Abstract
The ever-increasing rate of drug discovery data has complicated data analysis and potentially compromised data quality due to factors such as data handling errors. Parallel to this concern is the rise in blatant scientific misconduct. Combined, these problems highlight the importance of developing a method that can be used to systematically assess data quality. Benford's law has been used to discover data manipulation and data fabrication in various fields. In the authors' previous studies, it was demonstrated that the distribution of the corresponding activity and solubility data followed Benford's law distribution. It was also shown that too intense a selection of training data sets of regression model can disrupt Benford's law. Here, the authors present the application of Benford's law to a wider range of drug discovery data such as microarray and sequence data. They also suggest that Benford's law could also be applied to model building and reliability for structure-activity relationship study. Finally, the authors propose a protocol based on Benford's law which will provide researchers with an efficient method for data quality assessment. However, multifaceted quality control such as combinatorial use with data visualization may also be needed to further improve its reliability.
1. Introduction
With the recent unprecedented rates of increase in drug discovery data, so too has the complexity of analysis increased. Indeed, output from next-generation sequencing has grown from 10 MB/day to 40 GB/day on a single sequencer Citation[1]. Analysis of such data often requires internal and customized programs, and occasional bugs in these programs risk compromising the accuracy of the analysis. Professional misconduct constitutes another emerging serious problem in the scientific community; R. G. Steen concluded that 742 English language research papers were retracted from the PubMed database between 2000 and 2010 due to scientific misconduct and that the rate of such retractions is rising Citation[2].
The above problems highlight the importance of developing an effective method of systematically assessing data quality (data quality is defined as suitability for data analysis in this article), and a number of methods have already been proposed to counteract data manipulation, particularly with regard to the identification of possible data fabrication in the field of drug discovery and development Citation[3,4]. For example, Al-Marzouki et al. proposed the use of a statistical method that compares baseline data from one study with those from another Citation[5]. We previously proposed a novel method based on Benford's law Citation[6] and now provide an overview of how Benford's law can be applied in data quality assessment.
2. Benford's law and its application
In 1881, Simon Newcomb discovered a novel statistical principle later named Benford's law Citation[7]. Newcomb showed that the probability of the leading digit of any value is d1 and can be expressed as:
According to this formula, the probability that the first digit will be “1” is approximately 30%, not the 10% one would expect if all digits were equally likely (). In 1938, this finding was rediscovered and published by Frank Benford with 20 examples, including the areas of rivers, baseball statistics, and numbers in magazine articles Citation[8]. In 1996, Ted Hill constructed a rigorous mathematical proof that finally gave Benford's law the credibility it needed Citation[9].
Figure 1. Benford's law distribution and the χ2 statistic value. The X and Y axes indicate the first digit value and frequency, respectively. Diamonds and lines in (A) – (C) indicate Benford's law distribution. Squares in (B) and triangles in (C) indicate the first digit frequencies of two different sample data sets. The data set in (B) follows Benford's law distribution (χ2 = 0.02), whereas that in (C) does not (χ2 = 0.54).
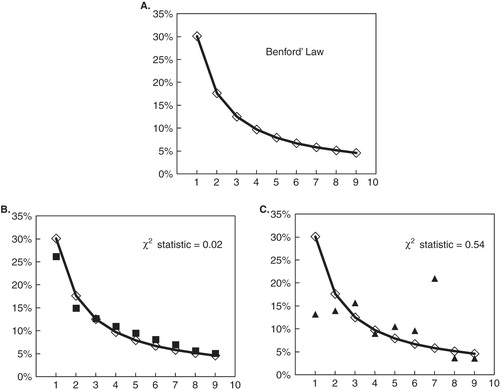
Of particular note is the fact that manipulated or artificially created numbers usually do not follow Benford's law, due in part to a misunderstanding of real data distributions Citation[10]. Such misunderstanding allows Benford's law to be used to discover data manipulation and fraud in various fields Citation[6]. Similarly, Benford's law can also be used to check data quality. For example, Brown et al. used either or both Benford's law and Zipf's law, often considered to be a generalized form of Benford's law, to check the analytical data of pollutant concentrations in ambient air Citation[11,12].
