Abstract
Background: The achievement of the human genome project provides a basis for the systematic study of the human genome from evolutionary history to disease-specific medicine. With the explosive growth of biological data, a growing number of biological databases are being established to support human-related research. Objective: The main objective of our study is to store, organize and share data in a structured and searchable manner. In short, we have planned the future development of new features in the database research area. Materials & methods: In total, we collected and integrated 680 human databases from scientific published work. Multiple options are presented for accessing the data, while original links and short descriptions are also presented for each database. Results & discussion: We have provided the latest collection of human research databases on a single platform with six categories: DNA database, RNA database, protein database, expression database, pathway database and disease database. Conclusion: Taken together, our database will be useful for further human research study and will be modified over time. The database has been implemented in PHP, HTML, CSS and MySQL and is available freely at https://habdsk.org/database.php.
Lay abstract
We have compiled the most recent collection of human research datasets into six categories – DNA database, RNA database, protein database, expression database, pathway database and disease database – on a single platform. In all, 680 human datasets were acquired and incorporated from scientifically published studies. There are several ways to retrieve the data, as well as original links and short descriptions for each database. The primary goal of our research is to store, organize and exchange data in an organized and searchable format. In brief, we have planned the future development of additional features in the database. Our database will be beneficial for future human research studies and will be updated throughout time. We firmly believe that every researcher should have access to essential biological databases, so we have gathered a collection of human-related databases that are regularly used and currently available but have not previously been presented in such a simple and welcoming manner.
Introduction
Biological databases are libraries of life sciences information that provide access to genomic data [Citation1–3] and analysis of genetic diseases, genetic genealogy or genetic fingerprinting for criminology [Citation4], physical, chemical and biological information on sequence, domain structure, function, three-dimensional structure and protein–protein interactions [Citation5,Citation6], relationships between medical conditions, symptoms and medications [Citation7], and information on cell signaling pathways [Citation8], representing a great contribution by the scientific community. Many databases have been published in this research area, including the Kyoto Encyclopedia of Genes and Genomes pathway [Citation9] BiGG Models [Citation10], Database Commons, MiST 3.0 [Citation11] and Pathway Commons databases [Citation12], which are databases that contain biological pathways for metabolic, signaling and regulatory pathway analysis. The DNA Data Bank of Japan [Citation13], GenBank [Citation14], the European Nucleotide Archive [Citation15] and Circadian Gene DataBase (CGDB) [Citation16] are DNA databases that can be used for the analysis of genomic information [Citation17], such as genetic diseases, genetic genealogy or genetic fingerprinting for criminology. The Eukaryotic Linear Motif database [Citation18], Protein Data Bank in Europe [Citation19], Database of Phospho-sites in Animals and Fungi [Citation20] and the Conserved Domains Database [Citation21] are protein databases that have been constructed from physical, chemical and biological information on proteins’ sequence, domain structure, function, three-dimensional structure and protein–protein interactions. miRTarBase [Citation22], RNA Central [Citation23] and NONCODE [Citation24] include a huge group of eukaryotic RNAs involved in the regulation of gene expression. CancerGeneNet [Citation25], Online Mendelian Inheritance in Man [Citation26] and The Cancer Genome Atlas [Citation27] provide information about the relationships between medical conditions, symptoms and medications. Expression Atlas [Citation28], ArrayExpress [Citation29] and BioExpress [Citation30] are expression databases constituting an international public repository that archives and freely distributes high-throughput gene expression and other functional genomics datasets. Biological databases contain large quantities of omics data; according to the 2020 Molecular Biology Database Collection study in the journal Nucleic Acids Research, a total of 1637 databases are publicly accessible online [Citation31–33], with a broad classification range. Several articles have been published in well-known journals relating to different organisms and components, such as the collection of 74 databases listed by Zou et al. [Citation34], Previously, we gathered and published 59 COVID-19-related databases [Citation35]. A comprehensive collection of the human databases is needed for the research community. Therefore, for more general-purpose and easy access, we have collected all the commonly used and currently available human databases to one platform, DataBases relevant to Human Research (DBHR) in which users can get the required category via a single click; for example, if a user needs a DNA database, they can directly get all 126 DNA databases on a single click and can choose the needed database. This is easier than searching each one via Google, and only updated database links have been provided (in the form of a table) [Citation34,Citation36–39]. As database classification based on data type is insightful, we allocate one major category to each database, although a single category can lead to multiple databases. The emphasis is on databases classified as DNA database, RNA database, protein database, expression database, pathway database and disease database. A comparison table of our work with previously published literature is shown in , which includes the category of the published work number of databases, the form of the data, PubMed reference number, year of publication and journal name. Furthermore, the DBHR can be explored in three ways: it can be searched either by clicking on the name or on the picture or by entering the name of the database in the search bar.
