Abstract
Background
This study evaluated the reliability and criterion validity of the Mywellness Key accelerometer (MWK) using treadmill protocols and indirect calorimetry.
Methods
Twenty-five participants completed two four-stage 20-minute treadmill protocols while wearing two MWK accelerometers. Reliability was assessed using raw counts. Validity was assessed by comparing the estimated VO2 calculated from the MWK with values from respiratory gas exchange.
Results
Good overall and point estimates of reliability were found for the MWK (all intraclass correlations > 0.93). Generalizability theory coefficients showed lower values for running speed (0.70) versus walking speed (all > 0.84), with the majority of the overall percentage of variability derived from the participant (68%–88% of the total 100%). Acceptable validity was found overall (Pearson’s r = 0.895–0.902, P < 0.0001), with an overall mean absolute error of 16.22% and a coefficient of variance of 16.92%. Bland-Altman plots showed an overestimation of energy expenditure during the running speed, but total kilocalories were underestimated during the protocol by approximately 10%.
Conclusion
Good validity was found during light and moderate walking, while running was slightly overestimated. The MWK may be useful for clinicians and researchers interested in promotion or assessment of physical activity.
Introduction
Promotion of physical activity is an important aspect of public health in the prevention of morbidity and mortality in the United States and other nations. Accelerometry is a useful assessment tool for measuring physical activity in individual and population-based studies, but only if the devices are valid and reliable. Even with the limitations for measuring whole body acceleration,Citation1 accelerometers are a practical way of assessing physical activity objectively in interventionsCitation2,Citation3 or in surveillance programs,Citation4 such as the US National Health and Nutrition Examination Survey.Citation5 Many validation studies have used various accelerometers in laboratory and field settings to assess activity levels in different populations.Citation6–Citation12
The Mywellness Key (MWK) has been introduced by Technogym SpA (Gambettola, Italy), and is a new consumer-based accelerometer. The device measures acceleration of movement using a uniaxial design and proprietary algorithms to determine the time and intensity when worn on clothing at a person’s waist. Two studies have investigated the MWK, and have shown strong concurrent validity with the ActiGraph during treadmill (r = 0.91, P < 0.01) and free-living (r = 0.73–0.76, P < 0.01) settings,Citation13 while a second study measured criterion validity with gas exchange (r = 0.94, P < 0.001; standard error of estimate 2.42 mL/kg per minute), although reliability was not assessed.Citation14 In addition, estimates and the variability of total energy expenditure against indirect calorimetry have not been evaluated for the MWK when assessing usability of the device. Therefore, this study evaluated the reliability and validity of the MWK during a range of exercise intensities to evaluate its utility as an objective physical activity monitor.
Materials and methods
Participants
Twenty-five participants were recruited from the University of South Carolina during 2011 using flyers posted at the campus. A convenience sample consisting of graduate students was used in this study. Inclusion criteria included both women and men with a body mass index of 18.5–29.9 kg/mCitation2, from any racial/ethnic group, who were physically able to walk briskly for 30 minutes and jog for at least 5 minutes. Height was limited to 148.6–185.4 cm, corresponding to the 2.5th and 97.5th percentile of height.Citation15 We excluded people with gait abnormalities (limps or injuries that impeded walking or running); current heart disease, stroke, type 2 diabetes, and peripheral vascular disease; current pregnancy; and those taking medications that might affect circulation or heart rate response, eg, beta-blockers and hypertensive medication.
