Abstract
Coronavirus main protease (3CLpro), a special cysteine protease in coronavirus family, is highly desirable in the life cycle of coronavirus. Here, molecular docking, ADMET pharmacokinetic profiles and molecular dynamics (MD) simulation were performed to develop specific 3CLpro inhibitor. The results showed that the 137 compounds originated from Chinese herbal have good binding affinity to 3CLpro. Among these, Cleomiscosin C, (+)-Norchelidonine, Protopine, Turkiyenine, Isochelidonine and Mallotucin A possessed prominent drug-likeness properties. Cleomiscosin C and Turkiyenine exhibited excellent pharmacokinetic profiles. Furthermore, the complex of Cleomiscosin C with SARS-CoV-2 main protease presented high stability. The findings in this work indicated that Cleomiscosin C is highly promising as a potential 3CLpro inhibitor, thus facilitating the development of effective drugs for COVID-19.
Plain Language Summary
In this work, computer aided drug design technology was used to study the main protease 3CLpro of novel coronavirus, and functional small molecules with inhibitory effects on novel coronavirus were screened from the compound library of natural products. The results showed that Cleomiscosin C is highly promising as a potential 3CLpro inhibitor with prominent binding affinity, pharmacokinetic profiles and stability.
As a new type of positive strand RNA virus β-Coronavirus [Citation1], SARS-CoV-2 is still characterized by high mutation rate. People infected with this virus will have fever, dry cough, fatigue, shortness of breath, and other symptoms [Citation2]. According to “Our World in Data” from Oxford University [Citation3], a total of 12,123,516,491 doses of novel coronavirus vaccine have been administered worldwide so far, with a vaccination rate of 66.72%. Nevertheless, many virus variants have occurred due to the high mutation characteristics of coronavirus [Citation4], thus greatly reducing the effect of antiviral drugs and vaccines. Therefore, developing novel antiviral drugs and vaccines is extremely significant.
At present, oral anti COVID-19 drugs are mainly divided into 3CLpro inhibitors and RNA-dependent-polymerase (RdRp) inhibitors, which are developed for different key targets of the virus life cycle. For example, Paxlovid developed by Pfizer is a 3CLpro inhibitor. Remdesivir, the first officially approved COVID-19 therapeutic drug by FDA and Molnupiravir are both the RdRp inhibitors. In addition, Dasabuvir was found to be a potential molecule for both PLpro and 3CLpro throughout molecular docking, MD simulation and MM/PBSA analysis within 53 FDA approved antiviral drugs [Citation5].
3-Chymotrypsin like protease (3CLpro) [Citation6,Citation7] is a homodimeric cysteine protease consisting of 306 amino acids and 3 domains, which can cleave coronavirus polyproteins at 11 conservative sites [Citation8]. As shown in , antiparallel β-barrel is the main secondary structure of domains I (residues 8–101) and II (residues 102-184) [Citation9,Citation10]. Domain III is an additional helical domain, its aggregation triggered the dimerization of 3CLpro, resulting in a mature dimer structure. Domain III is connected to domain II by long linker group (residues 185–200). The first seven residues from the N-terminus side are forming the N-finger, which is believed to have a significant role both in dimerization and in establishing proteolytic activity [Citation11]. The viral RNA was initially translated into polyproteins, and then cut into multiple active functional proteins by 3CLpro when it entered into human cells, such as viral replication protein RdRp, which participated in the replication of viral RNA. However, without the hydrolysis and cleavage of 3CLpro, the subsequent translation and replication cannot be carried out, and the generation of offspring viruses cannot continue even if the SARS-CoV-2 virus entered into the cells. As a result, inhibiting the activity of 3CLpro protease is capable of preventing virus replication. In addition, there is no reporting about the human homologues of 3CLpro, suggesting the negligible inhibition of 3CLpro inhibitors on human protease. Consequently, 3CLpro is an ideal anti-virus target.
The N-finger (residues 1-7) is depicted in yellow, domain I (residues 8–101) in blue, domain II (residues 102–184) in orange, the loop region (residues 185–200) in green, domain III (residues 201-306) in red.
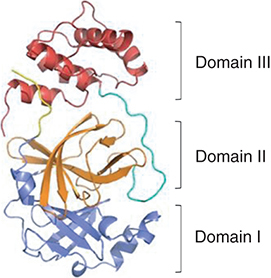
Chinese herbal medicine is an important part of traditional Chinese medicine. Recently, numerous researches have shown that a variety of natural compounds in Chinese herbal medicines have excellent biological activities favorable efficacy in anti-tumor, anti-inflammatory and antibacterial [Citation12]. In addition, the natural compounds in Chinese herbal medicine have a certain effect on the treatment and control of COVID-19 [Citation13]. Up to now, Chinese herbal medicine has made many great achievements in the medicine industry. For example, artemisinin developed by Tu Youyou, a Nobel laureate in medicine, has significantly reduced the death rate of malaria patients [Citation14]. In addition, paclitaxel has been proved to have excellent anti-tumor, anti-diabetes and anti-fungal effects [Citation15], also, the cephaletin has been found as a potential COVID-19 drug [Citation16]. The National Collection of Chinese Herbal Medicine contains a total of more than 4000 kinds of Chinese herbal medicine. More than 500 kinds of toxic traditional Chinese medicines were screened to make a list of toxic traditional Chinese medicines.