Table 1. Comparison of DBHR with other published work.
Materials & methods
Construction of DBHR
In this study we mainly focused on the collection of human databases. To avoid missing data, we used several keywords in PubMed [Citation43] for example, ‘human database’, ‘biological databases’, ‘database for human’ and have combined each and every category with major keywords such as ‘human protein databases’, ‘human DNA databases’ and so on (). We also manually collected the latest human databases from the journal Nucleic Acids Research [Citation44], which is the cutting-edge research journal on databases. After removing broken links, programming platforms including PhP, MySQL, HTML, CSS and JavaScript were used to construct DBHR (). By this method, we have provided a comparable human research database to the scientific community that is easy to operate and will be updated over time.
Database classification
Many articles have been published in well-known journals () [Citation32,Citation40–42,Citation45–47], which have collected databases relating to different organisms and components. For example, Zou et al. [Citation34] collected and published a list of 74 human databases in the journal Genomics, Proteomics and Bioinformatics, and Prakash et al. [Citation48] collected a list of 24 fungi databases which was published in the Journal of Clinical Microbiology. However, a comprehensive human database is also needed for the research community to sort and save all the human data for future researchers. Further, published work has collected the databases and has presented them in the form of a table, while in our work we have provided the table as well as the database of the databases, and have the comparison table () which shows a clear improvement. According to the diverse purposes of biological databases and published literature [Citation34,Citation49–51], we have classified the human-related biological databases into the following six categories.
DNA databases
The DNA databases provide access to genomic data contributed by the scientific community from more than 900 species whose sequencing and mapping is either completed or ongoing. There are now more than 57 completed microbial genomes and 245 reference sequences for eukaryotic organelles available in different DNA databases [Citation1]. DNA data can be used for the analysis of genetic diseases, genetic genealogy or genetic fingerprinting for criminology [Citation4]. Some databases allow for the management of DNA data from specific species [Citation34], such as DNA Data Bank of Japan [Citation13], GenBank [Citation14], the European Nucleotide Archive [Citation15] and CGDB [Citation16].
RNA databases
It is well known that only a limited amount of the human genome is transcribed into mRNAs, while the vast majority of the genome is transcribed into noncoding RNAs that do not code for proteins, these include microRNAs, nucleolar RNAs, piwiRNAs and long noncoding RNAs [Citation34]. An example of an RNA database is the microRNA database miRBase, which was first released in 2002 and is currently the most complete resource for information on microRNAs, a diverse group of eukaryotic RNAs involved in the regulation of gene expression.
Protein databases
A protein database is a collection of data that has been constructed from physical, chemical and biological information on proteins’ sequence, domain structure, function, three-dimensional structure and protein–protein interactions [Citation52]. The purpose of the protein databases is to arrange and annotate protein structures, providing the biological community with valuable access to experimental evidence, an example is the Protein Data Bank [Citation53]. Published scientific databases such as Antibodies Chemically Defined [Citation54], the Plant Protein Phosphorylation Database[Citation55] and the Structural Classification of Proteins database [Citation56] are well-known databases in the protein research area.
Disease databases
Disease databases provide information about the relationships among medical conditions, symptoms and medications [Citation7]. Comprehensive disease classification, integration and annotation are crucial to biomedical discovery. There is a variety of well-known and referenced databases that include a set of human genes and genetic phenotypes [Citation57], including The Cancer Genome Atlas [Citation27] and the International Cancer Genome Consortium data portal [Citation58].
