Abstract
Objective
To analyze the diagnostic value of quantitative features in multimodal magnetic resonance imaging (MRI) images to construct a radio-omics model for breast cancer.
Methods
Ninety-five patients with breast-related diseases from January 2020 to January 2021 were grouped into the benign group (n=57) and malignant group (n=38) according to the pathological findings. All cases were randomized as the training group (n=66) and validation group (n=29) in a 7:3 ratio based on the examination time. All subjects were examined by T1-weighted imaging (T1WI), T2-weighted imaging (T2WI), diffusion-weighted imaging (DWI), dynamic contrast enhancement (DCE), and apparent diffusion coefficient (ADC) multimodality MRI. The MRI findings were analyzed against pathological findings. A diagnostic breast cancer radiomics model was constructed. The diagnostic efficacy of the model in the validation group was analyzed, and the diagnostic efficacy was analyzed via the ROC curve.
Results
Fibroadenoma accounted for 49.12% of benign breast diseases, and invasive ductal carcinoma accounted for 73.68% of malignant breast diseases. The sensitivity of T1WI, T2WI, DWI, ADC, and DCE in diagnosing breast cancer was 61.14%, 66.67%, 73.30%, 78.95%, and 85.96%, using the four-fold table method. The area under the curves (AUCs) of T1WI, T2WI, DWI, ADC, and DCE for diagnosing breast cancer were 0.715, 0.769, 0.785, 0.835, and 0.792, respectively. The AUCs of plain scan, diffuse, enhanced, plain scan + diffuse, plain scan + enhanced, enhanced + diffuse, and plain scan + enhanced + diffuse for diagnosing breast cancer were 0.746, 0.798, 0.816, 0.839, 0.890, 0.906, and 0.927, respectively.
Conclusion
The construction of a radio-omics model by quantitative features in multimodal MRI images was valuable in the diagnosis of breast cancer. The value of radio-omics models such as plain scan + enhanced + diffuse was higher than the other models in diagnosing breast cancer and could be widely applied in clinical practice.
Introduction
Breast cancer is a major health concern for women due to its high mortality and morbidity, and its 5-year survival rate is less than 30% even with adjuvant chemotherapy for metastatic breast cancer.Citation1,Citation2 According to the International Agency for Research on Cancer, breast cancer is the most common cancer among women and the leading cause of cancer death, followed by colorectal cancer and lung cancer. In China, the incidence rate of breast cancer is the highest among female malignant tumors. With the increase in life pressure and the acceleration of life rhythm in recent years, the incidence rate of breast cancer has increased year by year, which has a great impact on women’s physical and mental health and quality of life. It has become the focus of medical research worldwide. Early diagnosis and early treatment are of great significance to improve the treatment effect and prognosis of breast cancer.Citation3,Citation4 There are many imaging methods for the examination and diagnosis of breast diseases, including breast ultrasound, mammography, and magnetic resonance imaging (MRI) examination. Among them, contrast-enhanced T1-weighted imaging (T1WI) and T2-weighted imaging (T2WI) are MRI routine scan sequences. T1WI refers to the height of tissue signal intensity in the magnetic resonance image reflecting the difference in tissue longitudinal relaxation, and T2WI is the basis of other scan sequences conduciveness to observing lesions. Both T1WI and T2WI have certain value in diagnosing breast cancer. Diffusion-weighted imaging (DWI) is used to evaluate the effect of radiotherapy and chemotherapy for breast cancer by analyzing the changes of micro diffusion of water inside and outside cells to check the functional environment of tissues and the physiological information of water molecule movement.Citation5 Apparent diffusion coefficient (ADC) is used to describe the speed and range of diffusion movement of molecules in different directions in DWI sequences, and is the most commonly used and basic indicator of MRI DWI, which can more accurately reflect the heterogeneity of tumor tissue and provide more accurate and reliable diagnostic results.Citation6 Dynamic contrast enhancement (DCE) can be used to analyze tissue vascular density and has a high value in detecting sensitivity of tumor vascular density changes and evaluating lymph node metastasis of breast cancer.Citation7 In recent years, with the rapid development of medical imaging technology and the proficient application of artificial intelligence, radio-omics has become a new method of medical diagnosis, the main content of which is to obtain quantitative features from standard medical images in high throughput, convert the images into a mineable data space, and then analyze these data for decision support. In this way, it is expected that imaging big data will be used to formulate cancer diagnosis and treatment plans, which not only provides an objective method for assessing tumor heterogeneity, but also adds a new dimension to precision medicine.Citation8 Radio-omics is mainly used in the imaging of head, neck, and lung diseases. Moreover, some studies believe that the radio-omics model may be helpful in the accurate diagnosis of breast cancer.Citation9
In this study, 95 patients with breast-related diseases were picked to analyze the diagnostic value of radio-omics model constructed based on quantitative features in MRI images for breast cancer.
