Abstract
Ecosystems are becoming damaged or degraded as a result of stresses especially associated with human activities. A healthy ecosystem is essential to provide the services that humans and the natural environment require and has tremendous social and economic value. Exploration of the definition of ecosystem health includes what constitutes health and what it means to be healthy. To evaluate ecosystem health, it is necessary to quantify ecosystem conditions using a variety of indicators. In this paper, the main principles and criteria for indicator selection, classification of indicators for different kinds of ecosystems, the most appropriate indicators for measuring ecosystem sustainability, and various methods and models for the assessment of ecosystem health are presented. Drivers, sustainability, and resilience are considered to be critical factors for ecosystem health and its assessment. Effective integration of ecological understanding with socioeconomic, biophysical, biogeochemical, and public‐policy dimensions is still the primary challenge in this field, and devising workable strategies to achieve and maintain ecosystem health is a key future challenge.
Definition of Ecosystem Health
Human activity is considered to be the major driver of global environmental change and has great impacts on the Earth system (CitationVitousek et al. 1997). Climate change (CitationMoss et al. 2010), land use change (CitationFoley et al. 2005), biodiversity loss (CitationButchart et al. 2010), and many other environmental issues highlight the tremendous effect of human activity in our increasingly demanding economic world. Climate change, including temperature and precipitation change, has affected the phenology of organisms, the range and distribution of species, and the composition and dynamics of plant communities. (CitationWalther et al. 2002, CitationParmesan and Yohe 2003, CitationRoot et al. 2003). Climate change has impacts on crop productivity and has consequences for food availability (CitationGodfray et al. 2010, CitationWheeler and von Braun 2013). Land use change, especially the expansion of croplands, pastures, plantations, and urban areas to meet human needs, has resulted in considerable losses of biodiversity (CitationFoley et al. 2005). Such decreases of biodiversity may reduce ecosystem services that are vital to agriculture, such as pollination (CitationTscharntke et al. 2005). Habitat destruction also results in loss of ecosystem services and species extinctions (CitationTilman et al. 2001). As well as direct damage, the resilience of ecosystems and the functions they maintain are compromised through direct and indirect impacts of humans and environmental stress.
Development of ecosystem health concept
Since the Stockholm Conference on Human Environment held in 1972, ecologists have paid close attention to the study of how natural ecosystems respond to the various kinds of stresses from human activity. In order to measure ecosystem response to stress, CitationRapport et al. (1985) followed the physiologist, the late Hans CitationSelye (1973, 1974), who provided a framework for stress physiology by identifying common symptoms and the phases of adaptation to stress in mammalian systems. Health here refers to the absence of symptoms of ecosystem dysfunction based on a group of indicators and using subjective judgment rather than scientific criteria. This is the rudiment of ecosystem health: physiological methods have been creatively imported into the study of ecology.
What constitutes “health” reflects the perspective through which we evaluate ecosystems and the indicators we use to assess them. CitationSchaeffer et al. (1988) defined ecosystem health as the absence of disease, and disease here was defined as the failure of the ecosystem to function within acceptable limits, thereby leading to an inadequate homeostatic repair mechanism. CitationRapport (1989) defined ecosystem health from three perspectives: vital signs and system integrity, counteractive capacity, and threats from stress on the environment that are influenced by social and cultural values. CitationCostanza (1992) proposed that “ecosystem health is closely linked to the idea of sustainability, which is seen to be a comprehensive, multi‐scale, dynamic measure of system resilience, organization, and vigor.” What is “healthy” involves the direction and magnitude of deviation from normal status. CitationMageau et al. (1995) suggested that a healthy ecosystem is one that is sustainable; that is, it has the ability to maintain its structure and function over time in the face of external stress.
Ecosystem health was discussed at international conferences at the beginning of the 1990s (CitationCostanza et al. 1992), and a “working definition” of ecosystem health was presented in terms of characteristics for sustainability, which is a function of activity, organization, and resilience. It was concluded that “an ecological system is healthy and free from ‘distress syndrome' if it is stable and sustainable—that is, if it is active and maintains its organization, and autonomy over time and is resilient to stress” (CitationHaskell et al. 1992). Previous definitions had been given in terms of organization, resilience and vigor. CitationRapport et al. (1998) suggested combining these three elements into a biophysical perspective and incorporating socioeconomic and human health perspectives. Different from the previous concepts concerned with natural ecosystems, this construct has been widely applied in human‐coupled ecosystems.
Criticisms of the concept of ecosystem health and its theoretical basis were further explored in the 1990s. An early criticism focused on the organism theory—the idea that ecosystems are structurally and functionally like organisms. CitationEhrenfeld (1992) and CitationSuter (1993) argued that ecosystems are not organisms, per se, so they do not have properties of organisms such as health. Another controversy over ecosystem health is whether it is an objective scientific concept. Using the term “health” to describe an ecosystem implies there is a state that is good or bad for the system. However, the assessment of good or bad states of ecosystems can only be based on the kind of ecosystem expected by society (CitationWicklum and Davies 1995). Ecosystem health is therefore a term of value judgment. Value judgment changes over time as our understanding of the natural world changes, so it is not appropriate as a scientific basis for managing the environment (CitationLancaster 2000).
However, as CitationCostanza (2012) argued, ecosystem health is a “normative” concept that implies specific societal goals, rather than an “objective” scientific concept. Homeostasis might not be a common property of organisms and ecosystems, but that is not a reason for discarding ecosystem health as a useful concept. Moreover, the normative terms are mostly institutionalized in national and international policy and law (CitationCallicott et al. 1999). For example, ecosystem health is used in the Rio Declaration on Environment and Development for setting up a global agenda for ecosystem management (CitationUN General Assembly 1992).
Ecosystem health and ecosystem services for sustainability
Ecosystem health is essential for an ecosystem to provide services that benefit the human population in terms of social and economic value. Ecosystem services are generally divided into four categories: provisioning, regulating, supporting, and cultural services (CitationCostanza et al. 1997, CitationMillennium Ecosystem Assessment 2005). Supply of services relies on a well‐conditioned ecosystem, and the capacity of service supply will be impaired if an ecosystem is unhealthy, for example through loss of biodiversity (CitationBalvanera et al. 2006, CitationWorm et al. 2006). In order to advance human welfare through improved ecosystem services, a better understanding of the integrated social‐ecological system is needed so that appropriate policies and practices can be formulated (CitationCarpenter et al. 2009). Moreover, advances are required in the design of appropriate finance, policy, and governance systems and the form of implementation in diverse social and ecological contexts (CitationDaily and Matson 2008). Ecosystem services have been put into implementation in environmental management in many countries. For example, in China, the national programs of payments for ecosystem services such as the Natural Forest Conservation Program (NFCP) and the Grain to Green Program (GTGP) have improved ecosystem conditions and produced positive social‐economic effects (CitationLiu et al. 2008). In the UK, the National Ecosystem Assessment (2011) increasingly provides a basis for advocating change to more sustainable states.
After a couple of decades of discussion, the concept of ecosystem health has been gradually accepted and applied in the world. However, the “standard” of health as a concept is influenced by value judgment and is not completely objective, but ecosystem health is proving to be a normative and useful concept in environmental management. Structural organization and vigor need to be considered in order to reflect ecosystem structure and function, and resilience is critical in reflecting the capacity to recover from a perturbation. Concurrently, social‐economic aspects are fundamental to assessment, especially when evaluating trade‐offs between ecosystem services, for example when comparing provisioning and supporting functions. Ecosystem health is closely related to the concept of sustainability, which is defined to meet the current and future societal need for ecosystem services. The potential to continuously supply ecosystem services for the coming generations has been highlighted as an important issue within environment justice.