Pathway databases
Pathway databases contain biological pathways for metabolic, signaling and regulatory pathway analysis. Several databases contain information on cell signaling that has been developed in accordance with data access and analysis methodologies [Citation8] and have been published in this research area. Examples include the Kyoto Encyclopedia of Genes and Genomes pathway database [Citation9], BiGG Models database [Citation10], MiST 3.0 [Citation11] and Pathway Commons [Citation12].
Expression databases
The Gene Expression Omnibus database is an international public repository that archives and freely distributes high-throughput gene expression and other functional genomics data sets [Citation59]. Translation makes it easier to understand biological processes under normal or disease-related conditions. Researchers trying to identify similarities and differences between organisms at the molecular level need resources to collect data on multi-organism tissue expression [Citation60].
Results & discussion
Statistics of DBHR
In this study we have curated the year-wise and category-wise databases, have modified or deleted all the outdated, broken and non-assessable database links (Supplementary Table 1), and have provided new and updated human databases (Supplementary Table 2), thus demonstrating the rapid growth of biological databases (A). In addition, the category-wise development of the DBHR is demonstrated by the different data categories (B) which represent tremendous growth and achievement for the scientific community, due to the rapid growth of these results. C shows the distribution of the categories as percentages.
Usage of DBHR
The DBHR has been developed to make searching easy and user-friendly. For easier and faster searching, three options are provided for finding a human database. First, DBHR can be browsed by the name of the category (A) or related image (B), a new feature of accessing the database that has not been provided before in such database fields. This search will lead to the category list page, and a brief overview with the original link of the required search will be accessed by clicking the needed database. Further, for database search, users can enter the name of the required database in the search bar (C). In C the BIOCYC database is used as an example from the disease databases to make it easier for users, some relevant work is shown in Table 2.
(A) Browsing by clicking the name. (B) Browsing by clicking the image. (C) Browsing using the search bar.
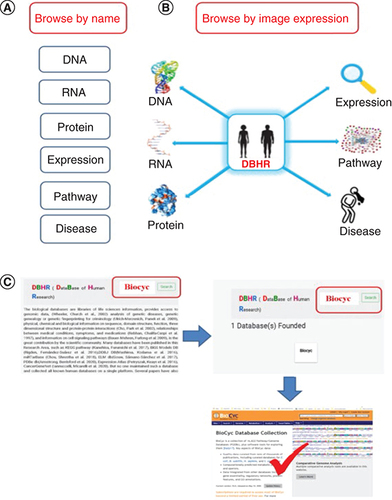
Table 2. Some relevant research work in the field of databases and resources.
Conclusion
The main objective of our study was to store, organize and share data in a structured and searchable manner, with the aim of facilitating the retrieval and visualization of data for humans. We strongly believe that every researcher should have access to important biological databases, we are therefore bringing together a set of human-related databases that are commonly used and currently available and have not previously been published in such an easy and user-friendly way. As database classification based on data type is insightful, we allocated one major category to each database, although a single category can lead to multiple databases. The emphasis is on databases classified as DNA database, RNA database, protein database, expression database, pathway database or disease database. We provided access to 680 up-to-date human databases in a fast, easy and user-friendly way, DBHR can be searched either by clicking on the name of the category or the category image, and also by entering the name of the database in the search bar. The facility will be upgraded with the passage of time.
Future perspective
According to the huge and rapid increase of human-related research databases, which cannot be handled without computational databases, and is rapidly becoming a critical component of modern biology. In any case, database research is always the initial step in all biological study, nevertheless, the utilization of multiple databases also aids researchers in understanding the evolution, structure, and function of all proteins. However, for further research, a comprehensive and large-scale database is required. As a result, as time passes, we will strive to deliver the most up-to-date human research databases with more specific categorization to the scientific community. Furthermore, as science progresses, we will offer some advanced searching in the near future.
Our facility, DBHR (DataBases relevant to Human Research) aims to provide useful insights for researchers with the gathering of all relevant human data to one platform.
DBHR provides access to data from sources that are difficult to locate.
DBHR gives details that may not have been published before in such an easy and user-friendly way in the open literature.
DBHR also monitors and updates dead and broken databases to ensure that only current information is presented.
