Abstract
In 1983, while investigators had identified a few human proteins as important regulators of specific biological outcomes, how these proteins acted in the cell was essentially unknown in almost all cases. Twenty-five years later, our knowledge of the mechanistic basis of protein action has been transformed by our increasingly detailed understanding of protein-protein interactions, which have allowed us to define cellular machines. The advent of the yeast two-hybrid (Y2H) system in 1989 marked a milestone in the field of proteomics. Exploiting the modular nature of transcription factors, the Y2H system allows facile measurement of the activation of reporter genes based on interactions between two chimeric or “hybrid” proteins of interest. After a decade of service as a leading platform for individual investigators to use in exploring the interaction properties of interesting target proteins, the Y2H system has increasingly been applied in high-throughput applications intended to map genome-scale protein-protein interactions for model organisms and humans. Although some significant technical limitations apply, Y2H has made a great contribution to our general understanding of the topology of cellular signaling networks.
Introduction
Within the last 25 years, the yeast two-hybrid (Y2H) system has helped transform our understanding of the molecular landscape of a cell. By 1983, mammalian application of increasingly powerful molecular biological techniques began to identify novel genes that encoded proteins that were important for study based on their functional criteria. For example, mutation of the oncogene Ras (Citation1) or amplification of the oncogene Myc (Citation2) was known to cause cancer in humans. The proteins encoded by these and other important genes were then further characterized primarily by simple overexpression of intact, truncated, or mutated derivatives of the protein of interest, followed by measurements of changes in gross cellular processes such as proliferation or morphological change. This “isolationist,” single protein–centered approach was marked by the very limited nature of mechanistic insights that could be gained from the relationship between a primary protein of interest and its protein partner(s). For some proteins such as Ras that had known orthologs in lower eukaryotes (Citation3), some insights into relevant signaling pathways could be gleaned by inference from genetic studies in those organisms (Citation4). For proteins without orthologs such as Myc, options were more restricted. In 1983, the primary means of identifying interacting proteins was through biochemical co-purification and protein sequencing. These techniques were difficult, laborious, and expensive.
As databases of potentially interesting proteins burgeoned through the 1980s, this pattern continued. While most signaling proteins were thought or known to have at least one effector, existing signaling pathways were sparsely populated and drawn to indicate linear connections between interacting proteins. At its initial description in 1989 (Citation5), it was not apparent that the Y2H assay was a technological advance that would transform our understanding of cell biology (Citation6–11). Initially described as a simple yet powerful tool to detect protein-protein interactions (PPIs), Y2H technology evolved in order to address PPIs of increasing complexity and to ultimately be applied on a genome-wide scale. As most revolutionary technologies are judged by the physical or intellectual products they create, we believe that the Y2H system can be judged by the changing paradigm of protein interactions it helped usher in: from binary protein interactions of 25 years ago, to canonical pathways, and, most recently, interconnected protein interaction networks.
Y2H Basics
The original Y2H system was developed as an assay to study binary protein interactions between proteins already known or those strongly suspected to associate (Citation5). The system capitalizes on the modular nature of transcription factors with split domains that can reconstitute protein function upon physical interaction (Citation12). The protein of interest (X), or bait, is fused to the DNA-binding domain (DBD) of a transcription factor such as Gal4 or LexA. (). The potential interactor protein (Y), or prey, is fused to a transcriptional activating domain (AD). DBD-X and AD-Y are co-expressed in an appropriate yeast strain that has been engineered to contain reporter genes with transcription depending on the association of DBD-X and AD-Y at the promoter. While the first described Y2H system used only a lacZ colorimetric reporter that was not amenable to high-throughput usage using 1980s technology, the subsequent addition (Citation8,Citation10) of secondary auxotrophic reporters opened the door for the use of Y2H for library screening. Some of the very earliest uses of Y2H for such screening involved oncoproteins such as Ras and Myc (Citation11,Citation13). The instructive case of screening with Ras set forth what was to become a typical paradigm: although for a number of years there had been accumulating evidence suggesting a functional relationship between Ras and a second oncoprotein, Raf, Y2H screening clarified the observation that a direct physical association underlies their functional relation (Citation11). Suddenly, proteins could quickly find partners.
Protein-protein interactions are detected by the mating of two haploid complementary yeast strains, each expressing a distinct expression plasmid. The first strain expresses a protein prey fused to a transcription activation domain (AD). The second strain expresses a protein bait fused to a DNA-binding domain (DBD) that binds to its cognate binding site, usually upstream of a reporter gene. If there is interaction between bait and prey, the AD is brought into proximity of the DBD to cause transcriptional activation of the reporter gene and lead to selection.
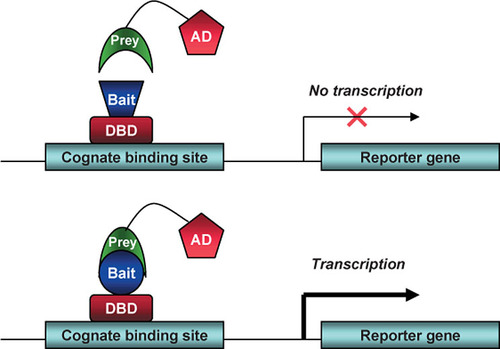
The establishment of Y2H library screening capabilities allowed many investigators to try to identify other proteins that interacted with their protein of interest. In the approach that was standard in the early 1990s, a yeast strain expressing bait and reporter constructs that had passed certain optimization steps (see next section on Y2H limitations) would be supertransformed with a cDNA library expressing AD-fused proteins. Then 1–3 × 106 transformants would be scored for activation of lacZ and the auxotrophic reporters. The basic technical manipulations required little more than low-cost microbiological growth media and minimal laboratory equipment, and were readily accomplishable by any laboratory with basic competence in molecular biology. The requisite plasmids and yeast strains were essentially free, distributed from the laboratories of the inventors of the technique. From start to finish, a screen could be performed in less than 6 weeks. These advantages underlie the essentially democratic nature of Y2H screening: with such a low investment cost, many could afford to attempt the approach.
Y2H Limitations
Before the development of Y2H, older biochemical techniques available to study protein interactions had specific limitations. For example, co-immunoprecipitation experiments required the existence of robust antibodies for target proteins, which were not always available. As a positive, the associations of proteins could be studied in native context, sometimes with complexes of partners; as a negative, binding of the immunoprecipitating antibody to the target protein had the potential to competitively block the binding of the target to partners of interest. The end point of a co-immunoprecipitation or other biochemical purification experiment was analysis of the pool of interacting proteins by protein sequencing or mass spectrometry. This required a very large amount of starting material; even when this was available, difficulties in purification of sufficient amounts of some proteins caused mass spectrometry to be inadequate for large-scale screening (Citation14) when dependent on technologies of the 1980s and early 1990s.