Materials and Methods
General Materials
The clinical data of 95 patients with breast-related diseases who received an initial diagnosis in our hospital from January 2020 to January 2021 were retrospectively analyzed. Inclusion criteria: (1) Patients aged 25–80 years old. (2) All patients were confirmed as having breast cancer by ultrasound examination, molybdenum target screening, and MRI examination. (3) Lesions were diagnosed as benign and malignant by biopsy or surgical resection. (4) The patient and their family members are informed and have good compliance. They could cooperate with the examination and treatment, and all signed the informed consent forms. Exclusion criteria: (1) Patients with other malignant tumors. (2) Patients with incomplete clinical data. (3) Patients with unclear MRI images. All patients were grouped into a benign group (n=57) and a malignant group (n=38) based on pathological results. All experimental operations were ratified by the hospital Ethics Committee and complied with the Declaration of Helsinki. The general information selection process is shown in .
Methods
Siemens MRI breast scanner Magnetom Espree Pink and 16-channel dual breast surface dedicated coil were used. The patient was instructed to lie prone on the examination table, raise their hands to the head, and place their breasts naturally suspended in the coil. Multimodal MRI examinations such as T1WI, T2WI, DWI, ADC, and DCE were performed sequentially. (1) T1WI parameters: horizontal axis position, 2.46ms TE, 6.00ms TR, 1.6.00mm layer thickness and 360 × 360mm FOV. (2) T2WI parameters: horizontal axis position, 54.00ms TE, 4000ms TR, 4.00mm layer thickness, and 340 × 340mm FOV. (3) DWI parameters: horizontal axis position, 50.00ms TE, 5500ms TR, 5.00mm layer thickness, 280 × 280mm. The diffusion sensitivity factors b of FOV are 50, 400, and 800s2/mm, respectively. Gd-DTPA contrast agent was injected at 2.5mL/s for 10 seconds. ADC diagrams were automatically generated by Siemens workstations. (4) DCE parameters: 3D fast gradient echo sequence, 4.00ms TE, 7.50ms TR, 2.00mm layer thickness. The transverse section was scanned 9 consecutive times for 8 minutes. Multi-modal MRI images were pretreated, and Region of Interest (ROI) was selected to extract quantitative features of radio-omics. Complete MRI images of all enrolled patients were collected, and the mammary gland of the DCE-MRI image was segmented layer by layer with the region of interest, that was, 3D segmentation, which could fully reflect the overall situation of the tumor and expose complete tumor information. Image analysis and measurement were carried out independently by two doctors of different seniority at the post-processing workstation. T2WI and DCE-MRI images were also used for delineation. When sketching ROI, first perform ROI delineation on DWI, then copy the ROI to the ADC plot to outline the lesion, and measure the corresponding ADC value. The measurement method: The small ROI method measured the ADC value: The ROI size was about 0.07cm2, two small circles were drawn as regions of interest in the largest area of the tumor to measure the ADC, and the average value of the two was taken as the measurement value after avoiding necrosis, cystic changes, bleeding and other areas. The area ROI method measured the ADC value: The area at the largest level of the tumor was delineated as an ROI measurement ADC, avoiding the cystic lesion and necrosis area visible to the naked eye. The volumetric ROI method measured the ADC value: The area of each layer of the tumor was delineated as the ROI measurement ADC, and the average ADC value of all the contoured layers of the tumor was taken as the measurement value. The ADC values measured by the two observers were averaged as the final measurement. The quantitative features of radio-omics were extracted, and plain scan (T1WI, T2WI), diffusion (DWI, ADC), enhanced (DCE) and other MRI examinations extracted from the ROI region of lesions were used to extract morphological features, first-order features, and texture features. The representative features with the highest discriminative power were selected and applied to construct a radio-omics model using Support Vector Machines (SVM):Citation10 SVM was a binary classification model in which SVM constructed a hyperplane so that the edges of two classes in a high-dimensional feature space were spaced at the maximum, and the vector that defined the hyperplane was called a support vector. Use the “e1071” package of R software to build the model, download the support vector machine package, import data, divide the data, use 2/3 of the data as the training set with 1/3 of the data as the verification set, build the model with the model prediction, and calculate the accuracy of the model prediction and other indicators.