Author contributions
Dr. Shahid Ullah designed and supervised the project with Dr. Tianshun Gao's assistance, performed data analysis. Farhan Ullah, Wajeeha Rahman, Muhmmad Ijaz and Gulzar Ahmad contributed to data analysis. Shahid Ullah wrote the manuscript. All authors reviewed the manuscript.
Acknowledgments
To avoid future conflict, we would like to say that our database is uploaded on S Khan Lab website http://www.habdsk.org/database.php, so that we have provided some content in this article. A warm welcome to Ramish Durran Khan, who joined us on 15 December 2021.
Supplementary data
To view the supplementary data that accompany this paper please visit the journal website at: www.tandfonline.com/doi/suppl/10.4155/fsoa-2021-0101
Financial & competing interests disclosure
This project is supported by National Natural Science Foundation of China (32100434) and Shenzhen’s introduction of talents and research start-up (392020). The authors have no other relevant affiliations or financial involvement with any organization or entity with a financial interest in or financial conflict with the subject matter or materials discussed in the manuscript apart from those disclosed.
No writing assistance was utilized in the production of this manuscript.
References
- WheelerDL , ChurchDM , LashAEet al.Database resources of the National Center for Biotechnology Information: 2002 update. Nucleic Acids Res.30(1), 13–16 (2002).
- KhansaJM , AnandV , SoomroK , JunaidM. Tremendous contribution of Dr. Shahid Ullah to scientific community during COVID-19 pandemic in the form of scientific research. J. Clin. Med. Res.2(5), 1–7 (2020).
- BourneP. Will a biological database be different from a biological journal?PLoS Comput. Biol.1(3), e34 (2005).
- Ulrich-MerzenichG , PanekD , ZeitlerH , WagnerH , VetterH. New perspectives for synergy research with the ‘omic’ technologies. Phytomedicine16(6–7), 495–508 (2009).
- RezaeiJ , RouzbehanY , ZahedifarM , FazaeliH. Effects of dietary substitution of maize silage by amaranth silage on feed intake, digestibility, microbial nitrogen, blood parameters, milk production and nitrogen retention in lactating Holstein cows. Anim. Feed Sci. Tech.202, 32–41 (2015).
- HelmyM , Crits-ChristophA , BaderGD. Ten simple rules for developing public biological databases. PLoS Comput. Biol.12(11), e1005128 (2016).
- RebhanM , Chalifa-CaspiV , PriluskyJ , LancetD. GeneCards: integrating information about genes, proteins and diseases. Trends Genet.13(4), 163 (1997).
- Bauer-MehrenA , FurlongLI , SanzF. Pathway databases and tools for their exploitation: benefits, current limitations and challenges. Mol. Syst. Biol.5(1), 290 (2009).
- KanehisaM , FurumichiM , TanabeM , SatoY , MorishimaK. KEGG: new perspectives on genomes, pathways, diseases and drugs. Nucleic Acids Res.45(D1), D353–D361 (2017).
- KingZA , LuJ , DrägerAet al.BiGG Models: a platform for integrating, standardizing and sharing genome-scale models. Nucleic Acids Res.44(D1), D515–D522 (2016).
- GumerovVM , OrtegaDR , AdebaliO , UlrichLE , ZhulinIB. MiST 3.0: an updated microbial signal transduction database with an emphasis on chemosensory systems. Nucleic Acids Res.48(D1), D459–D464 (2020).
- BlinovML , SchaffJC , RuebenackerOet al.Pathway Commons at Virtual Cell: use of pathway data for mathematical modeling. Bioinformatics30(2), 292–294 (2014).
- MashimaJ , KodamaY , KosugeTet al.DNA Data Bank of Japan (DDBJ) progress report. Nucleic Acids Res.44(D1), D51–D57 (2016).
- BensonDA , Karsch-MizrachiI , LipmanDJ , OstellJ , SayersEW. GenBank. Nucleic Acids Res.39(Suppl. 1), D32–D37 (2010).
- ToribioAL , AlakoB , AmidCet al.European Nucleotide Archive in 2016. Nucleic Acids Res.45(D1), D32–D36 (2017).
- LiS , ShuiK , ZhangYet al.CGDB: a database of circadian genes in eukaryotes. Nucleic Acids Res.45(D1), D397–D403 (2017).
