Abstract
Purpose
Home-time has been found to correlate well with modified Rankin Scale (mRS) scores in patients with stroke. This study aimed to determine its correlations in patients with different types of stroke at various time points after stroke in a non-Western population.
Methods
This study used linked data from multi-center stroke registry databases and a nationwide claims database of health insurance. Functional outcomes as measured with the modified Rankin Scale were obtained from the registry databases and home-time was derived from the claims database. Spearman correlation coefficients were used to assess the correlation between home-time and mRS scores. The area under the receiver operating characteristic curve (AUC) was used to evaluate the performance of home-time in predicting good functional outcome.
Results
This study included 7959 patients hospitalized for stroke or transient ischemic attack (TIA), for whom mRS scores were available in 6809, 6694, and 4330 patients at 90, 180, and 365 days, respectively. Home-time was highly correlated with mRS scores at the three time-points in patients with ischemic (Spearman’s rho −0.69 to −0.83) or hemorrhagic (Spearman’s rho −0.86 to −0.88) stroke, but the correlation was only weak to moderate in those with TIA (Spearman’s rho −0.32 to −0.58). Home-time predicted good functional outcome with excellent discrimination in patients with ischemic (AUCs >0.8) or hemorrhagic (AUCs >0.9) stroke but less so in those with TIA (AUCs >0.7).
Conclusion
Home-time was highly correlated with mRS scores and showed excellent discrimination in predicting good functional outcome in patients with ischemic or hemorrhagic stroke. Home-time could serve as a valid surrogate measure for functional outcome after stroke.
Keywords:
Introduction
Despite the decrease in age-standardized stroke mortality, the global burden of stroke, as measured in terms of the loss of disability-adjusted life years, keeps increasing.Citation1 In most countries, stroke is among the leading causes of acquired adult disability.Citation1 Consequently, functional recovery should be taken into account in the evaluation of stroke therapies. Although administrative claims data, derived from claims for healthcare services, are commonly used in epidemiological research and outcome studies of stroke patients,Citation2,Citation3 variables regarding post-stroke functional outcomes, such as the modified Rankin Scale (mRS) score, are often lacking.Citation4 Therefore, post-stroke mortality or readmission is used as the study endpoint in most claims-based outcome studies. However, such events are far from an ideal proxy for functional outcomes.
Post-stroke home-time, defined as the number of days alive and spent outside an acute care setting after the index acute stroke, is a highly valued patient-centered outcome.Citation5,Citation6 Recently, home-time has been found to correlate well with mRS scores in patients after ischemic stroke or other types of stroke.Citation7–Citation9 Besides, it can be easily retrieved from administrative claims databases and may potentially save the costs and efforts in obtaining mRS scores for large-scale registry-based studies. Nevertheless, the correlation between home-time and mRS scores has rarely been examined in non-Western countries.
A previous study found that home-time in stroke survivors varied across hospitals in different locations (urban/rural) and geographic regions under the Medicare system in the US.Citation10 In addition, home-time after stroke is largely determined by the availability of post-discharge care and support systems, which definitely vary in different healthcare systems and countries.Citation6 Therefore, the purpose of this study was to examine the correlation between home-time and mRS scores in Taiwanese stroke patients using data linkage between a population-based administrative claims database and hospital-based stroke registries.
