Abstract
Objective
The management of fibromyalgia (FM), a chronic musculoskeletal disease, remains challenging, and patients with FM are often characterized by high health care resource utilization. This study sought to explore potential drivers of all-cause health care resource utilization and other factors associated with high resource use, using a large electronic health records (EHR) database to explore data from patients diagnosed with FM.
Methods
This was a retrospective analysis of de-identified EHR data from the Humedica database. Adults (≥18 years) with FM were identified based on ≥2 International Classification of Diseases, Ninth Revision codes for FM (729.1) ≥30 days apart between January 1, 2008 and December 31, 2012 and were required to have evidence of ≥12 months continuous care pre- and post-index; first FM diagnosis was the index event; 12-month pre- and post-index reporting periods. Multivariable analysis evaluated relationships between variables and resource utilization.
Results
Patients were predominantly female (81.4%), Caucasian (87.7%), with a mean (standard deviation) age of 54.4 (14.8) years. The highest health care resource utilization was observed for the categories of “medication orders” and “physician office visits,” with 12-month post-index means of 21.2 (21.5) drug orders/patient and 15.1 (18.1) office visits/patient; the latter accounted for 73.3% of all health care visits. Opioids were the most common prescription medication, 44.3% of all patients. The chance of high resource use was significantly increased (P<0.001) 26% among African-Americans vs Caucasians and for patients with specific comorbid conditions ranging from 6% (musculoskeletal pain or depression/bipolar disorder) to 21% (congestive heart failure). Factors significantly associated with increased medications ordered included being female (P<0.001) and specific comorbid conditions (P<0.05).
Conclusion
Physician office visits and pharmacotherapy orders were key drivers of all-cause health care utilization, with demographic factors, opioid use, and specific comorbidities associated with resource intensity. Health systems and providers may find their EHRs to be a useful tool for identifying and managing resource-intensive FM patients.
Introduction
Fibromyalgia (FM) is a chronic musculoskeletal disease with evidence suggesting that it results from a dysfunction of central pain processing pathways.Citation1,Citation2 The clinical characteristics of FM include the hallmark symptom of chronic widespread pain, which is a key feature required for diagnosis,Citation3 as well as a constellation of other symptoms such as sleep disturbances, headaches, fatigue, reduced function, anxiety, and depression.Citation4 The prevalence of FM in the US has been estimated to be between ~2% and 6%,Citation5,Citation6 with middle-aged women disproportionately affected.Citation7
Management of FM remains challenging despite the development of guidelines that recommend use of several medication classesCitation8,Citation9 and the approval by the US Food and Drug Administration of three medications indicated for its treatment (duloxetine, milnacipran, and pregabalin). While opioids have been consistently reported to be one of the most commonly prescribed pain-related medications in patients with FM,Citation2,Citation10–Citation14 they are neither effective nor recommended for long-term management,Citation15,Citation16 with only weak opioids such as tramadol suggested in guidelines.Citation8,Citation9,Citation17 There is evidence suggesting that prescribing of opioids is also associated with a lower likelihood of being prescribed guideline-recommended FM medications.Citation2
In addition to the patient burden associated with FM resulting from impaired function, productivity, and quality of life,Citation18–Citation21 health care resource utilization is significantly higher in patients with FM than among non-FM controls.Citation22,Citation23 This greater utilization has a substantial economic impact that also appears to be greater as FM severity increases.Citation24 Identifying drivers of health care resource utilization in patients with FM and factors associated with this use can inform development of strategies to more efficiently manage these patients. The emergence and implementation of electronic health records (EHR) provides an opportunity to explore how these data can be used to evaluate and understand resource utilization in patients with FM.Citation23–Citation26 Such an understanding may provide a first step in developing strategies to improve health care for patients with FM and reduce costs.