Virtual screening [Citation17] based on molecular docking, pharmacokinetic and molecular dynamics refers to simulating the interaction between targets before biological activity screening, analyzing the possible related reactions between drugs and the body, so as to improve the discovery efficiency of lead compounds and improve the success rate of drug research and development. In 2020, through homology modeling, molecular docking and binding free energy calculation, Nelfinavir was found to have therapeutic effects on COVID-19.
Herein, 1047 natural compounds were collected from 34 Chinese herbal medicines as ligands to screen on the potential 3CLpro inhibitors. Firstly, molecular docking technology was performed to evaluate the binding free energy of natural compounds-3CLpro complex, screening out the compounds with prominent binding affinity with 3CLpro. Then the drug-likeness properties and pharmacokinetic characteristics of the selected compounds were further evaluated. Afterward, molecular dynamics simulation was employed to estimate the complex stability. Finally, the most promising 3CLpro inhibitors were confirmed according to the comprehensive performance evaluation.
Materials & methods
Molecular docking
Previous research has identified that the compound ML188 was an inhibitor of the SARS-CoV 3CLpro [Citation18]. As a non-covalent inhibitor, ML188 [Citation19] was found to be more effective in inhibiting SARS-CoV-2 3CLpro at 2.5 μM than that of inhibiting SARS-CoV 3CLpro. Therefore, the protein 7L0D [Citation20] containing the co-crystalline ligand ML188 was selected as the target.
The x-ray crystal three-dimensional structure of SARS-CoV-2 main protease 3CLpro (PDB ID: 7L0D, resolution: 2.39 Å) in complex with a non-covalent inhibitor ML188 was taken from the Protein Data Bank [Citation21]. Solvents, water molecules, other heteroatoms and ligand in protein were removed by using Pymol software. AutoDock Tools was used to add polar hydrogens to the structures, and then converting to pdbqt format. Co-crystallized ligand of 7L0D (ML188), as a control in docking studies, was extracted by using Pymol software, and then converted to corresponding formats.
The ligands used for molecular docking were selected from the catalogue of toxic Chinese herbal medicines in the national compilation of Chinese herbal medicines [Citation22]. The corresponding natural compound structure files were downloaded from PubChem database according to the CAS number provided in the literature. Ligands were converted to PDB format using Open Babel software [Citation23] and further prepared to pdbqt format using AutoDock Tools.
Docking simulations were performed with AutoDock Vina [Citation24] using the empirical free energy force field and Lamarckian genetic algorithm conformational search with default parameters [Citation25]. The ideal active site where the non-covalent ML188 inhibitor interacts with 3CLpro in the 7L0D complex was chosen as the lead-in site to screen the interaction and affinity of herbal ligands with 3CLpro, taking the similar strategy that has been described previously in several studies [Citation26–30]. The grid coordinates of protein ligand binding sites are as follows, x = 11.701 Å, y = -17.509 Å, z = 16.43 Å, while grid dimensions were set at 60 × 40 × 60 Å3, with a spacing of 0.775 Å between the grid points. Other parameters were set to default [Citation31,Citation32]. The interactions of protein–ligand predicted by docking researches were visualized by Pymol. The 2D-schematic diagram of protein–ligand interaction was generated using LigPlot [Citation33].
In silico prediction of molecular properties & pharmacokinetic ADMET profiles
A large number of potential therapeutic agents fail to reach clinical trials due to adverse parameters of ADMET [Citation34,Citation35]. Thus, testing these properties is useful for drug discovery. The molecular properties [Citation36] and ADMET properties [Citation37,Citation38] of the promising natural compounds were estimated using the online web server pkCSM [Citation39] and SwissADME [Citation40].
MW (Molecular weight), TPSA (topological polar surface area), Log-P (lipophilicity), N.HBA (number of hydrogen bond acceptors), N.HBD (number of hydrogen bond donors), N.RB (number of rotational bonds) were analyzed to evaluate the drug likeness of the potential inhibitors. If Log-p > 5, this deviation results in low solubility and poor oral absorption. For TPSA, when it is less than 140 Å and the number of rotatable bonds is less than 10, the compounds become more flexible and is more able to interact with the target receptor.
ADMET properties such as Water solubility, Intestinal absorption (human), VDss (human), Caco-2 permeability [Citation41], BBB permeability [Citation42,Citation43], CYP3A4 inhibitor [Citation44], CNS permeability and Hepatotoxicity [Citation45] were calculated for drug screening and development. Intestine is the primary site for an orally administered drug absorption, a molecule with an absorbance of less than 30% is considered to be poorly absorbed. In terms of distribution indicators, the distribution (VDss) size is considered high if its value is greater than 0.45, the higher the VDss is, the more of a drug is distributed in tissue rather than plasma. For the CNS index, compounds with LogPS < -3 are believed to be incapable of penetrating the CNS.
