Abstract
This paper investigates the impact of both geographical and relational proximity on the innovative performance of the firm. We address the role of one firm characteristic—its absorptive capacity—as a specific contingency affecting the relationship between different proximities and innovation. Using data from 158 high‐tech firms located in the Tiburtina Valley in Italy, we studied the relationship between these firms and their key customers. Our findings support the need to downplay the role of geographical proximity in promoting innovation. Our results also show that relational proximity to key customers has a complementary relationship with absorptive capacity, which positively moderates its influence on innovative performance.
Introduction
A steady stream of literature on industrial clusters, also known as industrial districts (Piore and Sabel Citation1984) or hot spots (Pouder and St. John Citation1996), has long maintained the importance of geographical proximity between organizations for innovation processes (Almeida and Kogut Citation1999; Folta, Cooper, and Baik Citation2006; Scott Citation2006; Woolley and Rottner Citation2008). However, some scholars have recently questioned the relationship between geographical proximity and innovation (Owen‐Smith and Powell Citation2004; Stuart and Sorenson Citation2003), arguing that the organization's knowledge base is rarely tied exclusively to local networks, even in the most advanced industrial clusters (Felzensztein, Gimmon, and Carter Citation2010; Molina‐Morales and Martinez‐Fernandez Citation2010). In addition to these widely held viewpoints, two recent developments addressing the core issue of innovation in industrial clusters have emerged (Camisón and Villar‐Lopez Citation2012). First, some authors have argued that the concept of proximity is multidimensional and not exclusively associated with the physical or geographical dimensions (Boschma Citation2005; Knoben and Oerlemans Citation2006). Additionally, other scholars, explaining the determinants of innovation in industrial clusters, have highlighted the uneven distribution of local knowledge resources due to firms' internal organizational resources and absorptive capacities (Cepeda‐Carrion, Cegarro‐Navarra, Jimenez‐Jimenez 2012; Giuliani and Bell Citation2005; Li, Veliyath, and Tan Citation2013; McCann and Folta Citation2008; Munari, Sobrero, and Malipiero Citation2012). Although the literature on innovation in clusters has argued that some firm characteristics might moderate the relationship between proximity and knowledge acquisition in start‐ups (Presutti, Boari, and Majocchi Citation2013), empirical evidence has yet to be collected regarding the moderating role of a key characteristic, namely, absorptive capacity, in the relation between proximity and innovation.
Taking into account these more recent perspectives, questions arise about the actual importance of distance to key partners to supporting innovative performance and, within this context, about the role played by a firm's internal attributes, such as absorptive capacity. In this study, we aim to answer two interrelated questions:
What is the direct impact of both geographical and relational proximities on the innovative performance of colocated firms?
What is the effect of the interaction between geographical and relational proximities and absorptive capacity on the innovative performance of colocated firms?
We tested our hypotheses through a survey of 158 firms located in a geographical cluster of small and medium‐sized high‐tech firms in the center of Italy, the Tiburtina Valley, which is close to Rome. We focus our attention on a high‐tech cluster for several reasons: in addition to the great importance of this type of cluster in the current environment (Fosfuri and Ronde Citation2004) and given the nature and relevancy of knowledge flows to innovation in high‐tech clusters, they constitute a suitable empirical context in which to address our research questions.
We found that geographical proximity between a firm and its key customers is always detrimental to innovative performance, regardless of whether there are high or low absorptive capacity levels. Moreover, we found that firms' innovation capabilities are positively influenced by high levels of relational proximity only when they also possess high absorptive capacity values.
These results are relevant, first, because they contribute to a better understanding of the importance of different dimensions of proximity in fostering innovation. Second, they contribute to evaluating the contingency role of internal resources, such as absorptive capacity, in the relationship between different forms of proximity and a firm's innovative performance.
We structure the remainder of the paper as follows. First, we propose the theoretical framework and justify our hypotheses. Then, we describe the empirical setting and method. We conclude with a discussion of the results and suggestions for further research.
Theoretical Framework
Innovation Processes, Dimensions of Proximity, and Absorptive Capacities
Over recent decades, there has been increasing interest in proximity and its potential effects on the competiveness and innovativeness of firms. Authors have emphasized the important role of geographical proximity in the success and development of areas characterized as Marshallian industrial districts (Becattini Citation1991; Brusco Citation1982). Districts and proximity have been considered the source of innovation and the foundation for developing winning regions (Piore and Sabel Citation1984). More recently, the Triple Helix perspective has used the notion of proximity as a central element of knowledge‐based systems. The knowledge‐based system transforms institutional conditions into a knowledge infrastructure or, in other words, a triple helix network of university‐industry‐government relations (Etzkowitz and Leydesdorff Citation2000). In a different context, the proximity notion has been applied to population density at the metropolitan level, demonstrating that the geographic proximity of individuals possessing high levels of human capital—skills, expertise, or creative capabilities—enables their interactions and that these interactions facilitate the spillover necessary for innovation (Knudsen et al. Citation2008). Similarly, authors like Florida (Citation2002) argue that it is the density and diversity of these talents in the territory that promote a small number of fast‐growing firms that in turn are the drivers of local development (Birch Citation1987; Kirchhoff Citation1994). Finally, from a strategic perspective, Porter (Citation1994) proposes the determinant role of proximity and location on competition and the competitive advantage of firms.
Considerable recent research has focused on analyzing those factors that affect a firm's innovation process (Yli‐Renko, Autio, and Sapienza Citation2001; Zahra and George Citation2002). According to these studies, firms' innovation processes derive more from interactions with external business actors than from a “solitary genius” (Burgelman and Hitt Citation2007; Davidsson, Citation2005; Smith and Cao Citation2007; Woolley and Rottner Citation2008). In other words, innovation is not a discrete process resulting from knowledge developed by an isolated firm but rather emerges through interactions (Ahuja, Citation2000; Slotte‐Kock and Coviello Citation2010) based mainly on external social relationships (Dyer and Singh Citation1998; Squire, Cousins and Brown Citation2006; Tsai and Ghoshal, Citation1998). This is the case for innovations driven by customers, and it was found to consistently hold even when drivers differ (e.g., for design‐driven innovations; Verganti Citation2011a).
These studies have also considered the location of the knowledge exchange process among partners (Audretsch and Lehmann Citation2006; Feldman Citation1994; Jaffe, Trajtenberg, and Henderson Citation1993), confirming the importance of geographical proximity given that knowledge spillovers are not without costs generated by geographical distance (Maskell and Malmberg Citation2007; Scott, Citation2006). Central to this argument is the traditional assumption in economics and geography that knowledge spillovers are geographically localized and locally bound (Alcacer and Chung Citation2007; Arikan Citation2009; Jaffe Trajtenberg, and Henderson Citation1993; Krugman Citation1991). Thus, stocks and flows of knowledge tend to be spatially concentrated and, to some extent, available only to the colocated actors (Bell, Tracey, and Heide Citation2009). In this context, innovation can be understood as a joint action among neighbors (Camisón and Villar‐Lopez Citation2012). Empirical studies confirm that spatially concentrated actors benefit from knowledge externalities, in that short distances literally bring people together, favor contacts for information exchange, and facilitate reciprocal exchanges of tacit knowledge (Gordon and McCann Citation2005; Maskell Citation2001; Waxell and Malmberg Citation2007).
Ever since Marshall (Citation1920) presented his seminal work on industrial districts, most scholars have accepted this positive view of colocation. However, an increasing number of subsequent studies have considered this argument to be overly simplistic, if not unrealistic, and have argued that there is no obvious relationship between externalities and geographical proximity (Huggins and Johnston Citation2010; Owen‐Smith and Powell Citation2004). According to Oinas (Citation1999), few empirical studies have proved that the process of knowledge exchange is actually local (Cooke Citation2001). Some studies even consider the role of physical or geographical proximity critically, arguing that it does not generate externalities per se (e.g., Boschma Citation2005). Instead, they suggest that the creation of new knowledge and interactive learning is more likely to occur inside nonlocal relationships and that the knowledge base is rarely tied exclusively to local networks (Owen‐Smith and Powell Citation2004; Saxenian Citation1994). Instead, global relationships provide crucial sources of knowledge that complement traditional local “buzz” as a dispenser of knowledge for the innovation process (Bathelt, Malmberg, and Maskell Citation2004; Presutti, Boari, and Majocchi Citation2013).