While Y2H avoided these limitations by use of a highly engineered, artificial reporting system, it possessed its own set of restrictions, one of which was that bait proteins possessing intrinsic transcriptional activity could not initially be studied since they would activate the reporter genes in the absence of interaction with an AD-fused partner. To circumvent this fault, a number of approaches were attempted with varying degrees of success. As one strategy, investigators fused transcriptionally active proteins to ADs and conducted screens of DBD libraries (Citation15). This fix, however, led to the need for a strategy to prescreen the DBD-fused libraries to eliminate a new set of transcriptionally activating baits. Another creative approach involved use of a RNA-polymerase III transcription system that was not responsive to classic RNA polymerase II transcriptionally activating proteins to enable screening of such baits (Citation16,Citation17). Another method removed transcriptional activating proteins from the nucleus entirely, creating a parallel reporter system based at the cell membrane (Citation18).
An important issue that rapidly emerged for investigators using Y2H was that most baits yielded at least some false positives, and some baits produced many. This led to significant investment of effort toward understanding their cause and reducing their incidence (Citation19–21). False-positive results can be broken down into two categories: technical and biological. Technical false positives are faults of the reporter systems in the Y2H assay when reporter activity is induced in the absence of any PPIs. Causes for such artifactual readouts include DBD- or AD-fused proteins that can weakly activate transcription of the reporter gene in the absence of mutual interaction, coupled with plasmid copy number changes or rearrangements that result in either overexpression of weak activators or auto-activation of the reporter (Citation22,Citation23). Biological false positives are genuine interactions that do not represent PPIs in the cellular milieu, but occur in the context of the Y2H system where all proteins are forced into the cell nucleus. Cellular compartmentalization or localization may prevent in vivo interaction of proteins that have the appropriate cognate recognition sequences. Arguably, biological false positives are more difficult to circumvent than technical false positives because the former are less amenable to technical optimization. In all, some have estimated that false positives account for 50% of the current high-throughput Y2H datasets (Citation24–26). Other high-throughput methodologies used to detect PPIs post no better marks, with spurious results also approximated at 50% (Citation26). Grappling with these potentially high error rates was a serious issue as laboratories began to broadly apply Y2H techniques.
Many investigators adapting the Y2H system wanted to ask more complicated questions about PPIs that were not intrinsically amenable in a yeast-based system. This desire was partially addressed by the development of Y2H system variants (Citation27). For example, many eukaryotic protein interactions depend on posttranslational modifications. To compensate for the absence of relevant modifying enzymes in yeast species, co-expression of appropriate modifiers, such as tyrosine kinases, along with the bait and prey, was used to successfully study signal transduction pathways (Citation28,Citation29).
Others wished to expand the scope of the Y2H system to study protein-RNA and protein-drug interactions. To accomplish this, investigators used a known RNA or ligand-binding protein conjugated to the DBD to serve as a “hook” (Citation30–33). This modification optimized the presentation of RNA or ligand-binding elements of bait proteins to nontraditional prey. Others sought to use Y2H techniques to detect specific mutations leading to loss of protein interaction. Investigators have used negative selection markers to search for mutations that disrupt binding of cell cycle–related proteins (Citation34,Citation35). In addition, a counterselective Y2H system has been used to search for drugs or peptide ligands that can disrupt specific protein-protein interactions (Citation34,Citation36–38). Finally, Stagljar and colleagues developed a Y2H derivative that allowed efficient study of membrane-tethered proteins. This was an important advance that rendered a notoriously difficult class of protein targets amenable to study (Citation18).
There have been a number of successes (notably in the arena of protein-RNA interactions or interactions of membranetethered proteins) using these complicated modifications to the Y2H assay. However, as of 2008, the general applicability of some of these Y2H variations as gold standard techniques is questionable. Many of the “evolved” forms of the Y2H system suffer from a very high background of false positives. Others, while deserving enormous commendation for creativity, are extremely technically difficult to set up because of the need to optimize multiple components (Citation27). Pragmatically, widespread use of particular modified Y2H systems has been precluded because of fairly robust alternative technologies available in the field for specific applications. Examples of techniques that outcompeted the Y2H system include chromatin immunoprecipitation for protein-DNA interactions and fluorescence polarization or structural model-based screening for protein-targeted drugs (Citation14).
Genome-wide Y2H: First Steps
The large-scale adaptation of the Y2H system by individual investigators was reflected by a surge in publications reporting screening results through the 1990s (). Although many of these studies initiated with an interaction predicted by a Y2H “hit,” validation of results was extensive, leading to high confidence in the interactions thus obtained. As the Y2H technique was applied to thousands of proteins, most biologically interesting signaling proteins were found to possess multiple interactors. Signaling pathway diagrams became more complex, and research strategies emphasized the analysis of how, and under what specific conditions, one protein modified the localization and function of each of its known partners to achieve a final functional end point (e.g., increased proliferation).
Numbers appearing across the graph represent citations of the seminal 1989 Fields and Song publication of a Y2H approach (Citation5). Green bars represent the results recovered in each year from the Web of Science for a topic line query “yeast two-hybrid” OR “yeast two hybrid.” Red bars represent results recovered in each year from a PubMed search using the terms, yeast and two-hybrid and YEAR. We hypothesize that the divergence of trends for the last set of data versus the first two in the years 2006 and 2007 reflects the growing use of results from high-throughput Y2H screening projects in studies not directly using the Y2H system.
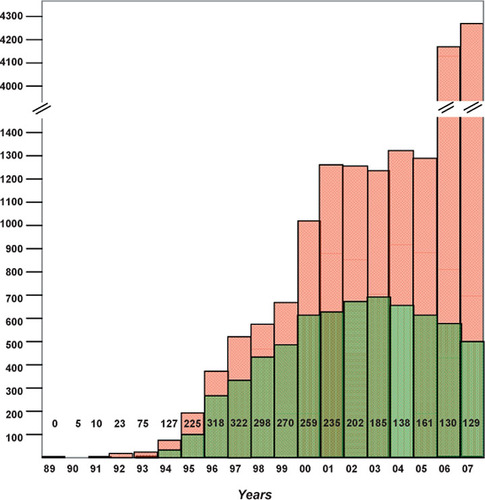
Since 2000, the publication of complete genome sequences for humans and several important model organisms (Citation39–43) spurred the search for methodologies to characterize previously undescribed open reading frames (ORFs). Clearly, the interaction of a protein product of an uncharacterized ORF with a protein of known function could be used to begin to ascribe functional specificity to the ORF (Citation14). In an attempt to keep the realm of knowledge of protein interactions on par with the rapidly increasing stream of genomic information, investigators adapted the Y2H system for high-throughput analyses (Citation23)(Citation44–53).
