Abstract
Background
Accurate identification of specific patient populations is a crucial tool in health care. A prerequisite for exploring the actions taken by general practitioners (GPs) on symptoms of cancer is being able to identify patients urgently referred for suspected cancer. Such system is not available in Denmark; however, all referrals are electronically stored. This study aimed to develop and test an algorithm based on referral text to identify urgent cancer referrals from general practice.
Methods
Two urgently referred reference populations were extracted from a research database and linked with the Primary Care Referral (PCR) database through the unique Danish civil registration number to identify the corresponding electronic referrals. The PCR database included GP referrals directed to private specialists and hospital departments, and these referrals were scrutinized. The most frequently used words were integrated in the first version of the algorithm, which was further refined by an iterative process involving two population samples from the PCR database. The performance was finally evaluated for two other PCR population samples against manual assessment as the gold standard for urgent cancer referral.
Results
The final algorithm had a sensitivity of 0.939 (95% confidence intervals [CI]: 0.905–0.963) and a specificity of 0.937 (95% CI: 0.925–0.963) compared to the gold standard. The positive and negative predictive values were 69.8% (95% CI: 65.0–74.3) and 99.0% (95% CI: 98.4–99.4), respectively. When applying the algorithm on referrals for a population without earlier cancer diagnoses, the positive predictive value increased to 83.6% (95% CI: 78.7–87.7) and the specificity to 97.3% (95% CI: 96.4–98.0).
Conclusion
The final algorithm identified 94% of the patients urgently referred for suspected cancer; less than 3% of the patients were incorrectly identified. It is now possible to identify patients urgently referred on cancer suspicion from general practice by applying an algorithm for populations in the PCR database.
Introduction
Cancer is a major healthcare burden in Denmark as in most other countries.Citation1 Many resources are thus allocated to research and quality improvement of the entire cancer care pathway. Urgent referral for cancer investigation was introduced in Denmark in 2008 to reduce the waiting time from first symptom presentation to treatment initiation, particularly the interval from referral to treatment.Citation2 Nevertheless, Denmark still lags behind most of the European countries in cancer survival.Citation3 Research has revealed that high use of urgent referrals among general practitioners (GPs) and short time to diagnosis may improve the prognosis.Citation4–Citation8 However, selecting patients for urgent cancer referral is a complex process; each GP is involved in the diagnosis of only 8–10 new cancer patients per year, but patients with potential cancer symptoms consult GPs on a daily basis.Citation9,Citation10 A Scottish study found a 6-fold variation in the use of urgent referrals among GPs, after excluding the highest and lowest 10% of the referring practices.Citation11 Thus, identifying the patients who have been urgently referred from general practice is crucial for achieving better insight into the use of urgent referrals for suspected cancer among GPs and the potential implications for cancer survival rates. Such new insight is of particular interest in healthcare systems, where GPs act as gatekeepers and initiators of the diagnostic pathway for 85% of all cancer patients.Citation12–Citation14
Urgent cancer referrals from general practice are currently not registered systematically in Denmark (like in the UK).Citation15 Unpublished studies have found that the ad hoc registration at Danish hospitals cannot validly identify all urgent referrals from GPs and cannot appropriately distinguish between urgent referrals from GPs and those from other healthcare professionals. Hospital consultants can redirect an urgent referral to a different route or convert an ordinary referral requested by a GP to an urgent referral. A sustainable method to identify urgent referrals from general practice is warranted: a way forward could be to exploit the systematically collected routine data from the existing electronic referral system, which is used for all referrals to private specialists and hospitals. These data have not been previously used for research and monitoring purposes.
The aim of this study was to develop and test a text-based algorithm for searching all referrals to identify urgent cancer referrals from general practice.
Methods
Setting and referral procedure
The study was conducted in one of the five Danish regions; the Central Denmark Region with 1.3 million inhabitants, 417 GP clinics with 831 GPs and ~8,000 new cancer patients annually. The Danish healthcare system provides free (tax-funded) access to general practice and hospital care, and most Danish citizens (98%) are listed with a particular general practice. The GP acts as gatekeeper to the rest of the healthcare system by referring patients to hospitals or private specialists when relevant.Citation14
An online guideline for urgent cancer referral describes the symptoms for several specific types of cancer; this also includes clinical and para-clinical findings that could release an urgent cancer referral from the GP. The guideline requests the referring GP to include the term “cancer” in the diagnostic text of the referral.Citation16
All Danish GPs use computerized administrative systems with electronic patient records. These systems communicate electronically with the rest of the healthcare system, including all referrals from general practices and private specialists. A copy of each referral is stored in the Central Referral Database.