In summary, research suggests not only that geographical proximity is insufficient to account for all knowledge spillovers (Sorenson, Rivkin, and Fleming Citation2006) but also that the concept of proximity is not exclusively limited to physical or geographical proximity (Boschma Citation2005; Knoben and Oerlemans Citation2006). A way to explain these results would be to identify the different dimensions of proximity, investigating the role of relational, cognitive, and epistemic proximities in facilitating knowledge dynamics among entrepreneurs (Boschma Citation2005). This framework indicates that including the social and cognitive dimensions of proximity reduces the need for geographical proximity, especially in the case of tacit knowledge transfer. Specifically, relational proximity may capture both social and cognitive interorganizational proximity (Leana and Van Buren Citation1999; Nooteboom et al., Citation2007). Because other intangible dimensions of proximity are crucial to the transfer of knowledge in network relations, it is recognized that relational proximity between actors, and not geographical proximity, fosters knowledge spillovers. In other words, there is nothing inherently spatial about the innovation process (Breschi and Lissoni Citation2001).
To gain a complete picture of the current debate about the role of proximity in firms' innovation processes, the firms' internal resources must also be included. Giuliani and Bell (Citation2005), for example, found that the uneven and selective distribution of local resources and of knowledge affects innovation activity. In the context of proximity, the individual firm's knowledge base is an additive and distinct attribute of its systemic resources and capacities. Consequently, firms vary in their capacity to exploit opportunities (Munari, Sobrero, and Malipiero Citation2012). The differences in the amount of internal knowledge held by a firm generate an uneven and selective distribution of resources and knowledge being transferred and received in a close environment (Cepeda‐Carrion, Cegarro‐Navarra, and Jimenez‐Jimenez Citation2012; Giuliani and Bell Citation2005; Sammarra and Biggiero Citation2008). Among a company's internal attributes, R&D efforts related to the firm's knowledge base, and thus its absorptive capacity (Hervas‐Oliver et al. Citation2012), are particularly significant. Previous research has associated a firm's absorptive capacity with organizational learning and the ability to apply information received from external sources (Cohen and Levinthal Citation1989; Valdaliso et al. Citation2011). In addition, empirical findings demonstrate that such absorptive capacity exerts a positive influence on innovation (Cassiman and Veugelers Citation2002; Hotho, Becker‐Ritterspach, and Saka‐Helmhout Citation2012; Huerg, Citation2006). The literature on innovation in clusters still needs to develop a focused analysis of the contingency role of absorptive capacity in the relation between proximity and innovation.
Hypothesis Development
The current study focuses on firms' relationships with their key customers. Key customers are defined as a firm's largest customers in terms of proportion of sales revenue. Supplier–customer relationships are universally recognized as being crucial for innovation (Von Hippel Citation1977) and have become an integral part of business‐to‐business operating strategies (Felzensztein, Gimmon, and Carter Citation2010; Langfield‐Smith and Greenwood, Citation1998; Squire, Cousins, and Brown 2009).
To address our research questions, we focus our hypothesis development on some key aspects. First, we evaluate the impact on innovation of two different dimensions of proximity. Moreover, as we believe that internal firm factors may affect the relation between proximity and innovation, we evaluate the moderating effect of absorptive capacity. More specifically, at the empirical level, we first consider the direct effects of geographical (Hp. 1) and relational proximity (Hp. 2) on the innovative performance of the high‐tech firms of our sample. Then, we consider the interaction effects of absorptive capacity with geographical and relational proximity on innovative performance (Hp. 3; Hp. 4).
Geographical Proximity and Innovative Performance
The role of geographical proximity is still hotly debated, with some current authors suggesting a positive effect of colocation but others arguing that geographical proximity has a negative impact on innovation (Gilsing et al., Citation2008; Hoppmann et al. Citation2013). Recently, an increasing number of scholars have challenged the view that physical proximity, in the context of industrial clusters, favors firm innovation (Antonelli Citation2000; Breschi and Lissoni Citation2001). Some authors maintain that the negative effects are particularly strong in the high‐tech context (e.g., Breschi and Lissoni Citation2001), where links with dispersed sources of knowledge that are distributed worldwide are essential (Friesl et al. Citation2008). Moreover, local interactions can generate spatial lock‐in, which is detrimental to learning interactions and, ultimately, to innovation (Capó‐Vicedo, Expósito‐Langa, and, and Molina‐Morales Citation2008). This perspective is sometime ascribed to the idea that clusters tend to become close networks (Boschma Citation2005), which might even isolate firms from external and profitable sources of knowledge and information through a lock‐in effect (Bathelt, Malmberg, and Maskell Citation2004; Romanelli and Khessina Citation2005). To avoid such spatial lock‐in, firms might pursue distant relationships that provide access to the outside world (Hendry, Brown, and De Fillippi Citation2000). Another perspective, though not subverting the previous one, is more focused on the diversity of accessed knowledge. Burt (Citation2004) claims that when innovation is the key issue, the sources of knowledge might not be the focal question. Empirical research shows that what matters for innovation is how diverse and even contradictory the information and its possible interpretations are.
By combining these two perspectives and by focusing on the relationship with key customers, we argue that geographical proximity with key customers might have a negative impact on innovation.
Thus, we formulate the following hypothesis.
H1: For firms located in industrial clusters, the geographical proximity to key customers is negatively associated with their innovative performance.
Relational Proximity and Innovative Performance
The relational proximity dimension refers to both social and cognitive interorganizational proximity (Leana and Van Buren Citation1999; Moran and Galunic Citation1998; Nooteboom et al. Citation2007). According to reports on embeddedness (Granovetter Citation1985; Uzzi Citation1997), social proximity entails the level of trust between a local firm and its customers. Cognitive proximity focuses on similarities in how the actors perceive, interpret, and evaluate the world. High levels of social and cognitive proximity can reduce the knowledge distance between business partners (Boschma Citation2005), broadening their common knowledge base and expertise. Therefore, relational proximity facilitates knowledge acquisition and, particularly, tacit knowledge exchange. When relational proximity between partners is high, agents act similarly, and more knowledge can be transferred when practices, institutional legacies, and work cultures are comparable (Mowery, Oxley, and Silverman Citation1996). According to Dakhil and Clercq (Citation2004), higher levels of common values and culture are typically associated with higher levels of innovation. When agents are involved in the same network and have a shared vision, reinforced by reciprocal trust, they tend to have similar perceptions about how to act, which promotes mutual understanding and the exchange of ideas and resources (Tsai and Ghoshal Citation1998). Moreover, when firms have similar referral structures, knowledge can be communicated, transferred, and acquired more effectively and efficiently (Knoben and Oerlemans Citation2006). In this context, parties tend to share goals and have a common understanding of which innovation is needed. In turn, a common attitude regarding how to perform research activities emerges, leading to an improvement in innovative performance (Krause, Handfield, and Tyler Citation2007). Shared vision can be regarded as a mechanism favoring knowledge integration between parties in a network (Inkpen and Tsang Citation2005); in this sense, it is a critical element in the innovation process. Finally, the presence of trust in business relationships makes reciprocal knowledge exchange more efficient, thus allowing it to substitute for the formal contracts, incentives, and monitoring mechanisms associated with typical business transactions. This, in turn, reduces the risk of reciprocal opportunism and, more generally, lowers transaction costs, promoting knowledge exchange among partners (Nahapiet and Ghoshal Citation1998).
Conversely, cultural conflicts and misunderstandings between firms and their customers can limit information and knowledge acquisition as well as interorganizational learning. When goals and cultures are incongruent, misunderstandings and conflicts between firms and customers are more likely to arise. Parties may become disappointed and restrict information exchange, which will negatively affect outcomes for the firms (Inkpen and Tsang, Citation2005; Krause, Handfield, and Tyler Citation2007). In summary, relational proximity facilitates interactions between actors and access to external knowledge, regardless of geographical distance. These arguments suggest a positive association between social and cognitive proximity and knowledge acquisition and, thus, innovative performance. This idea leads to our second hypothesis.