Feature selection: After selecting image group features, the Spearman correlation test is used for feature simplification to avoid overfitting. Spearman correlation analysis performed feature preprocessing, and texture parameters with correlation coefficient higher than 0.9(| r |>0.9) were removed to obtain the final feature parameters. The representative feature with the highest discrimination (AUC value) in each group is selected, and other features are deleted. Then, the LASSO algorithm is used to select key features with classification ability to establish a radio-omics model. The LASSO logistic regression model can reduce the dimensionality of high-dimensional data and screen out features with predictive value. In the feature selection process of the LASSO logistic regression model, the feature coefficients that do not have predictive value are reduced to zero, and these features are deleted. Then, other non-zero features are selected for cross-validation analysis with ten folds. Finally, the optimal feature is determined by the optimal average AUC value of the LASSO logistic regression model performing tenfold cross-validation in the training group. Spearman correlation test and LASSO logistic regression analysis were conducted using MATLAB R2018b (MathWorks Inc.) software.
Model establishment:Citation11 The imaging omics features of T1WI, T2WI, DWI, ADC, and DCE quantitative parameter maps of all cases were evaluated. The consistency of lesion segmentation within and between observers was evaluated, and ICC>0.75 indicates good consistency. All cases were randomized as a training group (n=66) and a validation group (n=29) in a 7:3 ratio based on the examination time. The MRI images are shown in . Then all T1WI, T2WI, DWI, ADC, DCE 5 sequences were combined as plain scan + enhanced group (T1WI+T2WI+DCEI), plain scan + diffuse group (T1WI+T2WI+DWI+ADC+ADC), enhanced + diffuse (DWI+ADC+DCE), and plain scan + enhanced + diffuse group (T1WI+T2WI+DWI+ADC+DCE).
Figure 2 MRI images of the training and validation group.
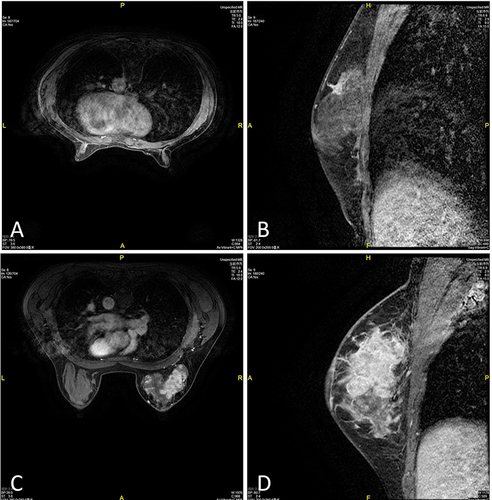
The model is established using a Support Vector Machine (SVM) classifier. SVM Classifier was implemented using Toolkit libsvm-3.21 in MATLAB (https://www.csie.ntu.edu.tw/ ~Cjlin/ibsvm/). For the combination of two or three sequences, support vector machine models with key features are trained based on training groups. ROC curves were drawn in the validation group to evaluate the model. To evaluate the performance of the model, MathWorks R2018b (MathWorks Inc.) software was used to calculate the area under the curve (AUC), accuracy, sensitivity, and specificity.
Outcome Measures
Clinicopathological data: The clinical data of the patients in two groups were collected, including the age, BMI, tumor size, menstruation, ethnicity, human epidermal growth factor receptor 2 (HER2) status, education, marital status, smoking, drinking, age of first menstruation, delivery, breast cancer family history, etc. The disease types and proportions of two groups of patients were analyzed.
Comparative analysis of MRI and pathological results: The four-fold table method was used to analyze the differences between MRI and pathological results. Sensitivity=True Benign Number/(True Benign Number+False Malignant Number) * 100%, specificity=True Malignant Number/(True Malignant Number+False Benign Number) * 100%.
Statistical Analysis
The experimental data were analyzed using SPSS 20.0 software. Measurement data such as age, tumor size, and initial menstrual age were shown as () and compared using a t-test. Enumeration data such as disease type, menstrual status, and marital status were expressed in the form of (%) and compared using χ2 or Fisher test. The statistically significant results were those with P<0.05. The receiver operating characteristic curve (ROC curve) was used to analyze the AUC, sensitivity, specificity, and accuracy thresholds of single and multi-radio-omics models.
Results
Comparison of General Information
In this experiment, 57 cases of benign breast diseases were diagnosed through pathological examination, with fibroadenoma accounting for the highest proportion (49.12%). Thirty-eight cases of malignant breast diseases were diagnosed, with invasive ductal carcinoma accounting for the highest proportion, accounting for 73.68%. In the malignant group, there were 25 cases of BI-RADS grade 4, 13 cases of BI-RADS grade 5, 19 cases of TNM grade I, 12 cases of grade II, and 7 cases of grade III. The age and tumor size of patients in the malignant group were significantly higher than those in the benign group (P<0.05, and ).