Based on this discussion, we define ecosystem health as the status and potential of an ecosystem to maintain its organizational structure, its vigor of function and resilience under stress, and to continuously provide quality ecosystem services for present and future generations in perpetuity.
Indicator System for Ecosystem Health
Criteria for indicator selection
Main principles
To evaluate ecosystem health, either qualitative or quantitative analysis needs to be made using a variety of indicators. Ideally, the suite of indicators should represent key information on the ecosystem structure and function and be specific for the goal of the assessment. In this regard, the following principles may be used for selection of key indicators.
1. Assess integrative ecosystem health
CitationCostanza (1992) and CitationRapport et al. (1998) proposed three groups of indicators, vigor, organization, and resilience (VOR), from the view of ecosystem sustainability to describe the holistic condition of a system, in which vigor could be represented by productivity, metabolism, etc.; organization by biodiversity indexes, parameters from network analysis, etc.; and resilience by growth range, recovery time after damage, etc. The VOR indicator system has been generally applied in the assessment of ecosystem health. Taking a broadly compatible approach, CitationJørgensen et al. (1995) used exergy, structural exergy and ecological buffer capacity to evaluate ecosystem health.
2. Describe the degradation of an ecosystem
CitationRapport et al. (1985) used ecosystem distress syndrome (EDS) for expression of an ecosystem in an unhealthy condition. It included specific indicators for description of the decline in nutrient pools, primary productivity, size distribution, and species diversity.
3. Reflect the function and structure of an ecosystem
CitationKay and Schneider (1992) used the term “ecosystem integrity” to refer to the ability of an ecosystem to maintain its organization through functional and structural attributes. CitationKarr (1993) used bio‐integrity indexes to evaluate aquatic ecosystem health in terms of changes in fish communities in terms of composition and distribution, species abundance, sensitive species, tolerant species, native species and exotic species, etc. CitationWaltner‐Toews (1996) emphasized that the choice of evaluation indicators need to be based on functional process, especially the evaluation of resilience under interference, including integrity, adaptability, and efficiency.
4. Represent sustainability of human‐coupled ecosystems
CitationRapport (1995) and CitationFairweather (1999) suggested that ecosystem health evaluation indicators include not only ecological indicators at multiple levels to capture the complexity of an ecosystem, but also biophysical indicators and social‐economic indicators to represent the quality and sustainability of ecosystem services provided for human society.
5. Present the goal of natural resources and ecosystem management
CitationJørgensen et al. (2011) proposed five criteria: “simple to apply and easily understood by laymen, relevant in the context, scientifically justifiable, quantitative and acceptable in terms of costs, when assessing ecosystem health from a practical environmental management point of view.”
Often, the selected indicators are more or less specific for a given stress or they are applicable only to a particular type of ecosystem or scale. Furthermore, the selection and optimization of ecosystem health indicators should reflect the independence of reference states and be applicable over extensive geographical areas (CitationJørgensen et al. 2011). The following criteria should be met for selection of the key indicators: (1) sensitive to stresses, (2) simple and easy to apply, (3) strong scientific basis, (4) possible to quantify, (5) anticipatory in responses to stresses, and (6) targeted to the goal of management.
Classification of indicators
Several systematic approaches to classification of indicators are in current usage. The following three are of general applicability.
Classification by the system of “stress, exposure, and response” is used by CitationJackson et al. (1990). Stressor indicators quantify a natural process, an environmental hazard, or a management action that effects changes in exposure and habitat. Exposure and habitat indicators identify the degree to which a system or its components might experience and respond to the stressor. Response indicators are measured to provide evidence of the biological condition of a resource at the level of organism, population, community, or ecosystem.
CitationCairns et al. (1993) proposed five groups of indicators for assessment purposes: (1) assessing current condition of the environment in order to judge its adequacy; (2) documenting trends in the condition over time, i.e., degradation or rehabilitation; (3) anticipating hazardous conditions before adverse impact in order to prevent damage; (4) identifying causative agents in order to specify appropriate management action; (5) demonstrating interdependence between indicators to make the assessment process more cost‐effectiveness and to reinforce political will to make environmentally sound management decisions. We summarize the major principles and characteristics of indicators for different purposes (), where scores are given for each characteristic of indicators on a scale of 1–5, from least important to most important.
CitationJørgensen et al. (2011) classified indicators into eight levels from the most reductionist to the most holistic. Level 1 covers the presence or absence of specific species; level 2 uses the ratio between classes of organisms; level 3 is based on concentrations of chemical compounds; level 4 applies concentration of entire trophic levels; level 5 uses process rates, for example primary production; level 6 covers composite indicators (biomass, respiration/production, production/biomass, primary producer/consumer, etc. [CitationOdum 1969]); level 7 encompasses holistic indicators such as resistance, resilience, buffering capacity, size, and connectivity of the ecological network, turnover rate of carbon, nitrogen, and energy, etc.; level 8 are thermodynamic variables, which we may call super holistic indicators, for example, they aim to capture the total image of the ecosystem without inclusion of details—exergy, structure exergy, emergy, entropy production, power, mass, and energy system retention time.
None of these systems are ideal, however, as they stand for the practical assessment of ecosystem health. The following biological, physicochemical, and socioeconomic indicators are proposed as the three main categories for ecosystem health assessment. shows the classification and common indicators of each category.
For the practical assessment of ecosystem health, we illustrate the following eight kinds of ecosystems, including forests, wetlands, rivers, lakes, estuaries and coastal zones, marine systems, agro‐ecosystems, and urban ecosystems, of which the first six are natural ecosystems, though variously affected by human activity, and the last two are human‐coupled ecosystems ().
Because of the complexity of an ecosystem, key species (sometimes termed focal species) are often identified to monitor the comprehensive ecosystem condition. Key species may have a dominant position in a certain ecosystem or are most sensitive to types of stress such as through change in climate or chemical pollution. Key species indicators have been widely used for ecosystem health assessment of forests or rivers, and may also be used in other ecosystems ().
Integrated indicator system for ecosystem sustainability
Due to limitations of a single‐indicator approach for ecosystem health assessment, various attempts have been made to build integrated indicator systems for certain stresses or assessment purposes. Resilience, equilibrium, and equity are three most important characters reflecting ecosystem sustainability.
Resilience represented by time lag in response and change in ecosystem production
Resilience is measured in terms of an ecosystem's capacity to rebound structurally and functionally from disturbance. Disturbance (or perturbation) has been central to ecosystem dynamics through evolutionary history, in some instances resulting in a rapid and rejuvenated recovery and in others in degradation and eventual collapse when resilience is exceeded (CitationVogel 1980, CitationSchlesinger et al. 1990). Both natural and human disturbances are stresses to ecosystems, which in turn may disrupt nutrient cycling, reduce productivity, cause the loss of biodiversity, and lead to other symptoms of ecosystem dysfunctions (CitationRapport et al. 1985).
“Resilience” is described as the capacity of an ecosystem to recover after dysfunction, while “resistance” is generally described as the capacity of maintaining ecosystem function under stress (CitationWhitford et al. 1999), which could be measured by the survival of selected species, primary productivity, nutrient cycling, biodiversity, and symbiosis persistence. Measurements of resilience could focus on the recovery rate and degree of those variables measured to evaluate resistance. To quantify resilience (R), we use time lag (TL) to express recovery rate and change in ecosystem production (CEP) to express recovery degree
where Tr refers to the time to total recovery; Td stands for the time at which disturbance ended; EPr refers to primary production after ecosystem recovery; EPd refers to primary production while the ecosystem is under disturbance.