Height was measured to the nearest cm using a stadiometer (Ayrton Corporation, Model S100, Prior Lake, MN). Weight was measured using an electronic scale (Home Health Care Digital Scale, Model MC-660, C-7300 v1.1) to the nearest 0.1 kg. Waist circumference was measured using a measurement tape 5 cm above the umbilicus to the nearest 0.5 cm. Body fat percentage was assessed using the generalized gender-specific three-site skinfold test from the American College of Sports Medicine’s guidelines for exercise testing and prescription.Citation16
Mywellness Key
The MWK is a small, lightweight (8.5 × 2.0 × 0.7 cmCitation3, 18.7 g), consumer-grade uniaxial accelerometer designed to measure vertical accelerations of the human body when in motion. This device was designed to integrate data from recreation center exercise machines and free-living activity, when worn at the waist, into a single energy expenditure value. The sampling frequency of the device is 16 Hz and measures acceleration from 0.06 to 12.0 g (1 g = 9.81 meters per secondCitation2) with a frequency response from 0.1 to 5 Hz.
Using an embedded algorithm, the MWK converts raw counts and outputs the physical activity level of an individual in real time during the course of the day to a liquid crystal display screen. The device uses a proprietary metric called “MOVE”, which is unitless, to measure the total volume of physical activity. It is similar to a metabolic equivalent (MET) minute. The device also records and displays the time in light (1.8–2.9 METs), moderate (3–5.9 METs), or vigorous activity ($6 METs). For this study, proprietary Technogym firmware and equations (firmware version 7190) were used to record counts directly from the device during the protocol in one-second epochs. This was converted into METs (conversion factor 3.5), VO2, and MOVE using Technogym algorithms.
Testing protocol
Each participant performed two identical treadmill tests. For the two tests, participants were randomly assigned two MWKs from a group of 10 supplied by the manufacturer. The first MWK was placed on a belt at the anterior of the right hip in line with the right knee. The second MWK was positioned directly above the first. The same MWKs were used at the same locations in both tests for each participant. The two tests were separated by at least 5 days.
The testing equipment consisted of a research-grade treadmill (Trackmaster, JAS, Model TMX42C, FullVision Inc, Newton, KS) and a Parvo gas analysis system (Parvo Medics, Sandy, UT). The treadmill protocol was computer-automated and consisted of a 2-minute warmup at 2.25 km/hour, and four 5-minute stages at 3.22 km/hour, 5.96 km/hour, 6.44 km/hour, and 7.24 km/hour. The incline was set at 0% grade for all tests. Participants were required to walk during the first three stages, and jog during the last stage of the protocol. MET intensities were derived from American College of Sports Medicine treadmill speed formulaeCitation16 and corresponded to 2.52, 3.82, 4.76, and 7.90 METs for stages 1 through 4. An informed consent and medical screening was completed before starting the study protocol. The study was reviewed and approved by the University of South Carolina Institutional Review Board.
Statistical analysis
Descriptive statistics (mean ± standard deviation) for age, height, weight, body mass index, waist circumference, and body fat percentages were calculated for all participants. Average counts and milliliters of oxygen consumption (VO2) were used from the last 2 minutes of each stage for validity and reliability comparisons.
Generalizability theory was used to partition and quantify sources of variance in MWK counts at each treadmill speed.Citation17 In contrast with classical test theory generalizability theory allows researchers to examine multiple sources of error and report reliability estimates systematically using a generalizability coefficient.Citation18 In this study a fully crossed design [participant (P) × test (T) × monitor (M)] was used to quantify the proportion of error. Intraclass correlation coefficientsCitation19 and coefficient of variance were used to assess the variation in reliability. In addition, repeated-measures analysis of variance were used for significance testing using Bonferroni adjustments.
Validity was assessed using mean absolute percent error and coefficient of variance between oxygen uptake measures and the MWK’s estimated oxygen uptake computed from counts. Mean absolute percent error was used to describe absolute variation in the point estimates. Pearson’s correlations were also used to test overall validityCitation20 Bland-Altman 95% limits of agreementCitation21,Citation22 were used to plot the bias of the results for individual and overall results, and to illustrate the spread of the data.
