Abstract
While proteomic methods have illuminated many areas of biological protein space, many fundamental questions remain with regard to systems-level relationships between mRNAs, proteins and cell behaviors. While mass spectrometric methods offer a panoramic picture of the relative expression and modification of large numbers of proteins, they are neither optimal for the analysis of predefined targets across large numbers of samples nor for assessing differences in proteins between individual cells or cell compartments. Conversely, traditional antibody-based methods are effective at sensitively analyzing small numbers of proteins across small numbers of conditions, and can be used to analyze relative differences in protein abundance and modification between cells and cell compartments. However, traditional antibody-based approaches are not optimal for analyzing large numbers of protein abundances and modifications across many samples. In this article, we will review recent advances in methodologies and philosophies behind several microarray-based, intermediate-level, ‘protein-omic’ methods, including a focus on reverse-phase lysate arrays and micro-western arrays, which have been helpful for bridging gaps between large- and small-scale protein analysis approaches and have provided insight into the roles that protein systems play in several biological processes.
(A) Each arrow indicates an experimental condition, which may represent the stimulation or inhibition of components of a biological system. Circles represent a protein modification measured. (B) Resulting data matrix from the experimental set up. Each column represents an experimental condition and the length of the column indicates the depth of measured protein data space.
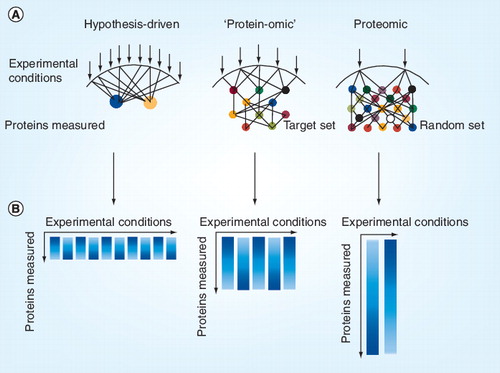
Four methodologies are indicated: (A) forward-phase arrays, where samples are labeled and applied to immobilized antibodies specific to certain proteins on nitrocellulose slides; (B) sandwich arrays, where captured proteins are detected by a second labeled antibody targeted to that protein; (C) reverse-phase lysate arrays, where complex mixtures of proteins are printed and immobilized onto slides and then specific proteins detected with a primary antibody; and (D) micro-western arrays, where samples are printed, electrophoresed to separate proteins based on size, then transferred to nitrocellulose, and then specific proteins detected with primary antibodies. A near-infrared dye-labeled secondary antibody can be used to generate fluorescent signal in (C & D) when used with the LI-COR Odyssey near-infrared scanner. Green shapes denote the targeted protein of interest while blue shapes denote proteins that nonspecifically cross-react with antibodies. The filled circles underneath each array represent the strength of each platform with respect to each criterion on the left.
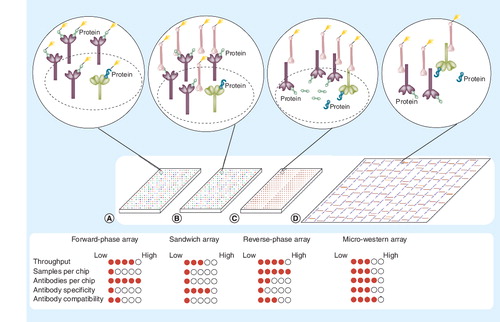
This article is intended to serve as an introduction to the philosophies and methodologies behind protein-omic approaches from our personal perspective. Throughout this article, the adjective ‘protein-omic’ will be applied to describe affinity-based methods used for the targeted analysis of tens to thousands of pre-defined protein targets. The philosophies behind protein-omic and proteomic methods are quite different and are suited to answer different sets of biological questions. The beginning of this article will be devoted to describing the philosophies and methodologies that were precursors of microarray-based protein-omic methods: namely the DNA microarray approach for analysis of mRNA expression and the western blotting method for analysis of proteins. We will then describe several early examples of protein microarray-based protein-omic approaches applied to the analysis of human cell signaling proteins and will then conclude by focusing on some of our recent advances in the development and application of micro-western arrays – a recent next iteration of the reverse-phase cell lysate array method – for interrogating two different questions in the fields of systems biochemistry and genomics: the architecture of EGF receptor (EGFR)-related signaling networks; and the relationship between heritable variation in the human genome and variation in protein expression and cellular behavior. We strongly believe that systems-level insight into many areas of biology will be accelerated by integrating protein-omic data with other systems-level datasets.
Advances in DNA sequencing technology – more specifically, the ability to sequence the whole genomes of organisms – ushered in the era of genomics. Knowledge of the sequences of genomes Citation[1,2], coupled with our ability to quantify changes in mRNA expression using DNA microarrays and other technologies Citation[3], were important early methodological drivers of the field of functional genomics. These methods enabled biologists to simultaneously monitor the relative expression levels of nearly all the genes in a genome. More recently, the further refinement of microarray methods and the development of more sophisticated sequencing methods Citation[4–6] have allowed for the identification Citation[7] and more precise quantification of the levels of lowly abundant mRNA and miRNA transcripts Citation[8] and identification of common and rare genetic polymorphisms. A distinguishing feature of next-generation sequencing and expression microarray methods versus most currently used protein analysis methods is their ability to simultaneously leverage the powers of multiplexing and throughput to obtain a relatively comprehensive understanding of mRNA expression space. Sequencing and microarray methods can be compared and contrasted with analytical chemistry-derived methods. While DNA microarrays and mass spectrometers can both analyze many genes or peptides from a single condition respectively, DNA microarrays capture a much larger proportion of total mRNA expression space than a mass spectrometer is able to capture for protein expression and modification space. In addition, while the number of DNA microarray experiments performed at any one time can be scaled up rather cheaply by using more microarrays, the number of experiments that can be performed by mass spectrometers cannot be easily scaled up without investing in many new instruments and trained personnel. In contrast to microarray approaches where targets can be preselected for studying a system of related genes or proteins, mass spectrometry approaches are not as easily amenable to the study of preselected protein targets. While one can more reproducibly target sets of peptides through the use of single- or multiple-reaction ion-monitoring approaches Citation[9], much more effort must be invested in determining the appropriate conditions for measuring peptides targeted with this approach than would have to be invested to analyze targeted gene sets with expression microarrays.
