The ongoing epidemic of obesity among children and adults worldwide has heightened the need for tools to identify obesity-related risk [Citation1]. The metabolic syndrome (MetS) is a cluster of cardiovascular risk factors associated with insulin resistance that appears to be produced by underlying processes of adipocyte dysfunction, systemic inflammation, and oxidative stress [Citation2,Citation3]. Traditional MetS criteria identify individuals who have abnormalities beyond population-based cutoffs in at least three components of MetS: elevated waist circumference (or in some cases for children, body mass index [Citation4]), high blood pressure, hypertriglyceridemia, low high-density lipoprotein ()-cholesterol, and elevated fasting plasma glucose [Citation5]. In both children and adults, individuals classified as having MetS by traditional criteria are more likely to develop future cardiovascular disease (CVD) [Citation6,Citation7] and Type 2 diabetes [Citation4,Citation7]. While MetS has been criticized for not providing added risk assessment beyond the sum of its individual components [Citation8], this identification of risk for future CVD and Type 2 diabetes mellitus (T2DM) raises the potential for MetS to be a tool to screen for those with elevated risk and target appropriate treatments.
Nevertheless, there are aspects of traditional MetS assessment that limit its use clinically. (1) Traditional MetS criteria represent a binary test that may miss detection of individuals with abnormalities in multiple MetS components just shy of the cutoffs. (2) Current MetS criteria assume that each individual component has equal weight toward identifying a patient’s MetS status. (3) After an individual is classified as having MetS, traditional MetS criteria are unable to follow worsening or detect improvement of that person’s metabolic risk over time. (4) MetS appears to manifest differently between males and females and among different races/ethnicities. Specifically, the cutoff values of traditional MetS criteria do not appear to adequately identify risk among African–American individuals, who have low prevalence of MetS classification despite having high prevalence of CVD and T2DM [Citation9]. (5) Current MetS criteria do not function as well in identifying elevated fasting insulin [Citation10] or high-sensitivity C reactive protein (hsCRP) [Citation11] among African-Americans, largely because of differences in the association between insulin resistance and lipid abnormalities in African-Americans, who are less likely to exhibit elevations in triglycerides and more likely to have elevations in MetS-associated factors such as Lp(a) [Citation9,Citation12].
Because of these limitations of traditional MetS criteria, we have derived and have begun to validate MetS severity Z-scores for adolescents [Citation13] and adults [Citation14] to be used in both risk identification and in following for changes in metabolic status over time. These scores are sex-specific and race-ethnicity-specific and weighted to reflect differences in how each of the components of MetS reflects the underlying pathophysiologic processes. The derivation of these scores utilized confirmatory factor analysis of cross-sectional data from the National Health and Nutrition Examination Survey (NHANES) on 4,174 adolescents aged 12–19 years and 6,870 adults aged 20–64 years [Citation13,Citation14]. Confirmatory factor analysis evaluates how a set of variables interact together to contribute to a behind-the-scenes latent ‘factor.’ Because a set of variables (in this case, the 5 traditional MetS components listed above) may differentially contribute to the latent factor (in this case, the ‘MetS factor’), the technique can be used to assign weights of each of these components according to their strength of association with MetS. Despite this weighting, the technique does not incorporate the potential for some features to exist together more commonly than others (such as high triglycerides and low cholesterol) which is a potential limitation. A further limitation is the inclusion of only serum levels of factors without qualitative assessment of components such as HDL-C.