Data
The Primary Care Referral (PCR) Database
The PCR database was established in 2014 by extracting referrals made by GPs from the Central Referral Database. Patient names, addresses, and phone numbers were deleted to ensure that all referrals included only one unique identifier: the civil registration (CPR) number.Citation17
The PCR database included information about referral date, referring GP, receiving department, text fields for diagnostic, patient history and clinical information, patient’s age and gender, and patient’s CPR number in encrypted form. The PCR database did not include referrals for radiology departments.
Populations derived from the PCR database
The PCR database had not been previously used for research purposes. The authors gradually increased their knowledge about the database during the study, which allowed them to further restrict the selection criteria to achieve the most optimal target population (). The sampling period for the populations was arbitrary; although no periods were overlapping. The sample size of each population was selected to ensure small confidence intervals (CIs) on the estimates.
Table 1 Characteristics of the four PCR-P
The PCR populations were used in the last two steps of the incremental development and in the subsequent evaluation of the algorithm. In order to be able to measure the performance of each stepped algorithm, a gold standard for urgent cancer referrals was developed. Each sampled referral was assessed by a researcher (BST) and categorized as “urgent cancer referral” or “not urgent cancer referral.” In case of any doubt, another researcher (LMG) conducted an independent assessment. In case of disagreement (<2% of all cases), referral texts were discussed until consensus had been reached on categorization. Referrals categorized as “not urgent cancer referral” were further subcategorized to obtain data on the reasons for false-positive findings. The assessors were blinded to the results of the stepped algorithms.
Primary Care Referral Population I (PCR-P I)
One thousand unique consecutive patients were selected from the PCR database from 4 March 2013 to 14 March 2013. The referral requests that have been included should have been submitted by a Danish general practice and directed to a hospital department. No other criteria were used in the selection. This population was labelled the PCR-P I.
Primary Care Referral Population II (PCR-P II)
Two thousand unique consecutive patients aged ≥40 years were selected from the PCR database from 1 February 2013 to 8 February 2013. The referral requests that have been included should have been submitted by a general practice from the Central Denmark Region and directed to a medical unit located in the Central Denmark Region. Such medical unit could be a somatic hospital department or one of the following (privately practicing) medical specialties, which also form part of the national urgent cancer pathways: otorhinolaryngology, dermatology, gynecology, surgery, plastic surgery, or neurology. This population was labelled the PCR-P II.
Primary Care Referral Population III (PCR-P III)
The third population included 2,500 unique consecutive patients with the same inclusion criteria for receivers as for the PCR-P II (described earlier). This population was selected from the PCR database from 9 February 2013 to 18 February 2013; 328 patients referred from GPs in out-of-hours services were excluded, which resulted in a total of 2,172 patients (all referred from GPs during daytime). This population was labelled the PCR-P III.
Primary Care Referral Population IV (PCR-P IV)
A total of 1,949 patients were selected on the basis of the same inclusion criteria as applied to the PCR-P III, except that patients with prior cancer diagnosis identified in the Danish Cancer Registry (ICD 10, chapter II, C0–C9 except from C44) were excluded.Citation18 This population was labelled the PCR-P IV.
The Cancer Referral Reference (CRR) database
Data from a parallel research study, which aimed to evaluate the impact of a continuing medical education in early cancer diagnosis, formed the basis of the CRR database. This database consisted of records on patients known to have been urgently referred for suspected cancer. The data were collected during an 8-month period from 1 September 2012 to 30 April 2013 by requesting all GPs in the Central Denmark Region to complete a one-page form with patient information each time they urgently referred a patient for cancer investigation.Citation19 The form comprised the patient’s CPR number, the referral date, and the type of urgent cancer pathway.