The study of the protein interaction map of bacteriophage T7 (Citation44) was the first genomic-wide application of Y2H technology. However, the first truly high-throughput Y2H screens addressed the genome of the Y2H host, Saccharomyces cerevisiae, itself, with the work of three competing groups appearing in 2000 and 2001 (Citation24,Citation52,Citation53). The goal of these studies was to begin to elucidate the protein interactions of the approximately 6000 gene products in the budding yeast. Although some of the work described involved the use of standard library screening, such an undertaking was greatly facilitated by modification of the Y2H system to increase its capacity for multiplexing a large number of reactions and to improve its compatibility with robotic automation. These high-throughput analyses were enabled by efficient exploitation of a key biological feature of yeast: the ability of yeast cells of opposite haploid mating types to mate to form a diploid cell.
As first described by Finley and colleagues in 1994 (Citation54), it was possible to transform a reporter strain of mating type α with a plasmid expressing DBD-X and a strain of mating type a with a plasmid expressing AD-Y. Upon mixing these yeast, a positive interaction result is scorable in the a/α diploid. Hence, if wishing to test 10 baits against 20 preys, it is only necessary to perform 20 transformations followed by mating (which could easily be performed on 1–2 culture plates or in a microtiter plate format), instead of 200 independent transformations. When considering genome-level screens, the arithmetic versus multiplicative nature of mating-based screening provides a critical enabling tool. The largest of the S. cerevisiae screens (Citation53) took a bi-directional approach, using a mating-based protein array in addition to a protein library method.
The array method involved the construction of approximately 6000 yeast transformants, each expressing a unique ORF fused to an AD arranged in specific positions on microassay plates. Large-scale homologous recombination-based cloning (Citation55) and transformation were performed using PCR-generated products of S. cerevisiae ORFs that contained 5′ and 3′ flanking sequences directly matching those contained within the AD vector. A set of 192 ORF-DBD fusion products was also constructed and transformed into a yeast strain of the opposite mating type. Mating of each of the 192 DBD fusions to each member of the AD hybrid array provided information on potential PPIs in S. cerevisiae. A parallel approach used by the investigators involved pooling roughly 6000 ORF-AD transformants into a library, while a similar set of roughly 6000 ORF-DBD transformants was arranged in microassay plates. Each of the DBD fusion yeast was mated with the AD yeast library, and potential interacting proteins were identified by growth selection followed by sequencing (Citation56).
Both Y2H screening modalities have some drawbacks, primary of which was reproducibility: only 20% of identified interactions by the array method were reproduced in a second screen of the array. The library method afforded improved reproducibility, with 68% of potential interactions being validated in independent experiments or multiple times in a single experiment. These numbers were not optimal. The capacity of the library and array methods to detect protein-protein interactions also differed. Only 8% of the total proteins used in the library screen identified interactors, as compared with 45% in the array screen. This discrepancy may be accounted for by the library screen’s more limited ability to detect PPIs that result in reduced growth rate or mating ability (Citation14) and may also reflect the lower reproducibility of positives identified by the array method. The library method’s redeeming feature, however, is that it is highly amenable to high-throughput screening. In comparison to library-based screening, a purely array-based screen is much more labor and time intensive, even with automation.
Over 5600 interactions, involving approximately 70% of yeast proteins, were detected by these initial high-throughput screens of S. cerevisiae (Citation24,Citation52,Citation53). As this set of interactions only constitutes a small portion of the 37,800–75,500 estimated interactions in budding yeast, much work remains to be done (Citation57–60). The insights gleaned from these reported interactions can be classified into several categories. First, the screens detected interactions of functionally unclassified proteins with proteins of known function, placing the former into the functional context of the latter. For example, one screen identified a function at the spindle pole body for one previously uncharacterized protein (Citation24). Second, proteins involved in two separate pathways, such as autophagy and cytoplasm-to-vacuole targeting, were shown to interact, urging further experiments to detect pathway cross-talk (Citation53). Finally, novel interconnections discovered between members of the same functional pathway, such as cell cycle progression, opened new avenues of investigation (Citation53).
Genome-wide Y2H: Complex Eukaryotes
Just as the genomic sequencing of increasingly complex organisms prompted the evolution of the Y2H system to high-throughput scale, the scaled-up Y2H system enabled the PPI-screening of proteomes of increasing size. In addition to S. cerevisiae, large-scale protein interaction screens have been conducted for several viruses and bacteria, Plasmodium falciparum, Caenorhabditis elegans, Drosophila melanogaster, and most recently, Homo sapiens (Citation44–51).
Focusing on the results addressing humans, Rual et al. used a modified minilibrary Y2H screen to test for PPIs among 7200 ORF-encoded proteins, or approximately 10% of the comprehensive human proteome (Citation49). This screen detected roughly 2800 interactions, comprising, the authors posited, ∼1% of the human protein interactome (i.e., the set of possible potential interactions). As a testament to the steady improvements in methodology, 78% of the detected interactions were verified by an independent co-affinity purification assay. Stelzl et al. also used a mini-pooling strategy to screen an array comprised of over 5500 human proteins derived primarily from a fetal brain cDNA library (Citation50). This study identified a network of approximately 3200 PPIs. Independent pull-down and co-immunoprecipitation assays of a representative sample of the interactions validated over 60% of the findings.