We observed a statistical justification to perform these factor analysis separately by sex and racial/ethnic group (for white, African-American, and Hispanic participants), to create equations for six subgroups for both adolescents and adults to account for any differences in how MetS was manifest between groups. The equations incorporate the relative weight of the individual MetS components which is multiplied by a particular individual’s measured clinical value for that component (WC in cm, systolic BP in mmHG, fasting plasma glucose in mg/dL, etc.). These values are then added (with the exception of HDL-cholesterol, which is subtracted since higher values are more favorable) to yield a score. While this is clearly complex, the scores are easily calculable online (http://mets.health-outcomes-policy.ufl.edu/calculator/) and could be incorporated into an electronic medical record system. The scores are standardized to reflect Z-scores, with a mean set to 0 and a range from negative infinity to positive infinity based on the number of standard deviations from the mean, and can easily be translated to percentiles that allow for a representation of one’s MetS severity relative to the population.
The initial validation of the scores compared the score to other continuous variables that are related to MetS that were also measured in NHANES. Scores exhibited high correlations to fasting insulin, hsCRP, and uric acid for both children and adults and in each of the three racial/ethnic groups. This demonstrated that indeed higher severity scores corresponded to higher levels of these markers associated with insulin resistance, systemic inflammation, and oxidative stress.
The more important validation evaluated the scores as a clinical tool for predicting future disease. For this, we relied on long-term data from individuals evaluated as school children mean age 13 years in the Princeton Lipid Research Clinic (LRC) study (1973–1976), who were then followed as adults mean age 38 years in the Princeton Follow-up Study (PFS) (1998–2003) and again at mean age 50 years in the Princeton Health Update (2010–2014) [Citation15,Citation16]. We found a reasonably high degree of correlation in scores between childhood and adulthood within the same individual (Pearson’s r = 0.41, p < 0.01), confirming prior studies of the persistence of metabolic phenotypes [Citation17]. We then evaluated childhood MetS severity scores for predicting adult disease, finding that individuals who went on to develop either CVD or T2DM by mid-adulthood had higher MetS Z-scores in childhood than either those who developed disease by later adulthood or those who were still healthy as adults () [Citation15,Citation16]. Indeed, for every standard deviation increase in MetS severity score in childhood, the odds of future CVD increased nearly 10-fold, and nearly three-fold for future T2DM by age 38 [Citation15,Citation16]. For each standard deviation increase in adult score at mean age 38, there was an increase in odds of 7.0 for future CVD and 5.2 for future T2DM by age 50. These data demonstrated the potential for use of the MetS severity score in identifying risk in adolescents and adults. But interestingly, when we looked at the change in score between LRC and PFS, each one standard deviation increase in score conferred an additional increase in odds of 3.4 for future CVD and 7.3 for future T2DM [Citation15,Citation16]. This highlights the potential to use this score to follow an individual for ominous increases in MetS severity score – and increased disease risk – over time [Citation18].
Figure 1. MetS Z score in childhood and adulthood by incident disease diagnosis. MetS Z-score values during childhood (1973-1976, mean age 13 years) and mid-adulthood (1998-2003, mean age 38 years) among individuals who developed cardiovascular disease (CVD)(A) or diabetes (B) by mid-adulthood, by later adulthood (2010-2014, mean age 50 years) or not at all. From references [Citation15] and [Citation16], used by permission. Comparison with disease-free group: * p<0.05, ** p<0.01. Comparison with incident disease between mid-adulthood and later adulthood: # p<0.05, ## p<0.01.
![Figure 1. MetS Z score in childhood and adulthood by incident disease diagnosis. MetS Z-score values during childhood (1973-1976, mean age 13 years) and mid-adulthood (1998-2003, mean age 38 years) among individuals who developed cardiovascular disease (CVD)(A) or diabetes (B) by mid-adulthood, by later adulthood (2010-2014, mean age 50 years) or not at all. From references [Citation15] and [Citation16], used by permission. Comparison with disease-free group: * p<0.05, ** p<0.01. Comparison with incident disease between mid-adulthood and later adulthood: # p<0.05, ## p<0.01.](/cms/asset/ca9a007c-6f36-4aae-91d7-30c43d03e1b8/ierk_a_1143360_f0001_oc.jpg)
The equations to generate these scores thus represent a means of estimating MetS severity in a way that corresponds to long-term disease risk. This brings up several clinical applications. (1) The MetS Z-score contributes a disease severity component to the traditional binary MetS assessment. In clinician–patient communication, this adds a quantitative component that could enhance the understanding of the degree of metabolic derangement and associated future disease risk [Citation19]. (2) The MetS Z-score also allows for the tracking of MetS severity over time. This allows for the assessment of therapy effectiveness in treating MetS. It also allows for both the clinician and patient to monitor MetS progress and changes in future disease risk over time. (3) Given the potential for patients to better understand their own health risks and progress over time, the MetS Z-score could also be used as a motivating factor for encouraging patients to attempt and adhere to various therapeutic interventions.