Populations derived from the CRR database
Two populations were extracted from the CRR database; these were labelled the Cancer Referral Reference Population I (CRR-P I) and the Cancer Referral Reference Population II (CRR-P II). From 1 to 20 September 2012, 127 consecutive patients were identified for the CRR-P I. From 1 November 2012 to 4 January 2013, 496 consecutive patients were identified for the CRR-P II.
Patients referred to the fast-track pathway for suspected breast cancer were excluded as their referrals were directed to radiology departments and thus would not be stored in the PCR database. For each urgently referred patient, GP referrals in the PCR database were identified within a period of ±2 weeks surrounding the referral date registered by the GP. Patients could be included only once (the first referral). The CRR populations were used in the first two steps of the development of the algorithm.
Algorithm development and stepwise refinement
To identify text fields and words used by GPs which could identify urgent referrals, populations known to be urgently referred (CRR-P I and CRR-P II) were used. To develop an algorithm that would best balance sensitivity (proportion of true positives correctly identified as such) and specificity (proportion of true negatives correctly identified as such), unselected populations (PCR-P I and PCR-P II) were used.Citation20
In order to avoid that the performed refinements and measured performance of the stepped algorithms would be tailored to a specific population, a new population was used for each successive step of the development. It was considered important that the populations (PCR-P III and PCR-P IV) were “unknown” in the developing steps of the algorithm in order to validly measure the performance of the final algorithm.
Step 1: Identifying search fields and most frequent words in urgent cancer referrals
Two researchers (BST and LMG) independently reviewed the 127 CRR-P I referrals and identified the text fields that were most frequently used by GPs while filling out an urgent cancer referral. Five fields were identified, namely diagnosis, supplementary diagnoses, patient history (anamnesis), supplementary information and supplementary clinical information (Supplementary material). Each referral was then thoroughly examined to identify the most commonly used words linked to cancer suspicion. The selected words were “pakke” (Danish term for cancer pathway), “cancer,” “malignant,” general medical terms such as “tumor” and “lump,” and cancer-specific terms such as “leukemia” and “lymphoma.” In order to embrace typical misspellings, spelling variations, and use of abbreviations, letter strings of the identifying words were made as short as possible. A step-1 algorithm was developed; this included the most frequently used strings for cancer, cancer pathways, and cancer types.
Step 2: Refining the step-1 algorithm to enhance the sensitivity
The step-1 algorithm was applied to the CRR-P II. Strings were then identified and included until the step-2 algorithm appeared saturated and obtained a sensitivity >95%. Among the added strings were symptoms such as “hemoptysis,” “hematuria,” “rectal bleeding,” and procedures like “colonoscopy” and “gastroscopy”.
Step 3: Refining the step 2-algorithm by differentiated search
The step-2 algorithm was applied to the PCR-P I. A frequent reason for false-positive (incorrectly identified) findings was the search fields “anamnesis,” “additional information,” or “additional diagnoses.” Therefore, specifically problematic combinations of words and fields were identified in an iterative process by reviewing text, omitting fields in a new algorithm, and performing repeated calculations of specificity and sensitivity. The increase in specificity was achieved under strict supervision to ensure the lowest possible drop in sensitivity.
Step 4: Refining the step-3 algorithm by excluding misleading strings
The step-3 algorithm was applied to the PCR-P II to further reduce the false-positive rates. Combinations of words that were especially problematic were identified. For example, “pakke,” which was one of the strongest identification words, was also used in combination with “heart” and “blood test.” Therefore, the algorithm was set to exclude the identified case if “pakke” appeared in the same text field as “heart,” “angina,” or “rheumatic.” These combinations of identifying words were gradually incorporated to maximize the specificity while maintaining a sensitivity >93%.
Step 5: Assessing the performance of the final algorithm
The final algorithm was applied to the PCR-P III and the PCR-P IV to calculate the performance. The two populations comprised patients aged ≥40 years who had been referred from general practice in the daytime; one included patients with prior cancer diagnosis (PCR-P III), whereas the other (PCR-P IV) did not.
In order to test the consistency of the performance at each step, the stepped algorithms were applied to each successive population (). The five steps in the development of the algorithm are shown in .
Figure 1 The stepwise development of the algorithm.