EHRs capture longitudinal, patient-level clinical data that represent integral components of provider care, allowing more complete evaluation of a wider range of variables than would be available in health care claims or other observational data sources.Citation25 Included in EHRs are textual data not associated with a diagnosis or other coding, which can be recorded as structured data (e.g., physical assessments) or as unstructured text.Citation25 Thus, an additional strength of EHR is this ability to capture unstructured information in the notes fields, and since many symptoms attributable to FM, such as sleep disturbances, fatigue, and headache, are not necessarily represented in claims, reports of these symptoms can be harvested from the unstructured data.Citation23,Citation25,Citation26 While integration of EHR into the management of FM has previously been suggested as a means to improve patient care,Citation26 only two published studies have applied this technology to FM, both with the purpose of better understanding factors associated with a diagnosis.Citation23,Citation27 Therefore, the purpose of this study was to identify potential drivers of all-cause health care resource utilization and characterize factors associated with high resource use, using a large EHR database to explore data from patients diagnosed with FM.
Methods
Data source
This retrospective analysis used structured and unstructured data from the de-identified Humedica EHR database, which has broad representation across all geographic regions of the US. The database longitudinally captures data from hospitals, medical groups, and integrated delivery networks, including information on demographics, diagnoses, and a complete range of inpatient and outpatient encounters as well as medications ordered. Humedica aggregates de-identified EHR data from providers across the continuum of care. Records are linked using a unique patient identifier and are fully compliant with the Health Insurance Portability and Accountability Act (HIPAA). The database was certified to satisfy conditions set forth in Sections 164.514 (a)-(b)1ii of the HIPAA privacy rule regarding the determination and documentation of statistically de-identified data. Therefore because this study used only de-identified patient records and did not involve the collection, use, or transmittal of individually identifiable data, Institutional Review Board (IRB) approval to conduct this study was not required.
The structured data include demographic information, clinical characteristics, and health care resource utilization. Unstructured data were searched for information on FM pain symptoms using Natural Language Processing (NLP) techniques. The Humedica NLP system was developed using vocabulary from the Unified Medical Language System that includes multiple medical dictionaries such as the Logical Observation Identifiers Names and Codes and the Systemized Nomenclature of Medicine-Clinical Terms. These data are harvested from the notes fields within the EHR, and each NLP concept included in the data is associated with a unique identifier and a date of observation, which also allows longitudinal tracking.
Population
The evaluated population consisted of adults (≥18 years at index) identified with FM based on ≥2 International Classification of Diseases, Ninth Revision codes for FM (729.1) ≥30 days apart between January 1, 2008 and December 31, 2012 and who had ≥12 months continuous care pre- and post-index, with continuous care determined using the “first month active” and “last month active” fields, reflecting the first and most recent months with recorded health care activity events in the Humedica data set. The first reported FM diagnosis was the index event. Patients were excluded if they had evidence during pre- or post-index study periods of a diagnosis, procedure, or an indication of transplant surgery or cancer, except for basal cell or squamous cell skin cancers and benign neoplasms.
Outcomes and analyses
All-cause health care resource utilization during the 12-month post-index period was examined using descriptive statistics (proportions of patients and units of resource) for the resource categories of inpatient admissions, emergency room, medications ordered, physician office visits, physical therapy, radiology, laboratory, and other outpatient visits (ie, excludes physician office visits, radiology, laboratory, and emergency room). For evaluation of medications, pharmacotherapy use was defined as evidence of a National Drug Code on an outpatient prescription order. The medication may have been prescribed for any of the patient’s conditions, including but not limited to FM. Evaluation of opioid use was also stratified as those mentioned in FM guidelines (weak opioids including tramadol, codeine and codeine combinations, and tapentadol) and those not recommended in guidelines.Citation8,Citation9,Citation17 Comorbidities and clinical symptoms, including those reported in prior FM studies, were identified during the pre- and post-index study periods from evidence of an International Classification of Diseases, Ninth Revision, Clinical Modification diagnosis code (for conditions where codes were available) or a positive mention of the comorbidity in the unstructured text of the EHR, and grouped according to categories and nomenclature used in prior FM studies.Citation3,Citation4–Citation7,Citation28
Multivariable analyses using generalized linear models (GLMs)Citation29 were performed with demographic, clinical, and pharmacotherapy factors as covariates to determine how these characteristics related to high resource utilization. For analyzing the number of visits and number of medications, which were counts, negative binomial regression generalized linear modeling with a negative binomial distribution and log link was used. Negative binomial regressions, rather than Poisson regressions, were used because overdispersion of the counts compared with the Poisson is usual with this type of data. Overdispersion was confirmed by examination of scale factors based on the ratio of observed variances compared with those expected under a Poisson model ie, the means. To identify those covariates showing significance, a stepwise regression approach with forward selection was used with P-values of 0.05 for both inclusion and removal of covariates from the model. The level of statistical significance for all statistical tests was 0.05. All statistical analyses were conducted using SAS version 9.4 (SAS Institute Inc., Cary, NC, USA).