Table 1 Analysis of the Proportion of Disease Types (Cases, %)
Table 2 Comparison of General Information ()
Comparative Analysis of MRI Examination results and Pathological Results
Four-fold table analysis showed that the sensitivity and specificity of T1WI in the diagnosis of breast cancer were 61.14% and 73.68%, respectively; The sensitivity and specificity of T2WI in diagnosing breast cancer were 66.67% and 71.05%, respectively; The sensitivity and specificity of ADC in diagnosing breast cancer were 73.30% and 78.95%, respectively; The sensitivity and specificity of DCE in diagnosing breast cancer were 78.95% and 86.84%, respectively; The sensitivity and specificity of DWI in the diagnosis of breast cancer were 85.96% and 84.21% respectively ().
Table 3 Comparative Analysis of MRI Examination Results and Pathological Results (Cases, %)
Extracting Radio-Omics Parameters Related to Lesions
According to the inclusion time, the cases were divided into a training group (n=66) and a validation group (n=29). No difference existed in age, BMI and tumor size between the two groups (P>0.05, ). MRI examinations such as plain scan (T1WI, T2WI), diffusion (DWI, ADC), and enhancement (DCE) was extracted from the ROI area of the lesion in the training group patients to extract morphological features, first-order features, and texture features. The representative MRI images of the training and validation group were shown in . The specific radio-omics parameters were shown in .
Table 4 Comparison of General Information Between Two Groups ()
Table 5 Radio-Omics Parameters Related to Lesions
The Value of a Single MRI Parameter in the Diagnosis of Breast Cancer
The SVM classifier was used to construct the imaging model for diagnosis of breast cancer based on the T1WI, T2WI, DWI, ADC, and DCE of patients in the training group. ROC curve analysis showed that the AUC of T1WI, T2WI, DWI, ADC, and DCE in diagnosis of breast cancer were 0.715, 0.769, 0.785, 0.835, and 0.792, respectively ( and ).
Table 6 The Value of a Single MRI in the Diagnosis of Breast Cancer
Value of Combined MRI Parameter in the Diagnosis of Breast Cancer
The SVM classifier was used to construct a multimodal imaging model for diagnosis of breast cancer based on the histological indicators of plain scan (T1WI, T2WI), diffusion (DWI, ADC), and enhancement (DCE) of patients in the training group. ROC curve analysis found that AUC of plain scan, diffuse, enhanced, plain scan + diffuse, plain scan + enhanced, enhanced + diffuse, plain scan + enhanced + diffuse diagnosis of breast cancer was 0.746, 0.798, 0.816, 0.839, 0.890, 0.906, 0.927 respectively ( and ).
Table 7 Value of Combined MRI in Diagnosis of Breast Cancer
Discussion
At present, the main imaging methods for clinical diagnosis of breast cancer include ultrasound, X-ray photography, and MRI. Ultrasound is widely used in the screening of breast cancer, but it is difficult to find lesions with small characterization due to the subjective influence of the examiner. X-ray photography is a common examination method in clinical diagnosis, which can be used to diagnose breast cancer by reflecting the size, shape, and microcalcification of the focus tissue. However, X-ray photography is more susceptible to female breast fat, and the cancer diagnostic performance of women who have dense breasts is low.Citation12 MRI radio-omics utilizes analysis techniques such as texture analysis and wavelet analysis to transform the information and features contained in MR images into quantifiable data. In traditional clinical environments, radiologists visually evaluate tumors to obtain tumor morphology and hemodynamic features and make diagnostic decisions. The characteristics of malignant lesions are usually irregular edges, burrs, rapid outflow, uneven internal enhancement, and edge-enhanced lesions.Citation8 The characteristics of benign lesions are smooth or quasi-circular edges, internal enhancement, uniform enhancement, non-enhanced internal separation, and type of contrast inflow. These features are not always visually distinguishable as they may overlap between two types of lesions. The main objective of radiomics is to extract and quantitatively evaluate hidden information in images that is not visible to the human eye, while eliminating observer bias related to manual examination.Citation13 DCE is currently the most commonly used MRI technique for diagnosing breast diseases, which distinguishes benign and malignant breast lesions by analyzing high-resolution morphological and hemodynamic changes of breast lesions.Citation14,Citation15 DWI is a widely used non-invasive MRI examination method in clinical practice without contrast injection. The benign and malignant breast diseases are determined by analyzing the diffusion changes of water molecules in histiocyte and structures. Because of its high specificity, DWI has become one of the common means for screening breast cancer.Citation16,Citation17 ADC plot is an apparent diffusion coefficient plot obtained by fitting the diffusion changes of water molecules in DWI with a single index, which can reflect the range of random movement of water molecules in the microstructure of lesion tissue per unit time. ADC maps have important value in diagnosing malignant tumors and differentiating tumor grading.Citation18,Citation19 In this experiment, the sensitivity of T1WI, T2WI, DWI, ADC and DCE in the diagnosis of breast cancer was 61.14%, 66.67%, 73.30%, 78.95%, and 85.96%. These above results suggested that combined MRI information had certain value in the diagnosis of breast cancer, among which DCE had a high value, which was similar to the research results of Kurihara H et al.Citation20 Some scholars have established a radiomics model based on DCE-MRI, and it has been found that this model has the potential to predict the efficacy of neoadjuvant chemotherapy for breast cancer.Citation21 Some partial breast MRI studies mainly extract features from DWI and DCE-MRI images for analysis, without considering T1WI or T2WI.Citation22,Citation23 Roy et alCitation24 confirm the importance of TIWI and T2WI in radiomics research. Therefore, each modality image should be considered during the study. ZhangCitation25 selects 22 radiomics features to construct the SVM model by lasso algorithm, and finds that 6 features have no statistical difference after comparing these features between the two groups. Although the clinical significance of these radiomics features is difficult to interpret at present, they still have value in the process of machine learning.