Molecular dynamics (MD) simulations
Molecular dynamics simulation [Citation46,Citation47] of protein–ligand complexes was performed using Gromacs software [Citation48], the protein–ligand complexes were prepared by using Charmm36 [Citation49] forcefield, and the ligand topology was generated by Swissparam [Citation50].
First, download the sdf file of the ligand from Pubchem according to the CAS number, then open the file with Avogadro program, hydrogenate it, and save it as mol2 file. Finally, the file is submitted to Swissparam for processing.
A solvated dodecahedron box of SPC [Citation51] water model with 1 nm distances was constructed between the box edge and atoms of the protein–ligand complexes. And 4 sodium (Na+) were inserted to neutralize all solvated systems. Afterward, the systems were minimized through 50000 steps of the steepest descent algorithm [Citation52]. Furthermore, 100 ps NVT balance and 100 ps NPT balance were carried out to make the system reach a stable state 300 K and 1 bar [Citation53]. Finally, 100 ns molecular dynamics simulations were carried out toward the complex.
Then the main parameters, such as root mean square deviation (RMSD) [Citation54,Citation55], root mean square fluctuation (RMSF) [Citation56], radius of gyration (Rg) [Citation57] and number of hydrogen bonds [Citation58] were performed to evaluate the stability of protein–ligand complexes [Citation59].
Results
Protein contact atlas analysis
The asteroid plot is an innovative method for analyzing the interactions between protein and ligand based on the multilevel visualization of noncovalent contacts [Citation60]. Here, the representative co-crystal structure 7L0D of 3CLpro and the key amino acids of this structure were obtained from the online website Protein-Contact-Atlas ().
The asteroid plots of ML188 co-crystal structure (PDB ID: 7L0D). Asteroid plot with the ligand highlighted in blue (central node). Directly contacting residues (first-shell residues) are shown in the inner circle, and the residues that contact these but not the ligand (second-shell residues) are shown in the outer circle. The residues are colored according to their secondary structures, and the size of each circle is scaled to denote the number of atomic contacts.
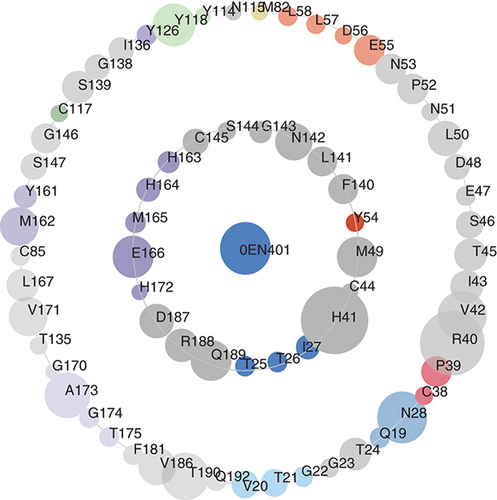
Molecular docking of 3CLpro inhibitors
listed the docking scores of partially natural compounds. As a positive control, the docking scores of co-crystal ligand ML188 was -8.1 kcal/mol. The specific docking modes [Citation61] of three natural compounds, Cleomiscosin C, (+)-Norchelidonine and Turkiyenine were exhibited in .
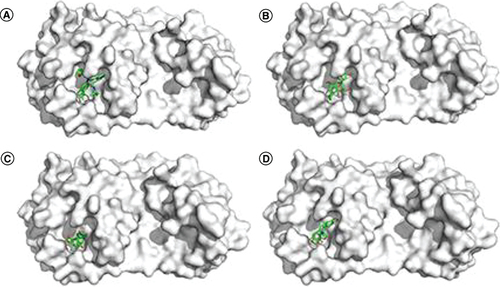
Table 1. Docking fraction of part compounds.
For Cleomiscosin C [Citation62], a compound from Xanthium sibiricum, displayed eight hydrogen bonds with the key amino acid residues of 3CLpro at Tyr54, Gly143, Ser144, Cys145 and Glu166, respectively, and the docking score was -8.6 kcal/mol. In addition, hydrophobic interactions were found between the amino acid residues and Cleomiscosin C at His41, Cys44, Met49, Phe140, Leu141, Asn142, His163, His164, Met165, His172, Asp187, Arg188 and Gln189 ().
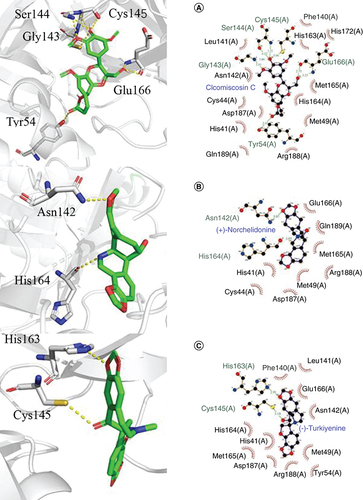
For (+)-Norchelidonine [Citation63], a compound from Chelidonium majus, only showed two hydrogen bonds with the key amino acid residues of 3CLpro, Asn142 and His164 with bond lengths of 3.07 Å and 3.05 Å, respectively, and the docking score was -8.7 kcal/mol. Similarly, hydrophobic interactions were found between the amino acid residues and (+)-Norchelidonine at His41, Cys44, Met49, Met165, Glu166, Asp187, Arg188 and Gln189 ().