At a practical level, the capacity for a system to have resistance and resilience can be tested by applying a perturbation or stress and observing the degree of initial deformation, then subsequent recovery. For example, soil can be exposed in the laboratory to stressors such as high temperature or heavy metals and indicators of its structure and function observed (CitationGriffiths and Philippot 2013, CitationGriffiths et al. 2008). A function such as ability to decompose organic matter, first decreases following the stress (resistance), then gradually recovers (time lag) but to a degree that may be less than the initial level.
Equilibrium represented by entropy‐based indicators
In ecology, “equilibrium” could describe a relatively stable state of an ecosystem during evolution, but is considered to be a “dynamic equilibrium,” because ecosystems are complex dynamic systems. Ecosystem development can be defined as a process in which a variety of biological communities, combined with physical environmental changes, will evolve until the evolutionary climax is achieved. Ecosystems can be viewed as non‐isolated systems able to maintain themselves in a far‐from‐equilibrium condition by exploiting the entropy exchanges with the surrounding environment (CitationSvirezhev 2000, CitationLudovisi 2014). Therefore, thermodynamics provides an evolutionary appropriate language to describe evolutionary process.
Entropy is a measure of the number of specific ways in which a thermodynamic system may be arranged, commonly understood as a measure of disorder. Since entropy is a state function, the change in the entropy of a system is the same for any process going from a given initial state to a given final state, whether the process is reversible or irreversible. The entropic distance from thermodynamic equilibrium and the entropy exchanges at the ecosystem boundaries are key variables describing the state of ecosystem. In the last decades, a batch of entropy‐based indicators have been proposed to describe the stage and orientation of ecosystem development, including exergy, structural information, entropy production, specific entropy production and the structure exergy ().
Among these indicators, the most widely used in ecosystem health assessment are exergy and structure exergy, which focus on the entropic distance from equilibrium and are able to capture the properties and cover the work capacity of an ecosystem. Exergy of an ecosystem accounts mostly for the free energy stored in biomass irrespective of the distribution among ecosystem components, while structure exergy emphasizes the structural differences among ecosystems having equivalent biomass (CitationJørgensen and Nielsen 1994).
Equity represented by degree of sharing and openness, and change in values of ecosystem services over time
To supply ecosystem services sustainably, an ecosystem needs to remain healthy and to maintain its functions. Valuing the services of an ecosystem's natural capital can be a useful guide when distinguishing and measuring where trade‐offs between human society and the rest of nature are possible and where they can be made to enhance human welfare in a sustainable manner (CitationCostanza et al. 1997, CitationFarber et al. 2002).
Equity of ecosystem services includes two aspects: space and time. “Space” refers to intragenerational equity of ecosystem services, which are valued by the degree of sharing and openness for access to production in an open market, macro or micro climate regulation, recreational value, culture and aesthetical value, etc. “Time” refers to intergenerational equity of ecosystem services, represented by the change in values of ecosystem services over time
where Vchange refers to the change in values of ecosystem services over time, Value1 and Value2 refer to the values of ecosystem services at time 1 and 2, and T refers to the time lag.
In a healthy ecosystem, change in values of ecosystem services over time should be stable, ensuring the sustainability of service provision. There are three conditions for Vchange: positive, 0, and negative. When Vchange is 0 or small, one may say that the intergenerational equity of ecosystem services is good and the ecosystem is relatively healthy and sustainable. However, if the increment is large relative to its original condition, it may indicate a long‐term degradation and hence weak sustainability. Negative Vchange represents the loss of intergenerational equity and the sustainability of ecosystem services has been damaged.
Ecosystem Health Assessment Methods
Methods for natural ecosystem
Holistic approach
1. Thermodynamic analysis
Exergy, a thermodynamic concept, has been applied to ecology since the 1970s. It is defined as the amount of work a system can perform when it is brought to thermodynamic equilibrium with its environment (CitationJørgensen and Mejer 1977, Citation). Exergy measures the amount of energy needed to break down the ecosystem. Structural exergy is the average exergy per total biomass in the system. With regard to the overall ecosystem health index consisting of system vigor, organization, and resilience (CitationCostanza 1992), exergy can express system vigor and resilience, while structural exergy represents system organization. Given resilience is multidimensional, focal buffer capacities involved in possible stress situations could be introduced to improve the ecosystem health assessment (CitationJørgensen 1995). Calculations of these indices are introduced in the Appendix: Table A1.
Based on exergy, structural exergy, and buffer capacities, CitationJørgensen (1995) assessed health states of 15 lake ecosystems. A clear relationship was found between these indicators and lake eutrophication states. Exergy and the first buffer capacity (phosphorus input as state variable) increase with increased eutrophication, while structural exergy and the other two buffer capacities (temperature and water flow as state variables) increase with the eutrophication up to a maximum value at a certain level of eutrophication above which they will decrease. CitationXu et al. (1999) investigated freshwater ecosystems stressed by four chemical stresses, acidification, copper, oil, and pesticide contamination with structural, functional, and ecosystem‐level indicators. Ecosystem‐level indicators included exergy, structural exergy, and zooplankton buffer capacity. The results showed that responses to chemical stress in ecosystem level and in structure and function of the studied ecosystems are highly correlated.
2. Network analysis
Network analysis extracts comprehensive information on the flow and cycling of matter from mass‐balanced flowcharts, including trophic structure and transfer efficiencies, and the organization of the food web (CitationField et al. 1989, CitationGaedke 1995). This approach is an important methodology in systems ecology to holistically analyze the complex interactions within an ecosystem (CitationFath et al. 2007), for it treats the ecosystem as a number of compartments interconnected with each other by flows of energy or matter. Major indicators include total system throughput (TST), ascendency (A), development capacity (C), average mutual information (I), and Finn's cycling index (FCI). TST represents the sum of all transfer processes occurring in the system and measures the level of overall system activity. Ascendency (A) involves ecosystem size and the degree of organization. Development capacity (C) denotes the diversity of the system flows scaled by the total system throughput. Average mutual information (I) represents the average constraint placed upon a single unit of flow in the network. Finn's cycling index (FCI) is the percentage of all fluxes of biomass that are generated by cycling. Calculations of these indices are introduced in the Appendix: Table A2.
Network analysis can efficiently characterize the vigor and organization of ecosystem. It is used to assess ecosystem health together with thermodynamic analysis. In the evaluation of the micro‐ and meio‐benthic community across the coastal area of the southern Adriatic Sea, Italy, structural exergy, ratio of ascendency to capacity, and Finn's cycling index are sensitive to anthropogenic pressure (CitationVassallo et al. 2006). As a result, these three indicators were proposed as candidate indices for coastal ecosystems health assessment.
Multi‐metric approach
Since the 1970s, researchers and managers have realized that monitoring of physical and chemical attributes of water are insufficient for aquatic ecosystem management. CitationKarr (1981) proposed a multi‐metric approach, based on an index of biological integrity (IBI) and including a range describing attributes of fish assemblages, to assess the water resource health. IBI integrates the functional and structural metrics of biological communities. CitationKarr (1981) selected 12 metrics from three categories: species richness and composition, trophic composition, and fish abundance and condition. Data were obtained for each of these metrics and metric values were given with a score of 1, 3, or 5, based on the deviation degree of the test sites from the reference sites (). Aggregation of the metric scores becomes the IBI measurement for each site.