Results
Of 265,341 subjects with a diagnosis of FM between January 1, 2008 and December 31, 2012, a total of 64,038 met all other criteria for inclusion in the analysis. This population was primarily female (81.4%) and Caucasian (87.7%), with a mean (standard deviation [SD]) age of 54.4 (14.8) years (). Comorbidities representing a variety of systems were present in substantial proportions of patients (), with hypertension (39.2%), hyperlipidemia (33.6%), arthritis/other arthropathies (33.6%), nausea/vomiting (30.9%), and depression (29.7%) the most frequent conditions pre-index. Similarly, these were the most common comorbidities post-index, although reported in higher percentages of patients (hypertension (53.0%), hyperlipidemia (47.2%), arthritis/other arthropathies (52.6%), nausea/vomiting (45.4%), and depression (46.8%)) ().
Table 1 Demographic characteristics (N=64,038)
Table 2 Clinical characteristics (N=64,038)
The mean (SD) pain score (pain scales were scored 0= no pain to 10= worst pain; the data source did not identify names of specific pain scales used) was 4.5 (3.9), but pain scores, which were taken from the structured data, were only available for 2,321 patients (3.6%) during the pre-index period. Among the 19,350 (30.2%) patients for whom pain scores could be identified in the post-index period, the mean (SD) score was 3.9 (4.8).
As shown in , the health care resource categories used by the highest proportion of patients during the 12-month post-index period, were “medications ordered” (91.6%) and “physician office visits” (87.5%). Approximately half of the patients (50.3%) had “other outpatient visits,” but resources indicative of diagnostic testing (ie, “laboratory” and “radiology”) not included in “other outpatient visits” were used by substantially fewer patients. During this period, each patient had a mean (SD) of 15.1 (18.1) outpatient visits to a physician or clinic (median visits 9; interquartile range [IQR] 4, 20); the number of physician or clinic visits accounted for 73.3% of all health care visits. While 26.6% of patients used emergency room services and 14.7% had hospitalizations, the units of service were low, with 0.8 (2.4) and 0.3 (1.3) visits per patient, respectively. Overall, FM patients visited a health care resource (physician office, clinic, hospital, emergency room, physical therapy, other health care visits), an average of 20.6 (22.6) times (median total health care visits 14; IQR 6, 27), during the post-index year.
Figure 1 Proportion of patients using health care resource categories during the 12-month post-index period among patients with fibromyalgia.