Comparisons of energy expenditure were tested using total computed kilocalories (kcals) from the 20-minute treadmill tests. The output from the Parvo for kcals was compared with a MWK-computed kcal value from the Technogym algorithm. Differences between the two devices were tested using a two-tailed Student’s t-test with an alpha level of P = 0.05. Accumulation of MET minutes from gas exchange and MOVE from the MWK were calculated from the average per minute values during the last 2 minutes of each stage, multiplied by the time in each stage (5 minutes), and combined. Statistical analyses were carried out using SAS version 9.2 (SAS Inc, Cary NC) and SPSS version 19.0 (IBM, Armonk, NY).
Results
General demographics are reported in . The population was relatively young (27.6 ± 4.5 years), and had low body fat (15.2% ± 7.8%). The study participants included men (n = 12) and women (n = 13), who were normal to overweight (mean body mass index 22.4 kg/mCitation2, range 19.3–27.8 kg/mCitation2).
Table 1 Descriptive characteristics of study participants
Reliability
Results from the generalizability theory analysis are shown in . The T term accounted for < 1 % of the total variability, indicating consistency in mean MWK counts between testing trials at each speed. The relatively low proportion of error from the monitor term at each treadmill speed suggests similar mean counts were obtained between monitor locations (top or bottom). The T × M interaction term contributed little toward the total variance, suggesting that the rank order of counts from each monitor location was similar between tests. The majority of the total variance in MWK counts was attributed to the mean differences between participants. The proportion of error from the participant term was relatively high across stages 1–3 (84%–88%) and noticeably lower during stage 4 (68%). Ideally, the participant term would account for the largest proportion of error, given that this is considered true error rather than “noise” from the instrumentation. The T × P interaction term represents the consistency in participant rank order between tests. Overall, slightly more variability in participant rank order was observed at the slowest treadmill speed. The M × P interaction term describes the variability in participant rank order between monitor locations. The amount of error attributable to the M × P interaction was considerably higher in stage 4 compared with stages 1–3 (19% versus 3%–4%, respectively). This finding emphasizes the need to standardize the monitor location during field and laboratory assessments. Overall, generalizability coefficients ranged from 0.84 to 0.89 for light and moderate walking, with a lower value found for the running stage (stage 4, 0.70).
Table 2 Generalizability theory reliability estimates for the Mywellness Key
Interunit reliability estimates are shown in . The analyses resulted in acceptable values for Pearson’s correlations for both tests (r = 0.97–0.98). When considering point estimates of reliability measures in each stage, correlations ranged from very good for stages 1–3 (walking, r = 0.95–0.96) to borderline (running, r = 0.79–0.84).
Figure 1 Reliability coefficients for the MWK.
Abbreviations: CI, confidence interval; ICC, intraclass correlation coefficient; MWK, Mywellness Key; CoV, coefficient of variance.
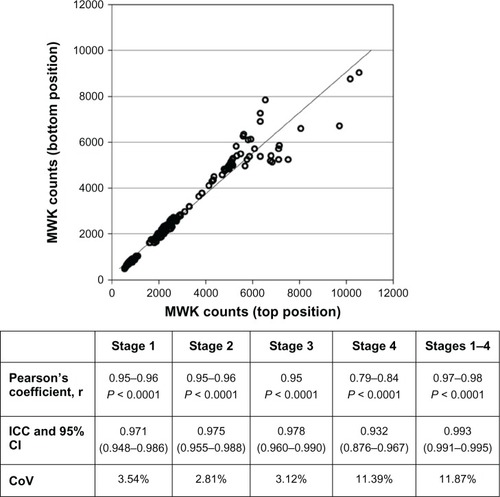
Intraclass correlation coefficients showed good values for reliability in each stage and when combined (minimum intraclass correlation coefficient of 0.93). The overall coefficient of variance was 11.87%, with the highest amount of variability related to stage 4 (coefficient of variance 11.39), while other stages were lower (2.81%–3.54%).