The ability to simultaneously analyze nearly all human genes distinguishes the field of genomics from proteomics. Fundamental and novel mechanistic insight is likely to result from the deep and comprehensive analysis of protein systems and their relationship to cell behaviors under many conditions.
The techniques and approaches used for monitoring mRNA expression can be traced back to the Southern blotting method Citation[10,11], which was initially used for measuring the size and identity of DNA fragments by applying a combination of gel electrophoresis and DNA hybridization methods. Adaptation of the Southern blotting method to the analysis of mRNA sizes and abundances was then described as the northern blotting method Citation[12–16]. The northern blotting method and the related ribonuclease protection assay were useful for the quantification of changes in mRNA abundance and splice site usage of small numbers of target genes following a small number of conditions Citation[17,18]. The method required a great deal of human intervention at multiple steps of the pipeline, including the application of each sample to wells of custom-made polyacrylamide gels by human hands. The method additionally required a great deal of effort at the stage of data analysis and interpretation. Arguably, it was not until the advent of DNA microarrays that the expression profiling approach was transitioned from answering small-scale, hypothesis-driven questions to providing discovery-driven insight regarding whole gene systems. The power to interrogate the expression changes of many genes simultaneously was then applied for addressing many fundamental biological questions, and represented a major leap forward in many fields of genetics and genomics. DNA microarrays and the related serial analysis of gene expression method were initially applied primarily by geneticists to complement other genetic approaches from model organisms Citation[3,19,20]. However, the philosophy and methodologies of proteomics have been developed and primarily applied by analytical chemists. Analytical biochemists, unlike geneticists, have historically been engaged in identification and quantification of system parts rather than in exploring the coordinated behavior of biological systems following large numbers of perturbations. While large-scale protein interaction maps have been defined with mass spectrometry approaches Citation[21,22], temporal quantitative analysis of those relationships is not straight-forward due to the challenges in quantitatively relating more than a handful of samples to each other with isotopic labeling methods.
Comprehensive analysis of protein systems across many conditions is not easily achieved by current proteomic methods
Functional genomic methods were able to extend their analytical reach to the simultaneous analysis of a nearly comprehensive set of all genes in an organism in a relatively short time frame. However, current proteomic approaches are still unable to reproducibly report on the abundance or modification of more than a fraction of total proteins in a population of cells across multiple conditions. For example, only 60% of 689 predicted open reading frames (ORFs) in Mycoplasma pneumoniaCitation[23], 66% of ORFs in yeast Citation[24], 54% of ORFs in Caenorhabditis elegansCitation[25] and 50% of ORFs in Arabidopsis thalianaCitation[26] have ever been detected by mass spectrometry projects aimed at identifying proteins in each organism. The inability to detect the full complement of predicted ORFs could be a result of the lack of expression of classes of proteins under the relatively small number of conditions examined in the studies. However, the observation that peptides from soluble, highly expressed proteins are typically over-represented versus lowly expressed transmembrane proteins Citation[27] and that non-mass spectrometry methods have previously detected many of these missed proteins Citation[28] suggests that current mass spectrometry methods reproducibly observe only a subset of sample peptides, which is biased towards more abundant proteins.
The difference in scope between genomic and proteomic approaches has been driven, in part, by the reality that the analysis of proteins is substantially more complex than for nucleic acids. First, complexity in protein isoforms, structure and function arises from the translation of mRNAs at multiple start sites; second, proteins are processed and modified at many sites in a manner that varies from protein to protein; and last, the physiochemical makeup of proteins and peptides is diverse, with major differences in polarity, charge and amenability to cleavage with a given set of proteases in a particular analytical pipeline.
A major attractive feature of mass spectrometry is that few or no affinity reagents are theoretically required to measure the abundance of a particular protein. Currently, no universal synthetic affinity reagent for the high-throughput analysis of all protein isoforms and modification states exists. Rather, a great deal of time and effort has to be expended to generate an affinity reagent to each protein isoform or modification of interest. The cheapest and quickest custom affinity reagents are typically polyclonal antibodies directed against small fragments of a protein. However, the total amount of affinity reagent generated with each immunization protocol is only sufficient for a relatively small number of protein analyses using conventional immunoblotting or similar approaches. After the reagent is consumed during use, a whole new pipeline of antibody generation and validation must then be undertaken to produce another new affinity reagent that may perform markedly differently to the last version with respect to antigen affinity and selectivity. Owing to these limitations, most large-scale protein analysis projects have relied heavily on mass spectrometric approaches. However, as DNA microarrays and antibody approaches can be likened to bullets specifically aimed at preselected targets, mass spectrometry can be likened to a shotgun: in each mass spectrometry experiment, a small subset of total targets is identified and quantified with a probability based on a complex function of variables including protein abundance, enrichment pipeline, the particular mass spectrometer and mode of operation used, and so on. For early discovery-driven efforts aimed at detecting new proteins and modifications, such an approach was ideal. For the analysis of biological systems, a more ideal approach would allow for the analysis of predefined target sets following large numbers of time points and perturbations.
Historically, researchers applying proteomic methods used either 2D gels to reduce the complexity of the starting pool of proteins based on size and isoelectric point Citation[29–31] or used multidimensional high-performance liquid chromatography (HPLC) Citation[32,33] to reduce the complexity of proteins based on hydrophobicity and charge and then used mass spectrometry to identify the bands in the gels Citation[34] or the fractions eluting from the HPLC column. The advent of isotopic labeling approaches for mass spectrometry Citation[35–40] enabled the more quantitative measurement of the relative abundances of proteins across samples. Currently, multiplexed versions of these isotopic labeling methods theoretically allow for the relative abundance of proteins to be assessed from up to eight conditions simultaneously. In practice, however, multiplexed isotopic labeling methods still require a great deal of expertise to avoid erroneous interpretation of the derived data Citation[41]. Requirement of isotopically pure labeling reagents also renders each experiment very expensive relative to the cost of standard immunoblotting experiments.