IBI has subsequently been expanded to other biota, such as invertebrates (CitationFore et al. 1996), vascular plants (CitationMack 2007), and algae (CitationKane et al. 2009) in rivers, streams, lakes, wetlands, and coastal estuaries, and even applied to terrestrial ecosystems (CitationBradford et al. 1998, CitationGuilfoyle et al. 2009). Generally speaking, representative indicators are selected from each of four major categories: (1) richness measures for diversity of the assemblage; (2) composition measures for identity and dominance; (3) tolerance measures that represent sensitivity to perturbation; and (4) trophic measures for information on feeding strategies and guilds (CitationBarbour et al. 1999). For scoring indicators, alternative methods are developed and widely in use. CitationBryce et al. (2002) used linear interpolation to place each metric value on a scale of 0 to 10. CitationMack (2007) converted raw metric values to metric scores (1, 2, 3, and 4) by quadrisceting the 95th percentile of the metric value. In order to better distinguish the degree of impairment, individuals could select the most appropriate method according to the specific situation.
Predictive model approach
A river invertebrate prediction and classification system (RIVPACS) was first proposed and designed for assessment of biological quality of rivers in the UK (CitationWright et al. 1993). Based on the initial proof of a strong correlation between the environmental features and faunal characteristics of 370 reference sites, RIVPACS utilized a set of physical and chemical data to predict the macroinvertebrate fauna condition (expected) in the absence of stress over a wide range of river types (CitationWright et al. 1984, CitationWright 1995). The ratio of the number of macroinvertebrate fauna observed at a test site to the expected taxa number (O:E ratio) at reference sites provides a measure of biological impairment, which is a reflection of the ecosystem health states of rivers. The test sites should have similar physical and chemical properties with the reference sites. Based on RIVPACS, the Australian river assessment scheme (AUSRIVAS; CitationDavies et al. 2000) and benthic assessment of sediment (BEAST, Canada; CitationReynoldson et al. 1995) predictive models were established to assess the ecological conditions of rivers.
Despite the extensive use of the predictive models, one major problem is that these models evaluate freshwater ecosystem health based on the macroinvertebrate assemblage alone, and assume that these species reflect all responses of the freshwater ecosystem to stresses. Hence, the predictive models are not applicable when the river is disturbed but the macroinvertebrate community is not affected.
Methods for human‐coupled ecosystems
Urban ecosystems and agro‐ecosystems are representative of human‐coupled ecosystems. They are both natural, social‐economic, complex ecosystems and play a crucial role in human life. In this section, methods to assess the health of urban ecosystem and agro‐ecosystem are introduced.
First, an appropriate indicator system should be established. For urban ecosystem health assessment, five factors (vigor, structure, resilience, ecosystem service function maintenance, and environmental impact or population health) are mostly considered (CitationGuo et al. 2002, CitationSu et al. 2009). Besides, conceptual models, such as pressure‐state‐response (PSR; CitationZeng et al. 2005) and driving‐force‐pressure‐state‐exposure‐effect‐action (DPSEEA; CitationSpiegel et al. 2001) are recommended to organize indicators in a systematic way. For agro‐ecosystem health assessment, CitationVadrevu et al. (2008) considered six factors (biodiversity, soil quality, topography, social organization, farm economics, and land economics), while CitationZhu et al. (2012) addressed three subsystems (natural ecosystem, social system, and economic system) from four perspectives: vigor, organization, resilience, and equity.
Based on an appropriate indicator system, analytical hierarchy process (AHP) and other mathematical models are used to integrate these indicators.
1. Analytical hierarchy process (AHP) model
Five steps are included in the AHP (CitationVadrevu et al. 2008, CitationZhu et al. 2012): (1) structure the estimation problems into a hierarchical framework; (2) derive models to combine variables on the basis of their relationships with ecosystem health at each level in the hierarchy; (3) examine the consistency of these models; (4) aggregate the data according to the hierarchical model into a single index; (5) assess the results based on the underlying data.
2. Mathematical models
As for mathematical models, fuzzy synthetic assessment model (CitationGuo et al. 2002, CitationZhou and Wang 2005), attribute theory model (CitationYan et al. 2007), and set pair analysis (CitationSu et al. 2009) are introduced to assess urban ecosystem health because of features like fuzziness and multiple attributes. Here we elaborate on the fuzzy synthetic assessment model (CitationZhou and Wang 2005), which examined the relative health conditions of Beijing from 1996 to 2003.
1) | Set up the fuzzy set. According to the years and indicators of Beijing urban ecosystem health assessment, three fuzzy sets were set up: the evaluated object set O = {1996, 1997, 1998, … , 2003}; the indicator set U = {x1, x2, … , x18}, and the remark set V = {healthy, unhealthy}. | ||||
2) | Relative health indicators were divided into two categories, the positive indicators whose value became higher when the urban ecosystem became healthier, and the negative indicators whose value reduced less while the urban ecosystem became healthier. Relative health memberships, uh, of positive indicators were calculated as follows:
Relative health memberships of negative indicators were calculated as follows:
| ||||
3) | Calculate the relative health membership matrix
| ||||
4) | Calculate the comprehensive assessment matrix
|
3. Artificial neural networks
As computational models based on the structure and function of biological neural networks, artificial neural networks (ANN) are perceived as nonlinear statistical data modeling tools and are capable of tackling complicated problems such as pattern recognition, clustering, classification, and simulation (CitationAraghinejad 2014). There are various types of neural networks, but the most commonly used in ecological modeling is multi‐layer, feed‐forward, neural networks trained by a back‐propagation algorithm such as a back‐propagation network (BPN).
A BPN comprises three or more neuron layers, including an input layer, one or more hidden layers and an output layer, with each layer including one or more neurons. Neurons from one layer are connected to all neurons in the adjacent layer, but have no lateral connections within any layer and no feedback connections (). Based on the explicit input and output data, BPN could be trained by comparing the calculated output with the actual output and modifying connection weights to reduce error. A back‐propagation network can be used for ecosystem health assessment, with main procedures as shown in .
Figure 1. Schematic illustration of a back‐propagation neural network. Vertical dotted lines indicate more neurons in the layer.
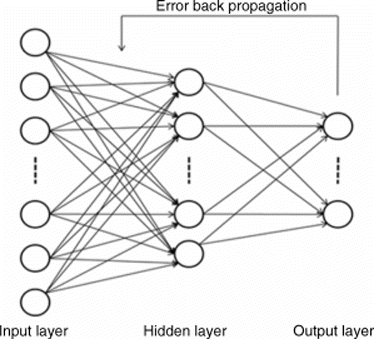
BPN models have been used to quantify the relationships between habitat variables (input) with specific species assemblages (output), such as macroinvertebrates (CitationGoethals et al. 2007), fish (CitationTirelli et al. 2009), and diatoms (CitationPhilibert et al. 2006). Similar to the predictive models mentioned previously, these BPN models can be used to assess the change of biological communities and subsequently the health of aquatic ecosystems.
4. Genetic algorithms
Genetic algorithms (GA) are optimum search techniques inspired by Charles Darwin's principle of “natural selection and survival of the fittest” (CitationHolland 1975, CitationGoldberg 1989). Similar to the process of population evolution, GA selects fit individuals for reproduction. Three main genetic operators involved in GA are selection, crossover, and mutation. From a population of randomly generated chromosomes, a subset of the fittest chromosomes is selected according to their adaptation and advances towards a new population of chromosomes by crossover and mutation. The procedure is applied iteratively on each population until some preselected criteria are met. GA may be used to choose the most appropriate indicators for predictive models of ecosystem health assessment (CitationD'Heygere et al. 2006, CitationSadeghi et al. 2013).
In summary, and based on available case studies, we classify the ecosystem health assessment methods in terms of natural or human‐coupled ecosystems. However, these methods may be applicable to other types of ecosystem health assessment, which needs to be further confirmed in more applications.