In recent years, with the rapid development of functional imaging technology and image acquisition technology, radio-omics has emerged. Radio-omics improves image analysis by automatically extracting a large number of quantitative features from images with high throughput, including collecting and reconstructing images, segmenting and recombining images, extracting and filtering features, establishing databases, and sharing datasets to construct models. Radio-omics has become an emerging method for imaging diagnosis of diseases.Citation26 Some scholarsCitation27 respectively used XGBoost and random forest to construct a risk prediction model for premenopausal/postmenopausal breast cancer, screen breast cancer related risk factors and rank them in importance. The top 10 risk factors list of breast cancer screened by the two algorithms shows that the main risk factors before and after menopause include total lactation time, cumulative use time of artificial contraceptive devices, polygenic risk scores (PRS), years of passive smoking, body mass index (BMI), weight information and age. Both models had AUC values greater than 70% before and after menopause. Some scholars used LightGBM model to fully fit target training to reduce model deviation and avoid overfitting, and compared it with XGBoost method. The experimental results showed that LightGBM algorithm had better classification effect, faster training speed and lower memory occupation for the feature vectors in this experiment. Thus, LightGBM model was expected to better assist doctors in diagnosis of breast cancer, improve accuracy and reduce misdiagnosis rate.Citation28 SVM is a Linear classifier, which can find the separation hyperplane by transforming the classification problem, maximize the realization of classification, and has the maximum possible margin. It is widely used in clinical construction of omics models.Citation29 Previous studiesCitation30 have shown that adding diffusion and kurtosis to DCE imaging data centers can improve diagnostic efficiency. T2WI and DKI are effective auxiliary sequences for improving the diagnostic efficiency of DCE-MRI. Models based on T1WI or ADC images exhibit lower diagnostic efficiency compared to other single sequence models. Adding the features of T1WI or ADC images to other models does not improve diagnostic performance, possibly due to the contribution of T1WI or ADC to the differential diagnosis of benign and malignant lesions. In this experiment, patients were divided into a training group and a validation group. Morphological features, first-order features, and texture features were extracted from the ROI area of the lesion in the training group through MRI examinations such as plain scan, diffusion, and enhancement. In order to further analyze the value of MRI imaging model in the diagnosis of breast cancer, ROC curve analysis was established in this experiment. The AUC results suggested that MRI radio-omics model had a certain predictive value in the diagnosis of breast cancer. Among them, the multimodal MRI radio-omics model had a higher predictive value than the single radio-omics model, which could help physicians to early diagnose breast cancer and give effective targeted treatment, and had important significance in improving the prognosis of patients. Different tissue comparisons provide specific representations of tissues and images based on physiological attributes and physics of each tissue type, and the integration of all imaging information from different radiation parameters provides a more complete view of potential biological tissue characteristics. Fusco et alCitation31 used dynamic, morphological and texture features to classify breast lesions in DCE-MRI, respectively, and compared the performance of five different machine learning algorithms according to the above features. The final results showed that the performance of tree-based classifier was better than that of artificial neural network, support vector machine and Bayesian classifier. Some scholarsCitation32 combined the features of mammography and DCE-MRI to identify benign and malignant non-palpable breast lesions, and the AUC of the classification model constructed by RF reached 0.903. Therefore, combined with the results of other scholars and this study, it can be seen that RF is an effective prediction tool.
