido, Japan . Soil Sci. Plant Nutr , 53 : 198 – 205 .
- Wagenet , RJ . 1998 . Scale issues in agroecological research chains . Nutr. Cycl. Agroecosys , 50 : 23 – 34 .
- Yagi , K and Minami , K . 1990 . Effect of organic-matter application on methane emission from some Japanese paddy fields . Soil Sci. Plant Nutr , 36 : 599 – 610 .
- Yan , X , Yagi , K , Akiyama , H and Akimoto , H . 2005 . Statistical analysis of the major variables controlling methane emission from rice fields . Global Change Biol , 11 : 1131 – 1141 .
- Yoshida , T . 1981 . “ Significance of symbiotic nitrogen fixation ” . In Sojyou Biseibutsu Kenkyu-kai , 305 – 310 . Tokyo : Hakuyu-sha . Eds Tshuchi no Biseibutsu (in Japanese)