Critical Issues and Perspectives
In our understanding, three critical issues have to be taken into consideration when assessing ecosystem health. What are the major drivers of change in ecosystem conditions and how do they interact? How can ecosystem sustainability be achieved for the continuous supply of ecosystem services? What resilience strategies should be put in place to allow ecosystems to respond to external stresses?
Drivers
The disturbances or stresses that act as the drivers of change in ecosystems can be broadly categorized as natural and anthropogenic. Natural drivers, like solar radiation, natural climatic variability, and extreme geological activities, exert a significant but one‐way influence on ecosystem services, in that they themselves do not get feedback from the ecological changes they cause. In contrast, anthropogenic drivers may result in a wide range of changes to ecosystems, but because people live within or close to the ecosystems they affect, anthropogenic drivers get substantial feedback from ecosystems (CitationMillennium Ecosystem Assessment 2005). The natural and anthropogenic drivers interact over time. For example, the local species loss may initially be the result of anthropogenic influences, but may render an ecosystem more sensitive or more liable to degradation in the face of major events caused by natural drivers. It is the combined effects of several global change stressors that have mobilized major international concern and remediation efforts in recent years (CitationHooper et al. 2012). Considering that human welfare depends on ecosystem services (provisioning, regulating, cultural, and supporting), appropriate human intervention in ecosystems and the maintenance of ecosystem health will be a key research topic for the future.
Sustainability
The CitationWorld Commission on Environment and Development (1987) defined sustainability as “use of the environment and resources to meet the needs of the present without compromising the ability of future generations to meet their needs.” Sustainability has been adopted as the main goal set for many local, national, and international conservation or development efforts. In recent years, social‐ecological sustainability has been increasingly recognized in ecosystem stewardship (CitationChapin et al. 2010). Humans are an integral part of social‐ecological systems that can both affect and respond to ecosystem processes, so the synergies and trade‐offs between ecological and societal well‐being must be addressed when moving efforts towards sustainability (CitationChapin et al. 2009). In order to maintain and improve the conditions of ecosystem health based on current understanding of the environment with inevitable uncertainty and abrupt change, three strategies should be promoted to foster social‐ecological sustainability: control over known anthropogenic stresses, while appropriate intervention is necessary for the health of ecosystems such as forests; pre‐emptive actions and polices needed to keep the vitality of ecosystems; and understanding of mutual interactions within social‐ecological systems and avoiding or escaping unsustainable social‐ecological traps (CitationCairns et al. 1993, CitationChapin et al. 2010).
Resilience
The resilience of an ecosystem is one of the three main indicators proposed for assessing ecosystem health and refers to its ability to maintain its structure and function under stress (CitationCostanza and Mageau 1999). In practice, there are two components of resilience, one is resistance and the other is recovery. Given that anthropogenic climate change acts as a key driver of ecosystem change in the 21st century, resistance should be the focus, rather than recovery, as an increasing frequency of extreme climatic events limits the time available for recovery, and the chronic characteristic of climate stressors has also made the role of recovery less effective (CitationHoegh‐Guldberg et al. 2007, CitationCote and Darling 2010, CitationDarling et al. 2010). Meanwhile, non‐climate stressors, like pest disease, pollution, and land use change, will act separately or interactively with climate change. In practice, the selection of resilience indicators must distinguish between resistance and recovery based on both expert consensus and satisfactory empirical evidence (CitationKnudby et al. 2013). Furthermore, in the face of environmental change, resilience, that is the capacity to revert to the pre‐existing state following disturbance, should not be the unquestioned goal of ecosystem stewardship, since change at appropriate times is also necessary for a healthy ecosystem (CitationJulius et al. 2008, Citation).
There are three methods for recovery of ecosystem health: restoration, rehabilitation, and remediation. Restoration involves returning an ecosystem to a high ecological integrity. To do this, rehabilitation is used. Sometimes, due to fundamental changes to ecosystem conditions, the original ecosystem cannot be restored and a new ecosystem will need to be created, and this is remediation (CitationGeorges River Catchment 2004). Without the natural or anthropogenic stressors, ecosystems themselves are likely to evolve over time; while in the presence of certain stressors, ecosystems undergo dramatic changes that can alter the ecosystem irreversibly due to the severity of disturbance and degradation or lack of adequate resources, which makes the aims of ecological restoration hard to be achieved. Remediation methods and technologies have been increasingly developed for ecosystem management. The aim of remediation is to create a new ecosystem to replace the original one that cannot be restored because it was either irreversibly damaged or entirely removed, or no reference ecosystem exists to serve as a model for restoration. Considering that remediation technologies are more and more specific to various materials and contaminants (USEPA), remediation practice should be undertaken with adequate knowledge and skills to avoid unnecessary disturbance to the existing species and wildlife habitat values of the ecosystem (CitationGeorges River Catchment 2004, CitationKhin et al. 2012).
After more than 20 years discussion and practical applications of ecosystem health, the primary challenge of this field is still the effective integration of ecological understanding with socioeconomic, biophysical, biogeochemical, and public policy dimensions (CitationRapport et al. 1998, Citation, CitationWaltner‐Toews et al. 2003, CitationDepledge and Galloway 2005, CitationLeslie and McLeod 2007, CitationChapin et al. 2009, CitationCote and Darling 2010, CitationCostanza 2012, CitationWatters et al. 2013). To address the major challenge, novel approaches are required for ecosystem management. Indicators of VOR that can be sensed remotely or mapped spatially are an essential adjunct to ground‐based observations. Tools and models for measuring, valuing, and mapping ecosystem services of value to human society are also required. The combination of indicators of VO&R and ecosystem services using various statistical analysis and models is needed to achieve higher levels of ecosystem management. The measures of sustainability should include three complementary approaches, which are long‐term historical analysis, integrated landscape simulation, and microcosm experiments. To avoid the limitations of each of these approaches individually in testing the ecosystem‐health–sustainability hypothesis, a combination of these three would provide a powerful suite of tests (CitationCostanza 2012).
In integrating scales from individuals to the whole systems, small changes can be magnified in multiple ways, potentially through nonlinear interactions, facilitating regime shift and collapse. Thus, maintaining the adaptive capacities and strengthening the resilience of ecosystems by preserving a balance among heterogeneity, modularity, and redundancy is necessary for the sustainable ecosystem services (CitationLevin and Lubchenco 2008). Furthermore, it's important to quantify ecosystem services as natural capital, and incorporate the natural capital into decisions on resource and land use at different scales (CitationDaily et al. 2009). Three key concept components are important: the science of ecosystem production functions and service mapping; the design of appropriate finance, policy, and governance systems; and the art of implementing these in diverse biophysical and social contexts (CitationDaily and Matson 2008). More socioeconomic data are needed to understand human‐coupled ecosystem changes (CitationPolasky 2008). Under global change, combinations of decision theory, thresholds, scenarios, and resilience thinking can expand awareness of the potential states and outcomes, as well as of the probabilities and consequences of outcomes under alternative decisions (CitationPolasky et al. 2011). To achieve sustainability cooperation, competition, public goods, and common‐pool resources are fundamental features of biological and social systems (CitationLevin 2014). With more concerted efforts and interdisciplinary collaboration, devising workable strategies for ecosystem health towards sustainability can be achieved.
Acknowledgments
This work was supported by the National Natural Science Foundation of China (41371488, 41420104004), the International Scientific Cooperation Program with Grant No. 2012DFA91150), and the Key Research Program of the Chinese Academy of Sciences (No. KZZD‐EW‐TZ‐12).
Literature Cited
- Aoki, I. 1989. Holological study of lakes from an entropy viewpoint—Lake Mendota. Ecological Modelling 45: 81–93.