Validity
Validity was evaluated using Pearson’s correlations, mean absolute percent error, coefficient of variance, and Bland-Altman plots. Graphical representation and values for Pearson’s correlations are displayed in . The two tests resulted in a correlation of r = 0.895–0.902, which was greater than the criterion value of 0.85. Total mean absolute percent error (±standard deviation) for the MWK for all stages was 16.22% ± 13.03% and the coefficient of variance was 16.92% (), with higher values of mean absolute percent error found in the running stage (stage 4). shows Bland-Altman plots of all data. The overall mean and 95% limits of agreement was 1.8 mL (−5.6, 9.3) VO2/kg per minute. The MWK was found to overestimate VO2 by 4.2 mL during stage 4. The mean values for stages 1–3 were close to 0, ranging from −0.5, 1.5, to 2.1 mL, respectively. The limits of agreement were narrow for stage 1 and increased through stage 4.
Figure 2 Validity of the MWK against gas exchange.
Abbreviation: MWK, Mywellness Key.
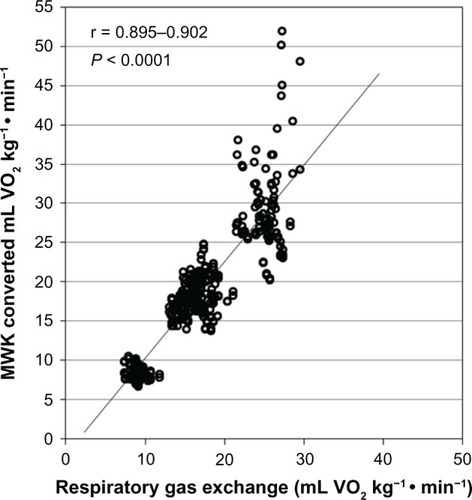
Figure 3 Bland-Altman plots of all data between converted MWK values and gas exchange (VO2, mL/kg per minute).
Abbreviation: MWK, Mywellness Key.
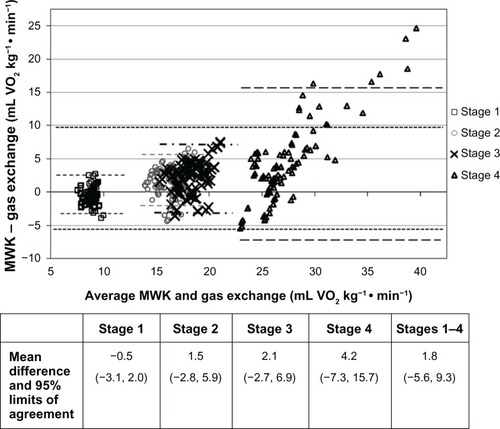
Table 3 Overall and point estimates of validity for the Mywellness Key
shows the comparisons between total energy expenditure (in kcals) derived from gas exchange and the MWK for the entire 20-minute exercise test protocol. Kcals between the two methods were significantly correlated (r = 0.78, P < 0.0001), with the MWK, underestimating energy expenditure by approximately 10.7 kcals or 10.4%, which was found to be significantly different (P < 0.001). Total MOVE was found to be correlated with gas exchange when each stage was used as separate data points (r = 0.88, P < 0.001). This implied a positive dose-effect accumulation of MOVE with intensity. Directly comparing MOVE with MET minutes showed an approximate ratio of 2.5 to 1, with a good correlation between the variables (r = 0.90).
Figure 4 Total energy expenditure from gas exchange (kilocalories and calculated MET minutes), MWK, and MOVE characteristic over a 20-minute treadmill test protocol (n = 100).
Abbreviations: MWK, Mywellness Key; MET, metabolic equivalents.