The MetS Z-score also presents many opportunities for future research. The MetS Z-score was initially designed to only assess metabolic disease severity itself. Validation studies have shown that the MetS Z-score is related to future T2DM and CVD risk and is associated with the presence of other metabolic abnormalities such as elevated fasting insulin and hsCRP. Future studies could be directed at how the MetS Z-score correlates to other future disease risk (such as stroke and chronic kidney disease), assessments of whether MetS Z-score provides additional risk prediction beyond that of the individual components, and its association with additional subclinical disease markers. Additionally, the MetS Z-score can be used in epidemiological studies. The scores were recently used to track MetS trends in a nationally representative sample of US adolescents participating in NHANES, revealing a temporally decreasing trend in MetS severity [Citation20]. Such findings could have significant impact on public health efforts. Lastly, the MetS Z-score could be used as an outcome measurement in clinical research trials.
Looking ahead five years, we see a need for further comparison of the MetS Z-score with other established scores such as the Framingham risk score, Reynolds Risk Score, and QRISK. Ultimately, the MetS Z-score may function as one component of risk that is best complimented with additional risk factors – from simple ones such as tobacco use to more complex ones that incorporate other serum CVD risk factors or lifestyle factors. In these coming years, we anticipate that the MetS Z-score will be more heavily utilized in both research and clinical settings. The MetS Z-score could be incorporated into electronic medical records, increasing physician awareness of this tool and allowing for chart review studies. More validation studies are being performed to determine specific MetS Z-score cutoffs for clinician use, indicating when to start interventions, when there is a significant risk for developing T2DM or CVD, or when a patient warrants additional follow-up studies. Ultimately, studies are needed to see if use of this score in clinical application results in patient motivation to the point of lifestyle change. In the big picture, the MetS Z-score represents a new perspective in understanding MetS that could be beneficial to researchers, clinicians, and patients.
Financial & competing interests disclosure
The authors have no relevant affiliations or financial involvement with any organization or entity with a financial interest in or financial conflict with the subject matter or materials discussed in the manuscript. This includes employment, consultancies, honoraria, stock ownership or options, expert testimony, grants or patents received or pending, or royalties.