- Araghinejad, S. 2014. Artificial neural networks. Pages 139–194 in Data-driven modeling: using MATLAB in water resources and environmental engineering. Springer, New York, New York, USA.
- Balvanera, P., A. B. Pfisterer, N. Buchmann, J. S. He, T. Nakashizuka, D. Raffaelli, and B. Schmid. 2006. Quantifying the evidence for biodiversity effects on ecosystem functioning and services. Ecology Letters 9: 1146–1156.
- Barbour, M. T., J. Gerritsen, B. D. Snyder, and J. B. Stribling. 1999. Rapid bioassessment protocols for use in streams and wadeable rivers. U.S. EPA, Washington, D.C., USA.
- Bradford, D. F., S. E. Franson, A. C. Neale, D. T. Heggem, G. R. Miller, and G. E. Canterbury. 1998. Bird species assemblages as indicators of biological integrity in Great Basin rangeland. Environmental Monitoring And Assessment 49: 1–22.
- Bryce, S. A., R. M. Hughes, and P. R. Kaufmann. 2002. Development of a bird integrity index: using bird assemblages as indicators of riparian condition. Environmental Management 30: 294–310.
- Butchart, S. H. M., et al. 2010. Global biodiversity: indicators of recent declines. Science 328: 1164–1168.
- Cairns, J., P. Mccormick, and B. Niederlehner. 1993. A proposed framework for developing indicators of ecosystem health. Hydrobiologia 263: 1–44.
- Callicott, J. B., L. B. Crowder, and K. Mumford. 1999. Current normative concepts in conservation. Conservation Biology 13: 22–35.
- Carpenter, S. R., et al. 2009. Science for managing ecosystem services: beyond the Millennium Ecosystem Assessment. Proceedings of the National Academy of Sciences USA 106: 1305–1312.
- Chapin, F. S., et al. 2010. Ecosystem stewardship: sustainability strategies for a rapidly changing planet. Trends in Ecology & Evolution 25: 241–249.
- Chapin, F. S. I., G. P. Kofinas, and C. Folke. 2009. Principles of ecosystem stewardship. Springer, New York, New York, USA.
- Costanza, R. 1992. Towards an operational definition of ecosystem health. Pages 237–256 in R. Costanza, editor. Ecosystem health: new goals for environmental management. Island Press, Washington, D.C., USA.
- Costanza, R. 2012. Ecosystem health and ecological engineering. Ecological Engineering 45: 24–29.
- Costanza, R., et al. 1997. The value of the world's ecosystem services and natural capital. Nature 387: 252–60.
- Costanza, R., and M. Mageau. 1999. What is a healthy ecosystem? Aquatic Ecology 33: 105–115.
- Costanza, R., B. G. Norton, and B. D. Haskell. 1992. Ecosystem health: new goals for environmental management. Island Press, Washington, D.C., USA.
- Cote, I. M., and E. S. Darling. 2010. Rethinking ecosystem resilience in the face of climate change. PLoS Biology 8:e1000438.
- Daily, G. C., and P. A. Matson. 2008. Ecosystem services: from theory to implementation. Proceedings of the National Academy of Sciences USA 105: 9455–9456.
- Daily, G. C., S. Polasky, J. Goldstein, P. M. Kareiva, H. A. Mooney, L. Pejchar, T. H. Ricketts, J. Salzman, and R. Shallenberger. 2009. Ecosystem services in decision making: time to deliver. Frontiers in Ecology and the Environment 7: 21–28.
- Darling, E. S., T. R. Mcclanahan, and I. M. Côté. 2010. Combined effects of two stressors on Kenyan coral reefs are additive or antagonistic, not synergistic. Conservation Letters 3: 122–130.
- Davies, P. E., J. F. Wright, D. W. Sutcliffe, and M. T. Furse. 2000. Development of a national river bioassessment system (AUSRIVAS) in Australia. Pages 113–124 in Assessing the biological quality of fresh waters: RIVPACS and other techniques. Proceedings of an International Workshop held in Oxford, UK, on 16–18 September 1997. Freshwater Biological Association (FBA), Far Sawrey, Ambleside, Cumbria, UK.
- Depledge, M. H., and T. S. Galloway. 2005. Healthy animals, healthy ecosystems. Frontiers in Ecology and the Environment 3: 251–258.
- D'heygere, T., P. L. Goethals, and N. De pauw. 2006. Genetic algorithms for optimisation of predictive ecosystems models based on decision trees and neural networks. Ecological Modelling 195: 20–29.
- Ehrenfeld, D. 1992. Ecosystem health and ecological theories. Pages 135–143 in R. Costanza, editor. Ecosystem health: new goals for environmental management. Island Press, Washington, D.C., USA.
- Fairweather, P. G. St. 1999. State of environmental indicators of “river health”: exploring the metaphor. Freshwater Biology 41: 221–234.
- Farber, S. C., R. Costanza, and M. Wilson. 2002. Economic and ecological concepts for valuing ecosystem services. Ecological Economics 41: 375–392.
- Fath, B. D., U. M. Scharler, R. E. Ulanowicz, and B. Hannon. 2007. Ecological network analysis: network construction. Ecological Modelling 208: 49–55.
- Field, J. G., F. Wulff, and K. H. Mann. 1989. The need to analyze ecological networks. Pages 3–12 in F. Wulff, J. G. Field, and K. H. Mann, editors. Network analysis in marine ecology: methods and applications. Springer, New York, New York, USA.
- Foley, J. A., et al. 2005. Global consequences of land use. Science 309: 570–574.
- Fore, L. S., J. R. Karr, and R. W. Wisseman. 1996. Assessing invertebrate responses to human activities: evaluating alternative approaches. Journal of the North American Benthological Society 15: 212–231.
- Gaedke, U. 1995. A comparison of whole-community and ecosystem approaches (biomass size distributions, food web analysis, network analysis, simulation models) to study the structure, function and regulation of pelagic food webs. Journal of Plankton Research 17: 1273–1305.
- Georges River Catchment . 2004. Ecological restoration and remediation projects—planning and implementation. Department of Infrastructure, Planning and Natural Resources, New South Wales, Australia.
- Godfray, H. C. J., J. R. Beddington, I. R. Crute, L. Haddad, D. Lawrence, J. F. Muir, J. Pretty, S. Robinson, S. M. Thomas, and C. Toulmin. 2010. Food security: the challenge of feeding 9 billion people. Science 327: 812–818.
- Goethals, P. L., A. P. Dedecker, W. Gabriels, S. Lek, and N. De pauw. 2007. Applications of artificial neural networks predicting macroinvertebrates in freshwaters. Aquatic Ecology 41: 491–508.
- Goldberg, D. E. 1989. Genetic algorithms in search, optimization and machine learning. Addison-Wesley, Reading, Massachusetts, USA.
- Griffiths, B. S., P. D. Hallett, H. L. Kuan, A. S. Gregory, C. W. Watts, and A. P. Whitmore. 2008. Functional resilience of soil microbial communities depends on both soil structure and microbial community composition. Biology and Fertility of Soils 44: 745–754.
- Griffiths, B. S., and L. Philippot. 2013. Insights into the resistance and resilience of the soil microbial community. FEMS Microbiology Review 37: 112–129.
- Guilfoyle, M. P., J. S. Wakeley, and R. A. Fischer. 2009. Applying an avian index of biological integrity to assess and monitor arid and semi-arid riparian ecosystems. U.S. Army Engineer Research and Development Center, Vicksburg, Mississippi, USA.
- Guo, X., J. Yang, and X. Mao. 2002. Primary studies on urban ecosystem health assessment. China Environment Science 22: 525–529.