Surprisingly, different Y2H datasets nominally probing the same interaction pool—for example, the human proteome—have tended to have minimal overlap. Out of the ∼2800 PPIs in the human proteome reported by Rual and the ∼3200 interactions reported by Stelzl, the two studies show concordance in merely 17 interactions (Citation49,Citation50). The explanation for this discrepancy lies partly in the limited clone set used in the two studies, each study encompassing less than roughly ∼30% of the predicted human genes. Regardless, the two groups screened approximately 1000 proteins in common (Citation61). Similar lack of overlap is seen when comparing the results of high-throughput Y2H screens of S. cerevisiae and D. melanogaster to other screens of the same species (Citation24,Citation45,Citation53,Citation61,Citation62). False-positive results produced by Y2H screens may partly explain the inconsistencies present in high-throughput datasets. Separately, there is likely to be a high rate of false negatives involved, based on extrapolation of results from many individual investigators. The technical reasons for potential false positives and negatives specific to Y2H have been discussed elsewhere (Citation23). At present, it is likely that existing networks are being greatly under-sampled.
To help establish the biological relevance of their findings, Rual and colleagues demonstrated that interacting pairs of their human interactome had an increased likelihood of gene co-expression, a common evolutionary-conserved upstream DNA sequence, mouse orthologs with a common phenotype, and annotation with common Gene Ontology (GO) terms. Similarly, Stelzl and colleagues supported the biological relevance of their interactome by demonstrating that their interactor proteins were found not only in human interaction clusters but also in model organism ortholog interaction clusters, and shared common GO annotations. A major direction of high-throughput Y2H screening is the drive to rapidly translate findings to potential insights into disease mechanisms. Both studies cross-referenced their datasets with the disease-associated genes in the Online Mendelian Inheritance in Man (OMIM) database. The datasets produced by the two studies placed over 600 disease-associated proteins into novel context, finding interactions that might offer directions for clinical research. Focusing on one pathway of known relevance to development and disease, Stelzl and colleagues performed functional analyses on two uncharacterized Wnt pathway interactors and identified them as modulators of canonical Wnt signaling.
While the focus of this review is the Y2H system, several other techniques are used to study cellular PPIs: mass spectroscopy of purified complexes (Citation63,Citation64), correlated mRNA expression profiling (Citation65,Citation66), genetic interaction (synthetic lethality) (Citation67,Citation68), and in silico analysis (computed predictions based on gene context) (Citation69–72). If one were to assume, in an ideal scientific world, that each technology is equally adept at detecting PPIs, one might expect overlapping interaction data obtained from studies using different techniques. However, in a meta-analysis of the ∼80,000 interactions currently described among yeast proteins, fewer than 5% were found to be corroborated by more than one methodology (Citation26). In this analysis of datasets, von Mering and colleagues reported that different techniques have dissimilar abilities in detecting interactions among certain functional classes of proteins (Citation26). For example, mass spectroscopy predicts notably few interactions between proteins involved in transport and sensing; a weakness owing to the difficulty in purification of these transmembrane proteins. Similarly, Y2H methodology seldom detects interactions between proteins involved in translation (Citation26). These findings clearly demonstrate the issue of system bias as a factor in constructing global networks.
In general, the Y2H system detects binary interactions and has the capability to detect relatively weak and transient interactions based on the overexpression of bait and prey in the nucleus, which can reach micromolar levels (Citation9). In contrast, mass spectroscopy is able to detect more complex interactions in which the energy driving the interaction is distributed among multiple protein partners. As a result of practical detection limits of current instrumentation, however, this technique is biased toward detection of proteins of greater abundance and stability (Citation14,Citation23,Citation26). For these reasons, these differing techniques can be considered complementary, rather than all-inclusive, in constructing an interactome. Nevertheless, there is good reason to take as “gold standard” interactions that are detected by both Y2H and biochemical techniques (Citation26,Citation73,Citation74).
Interpreting Signaling Networks
Already, the datasets obtained from genome-scale Y2H studies yield a number of insights into network-level cell signaling pathways and the structure of cellular PPI networks. While most proteins in a network have few interactors, certain “hub” proteins interact with a great number of other proteins. As further complication, not all hubs act equivalently. Detailed analyses of protein interactome datasets subclassified hubs into two categories: date and party (Citation75,Citation76). Date hubs contact their interactors at varying times and locations, acting as major convergence points for multiple signaling pathways. Party hubs are defined as proteins that interact with most of their partners concurrently, forming the basis of multiprotein cellular machines. Party hubs have more restricted functions than date hubs in guiding the interactions of proteins within individual pathways.
Genetic studies in lower eukaryotes have indicated that most proteins in a network can be mutationally inactivated without significant effects on essential signaling pathways (Citation75,Citation77–79). Notably, essential genes frequently encode hub proteins, and knockout of such genes in yeast confers a 3-fold rise in lethality as compared with knockout of nonhub encoding genes (Citation23,Citation76,Citation78,Citation80). In line with their function as convergence points, perturbations in party hubs in particular tend to sensitize the protein interactome to other perturbations (Citation76). It is not surprising to realize that core components of canonical genetically defined pathways of the pregenomic era are being recognized today as hubs (Citation75). In considering nonessential proteins, it is clear that natural fail-safes to circumvent a signaling “blockade” and death due to loss of a hub include the common use of parallel network connections and occupation of key positions by protein paralogues, which buttress the robustness of cellular networks.
It is important to note that many important viral, bacterial, and parasitic organisms remain to be subjected to proteome-scale analysis by Y2H and other tools. Increased understanding of the protein interactomes of human pathogens is likely to spawn numerous clinical applications. The targeting of protein interaction hubs in microorganisms, particularly when identified hub proteins lack mammalian homologs, may prove to be a powerful strategy in intelligent drug design (Citation23). With the currently available technology, exploring these pathogenic organisms is simple when contrasted with the exploration of more complex genomes.
Many have noted the importance of caution when attempting to extrapolate the topology of the complete protein interactome from the limited datasets obtained from high-throughput Y2H assays (Citation58,Citation61,Citation81). For example, the PPI maps obtained from the two largest S. cerevisiae (Citation24,Citation53) Y2H screens covered less than 10% of the entire yeast protein interactome. Interaction maps obtained from C. elegans and D. melanogaster screens also largely undersample the potential pool of interactions (Citation45,Citation47). While interaction data derived from other sources helps fill in the gaps, such that some estimate the yeast interactome to be 50% complete, much work remains to be done (Citation60). As a result of such limited sampling, the topologies of Y2H-derived scale-free sub-networks cannot be used to make confident generalizations about the topology of whole cellular networks. Nevertheless, they provide an indispensable base upon which to build truly robust networks in the near future.