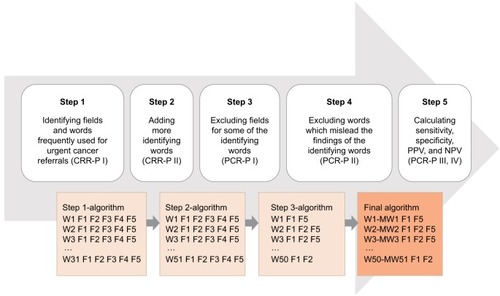
Table 2 The performance of the stepped algorithms on each population
Statistics
The populations were described in terms of inclusion criteria for requester, receiver and patient, number of patients, patient age (median and interquartile intervals), proportion of males, and urgent cancer referrals for all referred patients according to manual assessment with a CI of 95%.
As the stepped algorithms provided dichotomous outcomes in terms of urgent cancer referral (“yes” or “no”), simple performance statistics was applied to calculate the sensitivity, specificity, positive predictive value (PPV; proportion of test positives that are truly positive) and negative predictive value (NPV; proportion of test negatives that are truly negative) with 95% CI for each of the stepped algorithms to allow for comparisons.Citation20 Coding and analyses were performed using Stata version 13.0 software (StataCorp LP, College Station, TX, USA).
Ethical approval
The study was approved by the Danish Data Protection Agency (file no. 2009-41-3471). The establishment of the PCR database was approved by Danish Regions. The collection of data for the CRR database was approved by the Danish Health Authority (file no. 3-3013-149/1/HKR) and the Danish Data Protection Agency, the Central Denmark Region (file no. 1-16-02-262-12).
Results
Identification strings and search fields are provided for each of the four-stepped algorithms. The final algorithm consisted of 50 identifying words; 35 were combined with the exclusion of misleading words.
Gradual improvement of the first and second algorithms increased the sensitivity and the NPV, whereas the specificity and the PPV decreased. Specificity and PPV were both improved from the third to the final version of the algorithm ().
The final algorithm, which was applied to the PCR-P III, correctly identified 275 referrals as urgent cancer referrals and 1,760 as “not urgent cancer referrals.” However, it misclassified 119 referrals as “urgent cancer referrals” and 18 as “not urgent cancer referrals” ().
Three out of ten urgent cancer referrals included the word “cancer”; one of the words (defined as “cancer wording”) “kræft” (Danish term for cancer), “cancer,” “pakke,” or “c.” (abbreviation for cancer) was included in half of the referrals (). The most frequent identifying words that resulted in a false-positive finding were “kræft,” “cancer,” and “malign” (). These words could not be removed without decreasing the sensitivity of the algorithm. False-positive findings were mostly related to previously diagnosed cancer disease, earlier cancer investigation, hereditary cancer predisposition, or fear of cancer ().
Table 3 True- and false-positive findings of important identifying words from the final algorithm
Table 4 Subgroup categories of false-positive findings of the final algorithm
Exclusion of patients with prior cancer in the PCR-P IV implied that the PPV of the final algorithm increased to 83.6% (95% CI: 78.7–87.7), the specificity increased to 0.973 (95% CI: 0.964–0.980), whereas the sensitivity remained unchanged at 0.936 (95% CI: 0.898–0.963) ().
Discussion
Main findings
This study developed an algorithm capable of identifying urgent cancer referrals among all electronic referrals from Danish general practice to hospitals and private specialists. When applied for patients ≥40 years of age who were not diagnosed with cancer earlier, the algorithm was capable of identifying 93.6% of all urgent cancer referrals; <3% were false positives, and a PPV of 84% was obtained. This provides an acceptably low misclassification rate for use in epidemiological research and quality development.
Strengths and weaknesses
The strength of the algorithm development was the stepwise approach, which ensured optimal balance between sensitivity and specificity. However, this balance depends on the specific purpose. A higher sensitivity could be obtained (eg, to ensure that all urgently referred patients are included), but this would require acceptance of lower specificity and PPV. In epidemiological research and quality development, fairly high specificity and PPV were generally required to enable restriction. It was realized that some urgently referred patients might have been missed in this attempt to ensure that all included patients had actually been urgently referred.