- SchattnerP. Automated querying of genome databases. PLoS Comput. Biol.3(1), e1 (2007).
- GouwM , Sámano-SánchezH , Van RoeyK , DiellaF , GibsonTJ , DinkelH. Exploring short linear motifs using the ELM database and tools. Curr. Protoc. Bioinformatics58(1), 8.22.21–28.22.35 (2017).
- ArmstrongDR , BerrisfordJM , ConroyMJet al.PDBe: improved findability of macromolecular structure data in the PDB. Nucleic Acids Res.48(D1), D335–D343 (2020).
- UllahS , LinS , XuYet al.dbPAF: an integrative database of protein phosphorylation in animals and fungi. Sci. Rep.6, 23534 (2016).
- Marchler-BauerA , DerbyshireMK , GonzalesNRet al.CDD: NCBI’s conserved domain database. Nucleic Acids Res.43(D1), D222–D226 (2015).
- ChouC-H , ShresthaS , YangC-Det al.miRTarBase update 2018: a resource for experimentally validated microRNA-target interactions. Nucleic Acids Res.46(D1), D296–D302 (2018).
- RNAcentralConsortium. RNAcentral: an international database of ncRNA sequences. Nucleic Acids Res.43(D1), D123–D129 (2015).
- LiuC , BaiB , SkogerbøGet al.NONCODE: an integrated knowledge database of non-coding RNAs. Nucleic Acids Res.33(Suppl. 1), D112–D115 (2005).
- IannuccelliM , MicarelliE , SurdoPLet al.CancerGeneNet: linking driver genes to cancer hallmarks. Nucleic Acids Res.48(D1), D416–D421 (2020).
- AmbergerJS , BocchiniCA , SchiettecatteF , ScottAF , HamoshA. OMIM.org: Online Mendelian Inheritance in Man (OMIM®), an online catalog of human genes and genetic disorders. Nucleic Acids Res.43(D1), D789–D798 (2015).
- DiJia SL , LiD , XueH , YangD , LiuY. Mining TCGA database for genes of prognostic value in glioblastoma microenvironment. Aging (Albany NY)10(4), 592 (2018).
- PetryszakR , KeaysM , TangYAet al.Expression Atlas update – an integrated database of gene and protein expression in humans, animals and plants. Nucleic Acids Res.44(D1), D746–D752 (2016).
- ParkinsonH , KapusheskyM , ShojatalabMet al.ArrayExpress – a public database of microarray experiments and gene expression profiles. Nucleic Acids Res.35(Suppl. 1), D747–D750 (2007).
- StraussA , FendrichG , JahnkeW. Efficient uniform labeling of proteins expressed in baculovirus-infected insect cells using BioExpress® 2000 (Insect Cell) Medium (2019). www.eurisotop.com/efficient-uniform-labeling-proteins-expressed-baculovirus-infected-insect-cells-using-bioexpressr
- RigdenDJ , FernándezXM. The 2018 Nucleic Acids Research database issue and the online molecular biology database collection. Nucleic Acids Res.46(D1), D1–D7 (2018).
- RigdenDJ , FernándezXM. The 27th annual Nucleic Acids Research database issue and molecular biology database collection. Nucleic Acids Res.48(D1), D1–D8 (2020).
- RigdenDJ , FernándezXM. The 2021 Nucleic Acids Research database issue and the online molecular biology database collection. Nucleic Acids Res.49(D1), D1–D9 (2021).
- ZouD , MaL , YuJ , ZhangZ. Biological databases for human research. Genomics Proteomics Bioinformatics13(1), 55–63 (2015).
- UllahS , UllahA , RahmanWet al.An innovative user-friendly platform for COVID-19 pandemic databases and resources. Comput. Methods Programs Biomed. Update1, 100031 (2021).
- BolserDM , ChibonP-Y , PalopoliNet al.MetaBase – the wiki-database of biological databases. Nucleic Acids Res.40(D1), D1250–D1254 (2012).
- BrazasMD , YimDS , YamadaJT , OuelletteBF. The 2011 bioinformatics links directory update: more resources, tools and databases and features to empower the bioinformatics community. Nucleic Acids Res.39(Suppl. 2), W3–W7 (2011).