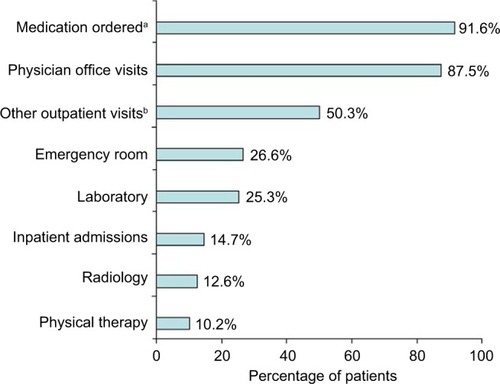
The mean (SD) medication orders per patient was 21.2 (21.5) (median medication orders 16; IQR 7, 29), and encompassed a mean of 11.3 (8.9) unique medications per patient. Among the medications (), opioids were the most frequently prescribed medication class, 44.3% of patients, followed by nonsteroidal anti-inflammatory drugs in 34.4%. Except for statins (24.4%), most of the drugs with high rates of prescribing were those that are often associated with pain (), including anti-epileptics (27.4%) and a variety of antidepressants, ranging from tricyclic antidepressants (10.2%) to selective serotonin reuptake inhibitors (20.0%).
Figure 2 Medications by class prescribed during the 12-month post-index period among patients with fibromyalgia.
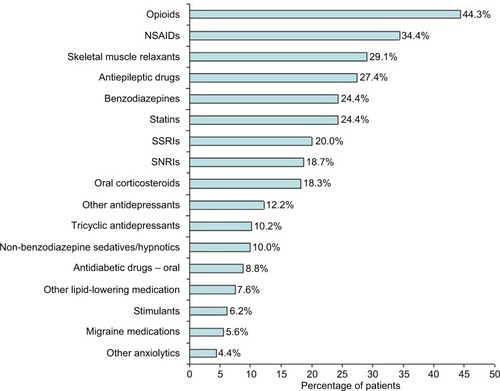
When opioids were stratified by those within FM treatment guidelines (ie, weak opioids), just over one-third of the patients (38.3%) who were prescribed opioids received weak opioids, representing 16.9% of the overall population. However, 23,347 patients (82.4% of patients receiving opioid orders) were prescribed opioids not within FM guidelines, although individual patients could be receiving multiple opioids, including those within and not within the guidelines. Patients receiving opioid orders had a mean of 2.8 (3.0) prescriptions for opioids within FM guidelines and 5.8 (7.2) prescriptions for opioids not within guidelines during the 12-months post-index.
The results from negative binomial regression of the total number of all-cause post-index health care visits () found the pre-index total number of health care visits to have the greatest association with higher post-index total health care visits (incident rate ratio 1.36). The model further showed that risk of higher health care resource utilization indicated by a higher number of total health care visits was significantly increased by 26% (P<0.001) among African-Americans () relative to the comparator group (Caucasians), that is, an incident rate ratio (IRR) indicating 1.26 times as many health care visits. Similarly, there were several comorbid conditions associated with increased number of health care visits, ranging from a 6% increase for musculoskeletal pain and depression/bipolar disorder (both IRR 1.06) to a 21% increase (IRR 1.21) for congestive heart failure (all P<0.001). Ordering of opioids not within FM guidelines was also associated with a 12% increase (IRR 1.12) in health care visits relative to those with orders for opioids within FM guidelines or without any opioid orders (P<0.001).
Table 3 Factors associated with total number of all-cause health care visits during 12-month post-index period
The results from the negative binomial regression of number of prescriptions () showed that the covariate associated with the greatest increase in prescriptions was being female, having an IRR with 23% more prescriptions than males. As with the factors contributing to overall health care resource utilization, the presence of specific comorbid conditions was also associated with increased medication prescriptions ().
Table 4 Factors associated with total number of medication orders during 12-month post-index period
Discussion
This analysis provides a practical example of how EHR may be used to characterize health care resource utilization and identify variables associated with high resource use. Consistent with studies using other data sources, this study found FM patients to be frequent users of health care resources and medications.Citation10,Citation11,Citation14,Citation20,Citation24 In this large population of FM patients, whose demographic characteristics were consistent with what may be expected for an FM population (ie, females of primarily middle age),Citation7 the primary drivers of all-cause health care resource utilization were “medications ordered” and “physician office visits.” These resource categories were also characterized by high units of use per patient in the post-index period. In particular, medication utilization showed that, on average, patients were prescribed 21.2 medication orders for 11.3 different medications during the 12-month post-index period.