Methods
Data Source and Linkage
Three data sources were linked for this study: the National Health Insurance Database (NHID), Cause of Death Registry and multi-center stroke registry databases. The NHID and Cause of Death Registry are provided by the Ministry of Health and Welfare and maintained by the Health and Welfare Data Science Center (HWDC) in Taipei City, Taiwan.Citation11 The NHID from 2001 to 2015 and the Cause of Death Registry were used in this study. The NHID is derived from the National Health Insurance program, which covers virtually all of Taiwan’s population. It includes registry for beneficiaries, ambulatory care claims, inpatient claims, and prescriptions dispensed at pharmacies. Each claim contains International Classification of Diseases, Ninth Edition, Clinical Modification (ICD-9-CM) diagnosis and procedure codes, expenditures per visit or admission, and details of drug prescriptions. The Cause of Death Registry contains a national identification number, date of birth, sex, date of death, and cause of death, which is recorded as ICD-9-CM codes up to the end of 2007 and as ICD-10-CM codes thereafter. For details of NHID and Cause of Death Registry, refer to Hsieh et al.Citation2
The Taiwan Stroke Registry (TSR), launched in August 2006, is the first nationwide stroke registry to collect real-world data on the quality of stroke care and provide evidence for healthcare policymaking.Citation4,Citation12 The ongoing TSR enrolls patients who are hospitalized for acute stroke or transient ischemic attack (TIA) within 10 days of symptom onset. More than 100,000 stroke events have been registered in the TSR up to 2015.Citation13 The functional status of each patient is measured using the mRS at 90 days, 180 days, and 365 days after stroke by in-person assessment or telephone interview. The multi-center stroke registry databases in this study were obtained from four TSR participating hospitals, including National Cheng Kung University Hospital, Chi Mei Medical Center, Ditmanson Medical Foundation Chiayi Christian Hospital, and Landseed Hospital.
The databases were linked by the national identification number in the HWDC to ensure security of personal information and the researchers could only access the linked databases in the HWDC. Because only aggregate data without any individual-level information could be released from the HWDC, informed consents from individual patients were not required. The research protocol was approved by the Institutional Review Board of National Cheng Kung University Hospital (IRB No. A-EX-106-053).
Study Population
Patients hospitalized for acute stroke between 2006 and 2010 were identified from the stroke registry databases (). After data linkage with the NHID, claims records of successfully linked patients were retrieved. Patients without data in the NHID were eliminated. Also excluded were those with a principal diagnosis other than ischemic stroke (ICD-9-CM 433 or 434), hemorrhagic stroke (ICD-9-CM 430, 431, or 432), and TIA (ICD-9-CM 435).
Variables
All the diagnosis codes (Supplemental Table 1) from the stroke hospitalization, as well as ambulatory and inpatient claims within the one-year look-back period prior to the stroke hospitalization were used to ascertain comorbidities.Citation14 The main outcome of interest was the follow-up mRS scores at 90 days, 180 days, and 365 days, which were obtained from the stroke registry databases. The mRS is a global measure of functional status. It is frequently used to assess functional outcome after stroke. The scale ranges from 0 to 6: 0 indicates no symptoms, 1–5 represent escalating degrees of disability and functional dependency, and 6 means death.Citation15 The mRS scores were dichotomized into good (mRS 0–2) and poor (mRS 3–6) outcomes.Citation16
The main independent variable was home-time evaluated at three time points, ie, 90 days, 180 days, and 365 days. Home-time was defined as the total number of days alive and out of acute care hospitals from the admission date of the index stroke hospitalization to the time point of evaluation or death, whichever came first ().
Statistical Analysis
Baseline characteristics were described with mean and standard deviations for continuous variables and counts and proportions for categorical variables. Stroke severity was assessed with the National Institutes of Health Stroke Scale (NIHSS). The distribution of home-time and mRS scores at the three time points was represented by box-and-whisker plots. The correlation of home-time with mRS scores was assessed by Spearman’s rank correlation analysis. Logistic regression analysis was used to model the association between home-time and good functional outcome. Model discrimination was assessed using the area under the receiver operating characteristic curve (AUC). The optimal cut-off value for home-time to predict good functional outcome was determined by the Youden index. In subgroup analyses, the study population was divided according to the types of stroke, ie, ischemic stroke, hemorrhagic stroke, and TIA and similar analysis procedures were repeated.
To further elaborate on the analogy between home-time and mRS scores, logistic regression analysis, using the baseline characteristics as independent variables, was performed to identify the predictors of home-time and mRS scores. In the analysis, home-time was dichotomized according to the optimal cut-off value mentioned above. All statistical analysis was performed using SAS version 9.4 (SAS Institute, Cary, NC).