The Next 25 Years
The ultimate goal of researchers is to combine the Y2H system and other technologies to define the comprehensive structural and functional properties of the protein-protein interactome. The continued application of classic two-hybrid screening techniques will continue to yield new candidate interactors. However, description of a full interactome structure will require much more than the assembly line–style processing of additional ORFs using existing high-throughput Y2H methods, as many proteins remain refractory to use in the classic methods. The accompanying article by Suter et al. (Citation82) summarizes a number of more recently developed technologies suitable for high-throughput screening that are being applied to build additional protein interaction datasets. As for Y2H and older technologies, there will likely be a significant rate of false positives, false negatives, and lack of overlap between studies with these tools. However, the set of refractory proteins with Y2H is likely to overlap only partially with the refractory proteins in the complementary platforms, allowing us to move asymptotically to a completely defined “potential” interaction landscape.
It is important to stress “potential,” rather than confirmed. First, just as high-throughput methodologies provide us with an abundance of PPI data, they also challenge us to devise high-throughput methodologies to validate and analyze such data. Bioinformatic analyses provide one increasingly important approach for evaluating PPI data by assisting to winnow valid from likely spurious potential interactions. For example, bioinformatic analyis by the Ideker group and others have discovered that interactions that are evolutionarily conserved among species (i.e., paralogues of interacting proteins are also known to interact) are more likely to be biologically relevant than interactions detected in a single species (Citation22,Citation83–88). Such an insight not only helps rank Y2H-detected interactions based on their detection in multiple species, but also allows researchers with interests in specific pathways to “model forward,” hypothesizing that orthologs of interactor pairs demonstrated in lower organisms are good candidates for interactors in mammals.
A current goal of bioinformatics researchers is to expand and optimize analytic platforms so that they can incorporate PPI data from all high- and low-throughput approaches, rank these data on reliability, and integrate these data with current information on protein and gene function, expression regulation, and relevance to biological processes. At present, the gold standard remains the independent confirmation and exploration of interaction significance by independent researchers committed to the study of one or more of the interacting proteins. An increasing number of PPI databases, such as the Human Proteome Reference Database (HPRD) (Citation89), systematically curate information from such sources. However, complementary high-throughput screening techniques that do not directly assess PPIs are becoming increasingly informative for PPI screens. One particularly fertile source of such information is provided by medium- and high-throughput genetic studies in lower organisms. Genetic interaction studies detect synthetic lethal relationships, wherein the deletion of two genes in functionally parallel pathways results in a significantly greater loss of viability when compared with the deletion of either individual gene. Progress in the field, such as the development of molecular barcode arrays and increasingly comprehensive deletion mutant sets, has broadened the scope of genetic analysis. Genetic interaction studies in yeast and worms have placed many protein complexes into functional pathways, illuminating the biological significance of PPIs in lower eukaryotes and of orthologous PPIs in humans (Citation90–92). As this and other data are merged into databases such as BioGRID (Citation93) and BIND (Citation94), development of apparently prosaic tools such as standardized nomenclature and reporting guidelines for interactions will be critical (Citation95).
As combined high-throughput methods suggest many new interactions between both well-known and minimally annotated proteins, discovering the meaning of these interactions poses a monumental challenge. Currently, high throughput–derived PPI data can report the number of connections that exist between a protein and its interaction partners, but cannot inform the functional nature of each interaction. There are many potential classes of interaction: inhibition, activation, scaffolding-facilitation, transience or stability, potency, temporal-specificity, subcellular localization-specificity, and others (Citation23). There remain significant knowledge gaps in this area for higher eukaryotes. Hence, accurate modeling and design of networks—the goal of systems biology—is at present most effective in prokaryotes and lower eukaryotes, for which these relations are more readily described. In the next 25 years, the potential for the understanding of human systems is unquestionably vast; as is the potential to improve the human condition.
Competing Interests Statement
The authors declare no competing interests.
Acknowledgments
The authors were supported by National Institutes of Health (NIH) RO1s CA63366 and CA113342; by Tobacco Settlement funding from the State of Pennsylvania (to E.A.G.); by an AACR-Littlefield Award; by NIH core grant no. CA-06927; and support from the Pew Charitable Fund to Fox Chase Cancer Center. We are grateful to members of the Golemis laboratory for critical comments on the manuscript.
Additional information
Funding
References
- McCoy, M.S., J.J.Toole, J.M.Cunningham, E.H.Chang, D.R.Lowy, and R.A.Weinberg. 1983. Characterization of a human colon/lung carcinoma oncogene. Nature302:79–81.
- Schwab, M., K.Alitalo, K.H.Klempnauer, H.E.Varmus, J.M.Bishop, F.Gilbert, G.Brodeur, M.Goldstein, and J.Trent. 1983. Amplified DNA with limited homology to myc cellular oncogene is shared by human neuroblastoma cell lines and a neuroblastoma tumour. Nature305:245–248.
- Capon, D.J., E.Y.Chen, A.D.Levinson, P.H.Seeburg, and D.V.Goeddel. 1983. Complete nucleotide sequences of the T24 human bladder carcinoma oncogene and its normal homologue. Nature302:33–37.
- Schwab, M., K.Alitalo, H.E.Varmus, J.M.Bishop, and D.George. 1983. A cellular oncogene (c-Ki-ras) is amplified, overexpressed, and located within karyotypic abnormalities in mouse adrenocortical tumour cells. Nature303:497–501.
- Fields, S. and O.Song. 1989. A novel genetic system to detect protein-protein interactions. Nature340:245–246.
- Chevray, P.M. and D.Nathans. 1992. Protein interaction cloning in yeast: identification of mammalian proteins that react with the leucine zipper of Jun. Proc. Natl. Acad. Sci. USA89:5789–5793.
- Chien, C.T., P.L.Bartel, R.Sternglanz, and S.Fields. 1991. The two-hybrid system: a method to identify and clone genes for proteins that interact with a protein of interest. Proc. Natl. Acad. Sci. USA88:9578–9582.
- Durfee, T., K.Becherer, P.L.Chen, S.H.Yeh, Y.Yang, A.E.Kilburn, W.H.Lee, and S.J.Elledge. 1993. The retinoblastoma protein associates with the protein phosphatase type 1 catalytic subunit. Genes Dev.7:555–569.
- Estojak, J., R.Brent, and E.A.Golemis. 1995. Correlation of two-hybrid affinity data with in vitro measurements. Mol. Cell. Biol.15:5820–5829.
- Gyuris, J., E.Golemis, H.Chertkov, and R.Brent. 1993. Cdi1, a human G1 and S phase protein phosphatase that associates with Cdk2. Cell75:791–803.