H2: For firms located in industrial clusters, relational (social and cognitive) proximity to key customers is positively associated with their innovative performance.
The Moderating Role of Absorptive Capacity
Firms vary in their capacity to understand, develop, and use certain external resources, particularly those related to knowledge and innovation. Previous studies have identified a firm's absorptive capacity as a key factor in improving its ability to benefit from external resources (Cohen and Levinthal Citation1989; Valdaliso, Aranguren, and Lopez 2011). This capacity is also considered to be a measure of the firm's capacity to innovate and to develop new knowledge (Zahra and George Citation2002). Extant research argues in favor of a positive relationship between absorptive capacity and innovative performance (Cepeda‐Carrion, Cegarro‐Navarra, Jimenez‐Jimenez 2012; Hotho, Becker‐Ritterspach, and Saka‐Helmhout Citation2012).
In the case of key customer relationships, the propensity to use knowledge depends on the firm's own absorptive capacity and on the level of firm integration into networks (Tether and Tajar Citation2008). Knowledge provided by key customers typically requires complex and intensive interaction between companies, in which both parties participate in interactive learning (Hertog Citation2000; Sundbo Citation2001). For example, studying the case of firms based in Hong Kong, Wu (Citation2008) empirically demonstrates that repeated transactions among partners promote knowledge exchange, leading to a positive impact on firm performance. The literature has emphasized the complementarities between external knowledge provided by customers or knowledge providers and a company's resources and capabilities (Friesl Citation2012; Tether and Tajar Citation2008).
We share Zaheer and Bell's (Citation2005) perspective, which claims that external and internal resources must be analyzed together to offer a complete explanation of a firm's performance, including innovative performance. External and internal resources can interact and affect each other. Different scholars have started to look at internal factors, such as absorptive capacity, as contingency factors in the firm's use of external alliances (Park, Chen, and Gallagher Citation2002) and in its capacity to extract value from its network position (Shipilov Citation2009).
Studies on firms located in clusters found that the uneven and selective distributions of local resources and of knowledge affect innovation activity, with absorptive capacity playing a direct positive role on firms' innovative performance (Giuliani and Bell Citation2005; Nooteboom et al. Citation2007). More recently, some researchers claim that the cluster literature overemphasizes the importance of geographical proximity while underestimating the role of network relations and the capacity of the firm (Ter Wal and Boschma Citation2009). In line with this perspective, other researchers suggest a contingency role for firms' internal resources, such as absorptive capacity, which might improve their ability to exploit external resources and thereby enhance their innovative performance (Hervas‐Oliver et al. Citation2012; Presutti, Boari, and Majocchi Citation2013). Accordingly, we expect that the exploitation of external resources, accessed through more or less proximate relationships with key customers, will be greater for companies with higher levels of absorptive capacity. In our research, we expect that a firm's absorptive capacity moderates the relationship between geographical and relational proximity and the firm's innovative performance. More specifically, higher levels of absorptive capacity should mitigate the negative effects of geographical proximity to customers on a firm's innovative performance. Moreover, we expect that higher levels of absorptive capacity will strengthen the positive effects of the cognitive and social proximity of customers on innovative performance.
H3: For firms located in industrial clusters, the effect of geographical proximity to key customers on innovative performance is moderated by the level of their absorptive capacity, such that geographical proximity will have a less negative effect if the levels of absorptive capacity are high.
H4: For firms located in industrial clusters, the effect of relational (social and cognitive) proximity to key customers on innovative performance is moderated by the level of their absorptive capacity, such that relational proximity will have a stronger positive effect if the levels of absorptive capacity are high.
Methods
Sample and Data
Over recent decades, the development of high‐tech clusters has become a popular strategy for firms and regions, and successful high‐tech clusters create jobs and attract capital, talent, and many other types of resources (Fosfuri and Ronde Citation2004; Longhi and Keeble Citation2000). In these contexts, innovation processes become fully developed; however, because these processes are so complex, firms also become much more dependent on external flows of knowledge resources (Bresnahan, Gambardella, and Saxenian Citation2001).
The field setting of this research consists of a geographical cluster of small and medium‐sized high‐tech firms located in one of the most important areas in central Italy, the Tiburtina Valley, which is approximately 14 km from the center of Rome. As in Silicon Valley in the United States, the high‐tech firms located in this restricted urban context have created a homogeneous agglomeration that currently represents the most typical example of a high‐tech metropolitan cluster in Italy. This cluster is also known for its high export rates due to the presence of several important foreign multinationals. The high number of specialized firms located in this small area has led to the development of new businesses and the rapid diffusion of knowledge among local actors (Majocchi, Odorici, and Presutti Citation2016). This urban cluster has already been the focus of previous international studies (Presutti, Boari, and Majocchi Citation2011).
At the beginning of our data collection (September 2013), the sectors represented in this area were (1) electronics (320 firms); (2) media (200 firms); and (3) new economy (e.g., manufacturers of new hardware, firms in the information services industry, Internet access providers, and telecommunication network managers; 522 firms). We focused on the electronics sector, which, according to the definition of the National Federation of Electronics Firms, consists of the computer industry, electronics in the strictest sense, and telecommunications. Specifically, we tested our hypotheses using survey data from 158 high‐tech firms in the electronics sector, defined according to standard industrial classification (SIC) codes, located within this cluster.
The data to test our hypotheses come from a direct survey using a specially designed questionnaire. The data collection process lasted approximately 5 months. The survey process was developed in four phases: (1) conducting literature reviews to develop the measurement scales; (2) developing the questionnaire (structured in a closed question‐answer form); (3) pretesting the questionnaire on three randomly selected sample firms; and (4) data collection. The firms in our sample belong to the “specialized suppliers” category according to the Pavitt classification in that they primarily produce and offer technology and services to their industrial customers. In this case, customers also access technology through the acquisition of products from suppliers (Yanez, Khalil, and Walsh Citation2010). Thus, for both customers and suppliers, firm knowledge acquisition and exploitation processes depend to a great extent on interactive learning between them. This situation encourages local firms to become increasingly specialized, resulting in strong differentiation in their internal knowledge bases. The R&D activity and the scope and extent of new products launched for these firms are strongly conditioned by knowledge acquired from their customers because new products need to be in line with customers' specific requirements (Dyer and Singh Citation1998).
To ensure that all firms in our sample were involved in innovation activities, we checked their business descriptions in the source database. Firms with no internal R&D activity or who only offered nontechnical services were excluded from the study. This process left a total of 275 firms, 158 of which accepted our request for a personal interview to complete the questionnaire (58% response rate). A comparison of differences in the mean values of the responding and nonresponding firms revealed no statistically significant response bias in terms of their 3‐year average sales revenues, firm age, or number of employees.
Our key informant was the entrepreneur. Firms were contacted by telephone to obtain the names of potential respondents (the entrepreneurs) and to determine whether they would agree to complete the survey through face‐to‐face interviews. The focus of our empirical research was on the vertical relationship between each firm and the customer that accounted for the highest proportion of sales revenue for the previous year (Yli‐Renko, Autio, and Sapienza Citation2001). Using a semistructured questionnaire, entrepreneurs were asked to use seven‐point Likert scales to express their opinions about all items. These items were selected to measure relational proximity with the firm's key customers. Moreover, entrepreneurs were asked to indicate the customer's location, the duration of their business relationship, the size and age of both firms, and the ratio of R&D expenditures of both firms to total sales. Finally, to capture innovation activity, firms were asked to indicate the number of new products developed as a result of this key customer relationship.
Overall, the firms in the sample developed an average of 7.3 new products per year (range 1–25). The average sales of the respondent firms amounted to €814,000, with an average age of 18.10 years. In addition, the average R&D expenditure in relation to total sales was 3.67% (range 0.01–15%). The key customers are similar in age to their suppliers (M = 18.16 years) but were on average smaller (Msales = €530.63). The number of key clients located within the district is 54. Table reports the descriptive statistics for the untransformed variables.
Table 1. Descriptive Statistics of the Untransformed Variables
Measures
Dependent Variable: Innovative Performance
We measured innovation activity by asking the firms how many new products or services they had developed during the previous three years as a result of the relationship with their key customers. We consider both product and services without distinction (Walsh and Linton 2011) in order to focus our analysis on the all range of innovation activities the impact on firms' sales (McDermott and Walsh Citation2001).