To determine whether or not an observed frequency distribution follows Benford's law's distribution, the χ2 statistic is used as follows Citation[13]:
where O is the observed frequency and E is the expected frequency (Benford's law distribution). When χ2 = 0, the observed distribution is judged to obey Benford's law completely ().
3. Application of Benford's law in drug discovery
Benford's law can be applied to drug discovery data in a number of fields. Hoyle et al. examined whether or not the distribution of mRNA transcription data from a large number of organisms obeys Benford's law Citation[13], and in our previous study, we found that the distribution of the corresponding activity and solubility data also obey Benford's law and that too intense a selection of training sets for the apparent predictive accuracy of the model can disrupt this rule Citation[6]. Further, several data sets available from ChEMBL (www.ebi.ac.uk/chembl) and NCBI (www.ncbi.nlm.nih.gov) have been found to follow Benford's law distribution (, dagger marks, double dagger marks, and section marks), indicating the robustness of Benford's law to drug discovery.
Table 1. Data sets in drug discovery and agreement with Benford's law.
While most natural data do indeed obey Benford's law, several conditions must be met to avoid false positives/negatives. In general, Benford's law can only be applied to data that are distributed across multiple orders of magnitude. However, data sets with limited data points tend not to follow Benford's law distribution. For example, although a PysProp solubility data set of 5,574 data points follows Benford's law distribution, 1% of randomly selected subsets do not Citation[6]. Thus, before applying Benford's law to assess data quality, the number of data points being considered must be confirmed to be at least 100.
4. Importance of quality assessment of chemogenomics databases
Prediction models for specific drug targets are frequently built using chemogenomics databases. However, because the accuracy of such models partially depends on the quality of the training data, quality assessment is essential to ensuring model accuracy. The chemogenomics database GVK BIO Online Structure Activity Relationship Database (GOSTAR; www.gvkbio.com), the largest manually curated SAR database and which contains all published and patented inhibitors against most biological targets and their associated SAR data, is as an example of a training data source.
To assess the quality of each data set in GOSTAR, we examined whether or not data sets follow Benford's law distribution (). We found that data sets for 145 of 352 molecular targets including over 1,000 activity data points (41.2%) did not follow Benford's law distribution (χ2 > 0.1). In one extreme case, the data set for corticotropin-releasing hormone receptor 1 (6,547 data points) failed Benford's distribution (χ2 = 1.26), as 71.6% of the activity values (4,687 data points) were the same (10 µM). This kind of situation is mainly observed in patents that include rough descriptions of assay data values. In contrast, in the same set, the values for acetylcholinesterase (5,363 data points) followed Benford's distribution (χ2 = 0.006). Taken together, these results suggest that Benford's law can be used to assess the quality of published data sets among chemogenomics databases as well as individual publications and patents.
5. Expert opinion
In addition to the applications described above, Benford's law may also be useful in assessing the reliability of quantitative structure-activity relationship/quantitative structure-property relationship (QSAR/QSPR) studies. As already mentioned, intense selection of training sets to obtain the apparent predictive performance of QSAR/QSPR models tends to disrupt the applicability of Benford's law Citation[6]. Benford's law is therefore a good indicator of whether or not training sets for a given QSAR/QSPR model have been randomly selected. We strongly suggest that training sets should be examined before using any QSAR/QSPR model.
A schematic flow diagram of our proposed protocol for data quality assessment using Benford's law with the χ2 statistic is shown in . We investigated whether or not the data of interest follow Benford's law distribution under the assumption that the data range over 2 orders of magnitudes with > 100 data points. If data did not follow Benford's law's distribution (χ2 > 0.1), we next considered the possibility that the data could have been compromised by factors such as data handling error, program bugs, or data fabrication. The ease of execution and understanding inherent with our proposed protocol marks it as useful for assessing data quality in drug discovery research.
Figure 3. Schematic flow diagram of our data quality checking protocol using Benford's law and the χ2 statistic.