Even with the most sophisticated separation methods and instruments currently available, only a limited slice of total protein expression and modification space can be analyzed with any single combination of methods and instruments. If one enriches for a certain protein modification type, then one inevitably loses the ability to simultaneously quantify and relate the levels of proteins containing different modifications. In addition, following enrichment of one modification type, one loses the ability to compare the ratio of modified and unmodified forms of proteins. Without enrichment methods, even modern mass spectrometers will typically be unable to reproducibly observe proteins of low abundance or low modifications stoichiometry due to the high competing signals of peptides from more abundant cellular proteins. While sensitivity for identifying a particular protein or peptide can be greatly increased by training a mass spectrometer on a particular precursor or product ion mass at a particular HPLC elution time window, a great deal of effort must be invested to learn what peptides will elute at different times following different protocols and under what fragmentation conditions a particular peptide can be observed or sequenced.
In summary, functional genomics approaches have recently enabled a more comprehensive analysis of relative mRNA transcript abundances from a theoretically unlimited number of samples. Conversely, proteomic approaches are typically able to monitor a small subset of the total protein space across relatively few sample conditions.
In contrast to discovery-driven proteomic approaches, most mammalian cell and molecular biologists have typically utilized hypothesis-driven approaches that involve the analysis of small numbers (typically on the order of five or ten) of protein targets per experiment. As an example, the EGFR tyrosine kinase has been studied by many groups for more than 20 years in the context of cancer biology (for reviews, see Citation[42–45]). Molecular and cellular biologists studying the effects of small-molecule inhibitors on the EGFR system have typically monitored the changes in modification or abundance of EGFR, and several downstream effectors from canonical pathways such as the Src family, MAPK, AKT, mTOR and the STAT family proteins Citation[46,47]. Thus, cell and molecular biologists have and typically continue to study the coordinated activity of a handful of proteins at a time despite the knowledge based on previous discovery-driven mass spectrometry experiments indicating that thousands of cellular proteins undergo changes in abundance, phosphorylation and other forms of modification following EGF stimulation. Cell biologists have typically relied on small-scale protein analysis methods such as immunohistochemical staining, western blots and ELISAs following genetic or other types of perturbations to study the EGFR system. Nearly all of these methods rely in some way on the use of antibodies or other similar affinity reagents.
In comparison to mass spectrometric approaches, antibody-based methods are unable to quantify protein abundances or modifications until an affinity reagent has been generated for detecting it. If one desires the ability to study thousands of proteins with affinity-based methods, thousands of unique affinity reagents must first be created and then validated for use with many cell types. Similarly, if one desires the ability to observe site-specific modifications of thousands of proteins, then a unique affinity reagent must be generated for each particular modification.
Targeted protein-omic methods are bridging the gap between proteomics & hypothesis-driven protein analysis approaches
Between the two extremes of discovery-oriented mass spectrometry approaches and hypothesis-driven western blotting approaches, a new group of affinity-based approaches has been developed in the past 15 years that leverages many of the increases in throughput, multiplexing and automation provided by microarray and flow-sorting devices. This class of protein analysis methods has previously been described as focused proteomics Citation[48] or targeted systems analysis approaches. Given the current trajectory of evolution of the methodologies, approaches and philosophies for comprehensively studying ever-larger sets of gene families, we describe this new burgeoning class of approaches with the adjective ‘protein-omic’ . This description underscores the importance that such approaches place on reproducibly studying collections of predefined protein targets. Protein-omic approaches are ideal for building models of biological systems because they allow for related proteins to be interrogated under many conditions using many complementary approaches. The proteins under investigation using such approaches are typically preselected based on previous knowledge of the system at hand.
Evolution of microarray-based protein-omic methods
In the late 1990s and early 2000s, many independent groups began to leverage the multiplexing, automation and throughput advantages of the microarray (for in-depth review see Citation[49]) and flow-sorting methodologies Citation[50–52] for analysis of protein systems. Three of the more prominent microarray-based approaches used to analyze protein abundances from complex cell lysate mixtures include forward-phase, sandwich, and reverse-phase cell lysate microarrays (RPLAs).
Forward-phase arrays
One of the first methods described was the forward-phase cell lysate array Citation[53]. With this method, antibodies were first deposited onto glass slides with a microarrayer. Subsequently, cells grown under different conditions were independently lysed, and their complex protein mixtures labeled independently with two differently colored dyes as in a standard mRNA expression profiling experiment. These two mixtures were then applied to the glass slides containing immobilized antibodies and the relative integrated intensities of the dyes were quantified to determine the relative abundances of proteins in the different cell samples. In practice, interpreting the data obtained by this method was complicated by the difficulty in labeling all proteins in a cell with a particular stoichiometry of dye molecules, as well as the difficulty in generating affinity reagents with the ability to quantitatively capture proteins from complex cell lysates.
Sandwich arrays
Another approach was subsequently described that functioned as a miniaturized sandwich ELISA Citation[54]. With this methodology, a monoclonal capture antibody was covalently immobilized onto the surface of glass slides. Following blocking of the slides with a protein such as bovine serum albumin, a complex lysate mixture was then incubated with the slides in order to capture particular proteins from the mixture. A second antibody was then added to detect the captured protein. This second antibody contained either a dye or another feature for quantifying the relative abundance of proteins in the complex mixture. While this methodology allowed for much greater selectivity in protein analysis owing to the use of two antibodies, it also suffered from the requirement for two monoclonal antibodies with high affinity and selectivity that bound to independent antigen epitopes so as not to compete with each other for binding to the antigen. An additional substantial limitation of this approach is the potential for multiple antibodies within the sample to cross-react with each other and interfere with the reporting of other antigens in the context of a complex lysate mixture.
A recent flow cell-based version of this approach has been described that mitigates some of the cross-reactivity problems inherent in multiplexed approaches that combine many antibodies in the same solution. In this new approach, magnetic bead-linked antibodies are incubated in sequential fashion with cell lysates in the solution phase. Then, following magnetic capture, these beads are incubated with secondary antibodies and quantified via a bead-based flow sorting machine Citation[55].