Independent Variables
Absorptive Capacity (AbsCap)
Following other recent research on measuring absorptive capacity (e.g., Cohen and Levinthal Citation1989; Meeus, Oerleman, and Hage Citation2001), we used R&D spending as a proxy to measure the level of a firm's investment in absorptive capacity useful to its innovation activity. This parameter was measured by considering the log value of the average ratio between R&D expenditures and total sales for the previous 3 years.
Geographical Proximity Between Actors (GeoProx)
In accordance with previous studies (Boschma Citation2005; Rallet and Torre Citation2000), we distinguished between key customers located within the district and those located outside. We defined a dummy variable equal to 1 for customers located inside the cluster and 0 otherwise. The number of customers located within the district is 54 (34.1%).
Relational Proximity (RelProx)
We used multi‐item measures to analyze the relational proximity between the firm and its key customer because these measures provide considerable advantages over single‐item measures (e.g., Churchill Citation1979). In our research, we use the relational proximity dimension to capture both social and cognitive interorganizational proximity, in accordance with many significant studies (e.g., Leana and Van Buren Citation1999; Moran and Galunic Citation1998; Nooteboom et al., Citation2007). According to literature concerning embeddedness (Granovetter Citation1985; Presutti, Boari, and Majocchi Citation2011; Uzzi Citation1997), the social proximity dimension measures the level of trust between a local firm and its customers. In particular, we focus on three dimensions of interorganizational trust: lack of opportunistic behavior, creation of common investments (degree of commitment), and presence of informal relationships. We selected six items to measure these dimensions of trust that have been used in many previous studies on trust, networks, and social capital. The cognitive proximity dimension encompasses similarities in the ways that firms and customers perceive, interpret, and evaluate the world. Therefore, a high level of cognitive proximity (Boschma Citation2005) can reduce the distance between the knowledge bases of business partners, thereby broadening their common knowledge base and expertise. In line with previous studies (Nahapiet and Ghoshal Citation1998), we measured the cognitive proximity between business partners with six items using seven‐point Likert scales. These items capture two interconnected aspects of relational cognitive proximity. First, they reflect the extent to which the business relationship is characterized by the development of common goals, norms, and reciprocal expectations involving the goodwill and trustworthiness of the exchange partner (Tsai and Ghoshal Citation1998). Second, the selected items verify the overlap of personal and business interests among partners, that is, the extent to which the business relationship is characterized by personal social ties (Nahapiet and Ghoshal Citation1998).
To verify the unidimensionality of the relational proximity construct (Churchill Citation1979), we submitted the scales used to analyze both cognitive and social dimensions to a factor analysis. Our original selection of 12 items was unsatisfactory from a statistical viewpoint (Kaiser Citation1960) because some single factors had eigenvalues greater than 1.0, and the loadings of some items did not exceed the cut‐off point of 0.30. Moreover, our scale showed a low degree of internal reliability (Nunnally Citation1978). Thus, by submitting the items to factor analysis, we reduced the number of items from 12 to 6 (3 items for social and 3 for cognitive proximities) to measure relational proximity. With this reduced combination of items, the measurement model performed well. The selected constructs demonstrate good internal consistency and reliability (details are included in the next section). The measure is a reflective manifestation of the underlying constructs as defined by Edwards (Citation2011) because it is the outcome of unobserved latent variables. Because we expected the effect of relational proximity to decrease for higher values of the variable, we considered a logarithmic specification of this variable.
Control Variables
We included some control variables to isolate the effect of the independent variables in the model. First, an important factor influencing innovation activity is the size of the involved partners (Acs and Audretsch Citation1991; Mowery, Oxley, and Silverman Citation1996; Tsai and Ghoshal Citation1998), in line with the idea that larger firms may invest more resources in R&D activities. As several studies suggest (e.g., Kogut and Zander Citation1992), both superior resources and economies of scale allow larger firms to exploit external knowledge successfully for their innovation process. We controlled for the effects of size by including the total sales of both the firms (Size) and their customers (CustSize).
Moreover, we included two additional control variables to evaluate the age of the firm (FirmAge) and of that its customers (CustAge). We assume that these elements will have an influence on the firm's learning ability and its knowledge exploitation process (Zahra, Jennings, and Kuratko Citation1999). We computed the age of the firms and of the customers as the number of years since their foundation. We used the log of these values to account for declining effects at higher values. Table reports the descriptive statistics of the variables and their correlations.
Table 2. Descriptive Statistics and Correlations
Reliability, Validity, and Data Analysis
Several precautions were taken to ensure that our data are reliable and valid. First, as mentioned previously, we pretested the survey with three entrepreneurs from our sample. Second, we used multi‐item measures to measure relational proximity because they provide considerable advantages over single‐item measures (Churchill Citation1979). We measured these items on the questionnaire using seven‐point Likert scales. As a first step of measure validation, to assess the unidimensionality of the research constructs (Churchill Citation1979), we factor analyzed the final scales with six items using the principal axis method, positing a single factor (exploratory factor analysis). After exploring the factor structure of the data, we submitted the data to confirmatory factor analysis. The results of this analysis verify that the measurement model performed well because the selected constructs demonstrate good internal consistency and reliability: the standardized factors are all above the recommended minimum of 0.40, and the average variances extracted are all above the recommended minimum of 0.50 (range 0.59–0.88). The composite reliabilities are all above the recommended minimum of 0.70 (Table ). Finally, the CFA showed that the measurement models fit the data reasonably well, with a goodness‐of‐fit index superior to 0.9 (GFI).
Table 3. Factor Analysis on Relational Proximity
Analysis, Techniques, and Results
To test our hypotheses, four models were analyzed using the ordinary least squares technique with Release 11 of the Stata software package. The estimated equation is:
where α0 is the constant intercept, βi is the regression coefficient, and ε is the error term.
Table presents the regression results for all models. The results are fairly constant across all specifications, and the explanatory power of the models is highly significant in terms of both R‐squared and adjusted R‐squared values. These results suggest that the models have been correctly specified and are stable. To determine whether our results are subject to multicollinearity, the variance inflation factors (VIFs) were also calculated for all models. The values of the mean VIF scores, which were well below the commonly used critical value of 10 (Cohen et al. Citation2003) and even the more restricted value of 5, suggest that in our study, multicollinearity is not a concern. The first column, Model 1, shows the estimated coefficients for the model containing our four control variables and the variable measuring absorptive capacity. The coefficients of the size variables were positive and statistically significant for the firm, its key clients, and absorptive capacity. These variables influenced the firm's level of innovative performance. In contrast, the variables on the age of both the firms and their clients were <0 but were not statistically significant. Therefore, our results did not support the view that age was a critical issue in determining a firm's innovative performance.
Table 4. Regression ResultsFootnotea
Models 2–4 include the independent variables, leading to a significant increase in the overall explanatory power of the models and confirming the significance of our independent variables. In Model 2, we tested for the impact of the dummy variable that gauges the location of clients within the cluster (GeoProx). The coefficient of the variable was negative and strongly significant (β = −3.23; p < .01), suggesting a relevant negative effect of being located nearby. These results fully support Hypothesis 1 in the sense that geographical proximity to key customers is detrimental to innovative performance. Next, Model 3 determined whether relational proximity had a positive effect on innovative performance (RelProx). In support of Hypothesis 2, the coefficient is positive and significant (β = 0.758; p < .10).
Next, we tested Hypotheses 3 and 4 using interaction terms to investigate the role of absorptive capacity in moderating the impact of relational and geographical proximities. Hypothesis 3 predicted that absorptive capacity would mitigate the negative effect of geographical proximity on innovation activity, whereas Hypothesis 4 predicted that it would reinforce the positive effect of relational proximity on innovation activity. Model 4 showed that although relational proximity loses its significance, there are significant interaction effects among the variables, as shown in Figures 1 and 2. To select high and low values, we followed the suggestions of Aiken, West, and Reno (Citation1991) in using 1 and 0 for categorical variables, as in the case of our geographical variable, and in using values corresponding to 1 S.D. above and 1 S.D. below the mean for continuous variables, as in the relational proximity case. The Y‐axis represents the dependent variable (innovation activity), and the X‐axis represents low and high geographical proximity, that is, within and outside the cluster (Figure 1), and low and high relational proximity (Figure 2). Figure 1 clearly shows (as both slope lines decrease) that Hypothesis 3 is not confirmed. Innovative performance is negatively influenced by geographical proximity for both high and low absorptive capacity levels. Moreover, geographical proximity, that is, being located in the same cluster as clients, has a particularly negative impact when the absorptive capacity is higher.