This high medication utilization may be related to the fact that these patients had a substantial comorbidity burden. This burden not only consisted of conditions that have been typically reported as being associated with FM, including numerous other musculoskeletal pain conditions and gastrointestinal disorders, but also conditions that have not generally been considered to be associated with FM such as hypertension and hyperlipidemia.Citation3,Citation4 The presence of conditions not necessarily associated with FM may not be surprising given the age demographic of this population, and yet other studies have found the same comorbidities in similar percentages of FM patients.Citation6,Citation11,Citation20,Citation24,Citation28,Citation30
As has been reported in previous studies of FM,Citation2,Citation10–Citation14 opioids were the most frequently prescribed medication class. Notably, the proportion of patients prescribed opioids not mentioned in FM guidelines was approximately twice the proportion who were prescribed opioids within guidelines. This is the first analysis to suggest and to quantify that such opioid prescribing may be associated with an increase in resource utilization in these patients. The reason for this association was not determined, but it has been reported that FM patients taking opioids have poorer outcomes than nonopioid users,Citation31 which may drive additional health care-seeking behavior. Recent studies have found improved scores for pain severity, pain interference, patient function, insomnia, disability severity, and depression severity in FM patients not taking opioids compared with those taking opioids.Citation15,Citation32 A recent observational study reported that the prescribing of opioids after FM diagnoses significantly reduced the likelihood of receiving a guideline-recommended medication.Citation2 Our findings of a 12% increase in health care visits for patients receiving opioids not within FM guidelines provide further evidence of the impact on health care resources in diagnosing and managing this complex condition, from the initial evaluation of patient’s need for such opioids to the follow-up and monitoring of their effectiveness.
While antidepressants from various classes were also commonly prescribed, almost half of the patients (46.8%) had a diagnosis of depression in the post-index period, so it is likely that at least some antidepressant prescriptions may have been for depression, which is a commonly reported comorbid condition in FM.Citation6,Citation11,Citation14,Citation20,Citation24 Reasons for prescribing medications are inconsistently captured in EHR databases due to variations in current data entry practices, ie, providers using EHRs in the US are not required to provide reasons for each medication order and so commonly not entered.
An important observation is that, while use of NLP enabled a search for patients who had documented pain scores, these were inconsistently formatted and reported. Pain severity was documented among only 3.6% of patients during the 12 months prior to FM diagnosis, despite substantial proportions of patients with pain-related comorbidities such as painful musculoskeletal conditions and headache. While the proportion of patients with pain scores in the post-index period was higher, potentially indicating a greater rate of pain assessment after FM diagnosis, the proportion with a documented pain assessment any time during the 12-month post-index period was nevertheless less than one-third (30.2%) of the FM population. Such inconsistent and infrequent documentation makes it difficult to evaluate changes in pain severity over time, and also highlights the likelihood that among patients with this pain condition, the presence and severity of pain may not be regularly evaluated and/or recorded. Whether this lack of pain evaluation is limited to FM or is widespread in other pain conditions is not known, since there are no known studies that have evaluated the frequency of use of pain measures in usual clinical practice among patients with chronic pain conditions. Nonetheless, the low availability of pre-index pain scores indicates that the lack of pain assessment reporting is common.
Limitations
Although a strength of this study was its use of data derived from EHR, especially since EHRs are now operational in most health systems and with data not otherwise available from other observational sources, the data reported here are subject to potential errors in coding or record keeping at the point of the health care provider, as is the case with all database analyses. Therefore, ≥2 International Classification of Diseases, Ninth Revision codes were used to identify the evaluated population in order to minimize errors in FM coding and to improve the accuracy of identifying the FM cohort. However, it is also possible that there were patients with FM who were excluded from the analysis based on this more conservative inclusion criterion. Furthermore, EHR datasets only document the order by the health care provider and are not confirmatory that the order was followed. This limitation may be especially relevant for pharmacotherapy; medications ordered by the health care provider and documented in the EHR may not necessarily have been filled or administered to patients. Another limitation is that since resource utilization was regardless of cause, no implications can be made as to what extent the resources used were associated directly with FM. In this regard, since many of these patients had comorbidities, the prescribing of a specific medication could have been for a condition other than FM.