Reverse-phase lysate arrays
With the development of the RPLA methodology, picoliter volumes of proteins were microarrayed directly to nitrocellulose-coated slides Citation[56–62]. Then, following noncovalent immobilization, the abundance and modification of proteins were measured with a primary antibody directed at a single protein or modification followed by the addition of a secondary antibody directed at the primary antibody analogously as in a standard western blot. This approach has achieved widespread use for quantification of a relatively small but growing number of proteins in both clinical and basic research applications. The approach has achieved the greatest usage in core type facilities where a great deal of effort has been devoted to screening and validation of antibodies for use in the approach. Typically, antibodies are first screened against pools of lysates from several different cell lines using a standard western blotting method. When a large proportion of the antibody signal is found to originate from a single band at a position on the blot corresponding to the predicted molecular weight, then the antibody is more likely to be useful with the RPLA approach Citation[63–65]. If multiple bands are observed, in addition to the band at the correct molecular weight, then the antibody may not be useful with the approach because RPLAs are unable to discriminate between proteins of different sizes. A potential problem with the RPLA methodological pipeline is that even if most of an antibody signal appears to originate from a single band when observing pooled lysates, each cell line may contain a unique series of cross-reacting proteins that may be beneath the level of detection when diluted in the context of a pool of lysates, but may result in substantial off-target signal when analyzed at maximal concentration in the context of a single cell line. Off-target signals observed in the context of a western blot will typically result in the addition of a constant background signal in the context of a RPLA. This constant signal results in compression of the dynamic range of the true signal. Dynamic range compression may result in the masking of true differences in protein expression or modification across cell lines or tissues.
When a series of prescreened phospho-specific antibodies were examined side-by-side using both western blots and RPLAs, four out of 34 antibodies generated identical signals, eight out of 34 antibodies showed signals with compressed dynamic range by RPLA and 22 out of 34 antibodies did not show a substantial change in signal when examined by RPLA that had showed substantial changes in signal by western blots Citation[66]. This antibody cross-reactivity problem is likely to extend to any antibody-based approach that results in signals with no meta-data such as protein size or charge. Such approaches would include immunohistochemistry and flow cytometry.
Owing to the requirement for testing a large number of antibodies to achieve a small number that faithfully report accurate protein abundances, the RPLA method has primarily been used to analyze a relatively small number of proteins across a large panel of samples. This approach has been useful for the analysis of phosphorylation states of clinical samples and for the temporal analysis of cellular phosphorylation states following stimulation of cells with growth factors, small-molecule inhibitors and siRNA reagents Citation[67].
We and probably many others were dismayed at the large amount of work required to achieve a modest set of validated antibodies for the approach. We have since developed the micro-western array approach described in the next section, which allows for more rapid screening of antibodies than was previously possible and subsequently allows for antibodies with modest off-target problems to be used in a rapid manner to interrogate many biological conditions. Thus, with a combination of RPLAs and micro-western arrays, we are able to scan deeper (i.e., more conditions) and wider (i.e., more proteins and modifications) into protein space than was previously possible.
Micro-western arrays
A few years ago, our laboratory began working to develop a method that would leverage the throughput and multiplexing capabilities of the RPLA method but allow for electrophoretic separation of protein samples such as in the common western blotting method. We developed the micro-western arraying method to alleviate some of the problems of cross-reactivity that have limited the number of antibodies that can be used with RPLAs Citation[68]. With this method, six cell lysates were deposited 96 times onto the surface of acrylamide gels with a noncontact microarrayer. Following electrophoresis and transfer to nitrocellulose, printed replicates of these six lysates were confined to individual wells via gasketing devices and each well was probed with two antibodies, permitting up to 192 unique proteins to be assessed per 96-well device. A total of 1152 protein measurements were therefore possible in the first iteration of the method for each micro-western array.
We developed a method to cast acrylamide gels in such a way that they could be subsequently arranged on the arrayer deck for the microarraying process. Casting the gels with sheets of fabric provided sufficient tensile strength to the gels to allow them to be laid out along the arrayer deck without being easily damaged.
Next, a method was designed that would allow us to deposit the samples onto gels and allow them to be electrophoresed en masse. Depositing the samples directly to the gel in ten iterative spotting deposits allowed for nearly local saturation of protein into the gel and required no physical separation combs or stacking gels. This format allowed for proteins from six independent cell lysates and one protein standard to be examined simultaneously and required approximately 2 h for microarraying two gels that would ultimately lead to the production of two 96-well micro-western arrays. A total of 3 days were required to conduct the entire method from the point of arraying the cell lysates to the stage of analyzing images.
We then applied the micro-western array methodology to reproducibly examine a larger number of phosphosites temporally across a larger number of conditions than had previously been described Citation[68]. Following the screening of approximately 200 antibodies for signals at the predicted molecular weight in A431 cells stimulated with EGF, we chose 91 phospho-specific antibodies for the study. The analysis implicated several putative mechanisms of receptor tyrosine kinase coactivation, including: transient inactivation of phosphatases; direct transphosphorylation of heterotypic receptor tyrosine kinases via receptor heterodimerization; indirect transphosphorylation of heterotypic receptor tyrosine kinases by intracellular tyrosine kinases; and release of latent growth factors via activation of extracellular proteases. We are currently working to test the relevance of each of these sources of receptor tyrosine kinase transphosphorylation and transactivation, and are building models of network influences that take into account much larger numbers of phosphosite nodes.
We have since collaborated with several other laboratories to perform a similar approach. Typically in the first step, we perform a discovery-oriented screen of protein or modification changes in cells from few conditions using approximately 96 antibodies aimed at monitoring many different cell processes and then in a subsequent step, we monitor the changes in a focused set of targets over a larger set of time points and conditions.
Advantages of protein-omic approaches
The increased number of experimental conditions able to be addressed by protein-omic approaches affords one the opportunity to measure the temporal response of the system to combinatorial stimulations and inhibitions. The data from such experiments can then be analyzed with abstract modeling methods, such as principal component analysis and multivariate linear regression techniques Citation[69]. Regression techniques have the added advantage of identifying significant covariates between activated proteins in signaling networks and cell phenotypic responses across the experimental conditions probed. Even probabilistic network inference techniques, such as Bayesian network models Citation[70], which are powerful for identifying conditional dependencies between cell signaling nodes in an experimental dataset, become more robust and meaningful due to the large experimental space and accuracy afforded by protein-omic approaches. Clustering techniques and self-organizing maps, which serve to divide similarly expressed genes or proteins into subgroups for visualization or functional interpretation, are also aided by the ability to quantitatively probe across a larger number of conditions afforded by protein-omic approaches.