Additional information
Notes on contributors
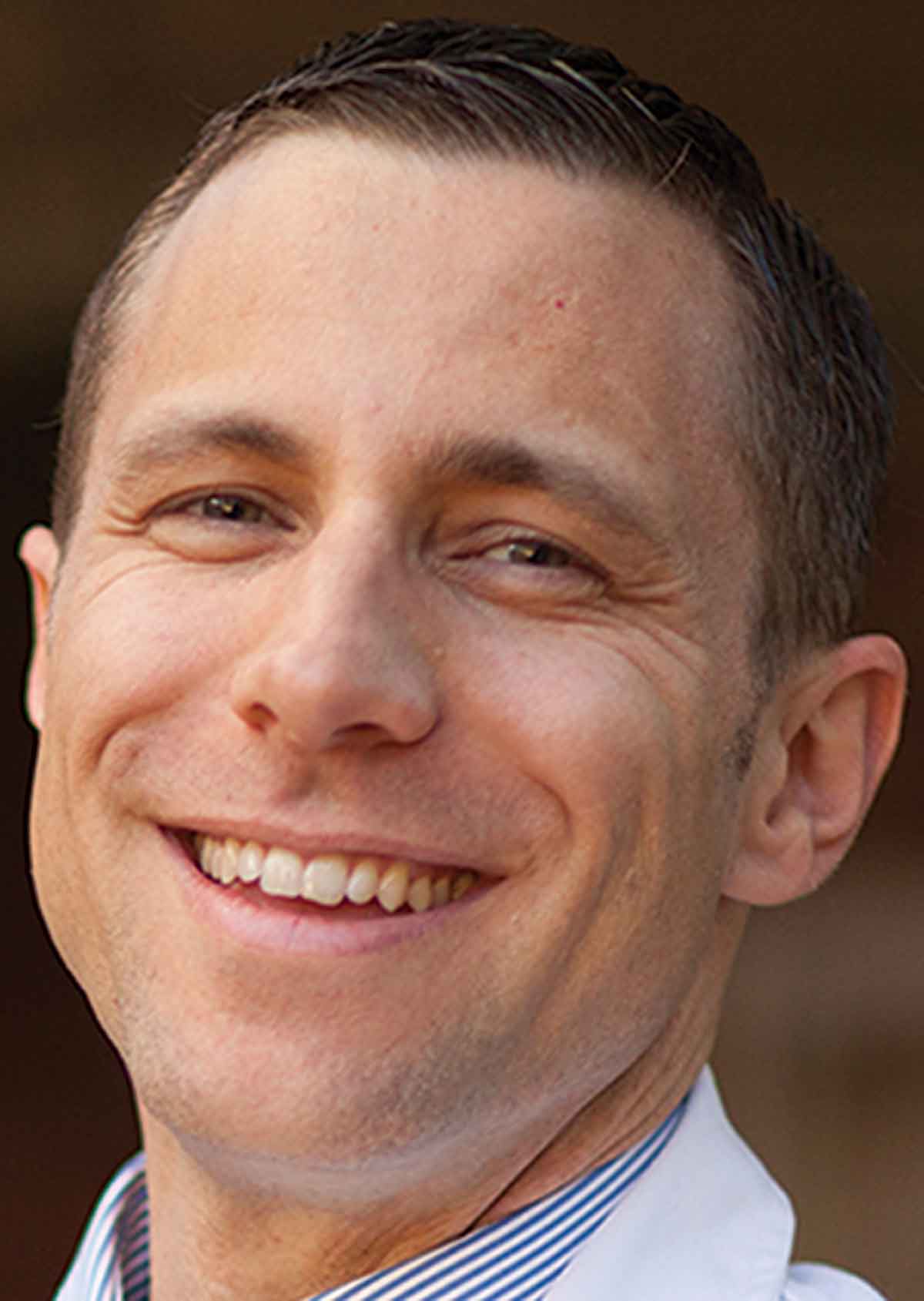
Arthur M. Lee

Matthew J. Gurka

Mark D. DeBoer
References
- DeBoer MD. Obesity, systemic inflammation, and increased risk for cardiovascular disease and diabetes among adolescents: a need for screening tools to target interventions. Nutrition. 2013;29(2):379–386.
- de Ferranti S, Mozaffarian D. The perfect storm: obesity, adipocyte dysfunction, and metabolic consequences. Clin Chem. 2008;54(6):945–955.
- DeBoer MD, Gurka MJ. Ability among adolescents for the metabolic syndrome to predict elevations in factors associated with type 2 diabetes and cardiovascular disease: data from the national health and nutrition examination survey 1999–2006. Metab Syndr Relat Disord. 2010;8(4):343–353.
- Morrison JA, Friedman LA, Wang P, et al. Metabolic syndrome in childhood predicts adult metabolic syndrome and type 2 diabetes mellitus 25 to 30 years later. J Pediatr. 2008;152(2):201–206.