Results
A total of 7959 patients with acute stroke or TIA were included in this study. Among them, 1150 (14.4%), 1265 (15.9%), and 3629 (45.6%) patients were lost to follow-up at 90, 180, and 365 days, respectively. Therefore, mRS scores at 90, 180, and 365 days were available for 6809, 6694 and 4330 patients, respectively. lists the baseline characteristics of the study population. The mean age was 71.8 years and 43.7% were female. Approximately three-fourths of patients were hospitalized for ischemic stroke. Supplemental Table 2 gives the comparisons of baseline characteristics between participants and those lost to follow-up. In general, patients lost to follow-up were younger, more likely to be male, and differed in the distribution of stroke types than participants. The prevalence of comorbidities and stroke severity were essentially similar between groups at 90 and 180 days. However, patients lost to follow-up at 365 days had milder stroke severity and less comorbidities including diabetes, ischemic heart disease, atrial fibrillation, heart failure, chronic obstructive pulmonary disease, and chronic kidney disease.
Table 1 Characteristics of the Study Population
and Supplemental Table 3 show the distribution of home-time across mRS scores at 90 days, 180 days, and 365 days, respectively, in patients with different types of stroke. Regardless of stroke types and time points of evaluation, home-time was inversely correlated with mRS scores (). In patients with ischemic stroke, home-time had a strong to very strong correlation with mRS scores with Spearman correlation coefficients ranging from −0.69 at 90 days to −0.83 at 365 days. In patients with hemorrhagic stroke, home-time had a very strong correlation with mRS scores (Spearman’s coefficients from −0.86 to −0.88). However, home-time was only weakly to moderately correlated with mRS scores in patients with TIA (Spearman’s coefficients from −0.32 to −0.58).
Figure 3 Box-and-whisker plots showing the distribution of home-times across mRS scores at 90, 180, and 365 days in all patients and subgroups of patients.
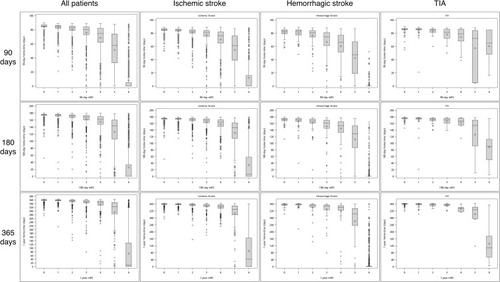
Table 2 The Correlation Coefficients Between Home-Time and the mRS Score by Spearman’s Rank Correlation Analysis
lists the AUCs and optimal cut-off values of home-time in predicting good functional outcome. Among stroke types, the AUCs were highest for hemorrhagic stroke with values of >0.9. Home-time also had excellent discrimination with AUCs >0.8 for ischemic stroke. Finally, home-time achieved an acceptable discrimination ability (AUCs >0.7) in predicting good functional outcome in patients with TIA. In all stroke patients, the optimal cut-off values of home-time to differentiate good from bad functional outcome were 79 days, 166 days, and 345 days at 90 days, 180 days, and 365 days, respectively. The optimal cut-off values of home-time in patients with hemorrhage stroke were lower than those in patients with ischemic stroke or TIA.
Table 3 Area Under Curve and Optimal Cut-off Values for Home-Time to Predict Good Functional Outcome
Supplemental Table 4 lists the odds ratios and confidence intervals of the baseline characteristics in predicting functional outcome at 90 days represented by home-time or mRS score in all study patients. Among the baseline characteristics, older age, a higher NIHSS score, diabetes, and chronic kidney disease were predictors of poor outcome when outcome was represented by either home-time or mRS, whereas hyperlipidemia protected against poor outcome.
Discussion
The main finding of this study was that home-time was highly associated with the degree of global disability as determined by the mRS. Home-time assessed at 90, 180, and 365 days showed excellent discrimination with AUCs >0.8 in predicting good functional outcome. In particular, home-time for patients with hemorrhage stroke exhibited outstanding discrimination with AUCs >0.9 in predicting good functional outcome at the three time points. These results were in line with previous studies in other countries,Citation7,Citation8 and suggested that home-time can serve as a surrogate measure of functional outcome after stroke for studies using Taiwan’s healthcare administrative databases.