Several studies have already shown a glimpse of the insight that can be obtained by combining protein-omic approaches with computational techniques in the study of cell signaling networks. For example, time courses of 31 intracellular signals were measured via quantitative western blotting with 16 combinatorial stimuli of extracellular ligand and matrix components Citation[71]. Simple statistical data analyses allowed for signaling nodes to be correlated with differentiation and self-renewal phenotypes in murine embryonic stem cells. In another study, sandwich arrays were utilized to compare the dynamics of 17 intracellular signals of primary human hepatocytes and human hepatocarcinoma cells under seven stimulatory conditions and seven inhibitors Citation[72]. Simple regression of the affinity-based signaling network dataset revealed unexpected downregulation of inflammatory signaling in transformed hepatocytes, reflecting their poor innate immunity. In addition to the discovery-oriented protein-omic work, more hypothesis-driven work has been performed to answer relevant questions in signal transduction, especially to elucidate network cross-talk. For example, cells are under constant stimulation by conflicting signals, and activation of the TNF receptor results in activation of both pro- and anti-apoptotic downstream pathways; however, healthy cells robustly elicit specific cell responses despite conflicting signals Citation[73]. Affinity-based measurements of 19 intracellular proteins over 24 h in the presence of pro-death ligand TNF or pro-survival EGF and insulin identified several unexpected, previously unknown activations of downstream signals (such as pro-survival ERK activation under TNF stimulation) Citation[53]. Further inspection of these downstream signals led to the discovery of several autocrine cascades that elicit extracellular activation of cell surface receptors, which in concert regulate cell death. Reanalysis of these data has suggested that the range of signals could be more important for signal transduction than absolute signal strength Citation[74]. In another study, authors queried whether the differences in apoptotic responses of different cell lines upon treatment with TNF and adenoviral stimulation were due to cell-specific differences in signal transduction or whether common effectors existed that could serve as converging nodes Citation[75]. A predictive partial least squares regression model of only five signals under combinatorial stimulation of TNF, adenovirus, and IFN-γ led to the understanding that there exists a common processing mechanism with which cells are able to efficiently render apoptotic decisions. The power of protein-omic methodologies for hypothesis-driven mechanistic research has also been demonstrated elsewhere in conjunction with other elegant computational methodologies Citation[76,77]. We are confident that such protein-omic approaches will be progressively more valuable and insightful as the number of proteins analyzed per experiment scales upward to the level of thousands of proteins across many conditions concurrent with improved methods and higher numbers of antibodies.
Expert commentary
Many protein-omic studies to date have focused on relating differential protein phosphorylation levels with differential cell behavior following stimulation with perturbing agents. However, many other fundamental systems-level questions regarding protein abundance and function remain unanswered and are prime candidates for leveraging the power of protein-omic approaches: what role does variation in the human genome play in regulating protein expression and localization; what is the degree to which mRNA and protein expression are correlated among tissues; which protein abundances are robust to environmental influences and which are most sensitive?
Such questions are within reach of protein-omic methods. As an example, single-nucleotide polymorphisms (SNPs) have previously been identified that correlate with either the levels of particular mRNAs or with the response of cells to chemotherapeutic agents. Protein-omic methods are ideal for performing the same types of analysis with proteins because they are able to easily examine relative differences in protein abundance across many samples.
While SNPs in cis-regulatory regions might influence mRNA expression, the expression of the corresponding proteins may be buffered through alterations in mRNA translation, protein degradation or both. Conversely, SNPs that affect the activity or stability of a protein degradation factor may have little influence on mRNA stability but may have a dramatic impact on the global expression levels of whole families of proteins. Because numerous post-transcriptional mechanisms affect protein levels, directly examining the influence of genome loci on protein abundances can potentially provide biological insight and identify phenotypic biomarkers that could not be captured through evaluation of the transcriptome alone. Initial attempts at understanding the genetic basis of protein expression variation have shown several successes in this regard but have been limited by assay sensitivity, dynamic range and throughput Citation[78–81].
To begin to address such large-scale biological questions with greater sensitivity and scalability, the development and validation of large collections of antibodies will be invaluable. Human transcription factors represent an attractive protein family for first-pass analysis given their over-arching importance for many biological processes and cell behaviors through gene regulation. By raising antibodies to all human transcription factors and validating their performance with micro-western arrays, their relative expression and localization could be assessed and related to other datasets including genome variation, mRNA expression and cellular phenotypes. In a first pass, antibody signals could be validated by measuring the signal above background coupled with the observed versus expected molecular weight of proteins as measured by micro-western arrays across a panel of diverse cell and tissue types. Those antibodies that result in multiple bands of different molecular weights would then have to be analyzed in future experiments by micro-western arrays or another immunoblotting approach. However, those antibodies that resulted in a single predominant band in a test set of cell lines would then be candidates for future use with RPLAs, flow cytometry or other approaches that either are higher in throughput than micro-western arrays or provide more detailed information regarding the cell-to-cell variation in protein expression. Antibodies directed at proteins with interesting correlations to genome variation or to cell phenotypes could then be subjected to further validation such as siRNA knockdown, overexpression or peptide competition analyses to validate that the antibody interacts specifically with its intended target and to validate the causal role of observed protein correlations.
Five-year view
The next 5 years will represent an exciting time for the refinement, advancement and application of protein-omic approaches. While several major roadblocks have limited the widespread application of protein-omic methods, we are optimistic that many of these roadblocks will be lifted in the next several years. In order to begin to assemble thousands of custom antibodies, we have contracted with private companies to generate antibodies and then have used micro-western arrays to validate their performance. Even after an antibody has been shown to generate a single band by a western blot in one particular cell line or context, the possibility always exists that the next cell line will express a protein that cross-reacts with the antibody, giving rise to off-target signals. In unpublished work, we have used micro-western arrays for partial validation of thousands of antibodies in dozens of cell lines. By using information from micro-western arrays, we have generated a candidate set of over 200 antibodies that are useful with the RPLA method and another 200 that have off-target bands but are useful with the micro-western array. Any interesting biological observations that we make with RPLAs can then be followed up with micro-western arrays and by functional validation via siRNA, overexpression studies or alternative methods. While we are currently identifying relationships between protein abundances and genetic variation in cell lines, we hope to also begin to examine the expression of proteins across many human tissues. In addition to total protein expression, we also plan to examine the localization of proteins on a large scale. Such information should be valuable for elucidating causal relationships between transcription factors and the genes whose expression they influence after perturbation.