The importance of multimodal MRI radiomics: (1) Improvement of the accuracy of early diagnosis of breast cancer. Multimodal MRI radiomics combines a variety of MRI imaging techniques, such as T1WI, T2WI, DCE, etc., which can comprehensively and in detail display the structure, function and metabolism of breast tissue. Comprehensive analysis of these multi-dimensional data will help to improve the detection rate and diagnostic accuracy of early breast cancer. (2) Development of individualized treatment regimens. Multi-modality MRI radiomics can provide rich biomarker information, such as tumor size, shape, margin, and hemodynamic characteristics, which is helpful to more accurately evaluate the biological behavior and prognosis of breast cancer. This information is essential for the development of an individualized treatment plan, which can help physicians to choose the most appropriate treatment method, improve the treatment effect, and reduce the recurrence rate.
Economic benefit analysis: (1) By reducing unnecessary medical expenses, early diagnosis can significantly improve the survival rate of breast cancer patients, and reduce the complexity and high cost of late treatment. The application of multimodal MRI radiomics can detect breast cancer at an early stage, thereby reducing unnecessary medical expenses and the economic burden on society and individuals. (2) Promoting the development of the medical industry. With the promotion and application of multimodal MRI radiomics technology, it will promote the development of related medical devices, diagnostic reagents, data processing software and other industries. This will not only create new economic growth points for the medical industry, but also help to promote the progress of the whole medical technology, forming a virtuous circle.
Multimodal MRI radiomics can more accurately identify and evaluate the disease of breast cancer patients by combining a variety of imaging techniques. This allows many patients to be diagnosed at an earlier stage, resulting in improved treatment outcomes and survival rates. However, while this technology brings benefits, it also exposes some ethical issues. First, for the protection of patient privacy, multi-modality MRI radiomics needs to collect a large amount of patient personal information, including biological characteristics, genetic information, etc. Disclosure of such information could lead to violation of patient privacy rights. Therefore, in the process of data collection, storage and use, medical institutions and relevant researchers should strictly abide by the principle of privacy protection to ensure the security of patient information.
Comparison of the advantages of multimodal MRI radiomics with other diagnostic methods: Compared with traditional imaging, multimodal MRI radiomics has obvious advantages in spatial resolution and tissue specificity. It can more accurately display the biological characteristics of tumors and provide more accurate diagnostic information for clinicians. Compared with biomarker detection, multimodal MRI radiomics can obtain rich biomarker information without invasive operation. In addition, it can also monitor the biological changes of tumors in real time, providing a strong basis for clinical treatment. Compared with genetic testing, multimodal MRI radiomics has the advantages of non-invasive, rapid and low cost. Although genetic testing is of great value in the diagnosis of breast cancer, multi-modal MRI radiomics can make up for the deficiency of genetic testing to a certain extent and provide more information for clinical decision-making.
Conclusion
To sum up, the construction of radio-omics models based on the quantitative characteristics of multimodal MRI images had a certain value in the diagnosis of breast cancer. Among them, combined radio-omics models such as plain scan + enhanced + diffuse had a higher value in the diagnosis of breast cancer and could be widely used in clinical practice. However, due to the short study time, small sample size, and only extracting the radiomics features within the tumor without adding the radiomics features around the tumor, the experimental results may have a contingency and cannot truly reflect the performance of the model to a certain extent. In addition, to facilitate image processing, only patients with mass lesions were included in the study, and patients with non-mass enhancement were not analyzed. In this study, only radiomics features were used to establish a model, and other clinical and genetic factors were not combined for comprehensive analysis. In the future, the experimental objects and research time will be expanded, and the research on the progress of radio-omics characteristics of the tissues around the tumor will be carried out.
Research innovation: Highly personalized: Multimodal MRI radiomics can achieve highly personalized diagnosis according to individual differences of patients. By analyzing the MRI features of different patients, the tailored diagnostic scheme was developed to improve the diagnostic accuracy. Application of deep learning technology: Deep learning technology is applied to multimodal MRI radiomics to achieve automatic extraction, classification and recognition of image data, and greatly improve the efficiency of diagnosis. In addition, deep learning technology can also find small myocardial infarction foci that are difficult to be identified by traditional imaging, which provides strong support for early diagnosis. Multi-parameter joint analysis: Multimodal MRI radiomics can comprehensively evaluate the biological characteristics of breast cancer by jointly analyzing a variety of imaging parameters, such as morphological, functional and metabolic parameters, and provide powerful guidance for clinical treatment. Artificial intelligence-assisted diagnosis: Combined with artificial intelligence technology, multimodal MRI radiomics can achieve rapid and accurate diagnosis of breast cancer, reduce the workload of doctors, and improve the efficiency of diagnosis.
Future perspectives: Data mining: Multi-modal MRI radiomics data were further mined to find more characteristic parameters with diagnostic value and improve the accuracy of breast cancer diagnosis. Interdisciplinary research: Strengthen multidisciplinary cooperation, such as biology, pathology, genetics, etc. to comprehensively reveal the pathogenesis of breast cancer and provide new ideas for diagnosis and treatment. Individualized treatment: To develop individualized treatment plans based on multimodal MRI radiomics features to achieve precision medicine. Optimization of artificial intelligence technology: The artificial intelligence algorithm was continuously optimized to improve the performance of multimodal MRI radiomics in breast cancer diagnosis.