Conclusion
Using data from EHR, substantial all-cause health care resource utilization was observed among FM patients. Physician office visits and pharmacotherapy were identified as the two main drivers of all-cause health care resource utilization, and opioids were the most frequently prescribed medication class overall. While two demographic factors (female and African-American) were associated with high resource use, the majority of variables associated with greater health care resource utilization were comorbid conditions representing a variety of organ systems. However, prescribing of opioids was also associated with greater health care resource utilization. These results suggest that EHR can be a useful tool for identifying patients with high health care resource utilization. Such identification represents an important first step in determining appropriate strategies for potentially managing patients with FM with a goal of improving patient outcomes and reducing medical costs.
Acknowledgments
The authors gratefully acknowledge the important contributions of Douglas Weldon of Truven Health Analytics and Erin Thomson, formerly of Truven Health Analytics, for their roles in the research design and analysis of data.
This study was sponsored by Pfizer. Editorial/medical writing support was provided by E Jay Bienen and was funded by Pfizer.
Disclosure
Elizabeth T Masters, Joseph C Cappelleri, and Steven Faulkner are employees and stockholders of Pfizer. Jay M Margolis and David M Smith are employees of Truven Health Analytics which was paid by Pfizer in connection with the development of this manuscript. The authors report no other conflicts of interest in this work.
References
- ClauwDJArnoldLMMcCarbergBHFibroCollaborativeThe science of fibromyalgiaMayo Clin Proc201186990791121878603
- HalpernRShahSNCappelleriJCMastersETClairAEvaluating guideline-recommended pain medication use among patients with newly diagnosed fibromyalgiaPain Pract201616810271039
- WolfeFClauwDJFitzcharlesM-AThe American College of Rheumatology preliminary diagnostic criteria for fibromyalgia and measurement of symptom severityArthritis Care Res2010625600610
- BennettRMClinical manifestations and diagnosis of fibromyalgiaRheum Dis Clin North Am200935221523219647138
- VincentALahrBDWolfeFPrevalence of fibromyalgia: a population-based study in Olmsted County, Minnesota, utilizing the Rochester Epidemiology ProjectArthritis Care Res (Hoboken)201365578679223203795
- WalittBNahinRLKatzRSBergmanMJWolfeFThe prevalence and characteristics of fibromyalgia in the 2012 National Health Interview SurveyPLoS One2015109e013802426379048
- WolfeFRossKAndersonJRussellIJHebertLThe prevalence and characteristics of fibromyalgia in the general populationArthritis Rheum199538119287818567
- CarvilleSFArendt-NielsenSBliddalHEULAR evidence based recommendations for the management of fibromyalgia syndromeAnn Rheum Dis200867453654117644548
- FitzcharlesMASte-MariePAGoldenbergDLNational Fibromyalgia Guideline Advisory Panel2012 Canadian Guidelines for the diagnosis and management of fibromyalgia syndrome: executive summaryPain Res Manag201318311912623748251
- WhiteLARobinsonRLYuAPKaltenboeckASamuelsSMallettDBirnbaumHGComparison of health care use and costs in newly diagnosed and established patients with fibromyalgiaJ Pain200910997698319556168
- BergerASadoskyADukesEMEdelsbergJZlatevaGOsterGPatterns of health care utilization and cost in patients with newly diagnosed fibromyalgiaAm J Manag Care201016SupplS126S13720586521
- McNettMGoldenbergDSchaeferCHufstaderMBaikRChandranAZlatevaGTreatment patterns among physician specialties in the management of fibromyalgia: results of a cross-sectional study in the United StatesCurr Med Res Opin201127367368321294700