For Turkiyenine [Citation64], also a compound from Chelidonium majus, showed two hydrogen bonds with the key amino acid residues of 3CLpro at Cys145 and His163, respectively, and the docking score was -9.1 kcal/mol. As presented in , there was also a hydrophobic interaction between the amino acid residues and Turkiyenine at His41, Met49, Tyr54, Phe140, Leu141, Asn142, Chis164, Met165, Glu166, Asp187 and Arg188.
In silico prediction of molecular properties & pharmacokinetic ADMET profiles
Further these 137 natural compounds selected with molecular docking results were evaluated by Lipinski's rules [Citation65]. listed the molecular properties of Cleomiscosin C, (+)-Norchelidonine, Protopine, Turkiyenine, Isochelidonine and Mallotucin A.
Table 2. Portended physiochemical parameters of the potential inhibitors.
Thereafter, in silico ADMET analysis was employed to predict the pharmacokinetic profile of compounds. As stated in the , Cleomiscosin C has a 100% absorption value and is better than the other 5 compounds in human intestinal absorption. Cleomiscosin C was an inhibitor of P-glycoprotein (P-gp I and P-gp II), but the other five compounds did not meet this index, and Mallotocin A was neither a P-gp I inhibitor nor a P-gp II inhibitor. Besides, since the inability of Cleomiscosin C and (+)-Norchelidonine to permeate across the blood–brain barrier (BBB), the central nervous system can be effectively protected from the effects of drugs. Only CNS permeability of Cleomiscosin C is less than -3, indicating that it cannot penetrate the central nervous system and can better protect the human body. Notably, cytochrome P450 is an important detoxification enzyme in the body, mainly found in the liver, this enzyme oxidizes foreign microorganisms to facilitate their excretion. The results displayed that Cleomiscosin C and Mallotucin A did not perform as inhibitors for CYP1A2, CYP2C19, CYP2C9, CYP2D6 and CYP3A4 enzymes, but (+)-Norchelidonine and Protopine were the inhibitors of two CYPs, Turkiyenine and Isochelidone were one of the inhibitors. Moreover, the Maximum Tolerated Dose and Hepatotoxicity tests of Cleomiscosin C, (+)-Norchelidonine, Protopine, Turkiyenine showed no adverse effects.
Table 3. Anticipated ADMET characteristics of the potential inhibitors.
Molecular dynamics simulations
Subsequently, molecular dynamics simulations, a calculation method for imitating the physical trajectory and state of atoms and molecules based on Newtonian mechanics, were performed to analyze the dynamic behavior of complex systems. The structural stability of Cleomiscosin C, (+)-Norchelidonine and Turkiyenine in complex with 3CLpro were simulated by molecular dynamics in this work, employing the co-crystal inhibitor (ML188) as the control group.
Root mean square deviation
As shown in A, the RMSD value of 3CLpro complex with ligand Cleomiscosin C fluctuated from 0.1 to 0.2 nm, with an average of 0.16 nm, has also showing a similar trend with ML188 complex. In addition, the mean RMSD values for 3CLpro in complex with (+)-Norchelidonine and Turkiyenine were 0.19 nm and 0.20 nm, respectively, with a slightly larger range of fluctuations.
RMSD: Root mean square deviation.
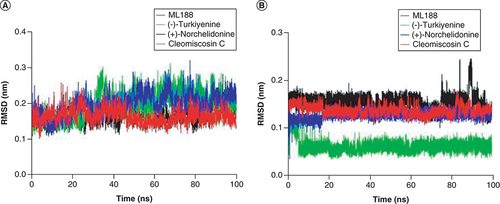
In addition, the RMSD values of the ligand Turkiyenine was lower than 0.1 nm, and the RMSD values of the ligands Cleomiscosin C and (+)-Norchelidonine showed no obvious change throughout the simulation process and fluctuated around 0.15 nm, suggesting the good binding ability of ligands with the active site on 3CLpro (B).
Root mean square fluctuation
Moreover, the RMSF value of (+)-Norchelidonine complex with 3CLpro showed significant fluctuations at ASP-48, MET-49, LEU-50 and ASN-51. Similarly, Turkiyenine complex with 3CLpro was also unstable during the simulation process, the obvious fluctuations of RMSF value of Turkiyenine-3CLpro complex were found at ARG-222, PHE-223, and THR-224. However, Cleomiscosin C-3CLpro complex exhibited superb stability and the RMSF value of key amino acid residues is small (). Notably, there is no obvious structural change during the whole simulation process, indicating the excellent effect of Cleomiscosin C-3CLpro complex.
Radius of gyration
Radius of gyration is an important index to characterize the compactness of protein structure and the change of protein looseness during simulation procedure. The abrupt fluctuation of RG values indicates the instability of protein structural. As shown in , these three complexes presented prominent structural stability during the whole simulation process, the RG value of them almost remained unchanged within 100 ns, and the fluctuation range was only about 0.05 nm.