Figure 1. Innovation Activity: The Interaction Effect of Geographical Proximity and Absorptive Capacity
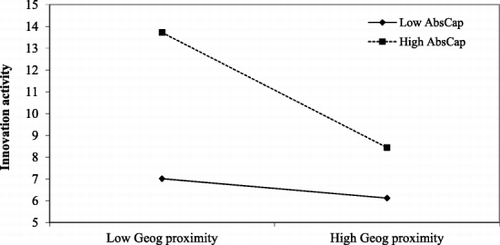
Figure 2. Innovation Activity: The Interaction Effect of Relational Proximity and Absorptive Capacity
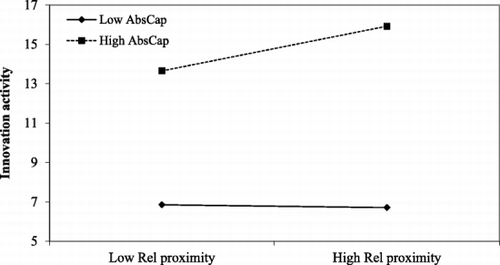
Figure 2 shows that innovative performance is clearly not influenced by a high level of relational proximity when the absorptive capacity of the firm is low. Only for high absorptive capacity values is innovative performance positively influenced by high levels of relational proximity to customers.
Discussion, Implications and Limitations
This paper adopted a multidimensional perspective on proximity to explore the effects of the geographical and relational (social and cognitive) proximities of high‐tech firms to their key customers in terms of innovative performance. Moreover, we investigated whether the firm's level of absorptive capacity moderates these effects.
First, our results suggest that the geographical proximity between firms and key customers reduces firms' innovative performance. Having customers located in the same cluster has a detrimental effect on firms' innovative performance. Geographical proximity with partners isolates firms from external sources that could be beneficial to their innovation activity through a lock‐in effect (Bathelt, Malmberg, and Maskell Citation2004; Romanelli and Khessina Citation2005). In this direction, other studies explain the negative impact of geographical proximity on innovation according to the network inertia problem (Boschma Citation2005; Uzzi, Citation1997), which limits the ability of a firm to exploit knowledge from different external sources of innovation when overembedded with geographically concentrated customers.
Regarding our specific context of high‐tech firms, the results could be ascribed to the fact that nearby business partners already have the same technological information as local firms, whereas exposure to many different external sources of technological knowledge is essential for exploring new innovation opportunities. This idea was supported by the characteristics of the larger and younger key customers of our sample; innovation opportunities could be better accessed through relationships with innovative and highly active customers located far from the cluster. The diversity associated with distant contacts can be essential for innovative contexts such as the investigated cluster (Friesl Citation2012; Hendry, Brown, and De Fillippi 2000). This idea is very much in line with what specifically happens in our empirical setting, where high‐tech firms require primary exploration activities to capture new ideas and improvements beyond the exploitation of the elements/resources/products they already have. These exploration activities are likely to be much better developed with distant rather than close partners, thanks to the diverse information and knowledge that can be accessed and combined (Gilsing et al., Citation2008; Hendry and Brown Citation2006; Hoppmann et al. Citation2013; Presutti, Boari, and Fratocchi Citation2007). Accordingly, our findings provide further support to the idea that diversity in accessed and combined information and knowledge could be much more important than a pure source (Burt Citation2004).
As expected, relational proximity was found to exert a positive impact on a firm's innovative performance. This result further supports the importance of following a broader multidimensional view of the proximity concept while also considering intangible proximity dimensions as important factors explaining the innovation process (Breschi and Lissoni Citation2001; Boschma Citation2005; Ter Wal and Boschma Citation2009; Torre Citation2008). Our results confirm that for high‐tech firms and their key customers, relational proximity seems even more relevant for knowledge transmission. Key customers can reinforce both the creation of new products and the technological distinctiveness of their primary suppliers. External knowledge is a particular requirement because high‐tech firms must leverage interorganizational customer relationships to broaden their stock of knowledge regarding both technology and R&D activity (Pirolo and Presutti Citation2010).
We found that a firm's absorptive capacity influences the role of geographical and relational proximity in innovation. In particular, and contrary to our expectations, we found that innovative performance is negatively influenced by geographical proximity to customers, regardless of whether the firm has high or low levels of absorptive capacity. Geographical proximity to customers has a particularly negative impact when absorptive capacity levels are high, thus suggesting that knowledgeable firms could be harmed by geographically proximate key customers.
Finally, with respect to the role played by absorptive capacity in the relation between relational proximity and innovation, we found that innovative firm performance was positively influenced by higher levels of relational proximity to customers only for firms with high absorptive capacity. In contrast, this proximity does not influence the innovative performance of firms with a lower level of absorptive capacity. This observation suggests that the combination of significant internal capacities and relational proximity is what matters most for innovative performance.
Our findings contribute to the literature on innovation in clusters, where the role of contingency factors in the relationship between proximity and innovation, although theoretically addressed (Presutti, Boari, and Majocchi Citation2013), still requires empirical evidence. In particular, our contribution focused on the moderating role of absorptive capacity.
Regarding the contingency role of absorptive capacity, our findings also illustrate that relational proximity has a complementary relationship with absorptive capacity, which positively moderates its influence on innovative firm performance. In contrast, absorptive capacity cannot substitute for a high level of geographical proximity by moderating its negative influence on innovative firm performance. A combination of high absorptive capacity and relational proximity to distant partners appears to be desirable.
By addressing the substitutive or complementary role of a firm's contingency factors, we also contribute to a growing focus in the literature on network advantage, which recognizes that individual actors might differently benefit from their network relations according to specific external contingencies (such as industry context and uncertainty characteristics) (Ahuja Citation2000) and internal contingencies (such as absorptive capacity, bargaining power, and protection against noncooperation of partners) (Shipilov Citation2009).
Our findings contribute to the more general understanding of the internal heterogeneity of knowledge distribution in clusters and its relation with innovation. Firm investments in high levels of absorptive capacity can help explain the access to external distant sources of knowledge, the impact on their innovative performance (Romanelli and Khessina Citation2005) and, consequently, the selective and uneven distribution of knowledge in the cluster (Giuliani and Bell Citation2005).
Finally, the insights obtained from this study contribute, even if indirectly, to the debate on the role of heterogeneous, that is, nonredundant, information and knowledge accessed through network relations and their impact on firms' innovative performance, a topic that is drawing greater attention in studies on geographical clusters and networks (Molina‐Morales and Martinez‐Fernandez Citation2006).
A large set of managerial implications arises from this study. Cluster firms should actively develop relationships with distant customers to favor knowledge acquisition useful to innovative performance (Presutti, Boari, and Fratocchi 2016). Firms must select those distant customers who can act as bridges to enable a local firm to enlarge its knowledge sources and innovation ideas. Thus, a well‐developed system of “global pipelines” connecting local firms to customers spread around the world would be extremely beneficial for a firm's innovation activity. In addition, our study highlights the importance of investing in absorptive capacity to benefit from relational proximity to customers. By combining high investments in absorptive capacity with relational proximity, firms are able to increase their innovative performance. Implications in terms of the role of nonredundant information accessed through relationally strong partnerships with geographically distant customers also arise from our study.