Ethics Approval and Consent to Participate
This study was approved by The Ethics Committee of Fujian Maternity and Child Health Hospital. Informed consent was obtained from participants for the participation in the study and all methods were carried out in accordance with relevant guidelines and regulations.
Author Contributions
All authors made a significant contribution to the work reported, whether that is in the conception, study design, execution, acquisition of data, analysis and interpretation, or in all these areas; took part in drafting, revising or critically reviewing the article; gave final approval of the version to be published; have agreed on the journal to which the article has been submitted; and agree to be accountable for all aspects of the work.
Disclosure
The authors declare that they have no competing interests.
Data Sharing Statement
The datasets used and/or analyzed during the current study are available from the corresponding author on reasonable request.
References
- Riggio AI, Varley KE, Welm AL. The lingering mysteries of metastatic recurrence in breast cancer. Br J Cancer. 2021;124(1):13–26. doi:10.1038/s41416-020-01161-4
- Bray F, Ferlay J, Soerjomataram I, Siegel RL, Torre LA, Jemal A. Global cancer statistics 2018: GLOBOCAN estimates of incidence and mortality worldwide for 36 cancers in 185 countries. CA Cancer J Clin. 2018;68(6):394–424. doi:10.3322/caac.21492
- Fumoleau P, Roché H, Kerbrat P, et al; French Adjuvant Study Group. Long-term cardiac toxicity after adjuvant epirubicin-based chemotherapy in early breast cancer: French results. Ann Oncol. 2006;17(1):85–92. doi:10.1093/annonc/mdj034
- Wang J, Sun T, Ouyang Q, Han Y, Xu B. A phase Ib study of TQB2450 plus anlotinib in patients with advanced triple-negative breast cancer. iScience. 2023;26(6):106876. doi:10.1016/j.isci.2023.106876
- Sumkin JH, Berg WA, Carter GJ, et al. Diagnostic performance of MRI, molecular breast imaging, and contrast-enhanced mammography in women with newly diagnosed breast cancer. Radiology. 2019;293(3):531–540. doi:10.1148/radiol.2019190887
- Hollingsworth AB, Pearce MR, Stough RG. Breast cancer survival following MRI detection in a high-risk screening program. Breast J. 2020;26(5):991–994. doi:10.1111/tbj.13813
- Marshall H, Pham R, Sieck L, Plecha D. Implementing abbreviated MRI screening into a breast imaging practice. AJR Am J Roentgenol. 2019;213(1):234–237. doi:10.2214/AJR.18.20396
- Cui Q, Sun L, Zhang Y, et al. Value of breast MRI omics features and clinical characteristics in Breast Imaging Reporting and Data System (BI-RADS) category 4 breast lesions: an analysis of radiomics-based diagnosis. Ann Transl Med. 2021;9(22):1677. doi:10.21037/atm-21-5441
- Upadhaya T, Morin O, Park CC. Second-harmonic generation image based histomics for the prediction of breast cancer recurrence. Int J Radiat Oncol Biol Phys. 2020;108(3):e70–e71. doi:10.1016/j.ijrobp.2020.07.1144
- Li Q, Luo Y, Liu D, Li B, Liu Y, Wang T. Construction and prognostic value of enhanced CT image omics model for noninvasive prediction of HRG in bladder cancer based on logistic regression and support vector machine algorithm. Front Oncol. 2023;12:966506. doi:10.3389/fonc.2022.966506
- LoGullo R, Horvat J, Reiner J, Pinker K. Multimodale, parametrische und genetische brustbildgebung [Multimodal, multiparametric and genetic breast imaging]. Radiologe. 2021;61(2):183–191. doi:10.1007/s00117-020-00801-3
- Zaric O, Farr A, Poblador Rodriguez E, et al. 7T CEST MRI: a potential imaging tool for the assessment of tumor grade and cell proliferation in breast cancer. Magn Reson Imaging. 2019;59:77–87. doi:10.1016/j.mri.2019.03.004
- Wang X, Wang X, Zhang Y, et al. Development of the prediction model based on clinical-imaging omics: molecular typing and sentinel lymph node metastasis of breast cancer. Ann Transl Med. 2022;10(13):749. doi:10.21037/atm-22-2844