Hydrogen bonds
Hydrogen bond is an important part of intermolecular force of protein–ligand complexes. The more hydrogen bonds formed in protein–ligand complexes, the stronger interaction between them. It should be pointed out that different software has different determination mechanisms for hydrogen bonds. The determination of hydrogen bonds in GROMACS software needs to meet two standards: (1) hydrogen bond distance r ≤ 0.35 nm; (2) angle alpha ≤30°. As shown in , the number of hydrogen bonds fluctuated throughout the 100 ns MD simulations, and the average number of hydrogen bonds was 2, 4, 1 and 1 for ML188-, Cleomiscosin C-, (+)-Norchelidonine-, and Turkiyenine-3CLpro complexes, respectively. This result was highly consistent with the number of hydrogen bonds determined by Ligplot, suggesting the high affinity and stability of Cleomiscosin C-3CLpro complex.
Discussion
In searching for small compounds to prohibit viral replication and transcription, virtual screening techniques were utilized to explore a chemical library from the toxic Chinese herbal medicines as potential SARS-CoV-2 3CLpro inhibitors.
The co-crystal ligand ML188 forms strong interactions with the direct contacted residues, such as: His41, Met49, Asn142, Glu166, Gln189, Arg188 and Asp187 in providing vital information for the following evaluation of the interaction patterns of the screened compounds.
Molecular docking results showed that, 137 natural compounds with docking scores less than -8.1 kcal/mol. Among them, Cleomiscosin C, (+)-Norchelidonine and Turkiyenine exhibited strong hydrogen bonds and hydrophobic interactions.
The drug-likeness is the most recent method proposed to identify compounds that are recommended for use in drugs that must respect Lipinski's rules, compounds need to conform to the following properties: molecular weight <500; N.HBD <5; N.HBA <10; N.RB <10; and the logarithm of octanol-water partition coefficient (Log-P) between -2 to 5. Among the 137 natural compounds with docking scores less than -8.1 kcal/mol, only Cleomiscosin C, (+)-Norchelidonine, Protopine, Turkiyenine, Isochelidonine and Mallotucin A complyed with Lipinski's rules. In silico ADMET analysis, Cleomiscosin C had the best pharmacokinetic profiles, followed by Turkiyenine.
The parameters RMSD, RMSF, RG and the number of intermolecular hydrogen bonds were used to assess the stability of protein–ligand complexes. Based on the above results, Cleomiscosin C in complex with 3CLpro was stable in the whole 100 ns simulation, and had excellent hydrogen bonding, so Cleomiscosin C was more valuable than other compounds.
Conclusion
In this work, 1047 natural compounds originated from 34 Chinese herbal medicines were carefully studied to develop the potential inhibitors of 3CLpro. Molecular docking results showed that 137 compounds possessed favorable binding affinity toward 3CLpro with docking scores lower than -8.1 kcal/mol. Among these, Cleomiscosin C, (+)-Norchelidonine, Protopine, Turkiyenine, Isochelidonine and Mallotucin A showed good drug-likeness properties by analyzing molecular properties. Besides, Cleomiscosin C and Turkiyenine showed excellent pharmacokinetic profiles in silico ADMET analysis. In addition, molecular dynamics simulation results further verified that Cleomiscosin C complex with 3CLpro had higher stability than other compounds. Hence, Cleomiscosin C is considerable hopeful to exploit as a potent 3CLpro inhibitor. However, further in vitro and in vivo evaluations are needed to validate the computational results for practical application.
A potential 3CLpro inhibitor of SARS-CoV-2 main protease were developed from Chinese herbal.
Virtual screening was employed to improve the efficiency of lead compound discovery through molecular docking, pharmacokinetic simulation and molecular dynamics simulations.
Cleomiscosin C shows good binding affinity to 3CLpro, prominent drug-likeness properties, and the Cleomiscosin C and SARS-CoV-2 main protease complex presented high stability, is promising as a potential inhibitor of SARS-CoV-2 main protease.
Author contributions
Conceptualization: Qingqing Rao and Shengxiang Yang; Data curation: Wenjing Shen; Investigation, Yi Kuang; Methodology: Yi Kuang and Xiaodong Ma; Resources: Xiaodong Ma, Qingqing Rao and Shengxiang Yang; Supervision: Qingqing Rao and Shengxiang Yang; Validation: Yi Kuang, Xiaodong Ma and Wenjing Shen; Writing – original draft: Yi Kuang and Xiaodong Ma; Writing – review & editing: Qingqing Rao and Shengxiang Yang.
Financial & competing interests disclosure
This work was supported by the “Pioneer” and “Leading Goose” R&D Program of Zhejiang (2022C02023); National Natural Science Foundation of China (32271527); Research Foundation of Talented Scholars of Zhejiang A&F University (2021LFR058); the Ability Establishment of Sustainable Use for Valuable Chinese Medicine resources (2060302) and the National Science & Technology Fundamental Resources Investigation Program of China (2018FY100800). The authors have no other relevant affiliations or financial involvement with any organization or entity with a financial interest in or financial conflict with the subject matter or materials discussed in the manuscript apart from those disclosed.