Some limitations of our study must be discussed to pinpoint opportunities for further research. First, our results refer to a high‐tech cluster. To generalize our theory, a test with more traditionally oriented clusters should be performed. Second, our definition of innovative performance is limited to the number of products and services developed by these firms in the past 3 years, regardless of the drivers of innovation (customer, process, design, etc.) and with no distinction between different types of innovation (i.e., disruptive and incremental). Further research could try to develop this point by addressing heterogeneity in innovation processes. Additionally, in this study, absorptive capacity was conceptualized only as an internal resource and as an indicator of capabilities. Further research could extend into other dimensions of strategic internal assets to gain a more complete picture of the importance of the mediating role of firms' internal resources and capabilities (Fernhaber, McDougall‐Covin, and Shepherd Citation2009). Researchers could also include other significant boundary conditions that might influence the importance of distant and near customer relationships for firms' innovation processes.
Finally, given that our study analyzes geographic and social proximity separately, further research could consider more complex relationships among these factors, such as combining effects, in line with the previous works of Parra‐Raquena, Molina Morales, and García Villaverde (Citation2010) and Nooteboom et al. (Citation2007).
Additional information
Notes on contributors
Manuela Presutti
Manuela Presutti is associate professor at the Department of Management, University of Bologna.
Cristina Boari
Cristina Boari is full professor at the Department of Management, University of Bologna.
Antonio Majocchi
Antonio Majocchi is full professor at the Department of Management, University of Pavia.
Xavier Molina‐morales
Xavier Molina‐Morales is full professor at the Department of Business Administration and Marketing, Universitata Jaume I.
References
- Acs, Z. J., and D. B. Audretsch (1991). “Innovation and Technological Change: An Overview,” in Innovation and Technological Change: An International Comparison. Eds. Z. J. Acs and D. B. Audretsch, Ann Arbor, University of Michigan Press, 39–59.
- Ahuja, G. (2000). “Collaboration Networks, Structural Holes, and Innovation: A Longitudinal Study,” Administrative Science Quarterly 45, 425–455.
- Aiken, L. S., S. G. West, and R. Reno (1991). Multiple Regression: Testing and Interpreting Interactions. Newbury Park, CA: SAGE Publications.
- Alcacer, J., and W. Chung (2007). “Location Strategies and Knowledge Spillovers,” Management Science 53(5), 760–776.
- Almeida, P., and B. Kogut (1999). “Localization of Knowledge and the Mobility of Engineers in Regional Networks,” Management Science 45, 905–917.
- Antonelli, C. (2000). “Collective Knowledge Communication and Innovation: The Evidence of Technological Districts,” Regional Studies 34(6), 535–547.
- Arikan, A. T. (2009). “Interfirm Knowledge Exchanges and the Knowledge Creation Capability of Cluster,” Academy of Management Review 34(4), 658–678.
- Audretsch, D. B., and F. Lehmann (2006). “Entrepreneurial Access and Absorption of Knowledge Spillovers: Strategic Board and Managerial Composition for Competitive Advantage,” Journal of Small Business Management 44(2), 155–166.
- Bathelt, H., A. Malmberg, and P. Maskell (2004). “Clusters and Knowledge: Local Buzz, Global Pipelines and the Process of Knowledge Creation,” Human Geography 28(1), 31–56.
- Becattini, G. (1991). “The Industrial District as a Creative Milieu,” in Industrial Change and Regional Development: The Transformation of New Industrial Spaces. Eds. G. Benko and M. Dunford. London: Belhaven Press, 150–180.
- Bell, S. J., P. Tracey, and J. B. Heide (2009). “The Organization of Regional Clusters,” Academy of Management Review 34(4), 623–642.
- Birch, D. (1987). Job Creation in America: How Our Smallest Companies Put the Most People to Work. New York: The Free Press.
- Boschma, R. A. (2005). “Role of Proximity in Interaction and Performance: Conceptual and Empirical Challenges,” Regional Studies 39(1), 41–45.
- Breschi, S., and F. Lissoni (2001). “Knowledge Spillovers and Local Innovation Systems: A Critical Survey,” Industrial and Corporate Change 10(4), 975–1005.
- Bresnahan, T., A. Gambardella, and A. Saxenian (2001). “Old Economy' Inputs for ‘New Economy’ Outcomes: Cluster Formation in the New Silicon Valleys,” Industrial and Corporate Change 10(4), 835–860.
- Brusco, S. (1982). “The Emilian Model: Productive Decentralization and Social Integration,” Cambridge Journal of Economics 2, 167–184.
- Burgelman, R. A., and M. A. Hitt (2007). “Entrepreneurial Action, Innovation and Approbriability,” Strategic Entrepreneurship Journal 1(3‐4), 349–352.
- Burt, R. S. (2004). “Structural Holes and Good Ideas,” American Journal of Sociology_ 110(2), 349–399.
- Camisón, C., and A. Villar‐lopez (2012). “On How Firms Located in an Industrial District Profit from Knowledge Spillovers: Adoption of an Organic Structure and Innovation Capabilities,” British Journal of Management 23(3), 361–382.
- Capó‐vicedo, J., M. Expósito‐langa, and, and F. X. Molina‐morales (2008). “Improving SME Competitiveness Reinforcing Interorganizational Networks in Industrial Clusters,” International Entrepreneurship and Management Journal 4(2), 147–169.
- Cassiman, B., and R. Veugelers (2002). “R&D Cooperation and Spillovers: Some Empirical Evidence from Belgium,” American Economic Review 92(4), 1169–1184.
- Cepeda‐carrion, G., J. G. Cegarro‐navarra, and D. Jimenez‐jimenez (2012). “The Effects of Absorptive Capacity on Innovativeness: Context and Information Systems Capability as Catalysts,” British Journal of Management 23(1), 110–129.
- Churchill, G. A. (1979). “A Paradigm for Developing Better Measures of Marketing Constructs,” Journal of Marketing Research 16(1), 64–73.
- Cohen, J., P. Cohen, S. West, and L. Aiken (2003). Applied Multiple Regression/Correlation Analysis for the Behavioural Sciences, 3rd ed. Mahwah, NJ: Lawrence Erlbaum Associates.
- Cohen, W. M., and D. A. Levinthal (1989). “Innovation and Learning: The Two Faces of R&D,” Economic Journal 99, 569–596.
- Cooke, P. (2001). “Regional Innovation Systems, Clusters, and the Knowledge Economy,” Industrial and Corporate Change 10(4), 945–974.
- Dakhil, M., and D. D. Clercq (2004). “Human Capital, Social Capital, and Innovation: A Multi‐Country Study,” Entrepreneurship and Regional Development 16(2), 107–128.
- Davidsson, P. (2005). Researching Entrepreneurship. New York: Springer.
- Dyer, J. H., and H. Singh (1998). “The Relational View: Cooperative Strategy and Sources of Interorganizational Competitive Advantage,” Academy of Management Review 23(4), 660–679.
- Edwards, J. R. (2011). “The Fallacy of Formative Measurement,” Organizational Research Methods 14(2), 370–388.
- Etzkowitz, H., and L. Leydesdorff (2000). “The Dynamics of Innovation: From National Systems and ‘Mode 2’ to a Triple Helix of University‐Industry‐Government Relations,” Research Policy 29(2), 109–123.
- Expósito‐langa, M., F. X. Molina‐morales, and J. Capó‐vicedo (2011). “New Product Development and Absorptive Capacity in Industrial Districts: A Multidimensional Approach,” Regional Studies 45(3), 319–331.
- Feldman, M. P. (1994). “Knowledge Complementarity and Innovation,” Small Business Economics 6(3), 363–372.
- Felzensztein, C., E. Gimmon, and S. Carter (2010). “Geographical Co‐Location, Social Networks and Inter‐firm Marketing Co‐Operation: The Case of the Salmon Industry,” Long Range Planning 43(5‐6), 675–690.
- Fernhaber, S., P. Mcdougall‐covin, and D. Shepherd (2009). “An International Entrepreneurship: Leveraging Internal and External Knowledge Sources,” Strategic Entrepreneurship Journal 3(4), 297–310.
- Florida, R. L. (2002). The Rise of the Creative Class: And How It's Transforming Work, Leisure, Community and Everyday Life. New York: Basic Books.
- Folta, T. B., A. Cooper, and Y. Baik (2006). “Geographic Cluster Size and Firm Performance,” Journal of Business Venturing 21(2), 217–242.
- Fosfuri, A., and T. Ronde (2004). “High‐Tech Clusters, Technology Spillovers, and Trade Secret Laws,” International Journal of Industrial Organization 22, 45–65.