By saving the spleen cells from rabbits used in the generation of panels of polyclonal antibodies, monoclonal antibodies can subsequently be developed and more efficiently and rigorously validated across panels of cell lines and tissues using micro-western arrays. In the next 5 years, our goal is to have at least one antibody against 50% of all human proteins that is functional in at least one affinity-based method.
Lack of core facilities for validating and maintaining antibody stocks for applying the methods, and for assisting in data analysis and management has hindered the widespread application of protein-omic methods. While most major universities have mass spectrometry and functional genomics core facilities, few have facilities for the integrated use of RPLA, micro-western arrays or other protein-omic methods. This disparity is perhaps driven by the lack of integrated commercial instruments for performing the methods. In addition, most NIH instrumentation grants are custom-tailored for purchasing one large instrument rather than a collection of small instruments for performing a customized pipeline of techniques. Unlike approaches that are driven by instrument vendors, many recent protein-omic approaches have no sales force to introduce them to the biological community. We have recently established a core facility at the University of Chicago for performing micro-western arrays and RPLAs and are currently working with other universities to assist in their establishment of core facilities and hope to see several more established in the next few years to help accelerate the application of protein-omic approaches.
In summary, we see many areas of human biology and genetics being pushed forward through the continued advancement and integration of proteomic methods with methods in genomics and systems biology. It has been suggested that the western blot should be retired for proteomics Citation[82]. While we agree that western blots are not useful for proteomics, high-throughput western blots, when used in clever combination with other affinity-based methods, are extremely useful for protein-omics – a new and burgeoning field that will serve as a bridge between many other fields including proteomics, cell biology, genomics and systems biology.
Key issues
• Current large-scale proteomic methods are not ideal for the study of systems of proteins across many biological conditions.
• Functional genomics methods allow a comprehensive view of mRNA expression and are a paradigm for the reproducible examination of protein systems.
• Microarray-based protein-omic methods have evolved to provide more quantitative and high-throughput protein abundance and modification information than was previously possible.
• Micro-western arrays enable the validation and application of an unprecedented number of antibodies for quantitating protein abundance and modification.
• Combining reverse-phase cell lysate microarrays, micro-western arrays, immunohistochemistry and other protein-omic methods with data regarding variation in human genome, RNA expression and cell phenotypes may provide fundamental insights into many existing questions in proteomics, cell biology, genomics and systems biology.
Financial & competing interests disclosure
Ronald J Hause Jr was the recipient of an NIH Genetics and Regulation predoctoral training award. Kin K Leung was the recipient of a NIH Cancer Biology predoctoral training award. This work was partially supported by a Career Development award from the University of Chicago NIH Breast Cancer Specialized Program of Research Excellence P50 CA125183–05 to Richard Baker Jones; a pilot award from the NIH Chicago Center for Systems Biology P50 GM081892–03 to Richard Baker Jones; and an award the American Cancer Society Illinois Division to Richard Baker Jones.Richard Baker Jones is a coinventor of the following patent application related to the micro-western array methodology: Title: Methods and Compositions related to Microscale Sample Processing and Evaluation. Status: Pending. The authors have no other relevant affiliations or financial involvement with any organization or entity with a financial interest in or financial conflict with the subject matter or materials discussed in the manuscript apart from those disclosed.
No writing assistance was utilized in the production of this manuscript.
References
- Venter JC, Adams MD Myers E et al. The sequence of the human genome. Science291, 1304–1351 (2001).
- Lander ES, Linton LM, Birren B et al. Initial sequencing and analysis of the human genome. Nature409, 860–921 (2001).
- Schena M, Shalon D, Davis RW, Brown PO. Quantitative monitoring of gene expression patterns with a complementary DNA microarray. Science270, 467–470 (1995).
- Cloonan N, Forrest ARR, Kolle G et al. Stem cell transcriptome profiling via massive-scale mRNA sequencing. Nat. Meth.5, 613–619 (2008).
- Nagalakshmi U, Wang Z, Waern K et al. The transcriptional landscape of the yeast genome defined by RNA sequencing. Science320, 1344–1349 (2008).
- Linnarsson S. Recent advances in DNA sequencing methods – general principles of sample preparation. Exp. Cell Res.316, 1339–1343 (2010).
- Landgraf P, Rusu M, Sheridan R et al. A mammalian microRNA expression atlas based on small RNA library sequencing. Cell129, 1401–1414 (2007).
- Trapnell C, Williams BA, Pertea G et al. Transcript assembly and quantification by RNA-Seq reveals unannotated transcripts and isoform switching during cell differentiation. Nat. Biotech.28, 511–515 (2010).
- Wolf-Yadlin A, Hautaniemi S, Lauffenburger DA, White FM. Multiple reaction monitoring for robust quantitative proteomic analysis of cellular signaling networks. Proc. Natl Acad. Sci. USA104, 5860–5865 (2007).
- Denhardt DT. A membrane-filter technique for the detection of complementary DNA. Biochem. Biophys. Res. Commun.23, 641–646 (1966).
- Southern EM. Detection of specific sequences among DNA fragments separated by gel electrophoresis. J. Mol. Biol.98, 503–517 (1975).
- Lehrach H, Diamond D, Wozney JM, Boedtker H. RNA molecular weight determinations by gel electrophoresis under denaturing conditions, a critical reexamination. Biochemistry16, 4743–4751 (1977).
- Alwine JC, Kemp DJ, Stark GR. Method for detection of specific RNAs in agarose gels by transfer to diazobenzyloxymethyl-paper and hybridization with DNA probes. Proc. Natl Acad. Sci. USA74, 5350–5354 (1977).
- Stoeckle MY, Guan L. Improved resolution and sensitivity of northern blots using polyacrylamide-urea gels. Biotechniques15, 227, 230–231 (1993).
- Maniatis T, Fritsch EF, Sambrook J. Molecular Cloning: A Laboratory Manual. Cold Spring Harbor Laboratory Press, NY, USA (1989).
- Chomczynski P. One-hour downward alkaline capillary transfer for blotting of DNA and RNA. Anal. Biochem.201, 134–139 (1992).
- Augenlicht LH, Kobrin D. Cloning and screening of sequences expressed in a mouse colon tumor. Cancer Res.42, 1088–1093 (1982).