No writing assistance was utilized in the production of this manuscript.
References
- DandachiD , GeigerG , MontgomeryMWet al.Characteristics, comorbidities, and outcomes in a multicenter registry of patients with human immunodeficiency virus and Coronavirus Disease 2019. Clin. Infect. Dis.73(7), E1964–E1972 (2021).
- ColonaVL , VasilouV , WattJ , NovelliG , ReichardtJKV. Update on human genetic susceptibility to COVID-19: susceptibility to virus and response. Human Genomics15(1), (2021).
- Our World in Data. https://ourworldindata.org/ (Accessed October 20, 2022).
- SunHB , LiHJ , HuangSP , ShiLX , XingZH , ShenJ. Case report of China/Tianjin's first novel Coronavirus Variant Omicron. Iran J. of Immunol.19(1), 115–120 (2022).
- BeraK , ReedaVSJ , BabilaPR , DineshDC , HritzJ , KarthickT. An in silico molecular dynamics simulation study on the inhibitors of SARS-CoV-2 proteases (3CLpro and PLpro) to combat COVID-19. Mol. Simul.47(14), 1168–1184 (2021).
- BerryM , FieldingB , GamieldienJ. Human coronavirus OC43 3CL protease and the potential of ML188 as a broad-spectrum lead compound: homology modelling and molecular dynamic studies. BMC Struct. Biol.15, 8 (2015).
- TangBW , HeFM , LiuDPet al.AI-Aided Design of Novel Targeted Covalent Inhibitors against SARS-CoV-2. Biomolecules12(6), 746 (2022).
- BachaU , BarrilaJ , Velazquez-CampoyA , LeavittSA , FreireE. Identification of Novel Inhibitors of the SARS Coronavirus Main Protease 3CLpro. Biochemistry43(17), 4906–4912 (2004).
- NovakJ , PotemkinVA. A new glimpse on the active site of SARS-CoV-2 3CLpro, coupled with drug repurposing study. Mol. Diversity26(5), 2631–2645 (2022).
- LataS , AkifM. Comparative protein structure network analysis on 3CLpro from SARS-CoV-1 and SARS-CoV-2. Proteins Struct. Funct. Bioinf.89(9), 1216–1225 (2021).
- KideraA , MoritsuguK , EkimotoT , IkeguchiM. Allosteric Regulation of 3CL Protease of SARS-CoV-2 and SARS-CoV observed in the crystal structure ensemble. J. Mol. Biol.433(24), 167324 (2021).
- ZhaoXH , HeX , ZhongXH. Anti-inflammatory and in-vitro antibacterial activities of Traditional Chinese medicine formula Qingdaisan. BMC Complem. Altern. M.16(1), 503 (2016).
- YangF , ZhangQ , YuanZSet al.Signaling potential therapeutic herbal medicine prescription for treating COVID-19 by collaborative filtering. Front. Pharmacol.12, 759479 (2021).
- YaoW , WangF , WangH. Immunomodulation of artemisinin and its derivatives. Sci. Bull.61(18), 1399–1406 (2016).
- TomaoF , BenedettiPanici P , TomaoS. Paclitaxel and Pazopanib in Ovarian Cancer. JAMA Oncology4(9), 1298–1299 (2018).
- FanH , HeS-T , HanPet al.Cepharanthine: A Promising Old Drug against SARS-CoV-2. Advanced Biology6(12), 2200148 (2022).
- SelvarajC , DineshDC , PedoneEMet al.SARS-CoV-2 ORF8 dimerization and binding mode analysis with class I MHC: computational approaches to identify COVID-19 inhibitors. Briefings Funct. Genomics Doi: 10.1093/bfgp/elac046 (2023) ( Online ahead of print).
- JacobsJ , Grum-TokarsV , ZhouYet al.Discovery, synthesis, and structure-based optimization of a series of N-(tert-Butyl)-2-(N-arylamido)-2-(pyridin-3-yl) acetamides (ML188) as potent noncovalent small molecule inhibitors of the severe acute respiratory syndrome coronavirus (SARS-CoV) 3CL Protease. J. Med. Chem.56(2), 534–546 (2013).
- LockbaumGJ , ReyesAC , LeeJMet al.Crystal Structure of SARS-CoV-2 Main Protease in Complex with the Non-Covalent Inhibitor ML188. Viruses13(2), 174 (2021).
- DosSantos Correia PR , DonatoDe Souza AH , ChaparroRA , TenorioBarajas AY , PortoSR. Molecular docking, ADMET analysis and molecular dynamics (MD) simulation to identify synthetic isoquinolines as potential inhibitors of SARS-CoV-2 MPRO. Curr. Comput.-Aided Drug Des.19, 1–14 (2023).
- BehzadiP , GajdácsM. Worldwide Protein Data Bank (wwPDB): a virtual treasure for research in biotechnology. Eur. J. Microbiol. Immu.11(4), 77–86 (2022).
- GaoL-Q , XuJ , ChenS-D. In Silico screening of potential Chinese herbal medicine against COVID-19 by Targeting SARS-CoV-2 3CLpro and Angiotensin Converting Enzyme II using molecular docking. Chin. J. Integr. Med.26(7), 527–532 (2020).