Urgent referrals for breast cancer could not be identified as these referrals were generally directed directly to a radiology department and not stored in the PCR database. Manual assessment, which established the gold standard, was made before applying the algorithm to the data, and the assessors were blinded to the results of the algorithm in order to ensure that misclassification was reduced to a minimum. The process was initiated by reviewing referrals that were known to be urgent cancer referrals (the CRR populations). This provided the insight that “cancer wording” is stated in only half of all referrals, and thus it was known that more cancer identification words than originally anticipated had to be included. The method based on actual referrals is considered more precise than identification of frequently used words through, for example, interviews or brainstorming sessions with GPs.
The measured performance of the final algorithm can be generalized to the rest of Denmark as there is no reason to believe that GPs in the Central Denmark Region communicate urgent referrals differently than other Danish GPs. Nevertheless, the GPs may improve their wording over time while writing an urgent referral letter and more often remember to include “cancer” in the diagnostic text as they are informed to do so in the guideline.Citation16 Such development would require regular adaptations of the algorithm based on measurements of performance.
One weakness of using a text-based algorithm to identify patients in the PCR database is the potential variation among GPs in the way they make referrals and communicate through referrals. The findings in this study suggest that it could be helpful to introduce a specific template for each urgent cancer pathway in the electronic referral system as this would require a simpler algorithm and allow distinction between different cancer pathways.
Implications and comparison with literature
Being able to identify the patients who have been urgently referred for suspected cancer from general practice opens up some new possibilities for register-based retrospective research. More accurate knowledge on cancer-related referral activity including referral, conversion, and detection rates in primary care may contribute to a better and more coherent healthcare organization.Citation15
Text-based algorithms can be developed and applied to the PCR database for other research purposes, for example, to investigate diabetes or thyroid disorders. Other countries with an electronic referral system may use the explained stepped approach to develop a comparable algorithm. The essential studies on PPVs for symptoms of cancer and the QCancer web tool are based on word recognition from routinely collected patient data in a large number of GP clinics in England.Citation21–Citation29 However, to our knowledge, no other studies have reported the methodological development of an algorithm aiming to sample specific patient populations through pattern recognition of word strings and text fields in referral letters.
Conclusion
This study has successfully developed an algorithm that identifies 94% of all patients urgently referred for suspected cancer. The PPVs were 69% for all adults aged ≥40 years and 84% when the cases with prior cancer were excluded.” The final algorithm helps to identify patients urgently referred for suspected cancer, and it can be used to monitor the cancer-related referral performance in general practice. The algorithm may constitute a valuable research tool in future studies as it could facilitate evaluations of the performance in general practice and the outcome for the patients. Similar algorithms may be developed for other types of disease.
Author contributions
KF and HB established the central referral database and the PCR database. BST and LG developed the algorithm supported by PV and FB. BST evaluated the algorithm and wrote the first draft of the article. PV, FB, LG, KF, and HB critically reviewed the article. All the authors read and commented on the final manuscript.
Acknowledgments
The authors thank the GPs in the Central Denmark Region, Anne Gammelgaard at Quality and Informatics in the Central Denmark Region, and the four radiology departments in Aarhus, Holstebro, Randers, and Viborg for providing the information to build the CRR database. This work was supported by the Novo Nordisk Foundation, the Danish Cancer Society, and the Foundation for Primary Health Care Research (Praksisforskningsfonden) in the Central Denmark Region (grant no. 1-15-1-72-13-09).
Disclosure
The authors report no conflicts of interest in this work.