- Haskell, B. D., B. G. Norton, and R. Costanza. 1992. What is ecosystem health and why should we worry about it. Pages 3–20 in R. Costanza, editor. Ecosystem health: new goals for environmental management. Island Press, Washington, D.C., USA.
- Hoegh-guldberg, O., et al. 2007. Coral reefs under rapid climate change and ocean acidification. Science 318: 1737–1742.
- Holland, J. H. 1975. Adaptation in natural and artificial systems: an introductory analysis with applications to biology, control, and artificial intelligence. University of Michigan Press, Ann Arbor, Michigan, USA.
- Hooper, D. U., E. C. Adair, B. J. Cardinale, J. E. K. Byrnes, B. A. Hungate, K. L. Matulich, A. Gonzalez, J. E. Duffy, L. Gamfeldt, and M. I. O'connor. 2012. A global synthesis reveals biodiversity loss as a major driver of ecosystem change. Nature 486: 105–108.
- Jackson, L. E., J. C. Kurtz, and W. S. Fisher, editors. 1990. Environmental monitoring and assessment program: ecological indicators. USEPA/600/3–90/060. U.S. Environmental Protection Agency, Office of Research and Development, Research Triangle Park, North Carolina, USA.
- Jørgensen, S. E. 1995. The application of ecological indicators to assess the ecological condition of a lake. Lakes & Reservoirs: Research & Management 1: 177–182.
- Jørgensen, S. E., and H. Mejer. 1977. Ecological buffer capacity. Ecological Modelling 3: 39–61.
- Jørgensen, S. E., and H. Mejer. 1979. A holistic approach to ecological modelling. Ecological Modelling 7: 169–189.
- Jørgensen, S. E., and S. Nielsen. 1994. Models of the structural dynamics in lakes and reservoirs. Ecological Modelling 74: 39–46.
- Jørgensen, S. E., S. Nielson, and H. Mejer. 1995. Emergy, environ, exergy and ecological modelling. Ecological Modelling 77: 99–109.
- Jørgensen, S. E., F. Xu, J. Marques, and F. Salas. 2011. Application of indicators for the assessment of ecosystem health. Pages 9–76 in S. Jørgensen, editor. Handbook of ecological indicators for assessment of ecosystem health. Second edition. CRC Press, Boca Raton, Florida, USA.
- Julius, S. H., J. M. West, and G. M. Blate. 2008. Introduction. Pages 2-1–2-24 in S. H. Julius and J. M. West, editors. Preliminary review of adaptation options for climate-sensitive ecosystems and resources. A Report by the U.S. climate change science program and the subcommittee on global change research. U.S. Environmental Protection Agency, Washington, D.C., USA.
- Julius, S. H., J. M. West, D. Nover, R. S. D. S. Hauser, A. C. Janetos, M. K. Walsh, and P. Backlund. 2013. Climate change and U.S. natural resources. Advancing the Nation's Capability to Adapt. Issues in Ecology 18.
- Kane, D. D., S. I. Gordon, M. Munawar, M. N. Charlton, and D. A. Culver. 2009. The Planktonic Index of Biotic Integrity (P-IBI): an approach for assessing lake ecosystem health. Ecological Indicators 9: 1234–1247.
- Karr, J. R. 1981. Assessment of biotic integrity using fish communities. Fisheries 6: 21–27.
- Karr, J. R. 1993. Defining and assessing ecological integrity: beyond water quality. Environmental Toxicology and Chemistry 12: 1521–1531.
- Karr, J. R., K. D. Fausch, P. L. Angermeier, P. R. Yant, and I. J. Schlosser. 1986. Assessing biological integrity in running waters: a method and its rationale. Illinois Natural History Survey Special Publication 5. Illinois Natural History Survey, Champaign, Illinois, USA.
- Kay, J., and E. Schneider. 1992. Thermodynamics and measures of ecological integrity. Ecological Indicators 159: 82.
- Khin, M. M., A. S. Nair, V. J. Babu, R. Murugan, and S. Ramakrishna. 2012. A review on nanomaterials for environmental remediation. Energy & Environmental Science 5: 8075–8109.
- Knudby, A., S. Jupiter, C. Roelfsema, M. Lyons, and S. Phinn. 2013. Mapping coral reef resilience indicators using field and remotely sensed data. Remote Sensing 5: 1311–1334.
- Lancaster, J. 2000. The ridiculous notion of assessing ecological health and identifying the useful concepts underneath. Human and Ecological Risk Assessment 6: 213–222.
- Leslie, H. M., and K. L. Mcleod. 2007. Confronting the challenges of implementing marine ecosystem-based management. Frontiers in Ecology and the Environment 5: 540–548.
- Levin, S. A. 2014. Public goods in relation to competition, cooperation, and spite. Proceedings of the National Academy of Sciences USA 111 (supplement 3): 10838–10845.
- Levin, S. A., and J. Lubchenco. 2008. Resilience, robustness, and marine ecosystem-based management. BioScience 58: 27–32.
- Liu, J., S. Li, Z. Ouyang, C. Tam, and X. Chen. 2008. Ecological and socioeconomic effects of China's policies for ecosystem services. Proceedings of the National Academy of Sciences USA 105: 9477–9482.
- Ludovisi, A. 2009. Exergy vs information in ecological successions: interpreting community changes by a classical thermodynamic approach. Ecological Modelling 220: 1566–1577.
- Ludovisi, A. 2012. Energy degradation and ecosystem development: theoretical framing, indicators definition and application to a test case study. Ecological Indicators 20: 204–212.
- Ludovisi, A. 2014. Effectiveness of entropy-based functions in the analysis of ecosystem state and development. Ecological Indicators 36: 617–623.
- Mack, J. J. 2007. Developing a wetland IBI with statewide application after multiple testing iterations. Ecological Indicators 7: 864–881.
- Mageau, M. T., R. Costanza, and R. E. Ulanowicz. 1995. The development and initial testing of a quantitative assessment of ecosystem health. Health 1: 201–213.
- Millennium Ecosystem Assessment. 2005. Global assessment report. Island Press, Washington, D.C., USA.
- Moss, R. H., et al. 2010. The next generation of scenarios for climate change research and assessment. Nature 463: 747–756.
- Odum, E. P. 1969. The strategy of ecosystem development. Science 164: 262–270.
- Parmesan, C., and G. Yohe. 2003. A globally coherent fingerprint of climate change impacts across natural systems. Nature 421: 37–42.
- Philibert, A., P. Gell, P. Newall, B. Chessman, and N. Bate. 2006. Development of diatom-based tools for assessing stream water quality in south-eastern Australia: assessment of environmental transfer functions. Hydrobiologia 572: 103–114.
- Polasky, S. 2008. Why conservation planning needs socioeconomic data. Proceedings of the National Academy of Sciences USA 105: 6505–6506.
- Polasky, S., S. R. Carpenter, C. Folke, and B. Keeler. 2011. Decision-making under great uncertainty: environmental management in an era of global change. Trends in Ecology & Evolution 26: 398–404.
- Rapport, D. J. 1989. What constitutes ecosystem health. Perspectives in Biology and Medicine 33: 120–132.
- Rapport, D. J. 1995. Ecosystem services and management options as blanket indicators of ecosystem health. Journal of Aquatic Ecosystem Health 4: 97–105.
- Rapport, D. J., et al. 1999. Ecosystem health: the concept, the ISEH, and the important tasks ahead. Ecosystem Health 5: 82–90.
- Rapport, D., R. Costanza, and A. Mcmichael. 1998. Assessing ecosystem health. Trends in Ecology & Evolution 13: 397–402.