- Vojtek, A.B., S.M.Hollenberg, and J.A.Cooper. 1993. Mammalian Ras interacts directly with the serine/threonine kinase Raf. Cell74:205–214.
- Brent, R. and M.Ptashne. 1981. Mechanism of action of the lexA gene product. Proc. Natl. Acad. Sci. USA78:4204–4208.
- Zervos, A.S., J.Gyuris, and R.Brent. 1993. Mxi1, a protein that specifically interacts with Max to bind Myc-Max recognition sites. Cell72:223–232.
- Golemis, E.A. (Ed.)(Ed.) 2005. Protein-Protein Interactions. CSH Laboratory Press, Cold Spring Harbor, NY.
- Du, W., M.Vidal, J.E.Xie, and N.Dyson. 1996. RBF, a novel RB-related gene that regulates E2F activity and interacts with cyclin E in Drosophila. Genes Dev.10:1206–1218.
- Marsolier, M.C., M.N.Prioleau, and A.Sentenac. 1997. A RNA polymerase III-based two-hybrid system to study RNA polymerase II transcriptional regulators. J. Mol. Biol.268:243–249.
- Marsolier, M.C. and A.Sentenac. 1999. RNA polymerase III-based two-hybrid system. Methods Enzymol.303:411–422.
- Stagljar, I., C.Korostensky, N.Johnsson, and S.te Heesen. 1998. A genetic system based on split-ubiquitin for the analysis of interactions between membrane proteins in vivo. Proc. Natl. Acad. Sci. USA95:5187–5192.
- Serebriiskii, I., J.Estojak, M.Berman, and E.A.Golemis. 2000. Approaches to detecting false positives in yeast two-hybrid systems. BioTechniques28:328–330 332-326.
- Serebriiskii, I., V.Khazak, and E.A.Golemis. 1999. A two-hybrid dual bait system to discriminate specificity of protein interactions. J. Biol. Chem.274:17080–17087.
- Serebriiskii, I.G., O.V.Mitina, J.Chernoff, and E.A.Golemis. 2001. Two-hybrid dual bait system to discriminate specificity of protein interactions in small GTPases. Methods Enzymol.332:277–300.
- Fields, S. 2005. High-throughput two-hybrid analysis. The promise and the peril. FEBS J.272:5391–5399.
- Ito, T., K.Ota, H.Kubota, Y.Yamaguchi, T.Chiba, K.Sakuraba, and M.Yoshida. 2002. Roles for the two-hybrid system in exploration of the yeast protein interactome. Mol. Cell. Proteomics1:561–566.
- Ito, T., T.Chiba, R.Ozawa, M.Yoshida, M.Hattori, and Y.Sakaki. 2001. A comprehensive two-hybrid analysis to explore the yeast protein interactome. Proc. Natl. Acad. Sci. USA98:4569–4574.
- Mrowka, R., A.Patzak, and H.Herzel. 2001. Is there a bias in proteome research?Genome Res.11:1971–1973.
- von Mering, C., R.Krause, B.Snel, M.Cornell, S.G.Oliver, S.Fields, and P.Bork. 2002. Comparative assessment of large-scale data sets of protein-protein interactions. Nature417:399–403.
- Fashena, S.J., I.Serebriiskii, and E.A.Golemis. 2000. The continued evolution of two-hybrid screening approaches in yeast: how to outwit different preys with different baits. Gene250:1–14.
- Osborne, M.A., S.Dalton, and J.P.Kochan. 1995. The yeast tribrid system—genetic detection of trans-phosphorylated ITAM-SH2-interactions. Biotechnology (N. Y.)13:1474–1478.
- Osborne, M.A., G.Zenner, M.Lubinus, X.Zhang, Z.Songyang, L.C.Cantley, P.Majerus, P.Burn, and J.P.Kochan. 1996. The inositol 5′-phosphatase SHIP binds to immunoreceptor signaling motifs and responds to high affinity IgE receptor aggregation. J. Biol. Chem.271:29271–29278.
- Park, Y.W., J.Wilusz, and M.G.Katze. 1999. Regulation of eukaryotic protein synthesis: selective influenza viral mRNA translation is mediated by the cellular RNA-binding protein GRSF-1. Proc. Natl. Acad. Sci. USA96:6694–6699.
- Putz, U., P.Skehel, and D.Kuhl. 1996. A tri-hybrid system for the analysis and detection of RNA--protein interactions. Nucleic Acids Res.24:4838–4840.
- SenGupta, D.J., B.Zhang, B.Kraemer, P.Pochart, S.Fields, and M.Wickens. 1996. A three-hybrid system to detect RNA-protein interactions in vivo. Proc. Natl. Acad. Sci. USA93:8496–8501.
- Wang, Z.F., M.L.Whitfield, T.C.IngledueIII, Z.Dominski, and W.F.Marzluff. 1996. The protein that binds the 3′ end of histone mRNA: a novel RNA-binding protein required for histone pre-mRNA processing. Genes Dev.10:3028–3040.
- Vidal, M., R.K.Brachmann, A.Fattaey, E.Harlow, and J.D.Boeke. 1996. Reverse two-hybrid and one-hybrid systems to detect dissociation of protein-protein and DNA-protein interactions. Proc. Natl. Acad. Sci. USA93:10315–10320.
- Vidal, M., P.Braun, E.Chen, J.D.Boeke, and E.Harlow. 1996. Genetic characterization of a mammalian protein-protein interaction domain by using a yeast reverse two-hybrid system. Proc. Natl. Acad. Sci. USA93:10321–10326.
- Huang, J. and S.L.Schreiber. 1997. A yeast genetic system for selecting small molecule inhibitors of protein-protein interactions in nanodroplets. Proc. Natl. Acad. Sci. USA94:13396–13401.
- Vidal, M. and H.Endoh. 1999. Prospects for drug screening using the reverse two-hybrid system. Trends Biotechnol.17:374–381.
- Young, K., S.Lin, L.Sun, E.Lee, M.Modi, S.Hellings, M.Husbands, B.Ozenberger, and R.Franco. 1998. Identification of a calcium channel modulator using a high throughput yeast two-hybrid screen. Nat. Biotechnol.16:946–950.
- International Human Genome Sequencing Consortium . 2004. Finishing the euchromatic sequence of the human genome. Nature431:931–945.
- Goffeau, A., B.G.Barrell, H.Bussey, R.W.Davis, B.Dujon, H.Feldmann, F.Galibert, J.D.Hoheisel, et al.. 1996. Life with 6000 genes. Science274:546, 563–567.