References
- EngholmGFerlayJChristensenNNORDCAN – a Nordic tool for cancer information, planning, quality control and researchActa Oncol201049572573620491528
- ProbstHBHussainZBAndersenOCancer patient pathways in Denmark as a joint effort between bureaucrats, health professionals and politicians – a national Danish projectHealth Policy20121051657022136810
- ColemanMPFormanDBryantHCancer survival in Australia, Canada, Denmark, Norway, Sweden, and the UK, 1995–2007 (the International Cancer Benchmarking Partnership): an analysis of population-based cancer registry dataLancet2011377976012713821183212
- TorringMLFrydenbergMHamiltonWHansenRPLautrupMDVedstedPDiagnostic interval and mortality in colorectal cancer: U-shaped association demonstrated for three different datasetsJ Clin Epidemiol201265666967822459430
- NealRDTharmanathanPFranceBIs increased time to diagnosis and treatment in symptomatic cancer associated with poorer outcomes? Systematic reviewBr J Cancer2015112SupplS92S10725734382
- TorringMLFrydenbergMHansenRPOlesenFVedstedPEvidence of increasing mortality with longer diagnostic intervals for five common cancers: a cohort study in primary careEur J Cancer20134992187219823453935
- RichardsMASmithPRamirezAJFentimanISRubensRDThe influence on survival of delay in the presentation and treatment of symptomatic breast cancerBr J Cancer199979585886410070881
- MollerHGildeaCMeechanDRubinGRoundTVedstedPUse of the English urgent referral pathway for suspected cancer and mortality in patients with cancer: cohort studyBMJ2015351h510226462713
- HamiltonWCancer diagnosis in primary careBr J Gen Pract20106057112112820132704
- NielsenTNHansenRPVedstedPSymptom presentation in cancer patients in general practiceUgeskr Laeger20101724128272831 Danish20961502
- BaughanPKeatingsJO’NeillBUrgent suspected cancer referrals from general practice: audit of compliance with guidelines and referral outcomesBr J Gen Pract20116159270070622054332
- AllgarVLNealRDDelays in the diagnosis of six cancers: analysis of data from the national survey of NHS patients: cancerBr J Cancer200592111959197015870714
- HansenRPVedstedPSokolowskiISondergaardJOlesenFTime intervals from first symptom to treatment of cancer: a cohort study of 2,212 newly diagnosed cancer patientsBMC Health Serv Res201111128422027084
- PedersenKMAndersenJSSondergaardJGeneral practice and primary health care in DenmarkJ Am Board Fam Med201225Suppl 1S34S3822403249
- MeechanDGildeaCHollingworthLRichardsMARileyDRubinGVariation in use of the 2-week referral pathway for suspected cancer: a cross-sectional analysisBr J Gen Pract20126260259059723211163
- PraksisinformationenRMKræftpakker Region Midtjylland [Guidelines for fast track pathways in the Central Denmark Region] Available from: https://www.sundhed.dk/sundhedsfaglig/praksisinformation/almen-praksis/midtjylland/patientforloeb/kraeftpakker/Accessed May 9, 2016
- PedersenCBThe Danish Civil Registration SystemScand J Public Health2011397222521775345
- GjerstorffMLThe Danish Cancer RegistryScand J Public Health2011397424521775350
- ToftegaardBBroFVedstedPA geographical cluster randomised stepped wedge study of continuing medical education and cancer diagnosis in general practiceImplement Sci20149115925377520
- KirkwoodBRSterneJACChapter 36: Measurement error: assessment and statisticsEssential Medical Statistics2nd edBlackwell Publishing2003429432
- HamiltonWThe CAPER studies: five case-control studies aimed at identifying and quantifying the risk of cancer in symptomatic primary care patientsBr J Cancer2009101Suppl 2S80S8619956169
- HamiltonWPetersTJBankheadCSharpDRisk of ovarian cancer in women with symptoms in primary care: population based case-control studyBMJ20093390959–535b299819706933
- HamiltonWKernickDClinical features of primary brain tumours: a case-control study using electronic primary care recordsBr J Gen Pract20075754269569917761056
- HamiltonWSharpDJPetersTJRoundAPClinical features of prostate cancer before diagnosis: a population-based, case-control studyBr J Gen Pract20065653175676217007705
- HamiltonWPetersTJRoundASharpDWhat are the clinical features of lung cancer before the diagnosis is made? A population based case-control studyThorax200560121059106516227326
- HamiltonWRoundASharpDPetersTJClinical features of colorectal cancer before diagnosis: a population-based case-control studyBr J Cancer200593439940516106247
- ShephardEAStapleySNealRDRosePWalterFMHamiltonWTClinical features of bladder cancer in primary careBr J Gen Pract201262602598604
- Hippisley-CoxJCouplandCSymptoms and risk factors to identify men with suspected cancer in primary care: derivation and validation of an algorithmBr J Gen Pract201363606e1e1023336443
- Hippisley-CoxJCouplandCSymptoms and risk factors to identify women with suspected cancer in primary care: derivation and validation of an algorithmBr J Gen Pract201363606e11e2123336450