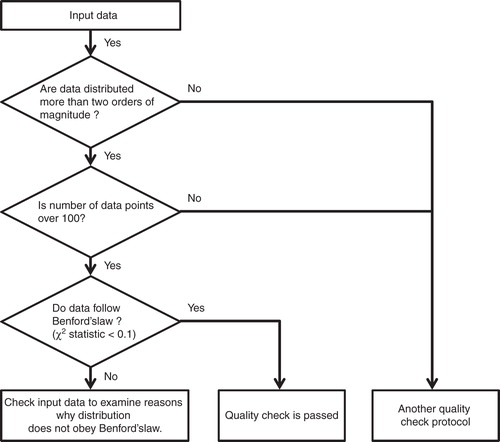
However, despite our promising findings, several limitations to our proposed protocol warrant mention. First, as mentioned above, our proposed protocol cannot be applied to data sets that either or both range within a single order of magnitude or include only a few dozen data points. Second, when inhibition activity (% inhibition) obeys Benford's law, activity remaining (100% inhibition) does not obey Benford's law. Third, our proposed protocol cannot detect data fabrication in data sets that are artificially created to follow Benford's law distribution, necessitating the use of other data quality assessment methods to complement ours. Most quality checking methods can be classified into two types: checking mechanically based on statistic, or empirically evaluating the data. Given that our proposed method falls into the former category, a complementary method should consider a different statistic rule. With empirical evaluation, visualization of data is an important factor, and empirically, frequent data visualization can aid in detecting data abnormalities; it is often avoided due to its time-consuming aspect. Fortunately, several user-friendly data visualization tools have been developed for use in the drug discovery field Citation[14]. The combination of data visualization and our proposed protocol may prove sufficient for assessing data analysis quality.
In conclusion, with the rise in quantity and complexity of drug data, data quality is becoming an increasingly important matter. Our proposed protocol based on Benford's law, which has been used to detect data fabrication in various fields, offers an efficient method for such assessment, albeit not without its limitations. Multifaceted quality control such as combinatorial use with data visualization may therefore be needed to improve its reliability.
Declaration of interest
The authors are all employees of Astellas Pharma, Inc.
Acknowledgements
The authors thank T Niimi, M Oku, K Mori, and H Fuji, and N Katayama for carefully reviewing the manuscript.
Bibliography
- Kahn SD. On the future of genomic data. Science 2011;331:728-9
- Steen RG. Retractions in the scientific literature: is the incidence of research fraud increasing? J Med Ethics 2011;37:249-53
- Buyse M, George SL, Evans S, The role of biostatistics in the prevention, detection and treatment of fraud in clinical trials. Stat Med 1999;18:3435-51
- Taylor R, McEntegart D, Stillman E. Statistical techniques to detect fraud and other data irregularities in clinical questionnaire data. Drug Inform J 2002;36:115-25
- Al-Marzouki S, Evans S, Marshall T, Are these data real? Statistical methods for the detection of data fabrication in clinical trials. BMJ 2005;331:267-70
- Orita M, Moritomo A, Niimi T, Use of Benford's law in drug discovery data. Drug Discov Today 2010;15:328-31
- Newcomb S. Note on the frequency of use of dierent digits in natural numbers. Amer J Math 1881;4:39-40
- Benford F. The law of anomalous numbers. Proc Am Philos Soc 1938;78:551-72
- Hill T. A statistical derivation of the significant-digit law. Stat Sci 1996;10:354-63
- Hill T. The difficulty of faking data. Chance 1999;12:27-31
- Brown RJC. The use of Zipf's law in the screening of analytical data: a step beyond Benford. Analyst 2007;132:344-9
- Brown RJC. Benford's law and the screening of analytical data: the case of pollutant concentrations in ambient air. Analyst 2005;130:1280-5
- Hoyle DC, Rattray M, Jupp R, Making sense of microarray data distributions. Bioinformatics 2002;18:576-84
- Howe JT, Mahieu G, Marichal P, Data reduction and representation in drug discovery. Drug Discov Today 2007;12:45–5