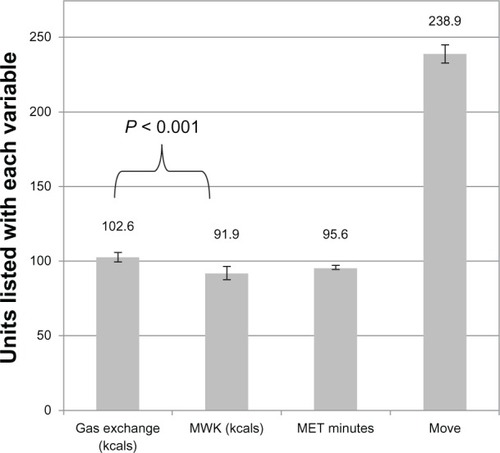
Discussion
In this analysis, we found high reliability (intraclass correlation coefficient 0.993) and good validity (Pearson’s r = 0.895–0.902) for the MWK when measuring group physical activity, although the device showed moderate amounts of interindividual variability (mean absolute percent error 16.22%, coefficient of variance 16.9%). Point estimates for the MWK slightly overestimated gas exchange by 1.8 mL VO2 on average, with the estimated VO2 at the running stage being the most variable. MWK values for energy expenditure underestimated total energy expenditure by about 10%. The error explained by generalizability theory was found to be derived mostly between participants and participant by monitor interactions, implying that variability between the devices is very low, and securing the MWK as critical in its application, especially at more vigorous speeds.
Overall, these results imply that the MWK could be useful in population-based physical activity studies for light and moderate activity, although individual results may show some variability, especially during vigorous activity. For reliability, we found that interindividual differences were more dependent on the placement of the device than other characteristics, such as weight or height, which did not contribute to excessive variability (data not shown). A suggested use for the MWK and other uniaxial accelerometers could be in physical activity programs that aim to increase minutes of light, moderate, and vigorous physical activity, with an emphasis on moderate activity. Using metrics from the real-time display may serve as a point of reference for individuals when trying to increase their minutes of a certain intensity or volume of activity as depicted by the MOVE metric. For estimating the amount of energy expenditure, users should be aware of the limitations of the MWK and other uniaxial accelerometers. Programming goals would be served by using the MOVE characteristic, which was found to correlate well with accumulation of physical activity across increasing levels of intensity. In our protocol, we found the ratio of MOVE to MET minutes to be about 2.5 to 1. Five hundred to 1000 MET minutes, which is used as the range associated with substantial health benefits for adults,Citation23,Citation24 would equal approximately 1250–2500 MOVE units throughout a week.
Variability may be improved by incorporating calibration procedures for each specific user that take into account factors such as body weight, gait, and gender. Even though such analyses could not be performed in this study because of the proprietary nature of the device, tailoring the parameters of future or existing physical activity monitors to key personal characteristics may reduce interpersonal error and enhance the accuracy of output metrics for the end user.
Comparison with other studies
For more than two decades, many studies have assessed the utility of physical activity monitors, particularly accelerom-eters, but to the authors’ knowledge, only two other studies have investigated the validity of the MWK.Citation13 Hermann et al showed a strong association between the MWK and the ActiGraph for treadmill walking (r = 0.91, P < 0.01), but did not elaborate on variability at each testing stage. We found a similar but slightly lower correlation with gas exchange measures than that in the study reported by Bergamin et al (r = 0.944, P < 0.001) which was conducted in an Italian population.Citation14 Similar measures were found between the MWK and other physical activity monitors. With the Caltrac accelerometer, the mean absolute percent error was found to be 23%, which was greater than the values with the MWK (mean absolute percent error 16.2%).Citation10 They also found increasing levels of variation as treadmill speed increased, which we also observed for the MWK. The authors stated that the Caltrac overestimated energy expenditure, and the variation was possibly attributed to an interaction with each participant’s fitness level. We found that the MWK also overestimated energy expenditure at similar intensities. In other studies, the ActiGraph and Kenz Lifecorder both significantly underestimated point estimates of energy expenditure at higher intensities when compared with indirect calorimetry (P < 0.001 at 188 m per minute),Citation6 although both devices were found to be accurate at slow to moderate walking speeds. Differences in the estimates could be attributed to the different algorithms used to express energy expenditure for the devices.