As seen in a previous study,Citation8 patients with hemorrhagic stroke had lower home-time and patients with TIA had higher home-time than those with ischemic stroke. It probably reflected the different functional prognosis among stroke types in that hemorrhagic stroke is the most severe type of stroke followed by ischemic stroke and TIA. Of note, the AUCs of home-time also differed among stroke types, with the highest value in hemorrhagic stroke and the lowest in TIA (). Therefore, the application of home-time in future stroke research should take into account the variation in home-time across stroke types. We speculate that the ability of home-time to discriminate good from bad functional outcome after stroke may depend on the distribution of functional prognosis in each type of stroke. For example, because patients with TIA generally do not have functional disability after the event, home-time is more likely affected by factors other than functional disability. Therefore, home-time was less correlated with mRS scores and had a lower discriminatory ability in predicting good functional outcome in patients with TIA.
The mRS has been the preferred outcome measure for most of the acute stroke trials in the past decades.Citation17 Typically, mRS rating is assessed in an unstructured patient interview by clinicians.Citation18 Although the mRS ranges from 0 to 6, it is generally dichotomized in stroke trials based on the initial severity of stroke.Citation19 This study did not intend to propose a new outcome measure for stroke trials by demonstrating the close correlation between home-time and the mRS score. Instead, it aimed to establish a valid proxy for functional outcome in future claims-based stroke research, in particular in those based on the NHID. Home-time can be treated as a continuous outcome variable on the one hand; on the other, it can be dichotomized to represent either good (mRS 0–2) or poor outcome (mRS 3–6) based on the optimal cut-off values. Therefore, researchers can use home-time as a proxy to evaluate the effect of a specific treatment on functional outcome in claims-based stroke studies, rather than simply using mortality as an endpoint.Citation20
Moreover, in large-scale registry-based studies where long-term follow-up assessment of mRS is costly and laborious, or even infeasible, home-time can be treated as an alternative and reliable patient-centered measure to assess stroke outcome. Record linkage between clinical registry databases and administrative claims databases may be an effective strategy to complement the advantages of each type of databases.Citation4 The combination of clinical data from registry databases and long-term outcome data from claims databases, such as home-time, can provide opportunities to investigate early predictors of functional recovery following stroke, such as blood pressure variabilityCitation21 and C reactive protein.Citation22 The linked databases can also be used to validate various stroke outcome prediction scores.Citation23
In addition to clinical investigations, home-time may potentially be used in other applications such as quality measurement and policymaking in healthcare considering that administrative claims databases have been commonly used in these fields.Citation24–Citation26 For example, healthcare providers and consumers can compare the quality of stroke care across hospitals using home-time as a proxy indicator. Policymakers can also refine resource allocation decisions based on the population-level trend of home-time after stroke. Being a composite measure of staying alive and out of acute care hospitals, home-time may be a preferred outcome variable over readmission, which is theoretically affected by the competing risk of death. In the past, stroke research based on administrative claims data has been limited by the lack of adequate clinical information.Citation27 In the future, researchers may have a chance to mitigate this shortcoming when conducting stroke outcome studies using the NHID through the use of home-time as a functional endpoint, combined with the use of a claims-based proxy for stroke severity.Citation28,Citation29
This study has the following limitations. First, home-time was defined as the time spent outside the acute care setting. We were unable to differentiate whether patients lived in their own home or in a skilled nursing facility, nursing home, or other chronic care facility. Second, the sample size of TIA patients was relatively small. The robustness of the study results for TIA patients is open to question and needs further examination with more samples. Third, the study results may only apply to claims-based research using the NHID as the data source. Further validation is needed before they can be used in studies based on administrative claims databases from other countries.