- O'boyleNM , BanckM , JamesCA , MorleyC , VandermeerschT , HutchisonGR. Open Babel: an open chemical toolbox. J. Cheminf.3(1), 33 (2011).
- NguyenNT , NguyenTH , PhamTNHet al.Autodock Vina adopts more accurate binding poses but Autodock4 forms better binding affinity. J. Chem. Inf. Model.60(1), 204–211 (2020).
- LiuZ , JiangD , ZhangC , ZhaoH , ZhaoQ , ZhangB. A novel fireworks algorithm for the protein–ligand docking on the AutoDock. Mobile Netw. Appl.26(2), 657–668 (2021).
- DaouiO , ElkhattabiS , ChtitaS. Rational identification of small molecules derived from 9,10-dihydrophenanthrene as potential inhibitors of 3CLpro enzyme for COVID-19 therapy: a computer-aided drug design approach. Struct. Chem.33(5), 1667–1690 (2022).
- DaouiO , ElkhattabiS , ChtitaS. Rational design of novel pyridine-based drugs candidates for lymphoma therapy. J. Mol. Struct.1270, 133964 (2022).
- DaouiO , ElkhattabiS , BakhouchMet al.Cyclohexane-1,3-dione derivatives as future therapeutic agents for NSCLC: QSAR modeling, in Silico ADME-Tox properties, and structure-based drug designing approach. ACS Omega8(4), 4294–4319 (2023).
- DaouiO , NourH , AbchirO , ElkhattabiS , BakhouchM , ChtitaS. A computer-aided drug design approach to explore novel type II inhibitors of c-Met receptor tyrosine kinase for cancer therapy: QSAR, molecular docking, ADMET and molecular dynamics simulations. J. Biomol. Struct. Dyn.1–18 (2022).
- DaouiO , ElkhattabiS , ChtitaS. Design and prediction of ADME/Tox properties of novel magnolol derivatives as anticancer agents for NSCLC using 3D-QSAR, molecular docking, MOLCAD and MM-GBSA studies. Lett. Drug Des. Discovery20(5), 545–569 (2023).
- TumskiyRS , TumskaiaAV. Multistep rational molecular design and combined docking for discovery of novel classes of inhibitors of SARS-CoV-2 main protease 3CLpro. Chem. Phys. Lett.780, 138894 (2021).
- GoodfordPJ. A computational procedure for determining energetically favorable binding sites on biologically important macromolecules. J. Med. Chem.28(7), 849–857 (1985).
- WallaceAC , LaskowskiRA , ThorntonJM. LIGPLOT: a program to generate schematic diagrams of protein–ligand interactions. Protein Eng. Des. Sel.8(2), 127–134 (1995).
- DaouiO , ElkhattabiS , ChtitaS , ElkhalabiR , ZgouH , BenjellounAT. QSAR, molecular docking and ADMET properties in silico studies of novel 4,5,6,7-tetrahydrobenzo[D]-thiazol-2-Yl derivatives derived from dimedone as potent anti-tumor agents through inhibition of C-Met receptor tyrosine kinase. Heliyon7(7), e07463 (2021).
- NourH , DaouiO , AbchirO , ElkhattabiS , BelaidiS , ChtitaS. Combined computational approaches for developing new anti-Alzheimer drug candidates: 3D-QSAR, molecular docking and molecular dynamics studies of liquiritigenin derivatives. Heliyon8(12), (2022).
- HughesJD , BlaggJ , PriceDAet al.Physiochemical drug properties associated with in vivo toxicological outcomes. Bioorg. Med. Chem. Lett.18(17), 4872–4875 (2008).
- FerreiraLLG , AndricopuloAD. ADMET modeling approaches in drug discovery. Drug Discov. Today24(5), 1157–1165 (2019).
- NorinderU , BergströmCaS. Prediction of ADMET Properties. Chem. Med. Chem.1(9), 920–937 (2006).
- PiresDEV , BlundellTL , AscherDB. pkCSM: predicting small-molecule pharmacokinetic and toxicity properties using graph-based signatures. J. Med. Chem.58(9), 4066–4072 (2015).
- DainaA , MichielinO , ZoeteV. SwissADME: a free web tool to evaluate pharmacokinetics, drug-likeness and medicinal chemistry friendliness of small molecules. Sci. Rep.7(1), 42717 (2017).
- MatsumotoT , KaifuchiN , MizuharaY , WarabiE , WatanabeJ. Use of a Caco-2 permeability assay to evaluate the effects of several Kampo medicines on the drug transporter P-glycoprotein. J. Nat. Med.-Tokyo72(4), 897–904 (2018).
- LiuL , ZhangL , FengHet al.Prediction of the blood–brain barrier (BBB) permeability of chemicals based on machine-learning and ensemble methods. Chem. Res. Toxicol.34(6), 1456–1467 (2021).
- LiH , YapCW , UngCY , XueY , CaoZW , ChenYZ. Effect of selection of molecular descriptors on the prediction of blood–brain barrier penetrating and nonpenetrating agents by statistical learning methods. J. Chem. Inf. Model.45(5), 1376–1384 (2005).