- Grundy SM, Cleeman JI, Daniels SR, et al. Diagnosis and management of the metabolic syndrome – an American Heart Association/National Heart, Lung, and Blood Institute Scientific Statement. Circulation. 2005;112(17):2735–2752.
- Morrison JA, Friedman LA, Gray-McGuire C. Metabolic syndrome in childhood predicts adult cardiovascular disease 25 years later: the Princeton Lipid Research Clinics follow-up study. Pediatrics. 2007;120(2):340–345.
- Wannamethee SG, Shaper AG, Lennon L, et al. Metabolic syndrome vs Framingham Risk Score for prediction of coronary heart disease, stroke, and type 2 diabetes mellitus. Arch Intern Med. 2005;165(22):2644–2650.
- Kahn R, Buse J, Ferrannini E, et al. The metabolic syndrome: time for a critical appraisal: joint statement from the American Diabetes Association and the European Association for the Study of Diabetes. Diabetes Care. 2005;28(9):2289–2304.
- Walker SE, Gurka MJ, Oliver MN, et al. Racial/ethnic discrepancies in the metabolic syndrome begin in childhood and persist after adjustment for environmental factors. Nutr Metabol Cardiovasc Dis. 2012;22(2):141–148.
- DeBoer MD, Dong L, Gurka MJ. Racial/ethnic and sex differences in the ability of metabolic syndrome criteria to predict elevations in fasting insulin levels in adolescents. J Pediatr. 2011;159(6):975–981.e3.
- DeBoer MD, Gurka MJ, Sumner AE. Diagnosis of the metabolic syndrome is associated with disproportionately high levels of high-sensitivity C-reactive protein in non-Hispanic black adolescents: an analysis of NHANES 1999–2008. Diabetes Care. 2011;34(3):734–740.
- Sumner AE, Cowie CC. Ethnic differences in the ability of triglyceride levels to identify insulin resistance. Atherosclerosis. 2008;196(2):696–703.
- Gurka MJ, Ice CL, Sun SS, et al. A confirmatory factor analysis of the metabolic syndrome in adolescents: an examination of sex and racial/ethnic differences. Cardiovasc Diabetol. 2012;11:128.
- Gurka MJ, Lilly CL, Oliver MN, et al. An examination of sex and racial/ethnic differences in the metabolic syndrome among adults: a confirmatory factor analysis and a resulting continuous severity score. Metabolism. 2014;63(2):218–225.
- DeBoer MD, Gurka MJ, Woo JG, et al. Severity of metabolic syndrome as a predictor of cardiovascular disease between childhood and adulthood: the Princeton Lipid Research Cohort Study. J Am Coll Cardiol. 2015;66(6):755–757.
- DeBoer MD, Gurka MJ, Woo JG, et al. Severity of the metabolic syndrome as a predictor of type 2 diabetes between childhood and adulthood: the Princeton Lipid Research Cohort Study. Diabetologia. 2015;58(12):2745–2752.
- Li S, Chen W, Srinivasan SR, et al. Relation of childhood obesity/cardiometabolic phenotypes to adult cardiometabolic profile: the Bogalusa Heart Study. Am J Epidemiol. 2012;176 Suppl 7:S142–S149.
- Vishnu A, Gurka MJ, DeBoer MD. The severity of the metabolic syndrome increases over time within individuals, independent of baseline metabolic syndrome status and medication use: the Atherosclerosis Risk in Communities Study. Atherosclerosis. 2015;243(1):278–285.
- DeBoer MD. Ethnicity, obesity and the metabolic syndrome: implications on assessing risk and targeting intervention. Expert Rev Endocrinol Metab. 2011;6(2):279–289.
- Lee AM, Gurka MJ, DeBoer MD. Trends in metabolic syndrome severity and lifestyle factors among adolescents. Pediatrics. 2016. doi: 10.1542/peds.2015-3177. [Epub ahead of print]