Conclusions
This validation study showed that home-time was closely correlated with the mRS score and predicted good functional outcome with excellent discrimination at 90, 180, and 365 days after stroke. Because it can be readily and reliably assessed from claims data, home-time may serve as a valid proxy for functional outcome after stroke in studies using administrative claims databases.
Acknowledgments
This research was supported in part by the Ministry of Science and Technology, Taiwan (grant number MOST 107-2320-B-006-035). We are grateful to the Health Data Science Center, National Cheng Kung University Hospital for providing administrative and technical support. We would like to thank Ms. Li-Ying Sung for English language editing.
Disclosure
The authors declare they have no conflicts of interest with respect to this research study.
References
- Feigin VL, Norrving B, Mensah GA. Global burden of stroke. Circ Res. 2017;120(3):439–448. doi:10.1161/CIRCRESAHA.116.30841328154096
- Hsieh CY, Su CC, Shao SC, et al. Taiwan’s National Health Insurance Research database: past and future. Clin Epidemiol. 2019;11:349–358. doi:10.2147/CLEP.S19629331118821
- Ung D, Kim J, Thrift AG, et al. Promising use of big data to increase the efficiency and comprehensiveness of stroke outcomes research. Stroke. 2019;50(5):1302–1309. doi:10.1161/STROKEAHA.118.02037231009352
- Hsieh CY, Wu DP, Sung SF. Registry-based stroke research in Taiwan: past and future. Epidemiol Health. 2018;40:e2018004. doi:10.4178/epih.e201800429421864
- Hannah D, Lindholm B, Maisch L. Certain uncertainty: life after stroke from the patient’s perspective. Circ Cardiovasc Qual Outcomes. 2014;7(6):968–969. doi:10.1161/CIRCOUTCOMES.114.00131525387779
- Shah S, Xian Y, Olson DM, et al. Home-time is a patient-centered outcome variable for stroke: an executive summary. J Neurosci Nurs. 2019;51(3):110–112. doi:10.1097/JNN.000000000000043730964840
- Fonarow GC, Liang L, Thomas L, et al. Assessment of home-time after acute ischemic stroke in medicare beneficiaries. Stroke. 2016;47(3):836–842. doi:10.1161/STROKEAHA.115.01159926892279
- Yu AYX, Rogers E, Wang M, et al. Population-based study of home-time by stroke type and correlation with modified Rankin score. Neurology. 2017;89(19):1970–1976. doi:10.1212/WNL.000000000000463129021355
- McDermid I, Barber M, Dennis M, et al. Home-time is a feasible and valid stroke outcome measure in national datasets. Stroke. 2019;50(5):1282–1285. doi:10.1161/STROKEAHA.118.02391630896358
- O’Brien EC, Xian Y, Xu H, et al. Hospital variation in home-time after acute ischemic stroke: insights from the PROSPER study (patient-centered research into outcomes stroke patients prefer and effectiveness research). Stroke. 2016;47(10):2627–2633. doi:10.1161/STROKEAHA.116.01356327625383
- 中華民國衛生福利部. Health and welfare data science center, health and welfare data science center. 2017 Available from: https://dep.mohw.gov.tw/DOS/np-2497-113.html. Accessed 520, 2020.
- Hsieh FI, Lien LM, Chen ST, et al. Get with the guidelines-stroke performance indicators: surveillance of stroke care in the Taiwan stroke registry: get with the guidelines-stroke in Taiwan. Circulation. 2010;122(11):1116–1123. doi:10.1161/CIRCULATIONAHA.110.93652620805428
- Lai EC, Man KK, Chaiyakunapruk N, et al. Brief report: databases in the Asia-Pacific region: the potential for a distributed network approach. Epidemiology. 2015;26(6):815–820. doi:10.1097/EDE.000000000000032526133022
- Cheng CL, Kao YH, Lin SJ, Lee CH, Lai ML. Validation of the National Health Insurance Research Database with ischemic stroke cases in Taiwan. Pharmacoepidemiol Drug Saf. 2011;20(3):236–242. doi:10.1002/pds.208721351304
- van Swieten JC, Koudstaal PJ, Visser MC, Schouten HJ, van Gijn J. Interobserver agreement for the assessment of handicap in stroke patients. Stroke. 1988;19(5):604–607. doi:10.1161/01.STR.19.5.6043363593
- Wardlaw JM, Murray V, Berge E, Del Zoppo GJ. Thrombolysis for acute ischaemic stroke. Cochrane Database Syst Rev. 2014;7:Cd000213.