- Friesl, M. (2012). “Knowledge Acquisition, Strategies and Company Performance in Young High Technology Companies,” British Journal of Management 23(3), 325–343.
- Friesl, M., B. A. Gilbert, P. P. Mcdougall, D. B. Audretsch (2008). “Clusters, Knowledge Spillovers and New Venture Performance: An Empirical Examination,” Journal of Business Venturing 23(4), 405–422.
- Gilsing, V., B. Nooteboom, W. Vanhaverbeke, and G. Duyster (2008). “Network Embeddedness and the Exploration of Novel Technologies: Technological Distance, Betweenness Centrality and Density,” Research Policy 37(10), 1717–1731.
- Giuliani, E., and M. Bell (2005). “The Micro‐Determinants of Meso‐Level Learning and Innovation: Evidence from a Chilean Wine Cluster,” Research Policy 34(1), 47–68.
- Gordon, I., and P. Mccann (2005). “Innovation, Agglomeration and Regional Development,” Journal of Economic Geography 5(5), 523–543.
- Granovetter, M. (1985) “Economic‐Action and Social‐Structure: The Problem of Embeddedness,” American Journal of Sociology 91(3), 481–510.
- Hendry, C., and J. Brown (2006). “Organizational Networking in UK Biotechnology Clusters,” British Journal of Management 17(1), 55–73.
- Hendry, C., J. Brown, and R. De Fillippi (2000). “Regional Clustering of High Technology Based Firms: Opto‐Electronics in Three Countries,” Regional Studies 34(2), 129–144.
- Hertog, D. (2000). “Knowledge‐Intensive Business Services as Co‐Producers of Innovation,” International Journal of Innovation Management 4(4), 491–528.
- Hervas‐oliver, J., J. Albors‐garrigos, B. De‐miguel, and A. Hidalgo (2012). “The Role of a Firm's Absorptive Capacity and the Technology Transfer Process in Clusters: How Effective Are Technology Centres in Low‐Tech Clusters?,” Entrepreneurship & Regional Development 24(7‐8), 523–559.
- Hoppmann, J., M. Peters, M. Schneider, and V. H. Hoffmann (2013). “The Two Faces of Market Support‐How Deployment Policies Affect Technological Exploration and Exploitation in the Solar Photovoltaic Industry,” Research Policy 42(4), 989–1003.
- Hotho, J. J., F. Becker‐ritterspach, and A. Saka‐helmhout (2012). “Enriching Absorptive Capacity through Social Interaction,” British Journal of Management 23(3), 383–401.
- Huergo, E. (2006) “The Role of Technological Management as a Source of Innovation: Evidence from Spanish Manufacturing Firms,” Research Policy 35(9), 1377–1388.
- Huggins, R., and A. Johnston (2010). “Knowledge Flow and Inter‐Firm Networks: The Influence of Network Resources, Spatial Proximity and Firm Size,” Entrepreneurship & Regional Development 22(5), 457–484.
- Inkpen, A. C., and E. W. K. Tsang (2005). “Social Capital, Networks, and Knowledge Transfer,” Academy of Management Review 30(1), 146–165.
- Jaffe, A. B., M. Trajtenberg, and R. Henderson (1993). “Geographic Localization of Knowledge Spillovers as Evidenced by Patent Citations,” Quarterly Journal of Economics 108(3), 577–598.
- Kaiser, H. F. (1960). “The Application of Electronic Computers to Factor Analysis,” Educational and Psychological Measurement 20(2), 141–151.
- Kirchhoff, B. A. (1994). Entrepreneurship and Dynamic Capitalism: The Economics of Business Firm Formation and Growth. Westport, CT: Praeger.
- Knoben, J., and L. A. G. Oerlemans (2006). “Proximity and Inter‐Organizational Collaboration: A Literature Review,” International Journal of Management Reviews 8(2), 71–89.
- Knudsen, B., R. Florida, K. Stolarick, and G. Gates (2008). “Density and Creativity in U.S. Regions,” Annals of the Association of American Geographers 98(2), 461–478.
- Kogut, B., and U. Zander (1992). “Knowledge of the Firm, Combinative Capabilities, and the Replication of Technology,” Organization Science 3(3), 383–397.
- Krause, D. R., R. B. Handfield, and B. B. Tyler (2007). “The Relationships between Supplier Development, Commitment, Social Capital Accumulation and Performance Improvement,” Journal of Operations Management 25(2), 528–545.
- Krugman, P. (1991). Geography and Trade. Cambridge, MA: MIT Press.
- Langfield‐smith, K., and M. R. Greenwood (1998). “Developing Co‐Operative Buyer‐Supplier Relationships: A Case Study of Toyota,” Journal of Management Studies 35(3), 331–354.
- Leana, C. R., and H. J. Van Buren (1999). “Organizational Social Capital and Employment Practices,” Academy of Management Review 24(3), 538–555.
- Li, W., R. Veliyath, and J. Tan (2013). “Network Characteristics and Firm Performance: An Examination of the Relationships in the Context of a Cluster,” Journal of Small Business Management 51(1), 1–22.
- Longhi, C., and D. Keeble (2000). “High‐Technology Clusters and Evolutionary Trends in the 1990s,” in High‐Technology Clusters, Networking and Collective Learning in Europe. Eds. D. Keeble and F. Wilkinson. Aldershot: Ashgate, 21–56.
- Majocchi, A., V. Odorici, and M. Presutti (2016). “Firms' Ownership and Internationalisation: Is It Context That Really Matters?,” European Journal of International Management 10(2), 202–222.
- Marshall, A. (1920). Principles of Economics. London: Macmillan.
- Maskell, P. (2001). “Towards a Knowledge‐Based Theory of the Geographical Cluster,” Industrial and Corporate Change 10(4), 921–943.
- Maskell, P., and A. Malmberg (2007). “Myopia, Knowledge Development and Cluster Evolution,” Journal of Economic Geography 7(5), 603–618.
- Mccann, B. T., and T. B. Folta (2008). “Location Matters: Where We Have Been and Where We Might Go in Agglomeration Research,” Journal of Management 34(3), 532–565.
- Mcdermott, H. K., and S. Walsh (2001). “A Framework for Technology Management in the Service Sector,” IEEE Transactions 48(3), 333–341.
- Meeus, M., L. A. Oerleman, and J. Hage (2001). “Patterns of Interactive Learning in a High Tech Region,” Organization Studies 22(1), 145–172.
- Molina‐morales, F., and M. T. Martinez‐fernandez (2006). “Industrial Districts: Something More than a Neighbourhood,” Entrepreneurship & Regional Development 18(6), 503–524.
- Molina‐morales, F., and M. T. Martinez‐fernandez. (2010). “Social Networks: Effects of Social Capital on Firm Innovation,” Journal of Small Business Management 48(2), 258–279.
- Moran, P., and C. Galunic (1998). “Harnessing Social Capital for Productive Resource Exchange,” Academy of Management Conference Proceedings. Briarcliff Manor, NY: Academy of Management.
- Morgan, K. (2004). “The Exaggerated Death of Geography: Learning, Proximity and Territorial Innovation Systems,” Journal of Economic Geography 4(1), 3–21.
- Mowery, D. C., J. E. Oxley, and B. S. Silverman (1996). “Strategic Alliances and Interfirm Knowledge Transfer,” Strategic Management Journal 17, Special Issue, 77–91.
- Munari, F., M. Sobrero, and A. Malipiero (2012). “Absorptive Capacity and Localized Spillovers: Focal Firms as Technological Gatekeepers in Industrial Districts,” Industrial and Corporate Change 21(2), 429–462.
- Nahapiet, J., and S. Ghoshal (1998). “Social Capital, Intellectual Capital, and the Organizational Advantage,” Academy of Management Review 23(2), 242–266.
- Nooteboom, B., W. Van Haverbeke, G. Duysters, V. Gilsing, and A. Van Den oord (2007). “Optimal Cognitive Distance and Absorptive Capacity,” Research Policy 36(7), 1016–1034.
- Nunnally, J. C. (1978). Psychometric Theory. New York: McGraw‐Hill.