- Heacock L, Lewin AA, Toth HK, Moy L, Reig B. Abbreviated MR imaging for breast cancer. Radiol Clin North Am. 2021;59(1):99–111. pii: S0033-8389(20)30115-9. doi:10.1016/j.rcl.2020.09.001
- Grubstein A, Rapson Y, Stemmer SM, et al. Timing to imaging and surgery after neoadjuvant therapy for breast cancer. Clin Imaging. 2021;71:24–28. doi:10.1016/j.clinimag.2020.10.043
- Carmona-Bozo JC, Manavaki R, Woitek R, et al. Hypoxia and perfusion in breast cancer: simultaneous assessment using PET/MR imaging. Eur Radiol. 2021;31(1):333–344. doi:10.1007/s00330-020-07067-2
- Pujara AC, Kim E, Axelrod D, Melsaether AN. PET/MRI in Breast Cancer. J Magn Reson Imaging. 2019;49(2):328–342. doi:10.1002/jmri.26298
- Partovi S, Sin D, Lu Z, et al. Fast MRI breast cancer screening - Ready for prime time. Clin Imaging. 2020;60(2):160–168. doi:10.1016/j.clinimag.2019.10.013
- Sorace AG, Virostko J, Wu C, et al. Abstract P1-01-02: quantitative breast MRI to predict response to neoadjuvant therapy in community imaging centers: preliminary results. Cancer Res. 2019;79(4 Supplement):P1-01-02-P1-01-02. doi:10.1158/1538-7445.SABCS18-P1-01-02
- Kurihara H, Shimizu C, Miyakita Y, et al. Molecular imaging using PET for breast cancer. Breast Cancer. 2016;23(1):24–32. doi:10.1007/s12282-015-0613-z
- Montemezzi S, Benetti G, Bisighin MV, et al. 3T DCE-MRI radiomics improves predictive models of complete response to neoadjuvant chemotherapy in breast cancer. Front Oncol. 2021;11:630780. doi:10.3389/fonc.2021.630780
- Ma M, Gan L, Jiang Y, et al. Radiomics analysis based on automatic image segmentation of DCE-MRI for predicting triple-negative and nontriple-negative breast cancer. Comput Math Methods Med. 2021;2021:2140465. doi:10.1155/2021/2140465
- Qi X, Wang W, Pan S, et al. Predictive value of triple negative breast cancer based on DCE-MRI multi-phase full-volume ROI clinical radiomics model. Acta Radiol. 2024;65(2):173–184. doi:10.1177/02841851231215145
- Roy S, Whitehead TD, Quirk JD, et al. Optimal co-clinical radiomics: sensitivity of radiomic features to tumour volume, image noise and resolution in co-clinical T1-weighted and T2-weighted magnetic resonance imaging. EBioMedicine. 2020;59:102963. doi:10.1016/j.ebiom.2020.102963
- Zhang Q, Peng Y, Liu W, et al. Radi omics based on multimodal MRI for the Di fferential Di agnosis of B enign and M alignant breast lesi ons. J Magn Reson Imaging. 2020;52(2):596–607. doi:10.1002/jmri.27098
- Latini F, Fahlström M, Fällmar D, Marklund N, Cunningham JL, Feresiadou A. Can diffusion tensor imaging (DTI) outperform standard magnetic resonance imaging (MRI) investigations in post-COVID-19 autoimmune encephalitis? Ups J Med Sci. 2022;127. doi:10.48101/ujms.v127.8562
- Li Q, Yang H, Wang P, Liu X, Lv K, Ye M. XGBoost-based and tumor-immune characterized gene signature for the prediction of metastatic status in breast cancer. J Transl Med. 2022;20(1):177. doi:10.1186/s12967-022-03369-9
- Pan J, Ma B, Hou X, et al. The construction of transcriptional risk scores for breast cancer based on lightGBM and multiple omics data. Math Biosci Eng. 2022;19(12):12353–12370. doi:10.3934/mbe.2022576
- Tong L, Wu H, Wang MD. Integrating multi-omics data by learning modality invariant representations for improved prediction of overall survival of cancer. Methods. 2021;189:74–85. doi:10.1016/j.ymeth.2020.07.008
- Sun SY, Ding Y, Li Z, et al. Multiparameter MRI Model With DCE-MRI, DWI, and Synthetic MRI Improves the Diagnostic Performance of BI-RADS 4 Lesions. Front Oncol. 2021;11:699127. doi:10.3389/fonc.2021.699127
- Fusco R, Sansone M, Filice S, et al. Pattern recognition approaches for breast cancer DCE-MRI classification: a systematic review. J Med Biol Eng. 2016;36(4):449–459. doi:10.1007/s40846-016-0163-7
- Zhao YF, Chen Z, Zhang Y, et al. Diagnosis of breast cancer using radiomics models built based on dynamic contrast enhanced MRI combined with mammography. Front Oncol. 2021;11:774248. doi:10.3389/fonc.2021.774248