- Kulesh DA, Clive DR, Zarlenga DS, Greene JJ. Identification of interferon-modulated proliferation-related cDNA sequences. Proc. Natl Acad. Sci. USA84, 8453–8457 (1987).
- Shalon D, Smith SJ, Brown PO. A DNA microarray system for analyzing complex DNA samples using two-color fluorescent probe hybridization. Genome Res.6, 639–645 (1996).
- Velculescu VE, Zhang L, Zhou W et al. Characterization of the yeast transcriptome. Cell88, 243–251 (1997).
- Wepf A, Glatter T, Schmidt A, Aebersold R, Gstaiger M. Quantitative interaction proteomics using mass spectrometry. Nat. Methods6, 203–205 (2009).
- Breitkreutz A, Choi H, Sharom JR et al. A global protein kinase and phosphatase interaction network in yeast. Science328, 1043–1046 (2010).
- Kühner S, van Noort V, Betts MJ et al. Proteome organization in a genome-reduced bacterium. Science326, 1235–1240 (2009).
- de Godoy LMF, Olsen JV, Cox J et al. Comprehensive mass-spectrometry-based proteome quantification of haploid versus diploid yeast. Nature455, 1251–1254 (2008).
- Schrimpf SP, Weiss M, Reiter L et al. Comparative functional analysis of the Caenorhabditis elegans and Drosophila melanogaster proteomes. PLoS Biol.7, e1000048 (2009).
- Baerenfaller K, Grossmann J, Grobei MA et al. Genome-scale proteomics reveals Arabidopsis thaliana gene models and proteome dynamics. Science320, 938–941 (2008).
- Beck M, Claassen M, Aebersold R. Comprehensive proteomics. Curr. Opin. Biotechnol.22, 3–8 (2011).
- Ghaemmaghami S, Huh W, Bower K et al. Global analysis of protein expression in yeast. Nature425, 737–741 (2003).
- O’Farrell PH. High resolution two-dimensional electrophoresis of proteins. J. Biol. Chem.250, 4007–4021 (1975).
- Gygi SP, Corthals GL, Zhang Y, Rochon Y, Aebersold R. Evaluation of two-dimensional gel electrophoresis-based proteome analysis technology. Proc. Natl Acad. Sci. USA97, 9390–9395 (2000).
- Delahunty C, Yates III JR. Protein identification using 2D-LC-MS/MS. Methods35, 248–255 (2005).
- Takahashi N, Ishioka N, Takahashi Y, Putnam FW. Automated tandem high-performance liquid chromatographic system for separation of extremely complex peptide mixtures. J. Chromatogr.326, 407–418 (1985).
- Lundell N, Markides K. Two-dimensional liquid chromatography of peptides: an optimization strategy. Chromatographia34, 369–375 (1992).
- Gatlin CL, Kleemann GR, Hays LG, Link AJ, Yates JR. Protein identification at the low femtomole level from silver-stained gels using a new fritless electrospray interface for liquid chromatography-microspray and nanospray mass spectrometry. Anal. Biochem.263, 93–101 (1998).
- Gygi SP, Rist B, Gerber SA, Turecek F, Gelb MH, Aebersold R. Quantitative analysis of complex protein mixtures using isotope-coded affinity tags. Nat. Biotechnol.17, 994–999 (1999).
- Ross PL, Huang YN, Marchese JN et al. Multiplexed protein quantitation in Saccharomyces cerevisiae using amine-reactive isobaric tagging reagents. Mol. Cell. Proteomics3, 1154–1169 (2004).
- Ong S, Blagoev B, Kratchmarova I et al. Stable isotope labeling by amino acids in cell culture, SILAC, as a simple and accurate approach to expression proteomics. Mol. Cell. Proteomics1, 376–386 (2002).
- Oda Y, Huang K, Cross FR, Cowburn D, Chait BT. Accurate quantitation of protein expression and site-specific phosphorylation. Proc. Natl Acad. Sci. USA96, 6591-6596 (1999).
- Zhang Y, Wolf-Yadlin A, Ross PL et al. Time-resolved mass spectrometry of tyrosine phosphorylation sites in the epidermal growth factor receptor signaling network reveals dynamic modules. Mol. Cell. Proteomics4, 1240–1250 (2005).
- Julka S, Regnier F. Quantification in proteomics through stable isotope coding: a review. J. Proteome Res.3, 350–363 (2004).
- Ow SY, Salim M, Noirel J, Evans C, Rehman I, Wright PC. iTRAQ Underestimation in simple and complex mixtures: ‘the good, the bad and the ugly’. J. Proteome Res.8, 5347–5355 (2009).
- Hynes NE, Lane HA. ERBB receptors and cancer: the complexity of targeted inhibitors. Nat. Rev. Cancer5, 341–354 (2005).
- Yarden Y, Sliwkowski MX. Untangling the ErbB signalling network. Nat. Rev. Mol. Cell. Biol.2, 127–137 (2001).
- Citri A, Yarden Y. EGF–ERBB signalling: towards the systems level. Nat. Rev. Mol. Cell. Biol.7, 505–516 (2006).
- Lemmon MA, Schlessinger J. Cell signaling by receptor tyrosine kinases. Cell141, 1117–1134 (2010).
- Jorissen RN, Walker F, Pouliot N, Garrett TPJ, Ward CW, Burgess AW. Epidermal growth factor receptor: mechanisms of activation and signalling. Exp. Cell. Res.284, 31–53 (2003).
- Hynes NE, MacDonald G. ErbB receptors and signaling pathways in cancer. Curr. Opin. Cell Biol.21, 177–184 (2009).
- MacBeath G. Protein microarrays and proteomics. Nat. Genet.32(Suppl.), 526–532 (2002).
- Liotta LA, Espina V, Mehta AI et al. Protein microarrays: meeting analytical challenges for clinical applications. Cancer Cell3, 317–325 (2003).
- Krutzik PO, Irish JM, Nolan GP, Perez OD. Analysis of protein phosphorylation and cellular signaling events by flow cytometry: techniques and clinical applications. Clin. Immunol.110, 206–221 (2004).
- Krutzik PO, Nolan GP. Intracellular phospho-protein staining techniques for flow cytometry: monitoring single cell signaling events. Cytometry55A, 61–70 (2003).