- BernauerMWuNChenSYPengXBoulangerLZhaoYUse of select medications prior to duloxetine initiation among commercially-insured patientsJ Pain Res20155271278
- SchaeferCMannRMastersETThe comparative burden of chronic widespread pain and fibromyalgia in the United StatesPain Pract201616556557925980433
- PengXRobinsonRLMeasePLong-term evaluation of opioid treatment in fibromyalgiaClin J Pain201531171324480913
- PainterJTCroffordLJChronic opioid use in fibromyalgia syndrome: a clinical reviewJ Clin Rheumatol2013192727723364665
- FitzcharlesMASte-MariePAGoldenbergDL2012Canadian guidelines for the diagnosis and management of fibromyalgia syndrome Available from: http://fmguidelines.ca/?page_id=21Accessed April 8, 2016
- HoffmanDLDukesEThe health status burden of people with fibromyalgia: a review of studies that assessed health status with the SF-36 or the SF-12Int J Clin Pract200862111512618039330
- LuoXCappelleriJCChandranAThe burden of fibromyalgia: assessment of health status using the EuroQol (EQ-5D) in patients with fibromyalgia relative to other chronic conditionsHealth Outcomes Res Med201124e203e214
- SchaeferCChandranAHufstaderMThe comparative burden of mild, moderate and severe fibromyalgia: results from a cross-sectional survey in the United StatesHealth Qual Life Outcomes2011917121859448
- McDonaldMDiBonaventuraMDUllmanSJPMusculoskeletal pain in the workforce: the effects of back, arthritis and fibromyalgia pain on quality of life and work productivityJ Occup Environ Med201153776577021685799
- KnightTSchaeferCChandranAZlatevaGWinkelmannAPerrotSHealth-resource use and costs associated with fibromyalgia in France, Germany, and the United StatesClinicoecon Outcomes Res2013517118023637545
- MastersETMardekianJEmirBClairAKuhnMSilvermanSElectronic medical record data to identify variables associated with a fibromyalgia diagnosis: importance of health care resource utilizationJ Pain Res2015813113825784819
- ChandranASchaeferCRyanKBaikRMcNettMZlatevaGThe comparative economic burden of mild, moderate, and severe fibromyalgia: results from a retrospective chart review and a cross-sectional survey of working-age U.S. adultsJ Manag Care Pharm201218641542622839682
- HayrinenKSarantoKNykanenPDefinition, structure, content, use and impacts of electronic health records: a review of the research literatureInt J Med Inform200877529130417951106
- WellsAFArnoldLMCurtisCEDuneganLJLappCWMcCarbergBHClairAIntegrating health information technology and electronic health records into the management of fibromyalgiaPostgrad Med20131254707723933895
- EmirBMastersETMardekianJClairAKuhnMSilvermanSLIdentification of a potential fibromyalgia diagnosis using random forest modeling applied to electronic medical recordsJ Pain Res2015827728826089700
- GoreMTaiKSChandranAZlatevaGLeslieDClinical comorbidities, treatment patterns, and health care costs among patients with fibromyalgia newly prescribed pregabalin or duloxetine in usual careJ Med Econ2012151193121970699
- DobsonABarnettAAn Introduction to Generalized Linear Models3rd edBoca Raton, FLChapman & Hall2008
- VincentAWhippleMOMcAllisterSJAlemanKMSt SauverJLA cross-sectional assessment of the prevalence of multiple chronic conditions and medication use in a sample of community-dwelling adults with fibromyalgia in Olmsted County, MinnesotaBMJ Open20155e006681
- GoldenbergDLClauwDJPalmerREClairAGOpioid use in fibromyalgia: a cautionary taleMayo Clin Proc201691564064826975749
- FitzcharlesMAFareghNSte-MariePAShirYOpioid use in fibromyalgia is associated with negative health related measures in a prospective cohort studyPain Res Treat2013201389849323577251