Reliability measures indicate that the MWK is similar to the ActiGraph, which has shown an intraclass correlation coefficient of 0.98 (95% confidence interval 0.97–0.98).Citation7 In the study with the ActiGraph, the device underestimated vigorous activity energy expenditure above 9 km/hour, which is in contrast with our study, in which the MWK overestimated energy expenditure. When compared with the Tritrac accelerometer, the MWK showed higher values for reliability (Tritrac, r = 0.87–0.92), although validity, measured by indirect calorimetry, was slightly higher on the Tritrac (r = 0.94, standard error of estimate 0.014 kcal/kg).Citation12 The greatest source of variation for the reliability of the MWK was similar to findings in a study reported by Welk et al, where variation for the Actical, Biotrainer, Tritrac, and CSA/MTI ActiGraph was attributed to interindividual differences rather than interdevice differences.Citation11 Low interdevice differences indicate that monitor to monitor variability is not normally a concern with research grade accelerometers, or as we found, with the MWK. Many of the differences between the devices may reflect differences in advancement of filtering or hardware between devices, the participants used, the test protocol, how the device was secured, or differences in the algorithms used. Design features for future models should consider three-dimensional outputs to minimize the error reflected by possible variability in positioning on the participant.
Strengths and limitations
In this study, the energy expenditure values only represent the average values for our participants and cannot easily account for interindividual differences in energy costs within the population with regard to mechanical efficiency or absolute work rates.Citation25 In fact, accelerometry data over a short time may not be representative of what a person usually does,Citation26 and does not take into account walking on an incline, carrying loads, performing strength training, or exclusively upper body motion. Fortunately, human movement for healthy individuals is made up mostly of ambulatory activities,Citation27 which is the principal activity measured by accelerometers. Our sample was limited to normal to overweight men and women. Assessment of individuals with higher body mass indexes or high body fat percentages may require additional validation due to increased variation in how the participant wears the device. The strengths of this study include the use of criterion gas exchange measures and generalizability theory to investigate multiple sources of variation, which have rarely been used in physical activity monitor studies.Citation11,Citation28 We suggest that validity and reliability studies should use generalizability theory to determine similar contributions to variation in other devices.
Conclusion
The MWK is an activity monitor that measures the amount of physical activity performed during free-living and structured exercise. This study examined the reliability and validity of the MWK during structured treadmill exercise and found it to be an adequate assessment device for population level measurements during slow or moderate locomotion speeds. For the MWK and other uniaxial accelerometers, minimizing the error for individuals’ exercise intensity is challenging. Future designs should take into consideration calibrations to reduce interindividual error and triaxial designs to reduce error arising from placement of the monitor. The MWK may be useful in physical activity programs where increasing minutes in categories of physical activity or when the volume of physical activity is the main outcome. The MWK seems to be a good device for measuring population-based physical activity in normal to overweight younger adults, and has value-added potential by the incorporation of real-time feedback capability for use as an aid in health promotion.
Acknowledgments
We would like to thank Gregory Welk of Iowa State University, and Stewart Trost of Oregon State University for their valuable contribution to the methodological content of this paper. We would also like to thank Patrick Crowley sincerely for the data collection portion of this study, Sara Wilcox for her valuable input, and Silvano Zanuso for technical support.
Disclosure
This study was funded by Technogym, SpA, manufacturer of the Mywellness Key. The authors have no other conficts of interest to declare.
References
- TroianoRPFreedsonPSPromises and pitfalls of emerging measures of physical activity and the environmentAm J Prev Med201038668268320494248
- MatthewsCEWilcoxSHanbyCLEvaluation of a 12-week home-based walking intervention for breast cancer survivorsSupport Care Cancer200715220321117001492
- PintoBMPapandonatosGDGoldsteinMGMarcusBHFarrellNHome-based physical activity intervention for colorectal cancer survivorsPsychooncology992011 [Epub ahead of print.]