- Perez OD, Nolan GP. Simultaneous measurement of multiple active kinase states using polychromatic flow cytometry. Nat. Biotechnol.20, 155–162 (2002).
- Haab BB, Dunham MJ, Brown PO. Protein microarrays for highly parallel detection and quantitation of specific proteins and antibodies in complex solutions. Genome Biol.2, RESEARCH0004 (2001).
- Nielsen UB, Cardone MH, Sinskey AJ, MacBeath G, Sorger PK. Profiling receptor tyrosine kinase activation by using Ab microarrays. Proc. Natl Acad. Sci. USA100, 9330–9335 (2003).
- Poetz O, Henzler T, Hartmann M et al. Sequential multiplex analyte capturing for phosphoprotein profiling. Mol. Cell. Proteomics9, 2474–2481 (2010).
- Paweletz CP, Charboneau L, Bichsel VE et al. Reverse phase protein microarrays which capture disease progression show activation of pro-survival pathways at the cancer invasion front. Oncogene20, 1981–1989 (2001).
- Liotta LA, Paweletz CP, Charboneau L et al. Reverse phase protein microarrays which capture disease progression show activation of pro-survival pathways at the cancer invasion front. Oncogene20, 1981–1989 (2001).
- Paweletz CP, Liotta LA, Petricoin EF. New technologies for biomarker analysis of prostate cancer progression: laser capture microdissection and tissue proteomics. Urology57, 160–163 (2001).
- Wulfkuhle JD, McLean KC, Paweletz CP et al. New approaches to proteomic analysis of breast cancer. Proteomics1, 1205–1215 (2001).
- Charboneau L, Tory H, Chen T et al. Utility of reverse phase protein arrays: applications to signalling pathways and human body arrays. Brief. Funct. Genomic Proteomic1, 305–315 (2002).
- Espina V, Munson PJ, Petricoin E et al. Proteomic profiling of the NCI-60 cancer cell lines using new high-density reverse-phase lysate microarrays. Proc. Natl Acad. Sci. USA100, 14229–14234 (2003).
- Grubb RL, Calvert VS, Wulkuhle JD et al. Signal pathway profiling of prostate cancer using reverse phase protein arrays. Proteomics3, 2142–2146 (2003).
- Sheehan KM, Calvert VS, Kay EW et al. Use of reverse phase protein microarrays and reference standard development for molecular network analysis of metastatic ovarian carcinoma. Mol. Cell. Proteomics4, 346–355 (2005).
- Mills GB, Liotta LA, Petricoin EF et al. Use of reverse phase protein microarrays and reference standard development for molecular network analysis of metastatic ovarian carcinoma. Mol. Cell. Proteomics4, 346–355 (2005).
- Tibes R, Qiu Y, Lu Y et al. Reverse phase protein array: validation of a novel proteomic technology and utility for analysis of primary leukemia specimens and hematopoietic stem cells. Mol. Cancer Ther.5, 2512–2521 (2006).
- Sevecka M, MacBeath G. State-based discovery: a multidimensional screen for small-molecule modulators of EGF signaling. Nat. Methods3, 825–831 (2006).
- Sevecka M, Wolf-Yadlin A, Macbeath G. Lysate microarrays enable high-throughput, quantitative investigations of cellular signaling. Mol. Cell. Proteomics10(4), M110.005363 (2011).
- Ciaccio MF, Wagner JP, Chuu C, Lauffenburger DA, Jones RB. Systems analysis of EGF receptor signaling dynamics with microwestern arrays. Nat. Methods7, 148–155 (2010).
- Janes KA, Yaffe MB. Data-driven modelling of signal-transduction networks. Nat. Rev. Mol. Cell. Biol.7, 820–828 (2006).
- Woolf PJ, Prudhomme W, Daheron L, Daley GQ, Lauffenburger DA. Bayesian analysis of signaling networks governing embryonic stem cell fate decisions. Bioinformatics21, 741–753 (2005).
- Prudhomme W, Daley GQ, Zandstra P, Lauffenburger DA. Multivariate proteomic analysis of murine embryonic stem cell self-renewal versus differentiation signaling. Proc. Natl Acad. Sci. USA101, 2900–2905 (2004).
- Alexopoulos LG, Saez-Rodriguez J, Cosgrove BD, Lauffenburger DA, Sorger PK. Networks inferred from biochemical data reveal profound differences in toll-like receptor and inflammatory signaling between normal and transformed hepatocytes. Mol. Cell. Proteomics9, 1849–1865 (2010).
- Janes KA, Gaudet S, Albeck JG, Nielsen UB, Lauffenburger DA, Sorger PK. The response of human epithelial cells to TNF involves an inducible autocrine cascade. Cell124, 1225–1239 (2006).
- Janes KA, Reinhardt HC, Yaffe MB. Cytokine-induced signaling networks prioritize dynamic range over signal strength. Cell135, 343–354 (2008).
- Miller-Jensen K, Janes KA, Brugge JS, Lauffenburger DA. Common effector processing mediates cell-specific responses to stimuli. Nature448, 604–608 (2007).
- Cosgrove BD, Alexopoulos LG, Hang T et al. Cytokine-associated drug toxicity in human hepatocytes is associated with signaling network dysregulation. Mol. BioSyst.6, 1195 (2010).
- Nakakuki T, Birtwistle MR, Saeki Y et al. Ligand-specific c-Fos expression emerges from the spatiotemporal control of ErbB network dynamics. Cell141, 884–896 (2010).
- Melzer D, Perry JRB, Hernandez D et al. A genome-wide association study identifies protein quantitative trait loci (pQTLs). PLoS Genet.4, e1000072 (2008).
- Garge N, Pan H, Rowland MD et al. Identification of quantitative trait loci underlying proteome variation in human lymphoblastoid cells. Mol. Cell. Proteomics9, 1383–1399 (2010).
- Foss EJ, Radulovic D, Shaffer SA et al. Genetic basis of proteomevariation in yeast. Nat. Genet.39, 1369–1375 (2007).
- Ghazalpour A, Bennett B, Petyuk VA et al. Comparative analysis of proteome and transcriptome variation in mouse. PLoS Genet.7, e1001393 (2011).
- Mann M. Can proteomics retire the western blot? J. Proteome Res.7, 3065 (2008).