- Quinn TJ, Dawson J, Walters MR, Lees KR. Functional outcome measures in contemporary stroke trials. Int J Stroke. 2009;4(3):200–205. doi:10.1111/j.1747-4949.2009.00271.x19659822
- Taylor-Rowan M, Wilson A, Dawson J, Quinn TJ. Functional assessment for acute stroke trials: properties, analysis, and application. Front Neurol. 2018;9:191. doi:10.3389/fneur.2018.0019129632511
- Broderick JP, Adeoye O, Elm J. Evolution of the modified rankin scale and its use in future stroke trials. Stroke. 2017;48(7):2007–2012. doi:10.1161/STROKEAHA.117.01786628626052
- Hsieh CY, Huang HC, Wu DP, Li CY, Chiu MJ, Sung SF. Effect of rehabilitation intensity on mortality risk after stroke. Arch Phys Med Rehabil. 2018;99(6):1042–1048.e1046. doi:10.1016/j.apmr.2017.10.01129108967
- Divani AA, Liu X, Di Napoli M, et al. Blood pressure variability predicts poor in-hospital outcome in spontaneous intracerebral hemorrhage. Stroke. 2019;50(8):2023–2029. doi:10.1161/STROKEAHA.119.02551431216966
- Di Napoli M, Slevin M, Popa-Wagner A, Singh P, Lattanzi S, Divani AA. Monomeric C-reactive protein and cerebral hemorrhage: from bench to bedside. Front Immunol. 2018;9:1921. doi:10.3389/fimmu.2018.0192130254628
- Lattanzi S, Pulcini A, Corradetti T, et al. Prediction of outcome in embolic strokes of undetermined source. J Stroke Cerebrovasc Dis. 2020;29(1):104486. doi:10.1016/j.jstrokecerebrovasdis.2019.10448631706753
- Palmer WL, Bottle A, Davie C, Vincent CA, Aylin P. Meeting the ambition of measuring the quality of hospitals’ stroke care using routinely collected administrative data: a feasibility study. Int J Qual Health Care. 2013;25(4):429–436. doi:10.1093/intqhc/mzt03323584363
- Balinskaite V, Bottle A, Shaw LJ, Majeed A, Aylin P. Reorganisation of stroke care and impact on mortality in patients admitted during weekends: a national descriptive study based on administrative data. BMJ Qual Saf. 2018;27(8):611–618. doi:10.1136/bmjqs-2017-006681
- Hartley J, Li Y, Kunkel L, Crowcroft NS. The burden of infant group B streptococcal infections in Ontario: analysis of administrative data to estimate the potential benefits of new vaccines. Hum Vaccin Immunother. 2019;15(1):193–202. doi:10.1080/21645515.2018.151166630130440
- Yu AY, Holodinsky JK, Zerna C, et al. Use and utility of administrative health data for stroke research and surveillance. Stroke. 2016;47(7):1946–1952. doi:10.1161/STROKEAHA.116.01239027174527
- Sung SF, Hsieh CY, Kao Yang YH, et al. Developing a stroke severity index based on administrative data was feasible using data mining techniques. J Clin Epidemiol. 2015;68(11):1292–1300. doi:10.1016/j.jclinepi.2015.01.00925700940
- Hsieh CY, Lin HJ, Chen CH, Li CY, Chiu MJ, Sung SF. “Weekend effect” on stroke mortality revisited: application of a claims-based stroke severity index in a population-based cohort study. Medicine. 2016;95(25):e4046. doi:10.1097/MD.000000000000404627336904