- MarquezDXHoyemRFoggLBustamanteEEStaffilenoBWilburJPhysical activity of urban community-dwelling older Latino adultsJ Phys Act Health20118 Suppl 2S161S17021918229
- Tudor-LockeCBrashearMMJohnsonWDKatzmarzykPTAccelerometer profiles of physical activity and inactivity in normal weight, overweight, and obese US men and womenInt J Behav Nutr Phys Act201076020682057
- AbelMGHannonJCSellKLillieTConlinGAndersonDValidation of the Kenz Lifecorder EX and ActiGraph GT1M accelerometers for walking and running in adultsAppl Physiol Nutr Metab20083361155116419088773
- BrageSWedderkoppNFranksPWAndersenLBFrobergKReexamination of validity and reliability of the CSA monitor in walking and runningMed Sci Sports Exerc20033581447145412900703
- BrooksAGGunnSMWithersRTGoreCJPlummerJLPredicting walking METs and energy expenditure from speed or accelerometryMed Sci Sports Exerc20053771216122316015141
- NicholsJFMorganCGChabotLESallisJFCalfasKJAssessment of physical activity with the Computer Science and Applications Inc, accelerometer: laboratory versus field validationRes Q Exerc Sport2000711364310763519
- PambiancoGWingRRRobertsonRAccuracy and reliability of the Caltrac accelerometer for estimating energy expenditureMed Sci Sports Exerc19902268588622287266
- WelkGJSchabenJAMorrowJRJrReliability of accelerometry-based activity monitors: a generalizability studyMed Sci Sports Exerc20043691637164515354049
- NicholsJFMorganCGSarkinJASallisJFCalfasKJValidity, reliability, and calibration of the Tritrac accelerometer as a measure of physical activityMed Sci Sports Exerc199931690891210378921
- HerrmannSDHartTLLeeCDAinsworthBEEvaluation of the MyWellness Key accelerometerBr J Sports Med201145210911319736173
- BergaminMErmolaoASieverdesJCZaccariaMZanusoSValidation of the MyWellness Key in walking and running speedsJ Sports Sci Med2012115763
- TayyariFSmithJOccupational Ergonomics: Principles and Applications1st edNew York, NYChapman & Hall1997
- American College of Sports MedicineACSM’s Guidelines for Exercise Testing and Prescription8th edPhiladelphia, PALippincott Williams & Wilkins2010
- GleserGCCronbachLJRajaratnamNGeneralizability of scores infuenced by multiple sources of variancePsychometrika19653043954185217607
- BrennanRLGeneralizability TheoryNew York, NYSpringer2001
- AtkinsonGNevillAMStatistical methods for assessing measurement error (reliability) in variables relevant to sports medicineSports Med19982642172389820922
- DowdaMPateRRSallisJFAgreement between student-reported and proxy-reported physical activity questionnairesPediatr Exerc Sci200719331031818019589
- ChatburnRLEvaluation of instrument error and method agreementAANA J19966432612689095698
- BlandJMAltmanDGStatistical methods for assessing agreement between two methods of clinical measurementLancet1986184763073102868172
- US Department of Health and Human Services2008 Physical Activity Guidelines for Americans2008 Available from: http://www.health.gov/paguidelines/guidelines/default.aspxAccessed October 1, 2012
- US Department of Health and Human ServicesDepartment of Health and Human Services. Physical Activity Guidelines Advisory Committee Report2008 Available from: http://www.health.gov/PAGuidelines/Report/Accessed October 1, 2012
- AinsworthBEHaskellWLLeonASCompendium of physical activities: classification of energy costs of human physical activitiesMed Sci Sports Exerc199325171808292105
- GretebeckRJMontoyeHJVariability of some objective measures of physical activityMed Sci Sports Exerc19922410116711721435166
- US Bureau of Labor StatisticsBLS Spotlight on Statistics: Sports and ExerciseMay2008 Available from: http://www.bls.gov/spotlight/2008/sports/pdf/sports_bls_spotlight.pdfAccessed February 15, 2012
- WickelEEWelkGJApplying generalizability theory to estimate habitual activity levelsMed Sci Sports Exerc20104281528153420139788