- SyedS , ClemensPL , LathersDet al.Lack of effect of brivanib on the pharmacokinetics of midazolam, a CYP3A4 Substrate, administered intravenously and orally in healthy participants. J. Clin. Pharmacol.52(6), 914–921 (2012).
- XuWD , XiaoMZ , LiJYet al.Hepatoprotective effects of Di Wu Yang Gan: a medicinal food against CCl4-induced hepatotoxicity in vivo and in vitro. Food Chem.327, 127093 (2020).
- SahuSN , PattanayakSK. Molecular docking and molecular dynamics simulation studies on PLCE1 encoded protein. J. Mol. Struct.1198, 126936 (2019).
- DeVivo M , MasettiM , BottegoniG , CavalliA. Role of molecular dynamics and related methods in drug discovery. J. Med. Chem.59(9), 4035–4061 (2016).
- VanDer Spoel D , LindahlE , HessB , GroenhofG , MarkAE , BerendsenHJC. GROMACS: fast, flexible, and free. J. Comput. Chem.26(16), 1701–1718 (2005).
- CroitoruA , ParkSJ , KumarAet al.Additive CHARMM36 force field for nonstandard amino acids. J. Chem. Theory Comput.17(6), 3554–3570 (2021).
- ZoeteV , CuendetMA , GrosdidierA , MichielinO. SwissParam: a fast force field generation tool for small organic molecules. J. Comput. Chem.32(11), 2359–2368 (2011).
- GrotzKK , SchwierzN. Optimized magnesium force field parameters for biomolecular simulations with accurate solvation, ion-binding, and water-exchange properties in SPC/E, TIP3P-fb, TIP4P/2005, TIP4P-Ew, and TIP4P-D. J. Chem. Theory Comput.18(1), 526–537 (2022).
- Mosquera-YuquiF , Lopez-GuerraN , Moncayo-PalacioEA. Targeting the 3CLpro and RdRp of SARS-CoV-2 with phytochemicals from medicinal plants of the Andean Region: molecular docking and molecular dynamics simulations. J. Biomol. Struct. Dyn.40(5), 2010–2023 (2022).
- IbrahimMaA , AbdelrahmanAHM , Jaragh-AlhadadLAet al.Exploring Toxins for Hunting SARS-CoV-2 Main Protease Inhibitors: Molecular Docking, Molecular Dynamics, Pharmacokinetic Properties, and Reactome Study. Pharmaceuticals15(2), (2022).
- Velazquez-LiberaJL , Duran-VerdugoF , Valdes-JimenezA , Nunez-VivancoG , CaballeroJ. LigRMSD: a web server for automatic structure matching and RMSD calculations among identical and similar compounds in protein–ligand docking. Bioinformatics36(9), 2912–2914 (2020).
- BorhaniDW , ShawDE. The future of molecular dynamics simulations in drug discovery. J. Comput.-Aided Mol. Des.26(1), 15–26 (2012).
- FaroukA , BaigMH , KhanMI , ParkT , AlotaibiSS , DongJJ. Screening of inhibitors against SARS-CoV-2 spike protein and their capability to block the viral entry mechanism: a viroinformatics study. Saudi J. Biol. Sci.28(6), 3262–3269 (2021).
- YamamotoE , AkimotoT , MitsutakeA , MetzlerR. Universal relation between instantaneous diffusivity and radius of gyration of proteins in aqueous solution. Phys. Rev. Lett.126(12), (2021).
- MenendezCA , AccordinoSR , GerbinoDC , AppignanesiGA. Hydrogen bond dynamic propensity studies for protein binding and drug design. PLOS ONE11(10), (2016).
- LiuK , WatanabeE , KokuboH. Exploring the stability of ligand binding modes to proteins by molecular dynamics simulations. J. Comput.-Aided Mol. Des.31(2), 201–211 (2017).
- SongPL , WangG , SuYet al.Strategy and validation of a structure-based method for the discovery of selective inhibitors of PAK isoforms and the evaluation of their anti-cancer activity. Bioorg. Chem.91, 103168 (2019).
- TianW , ChenC , LeiX , ZhaoJL , LiangJ. CASTp 3.0: computed atlas of surface topography of proteins. Nucleic Acids Res.46(W1), W363–W367 (2018).
- KanS , ChenG , HanCet al.Chemical constituents from the roots of Xanthium sibiricum. Nat. Prod. Res.25(13), 1243–1249 (2011).
- KadanG , GözlerT , HesseM. (+)-Norchelidonine from Chelidonium majus. Planta Med.58(05), 477–477 (1992).
- KobayashiH , SasanoY , KanohN , KwonE , IwabuchiY. Total synthesis of the proposed structure of turkiyenine. Eur. J. Org. Chem.2016(2), 270–273 (2016).
- ChagasCM , MossS , AlisaraieL. Drug metabolites and their effects on the development of adverse reactions: Revisiting Lipinski's Rule of Five. Int. J. Pharm.549(1-2), 133–149 (2018).