- McPherson, J.D., M.Marra, L.Hillier, R.H.Waterston, A.Chinwalla, J.Wallis, M.Sekhon, K.Wylie, et al.. 2001. A physical map of the human genome. Nature409:934–941.
- C. elegans Sequencing Consortium . 1998. Genome sequence of the nematode C. elegans: a platform for investigating biology. Science282:2012–2018.
- Adams, M.D., S.E.Celniker, R.A.Holt, C.A.Evans, J.D.Gocayne, P.G.Amanatides, S.E.Scherer, P.W.Li, et al.. 2000. The genome sequence of Drosophila melanogaster. Science287:2185–2195.
- Bartel, P.L., J.A.Roecklein, D.SenGupta, and S.Fields. 1996. A protein linkage map of Escherichia coli bacteriophage T7. Nat. Genet.12:72–77.
- Giot, L., J.S.Bader, C.Brouwer, A.Chaudhuri, B.Kuang, Y.Li, Y.L.Hao, C.E.Ooi, et al.. 2003. A protein interaction map of Drosophila melanogaster. Science302:1727–1736.
- LaCount, D.J., M.Vignali, R.Chettier, A.Phansalkar, R.Bell, J.R.Hesselberth, L.W.Schoenfeld, I.Ota, et al.. 2005. A protein interaction network of the malaria parasite Plasmodium falciparum. Nature438:103–107.
- Li, S., C.M.Armstrong, N.Bertin, H.Ge, S.Milstein, M.Boxem, P.O.Vidalain, J.D.Han, et al.. 2004. A map of the interactome network of the metazoan C. elegans. Science303:540–543.
- Rain, J.C., L.Selig, H.De Reuse, V.Battaglia, C.Reverdy, S.Simon, G.Lenzen, F.Petel, et al.. 2001. The protein-protein interaction map of Helicobacter pylori. Nature409:211–215.
- Rual, J.F., K.Venkatesan, T.Hao, T.Hirozane-Kishikawa, A.Dricot, N.Li, G.F.Berriz, F.D.Gibbons, et al.. 2005. Towards a proteome-scale map of the human protein-protein interaction network. Nature437:1173–1178.
- Stelzl, U., U.Worm, M.Lalowski, C.Haenig, F.H.Brembeck, H.Goehler, M.Stroedicke, M.Zenkner, et al.. 2005. A human protein-protein interaction network: a resource for annotating the proteome. Cell122:957–968.
- Uetz, P., Y.A.Dong, C.Zeretzke, C.Atzler, A.Baiker, B.Berger, S.V.Rajagopala, M.Roupelieva, et al.. 2006. Herpesviral protein networks and their interaction with the human proteome. Science311:239–242.
- Fromont-Racine, M., A.E.Mayes, A.Brunet-Simon, J.C.Rain, A.Colley, I.Dix, L.Decourty, N.Joly, et al.. 2000. Genome-wide protein interaction screens reveal functional networks involving Sm-like proteins. Yeast17:95–110.
- Uetz, P., L.Giot, G.Cagney, T.A.Mansfield, R.S.Judson, J.R.Knight, D.Lockshon, V.Narayan, et al.. 2000. A comprehensive analysis of protein-protein interactions in Saccharomyces cerevisiae. Nature403:623–627.
- Finley, R.L., Jr. and R.Brent. 1994. Interaction mating reveals binary and ternary connections between Drosophila cell cycle regulators. Proc. Natl. Acad. Sci. USA91:12980–12984.
- Ma, H., S.Kunes, P.J.Schatz, and D.Botstein. 1987. Plasmid construction by homologous recombination in yeast. Gene58:201–216.
- Sanger, F., S.Nicklen, and A.R.Coulson. 1977. DNA sequencing with chain-terminating inhibitors. Proc. Natl. Acad. Sci. USA74:5463–5467.
- Grigoriev, A. 2003. On the number of protein-protein interactions in the yeast proteome. Nucleic Acids Res.31:4157–4161.
- Han, J.D., D.Dupuy, N.Bertin, M.E.Cusick, and M.Vidal. 2005. Effect of sampling on topology predictions of protein-protein interaction networks. Nat. Biotechnol.23:839–844.
- Walhout, A.J., S.J.Boulton, and M.Vidal. 2000. Yeast two-hybrid systems and protein interaction mapping projects for yeast and worm. Yeast17:88–94.
- Hart, G.T., A.K.Ramani, and E.M.Marcotte. 2006. How complete are current yeast and human protein-interaction networks?Genome Biol.7:120.
- Parrish, J.R., K.D.Gulyas, R.L., and Finley, Jr. 2006. Yeast two-hybrid contributions to interactome mapping. Curr. Opin. Biotechnol.17:387–393.
- Stanyon, C.A., G.Liu, B.A.Mangiola, N.Patel, L.Giot, B.Kuang, H.Zhang, J.Zhong, and R.L.FinleyJr. 2004. A Drosophila protein-interaction map centered on cell-cycle regulators. Genome Biol.5:R96.
- Gavin, A.C., M.Bosche, R.Krause, P.Grandi, M.Marzioch, A.Bauer, J.Schultz, J.M.Rick, et al.. 2002. Functional organization of the yeast proteome by systematic analysis of protein complexes. Nature415:141–147.
- Ho, Y., A.Gruhler, A.Heilbut, G.D.Bader, L.Moore, S.L.Adams, A.Millar, P.Taylor, et al.. 2002. Systematic identification of protein complexes in Saccharomyces cerevisiae by mass spectrometry. Nature415:180–183.
- Cho, R.J., M.J.Campbell, E.A.Winzeler, L.Steinmetz, A.Conway, L.Wodicka, T.G.Wolfsberg, A.E.Gabrielian, et al.. 1998. A genome-wide transcriptional analysis of the mitotic cell cycle. Mol. Cell2:65–73.
- Hughes, T.R., M.J.Marton, A.R.Jones, C.J.Roberts, R.Stoughton, C.D.Armour, H.A.Bennett, E.Coffey, et al.. 2000. Functional discovery via a compendium of expression profiles. Cell102:109–126.
- Mewes, H.W., D.Frishman, U.Guldener, G.Mannhaupt, K.Mayer, M.Mokrejs, B.Morgenstern, M.Munsterkotter, et al.. 2002. MIPS: a database for genomes and protein sequences. Nucleic Acids Res.30:31–34.