- Oinas, P. (1999). “Distance and Learning: Does Proximity Matter?,” in Learning Regions, Theory, Policy and Practice. Eds. S. Bakkers, R. P. J. H. Rutten, K. Morgan, F. Boekema. Aldershot, UK: Edward Elgar, 57–69.
- Owen‐smith, J., and W. W. Powell (2004). “Knowledge Networks as Channels and Conduits: The Effects of Spillovers in the Boston Biotechnology Community,” Organization Science 15(1), 5–21.
- Park, S. H., R. Chen, and S. Gallagher (2002). “Firm Resources as Moderators of the Relationship between Market Growth and Strategic Alliances in Semiconductor Start‐Ups,” The Academy of Management Journal 45, 527–545.
- Parra‐requena, G., F. X. Molina morales, and P. García villaverde (2010). “The Mediating Effect of Cognitive Social Capital on Knowledge Acquisition in Clustered Firms,” Growth and Change: A Journal of Urban and Regional Policy 41(1), 59–84.
- Piore, M. J., and C. F. Sabel (1984). The Second Industrial Divide. New York: Basic Books.
- Pirolo, L., and M. Presutti. (2010). “The Impact of Social Capital on the Start‐Ups' Performance Growth,” Journal of Small Business Management 48 (2), 197–227.
- Porter, M. E. (1994). “The Role of Location in Competition,” Journal of the Economics of Business 1(1), 35–40.
- Pouder, R., and C. St. john (1996). “Hot Spots and Blind Spots: Geographic Clusters of Firms and Innovation,” Academy of Management Review 21, 1192–1225.
- Presutti, M., C. Boari, and L. Fratocchi (2007). “Knowledge Acquisition and the Foreign Development of High‐Tech Start‐Ups: A Social Capital Approach,” International Business Review 16(1), 23–46.
- Presutti, M., C. Boari, and L. Fratocchi. (2016). “The Evolution of Inter‐Organisational Social Capital with Foreign Customers: Its Direct and Interactive Effects on SMEs' Foreign Performance,” Journal of World Business 51(2016), 760–773.
- Presutti, M., C. Boari, and A. Majocchi (2011). “The Importance of Proximity for the Start‐Ups' Knowledge Acquisition and Exploitation,” Journal of Small Business Management 49(3), 361–389.
- Presutti, M., C. Boari, and A. Majocchi. (2013). “Inter‐Organizational Geographical Proximity and Local Start‐Ups' Knowledge Acquisition: A Contingency Approach,” Entrepreneurship & Regional Development: An International Journal 25(5‐6), 446–467.
- Rallet, A., and A. Torre (2000). “Is Geographical Proximity Necessary in the Innovation Networks in the Era of Global Economy?,” Geojournal 49(4), 373–380.
- Romanelli, E., and O. M. Khessina (2005). “Regional Industrial Identity: Cluster Configurations and Economic Development,” Organization Science 16(4), 344–358.
- Sammarra, A., and L. Biggiero (2008). “Heterogeneity and Specificity of Inter‐Firm Knowledge Flows in Innovation Networks,” Journal of Management Studies 45(4), 800–829.
- Saxenian, A. (1994). Regional Advantage: Culture and Competition in Silicon Valley and Route 128. Cambridge, MA: Harvard University Press.
- Scott, A. J. (2006). “Entrepreneurship, Innovation and Industrial Development: Geography and the Creative Field Revisited,” Small Business Economics 26, 1–24.
- Shipilov, A. (2009). “Firm Scope Experience, Historic Multimarket Contact with Partners, Centrality and the Relationship between Network Position and Performance,” Organization Science 20(1), 85–106.
- Slotte‐kock, S., and N. Coviello (2010). “Entrepreneurship Research on Network Processes: A Review and Ways Forward,” Entrepreneurship Theory and Practice 34, 31–57.
- Smith, K., and Q. Cao (2007). “An Entrepreneurial Perspective on the Firm‐Environment Relationship,” Strategic Entrepreneurship Journal 1, 316–329.
- Sorenson, O., J. W. Rivkin, and L. Fleming (2006). “Complexity, Networks and Knowledge Flow,” Research Policy 35(7), 994–1017.
- Squire, B., P. D. Cousins, and S. Brown (2006). “Cooperation and Knowledge Transfer within Buyer–Supplier Relationships: The Moderating Properties of Trust, Relationship Duration and Supplier Performance,” British Journal of Management 20(4), 461–477.
- Stuart, T., and O. Sorenson (2003). “The Geography of Opportunity: Spatial Heterogeneity in Founding Rates and the Performance of Biotechnology Firms,” Research Policy 32(2), 229–253.
- Sundbo, J. (2001). The Strategic Management of Innovation. Cheltenham, UK: Edward Elgar.
- Ter wal, A. L. J., and R. A. Boschma (2009). “Applying Social Network Analysis in Economic Geography: Framing Some Key Analytic Issues,” Annals of Regional Science 43(3), 739–756.
- Ter wal, A. L. J., and R. A. Boschma. (2011). “Co‐Evolution of Firms, Industries and Networks in Space,” Regional Studies 45, 919–933.
- Tether, B., and A. Tajar (2008). “Beyond Industry–University Links: Sourcing Knowledge for Innovation from Consultants, Private Research Organizations and the Public Science‐Base,” Research Policy 37(9), 1079–1095.
- Torre, A. (2008). “On the Role Played by Temporary Geographical Proximity in Knowledge Transmission,” Regional Studies 42(6), 869–889.
- Tsai, W. P., and S. Ghoshal (1998). “Social Capital and Value Creation: The Role of Intrafirm Networks,” Academy of Management Journal 41(4), 464–476.
- Uzzi, B. (1997). “Social Structure and Competition in Interfirm Networks: The Paradox of Embeddedness,” Administrative Science Quarterly 42(1), 35–67.
- Valdaliso, J., A. Elola, M. Aranguren, and S. Lopez (2011). “Social Capital, Internationalization and Absorptive Capacity: The Electronics and ICT Cluster of the Basque Country,” Entrepreneurship and Regional Development: An International Journal 23(9–10), 707–773.
- Verganti, R. (2011a). “Radical Design and Technology Epiphanies: A New Focus for Research on Design Management,” Journal of Product Innovation Management 28(2), 384–388.
- Verganti, R.. (2011b). “Designing Breakthrough Products,” Harvard Business Review 89(10), 114–120.
- Von hippel, E. (1977). “Has a Customer Already Developed Your Next Product?,” Sloan Management Review 18(2), 73–74.
- Waxell, A., and A. Malmberg (2007). “What Is Global and What Is Local in Knowledge‐Generating Interaction? The Case of the Biotech Cluster in Uppsala, Sweden,” Entrepreneurship and Regional Development: An International Journal 19(2), 137–159.
- Woolley, J. L., and R. N. Rottner (2008). “Innovation Policy and Nanotechnology Entrepreneurship,” Entrepreneurship Theory and Practice 32, 791–811.
- Wu, W. P. (2008). “Dimensions of Social Capital and Firm Competitiveness Improvement: The Mediating Role of Information Sharing,” Journal of Management Studies 45(1), 122–146.
- Yanez, M., T. M. Khalil, and S. T. Walsh (2010). “IAMOT and Education: Defining a Technology and Innovation Management (TIM) Body‐of‐Knowledge (BoK) for Graduate Education (TIM BoK),” Technovation 30(7–8), 389–400.
- Yli‐renko, H., E. Autio, and H. J. Sapienza (2001). “Social Capital, Knowledge Acquisition, and Knowledge Exploitation in Young Technology‐Based Firms,” Strategic Management Journal 22(6), 7, 587–613.
- Zaheer, A., and G. G. Bell (2005). “Benefiting from Network Position: Firm Capabilities, Structural Holes, and Performance,” Strategic Management Journal 26(9), 809–825.
- Zahra, S. A., and G. George (2002). “Absorptive Capacity: A Review, Reconceptualization, and Extension,” Academy of Management Review 27(2), 185–203.
- Zahra, S., D. Jennings, and D. Kuratko (1999). “The Antecedents and Consequences of Firm‐Level Entrepreneurship: The State of the Field,” Entrepreneurship: Theory and Practice 24(2), 45–65.