- ChenC , HuangH , WuCH. Protein bioinformatics databases and resources. Methods Mol. Biol.1558, 3–39 (2017).
- RigdenDJ , Fernández-SuárezXM , GalperinMY. The 2016 database issue of Nucleic Acids Research and an updated molecular biology database collection. Nucleic Acids Res.44(D1), D1–D6 (2016).
- XuD , XuY. Protein databases on the internet. Curr. Protoc. Mol. Biol.2004, Unit 19.4 (2004).
- BaderGD , CaryMP , SanderC. Pathguide: a pathway resource list. Nucleic Acids Res.34(Suppl. 1), D504–D506 (2006).
- HarperR. Access to DNA and protein databases on the internet. Curr. Opin. Biotechnol.5(1), 4–18 (1994).
- National Library of Medicine. PubMed. https://pubmed.ncbi.nlm.nih.gov/
- Stoddard JSaB. Nucleic Acids Research. (2021).
- XuD. Protein databases on the internet. Curr. Protoc. Protein Sci.2012 , Unit 2.6 (2012).
- CelisJE , ØstergaardM , JensenNA , GromovaI , RasmussenHH , GromovP. Human and mouse proteomic databases: novel resources in the protein universe. FEBS Lett.430(1–2), 64–72 (1998).
- UllahS , RahmanW , UllahF , AhmadG , IjazM , GaoT. DBPR: DataBase of Plant Research. (2021).
- PrakashPY , IrinyiL , HallidayC , ChenS , RobertV , MeyerW. Online databases for taxonomy and identification of pathogenic fungi and proposal for a cloud-based dynamic data network platform. J. Clin. Microbiol.55(4), 1011–1024 (2017).
- UllahS , UllahF , RahmanwIM , AhmadG , UllahW. EDBCO-19: Emergency Data Base of COVID-19. J. Clin. Med. Res.2(4), 1–4 (2020).
- AdlSM , SimpsonAG , LaneCEet al.The revised classification of eukaryotes. J. Eukaryot. Microbiol.59(5), 429–514 (2012).
- SantusE , MarinoN , CirilloDet al.Artificial intelligence-aided precision medicine for COVID-19: strategic areas of research and development. J. Med. Internet Res.23(3), e22453 (2021).
- ShinW-H , KumazawaK , ImaiK , HirokawaT , KiharaD. Current challenges and opportunities in designing protein–protein interaction targeted drugs. Adv. Appl. Bioinform. Chem.13, 11–25 (2020).
- UddinH , RahmanA , KhanRet al.Effect of cotton seed cake on cattle milk yield and composition at Livestock Research and Development Station Surezai, Peshawar, Pakistan. Pak. J. Nutr.12(5), 468 (2013).
- LimaWC , GasteigerE , MarcatiliP , DuekP , BairochA , CossonP. The ABCD database: a repository for chemically defined antibodies. Nucleic Acids Res.48(D1), D261–D264 (2020).
- YaoQ , XuD. Bioinformatics analysis of protein phosphorylation in plant systems biology using P3DB. Methods Mol. Biol.1558, 127–138 (2017).
- LoConte L , AileyB , HubbardTJ , BrennerSE , MurzinAG , ChothiaC. SCOP: a structural classification of proteins database. Nucleic Acids Res.28(1), 257–259 (2000).
- HamoshA , ScottAF , AmbergerJS , BocchiniCA , MckusickVA. Online Mendelian Inheritance in Man (OMIM), a knowledgebase of human genes and genetic disorders. Nucleic Acids Res.33(Suppl. 1), D514–D517 (2005).
- ZhangJ , BaranJ , CrosAet al.International Cancer Genome Consortium Data Portal – a one-stop shop for cancer genomics data. Database (Oxford)2011, bar026(.2011).
- CloughE , BarrettT. The Gene Expression Omnibus database. Methods Mol. Biol.1418, 93–110 (2016).
- PalascaO , SantosA , StolteC , GorodkinJ , JensenLJ. TISSUES 2.0: an integrative web resource on mammalian tissue expression. Database (Oxford)2018, bay003(.2018).