- Tong, A.H., M.Evangelista, A.B.Parsons, H.Xu, G.D.Bader, N.Page, M.Robinson, S.Raghibizadeh, et al.. 2001. Systematic genetic analysis with ordered arrays of yeast deletion mutants. Science294:2364–2368.
- Enright, A.J., I.Iliopoulos, N.C.Kyrpides, and C.A.Ouzounis. 1999. Protein interaction maps for complete genomes based on gene fusion events. Nature402:86–90.
- Marcotte, E.M., M.Pellegrini, H.L.Ng, D.W.Rice, T.O.Yeates, and D.Eisenberg. 1999. Detecting protein function and protein-protein interactions from genome sequences. Science285:751–753.
- Overbeek, R., M.Fonstein, M.D’Souza, G.D.Pusch, and N.Maltsev. 1999. The use of gene clusters to infer functional coupling. Proc. Natl. Acad. Sci. USA96:2896–2901.
- Pellegrini, M., E.M.Marcotte, M.J.Thompson, D.Eisenberg, and T.O.Yeates. 1999. Assigning protein functions by comparative genome analysis: protein phylogenetic profiles. Proc. Natl. Acad. Sci. USA96:4285–4288.
- Huynen, M., B.Snel, W.LatheIII, and P.Bork. 2000. Predicting protein function by genomic context: quantitative evaluation and qualitative inferences. Genome Res.10:1204–1210.
- Marcotte, E.M., M.Pellegrini, M.J.Thompson, T.O.Yeates, and D.Eisenberg. 1999. A combined algorithm for genome-wide prediction of protein function. Nature402:83–86.
- Friedman, A. and N.Perrimon. 2007. Genetic screening for signal transduction in the era of network biology. Cell128:225–231.
- Han, J.D., N.Bertin, T.Hao, D.S.Goldberg, G.F.Berriz, L.V.Zhang, D.Dupuy, A.J.Walhout, et al.. 2004. Evidence for dynamically organized modularity in the yeast protein-protein interaction network. Nature430:88–93.
- Friedman, A. and N.Perrimon. 2006. A functional RNAi screen for regulators of receptor tyrosine kinase and ERK signalling. Nature444:230–234.
- Jeong, H., S.P.Mason, A.L.Barabasi, and Z.N.Oltvai. 2001. Lethality and centrality in protein networks. Nature411:41–42.
- Strogatz, S.H. 2001. Exploring complex networks. Nature410:268–276.
- Winzeler, E.A., D.D.Shoemaker, A.Astromoff, H.Liang, K.Anderson, B.Andre, R.Bangham, R.Benito, et al.. 1999. Functional characterization of the S. cerevisiae genome by gene deletion and parallel analysis. Science285:901–906.
- Gandhi, T.K., J.Zhong, S.Mathivanan, L.Karthick, K.N.Chandrika, S.S.Mohan, S.Sharma, S.Pinkert, et al.. 2006. Analysis of the human protein interactome and comparison with yeast, worm and fly interaction datasets. Nat. Genet.38:285–293.
- Suter, B., S.Kittanakom, and I.Stagljar. Interactive proteomics: what lies ahead?BioTechniques44: 681–691.
- Deane, C.M., L.Salwinski, I.Xenarios, and D.Eisenberg. 2002. Protein interactions: two methods for assessment of the reliability of high throughput observations. Mol. Cell. Proteomics1:349–356.
- Kelley, B.P., B.Yuan, F.Lewitter, R.Sharan, B.R.Stockwell, and T.Ideker. 2004. PathBLAST: a tool for alignment of protein interaction networks. Nucleic Acids Res.32:W83–W88.
- Stuart, J.M., E.Segal, D.Koller, and S.K.Kim. 2003. A gene-coexpression network for global discovery of conserved genetic modules. Science302:249–255.
- Yu, H., N.M.Luscombe, H.X.Lu, X.Zhu, Y.Xia, J.D.Han, N.Bertin, S.Chung, et al.. 2004. Annotation transfer between genomes: protein-protein interologs and protein-DNA regulogs. Genome Res.14:1107–1118.
- Bandyopadhyay, S., R.Sharan, and T.Ideker. 2006. Systematic identification of functional orthologs based on protein network comparison. Genome Res.16:428–435.
- Suthram, S., T.Sittler, and T.Ideker. 2005. The Plasmodium protein network diverges from those of other eukaryotes. Nature438:108–112.
- Peri, S., J.D.Navarro, T.Z.Kristiansen, R.Amanchy, V.Surendranath, B.Muthusamy, T.K.Gandhi, K.N.Chandrika, et al.. 2004. Human protein reference database as a discovery resource for proteomics. Nucleic Acids Res.32:D497–D501.
- Collins, S.R., K.M.Miller, N.L.Maas, A.Roguev, J.Fillingham, C.S.Chu, M.Schuldiner, M.Gebbia, et al.. 2007. Functional dissection of protein complexes involved in yeast chromosome biology using a genetic interaction map. Nature446:806–810.
- Pierce, S.E., E.L.Fung, D.F.Jaramillo, A.M.Chu, R.W.Davis, C.Nislow, and G.Giaever. 2006. A unique and universal molecular barcode array. Nat. Methods3:601–603.
- Zhong, W. and P.W.Sternberg. 2006. Genome-wide prediction of C. elegans genetic interactions. Science (New York, N. Y.)311:1481–1484.
- Breitkreutz, B.J., C.Stark, T.Reguly, L.Boucher, A.Breitkreutz, M.Livstone, R.Oughtred, D.H.Lackner, et al.. 2008. The BioGRID Interaction Database: 2008 update. Nucleic Acids Res.36:D637–D640.
- Alfarano, C., C.E.Andrade, K.Anthony, N.Bahroos, M.Bajec, K.Bantoft, D.Betel, B.Bobechko, et al.. 2005. The Biomolecular Interaction Network Database and related tools 2005 update. Nucleic Acids Res.33:D418–D424.
- Orchard, S., L.Montechi-Palazzi, E.W.Deutsch, P.A.Binz, A.R.Jones, N.Paton, A.Pizarro, D.M.Creasy, et al.. 2007. Five years of progress in the Standardization of Proteomics Data 4th Annual Spring Workshop of the HUPO-Proteomics Standards Initiative, April 23-25, 2007 Ecole Nationale Superieure (ENS), Lyon, France. Proteomics7:3436–3440.