- Rapport, D. J., H. Regier, and T. Hutchinson. 1985. Ecosystem behavior under stress. American Naturalist 125: 617–640.
- Reynoldson, T. B., R. C. Bailey, K. E. Day, and R. H. Norris. 1995. Biological guidelines for freshwater sediment based on BEnthic Assessment of SedimenT (the BEAST) using a multivariate approach for predicting biological state. Australian Journal of Ecology 20: 198–219.
- Root, T. L., J. T. Price, K. R. Hall, S. H. Schneider, C. Rosenzweig, and J. A. Pounds. 2003. Fingerprints of global warming on wild animals and plants. Nature 421: 57–60.
- Roset, N., G. Grenouillet, D. Goffaux, D. Pont, and P. Kestemont. 2007. A review of existing fish assemblage indicators and methodologies. Fisheries Management and Ecology 14: 393–405.
- Sadeghi, R., R. Zarkami, K. Sabetraftar, and P. Van damme. 2013. Application of genetic algorithm and greedy stepwise to select input variables in classification tree models for the prediction of habitat requirements of Azolla filiculoides (Lam.) in Anzali wetland, Iran. Ecological Modelling 251: 44–53.
- Schaeffer, D. J., E. E. Herricks, and H. W. Kerster. 1988. Ecosystem health. 1. Measuring ecosystem health. Environmental Management 12: 445–455.
- Schlesinger, W. H., J. Reynolds, G. Cunningham, L. Huenneke, W. Jarrell, R. Virginia, and W. Whitford. 1990. Biological feedbacks in global desertification. Science 247: 1043–1048.
- Selye, H. 1973. The evolution of the stress concept. American Scientist 61: 692–699.
- Seyle, H. 1974. Stress without distress. Lippincott, New York, New York, USA.
- Spiegel, J. M., M. Bonet, A. Yassi, E. Molina, M. Concepcion, and P. Mast. 2001. Developing ecosystem health indicators in Centro Habana: a community-based approach. Ecosystem Health 7: 15–26.
- Su, M. R., Z. F. Yang, B. Chen, and S. Ulgiati. 2009. Urban ecosystem health assessment based on emergy and set pair analysis—a comparative study of typical Chinese cities. Ecological Modelling 220: 2341–2348.
- Suter, G. W. 1993. A critique of ecosystem health concepts and indexes. Environmental Toxicology And Chemistry 12: 1533–1539.
- Svirezhev, Y. M. 2000. Thermodynamics and ecology. Ecological Modelling 132: 11–22.
- Tilman, D., J. Fargione, B. Wolff, C. D'antonio, A. Dobson, R. Howarth, D. Schindler, W. H. Schlesinger, D. Simberloff, and D. Swackhamer. 2001. Forecasting agriculturally driven global environmental change. Science 292: 281–284.
- Tirelli, T., L. Pozzi, and D. Pessani. 2009. Use of different approaches to model presence/absence of Salmo marmoratus in Piedmont (Northwestern Italy). Ecological Informatics 4: 234–242.
- Tscharntke, T., A. M. Klein, A. Kruess, I. Steffan-dewenter, and C. Thies. 2005. Landscape perspectives on agricultural intensification and biodiversity—ecosystem service management. Ecology Letters 8: 857–874.
- UK National Ecosystem Assessment. 2011. The UK National Ecosystem Assessment. UNEP-WCMC, Cambridge, UK.
- UN General Assembly. 1992. Rio declaration on environment and development. Agenda 21. United Nations, New York, New York, USA.
- Vadrevu, K. P., J. Cardina, F. Hitzhusen, I. Bayoh, R. Moore, J. Parker, B. Stinner, D. Stinner, and C. Hoy. 2008. Case study of an integrated framework for quantifying agroecosystem health. Ecosystems 11: 283–306.
- Vassallo, P., M. Fabiano, L. Vezzulli, R. Sandulli, J. C. Marques, and S. E. Jørgensen. 2006. Assessing the health of coastal marine ecosystems: a holistic approach based on sediment micro and meio-benthic measures. Ecological Indicators 6: 525–542.
- Vitousek, P. M., H. A. Mooney, J. Lubchenco, and J. M. Melillo. 1997. Human domination of Earth's ecosystems. Science 277: 494–499.
- Vogel, R. J 1980. The ecological factors that produce perturbation-dependent ecosystems. Page 63–94 in Cairns, J. Jr., editor. The recovery process in damaged ecosystems. Ann Arbor Science Press, Ann Arbor, Michigan, USA.
- Walther, G. R., E. Post, P. Convey, A. Menzel, C. Parmesan, T. J. C. Beebee, J. M. Fromentin, O. Hoegh-guldberg, and F. Bairlein. 2002. Ecological responses to recent climate change. Nature 416: 389–395.
- Waltner-toews, D. 1996. Ecosystem health—a framework for implementing sustainability in agriculture. BioScience 46: 686–689.
- Waltner-toews, D., J. J. Kay, C. Neudoerffer, and T. Gitau. 2003. Perspective changes everything: managing ecosystems from the inside out. Frontiers in Ecology and the Environment 1: 23–30.
- Watters, G. M., S. L. Hill, J. T. Hinke, J. Matthews, and K. Reid. 2013. Decision-making for ecosystem-based management: evaluating options for a krill fishery with an ecosystem dynamics model. Ecological Applications 23: 710–725.
- Wheeler, T., and J. Von braun. 2013. Climate change impacts on global food security. Science 341: 508–513.
- Whitford, W. G., D. Rapport, and A. Desoyza. 1999. Using resistance and resilience measurements for “fitness” tests in ecosystem health. Journal of Environmental Management 57: 21–29.
- Wicklum, D., and R. W. Davies. 1995. Ecosystem health and integrity. Canadian Journal of Botany 73: 997–1000.
- World Commission on Environment and Development. 1987. Our common future. Oxford University Press, Oxford, UK.
- Worm, B., et al. 2006. Impacts of biodiversity loss on ocean ecosystem services. Science 314: 787–790.
- Wright, J. F. 1995. Development and use of a system for predicting the macroinvertebrate fauna in flowing waters. Australian Journal of Ecology 20: 181–197.
- Wright, J. F., P. D. Armitage, M. T. Furse, and D. Moss. 1984. Classification of sites on British rivers using macroinvertebrates. Verhandlung Internationale Vereinigung Limnologie 22: 1939–1943.
- Wright, J. F., M. T. Furse, and P. D. Armitage. 1993. RIVPACS: a technique for evaluating the biological quality of rivers in the UK. European Water Pollution Control 3: 15–25.
- Xu, F.-L., S. E. Jørgensen, and S. Tao. 1999. Ecological indicators for assessing freshwater ecosystem health. Ecological Modelling 116: 77–106.
- Yan, W., X. Yuan, and Z. Xing. 2007. Urban ecosystem health assessment based on attribute theory: a case study in the New District of northern Chongqing City. Chinese Journal of Ecology 26: 1679–1684.
- Zeng, Y., G. Shen, S. Huang, and M. Wang. 2005. Assessment of urban ecosystem health in Shanghai. Resources and Environment in the Yangtze Basin 10: 208–212.
- Zhou, W., and R. Wang. 2005. An entropy weight approach on the fuzzy synthetic assessment of Beijing urban ecosystem health, China. Acta Ecologica Sinica 25: 3244–3251.
- Zhu, W., S. Wang, and C. D. Caldwell. 2012. Pathways of assessing agroecosystem health and agroecosystem management. Acta Ecologica Sinica 32: 9–17.
Ecological Archives
An appendix is available online: http://dx.doi.org/10.1890/EHS14‐0013.1.sm