Abstract
The proportion of older people in the world population is expected to increase rapidly during the upcoming decades. Consequently, the number of patients with multimorbidity will increase dramatically. In epidemiologic research, the concepts of multimorbidity, comorbidity, and complications have been confusing, and some of these concepts are used interchangeably. In this commentary, the authors propose a clear terminology for clinical concepts describing different aspects of multimorbidity and elucidate the relationship between these clinical concepts and their epidemiologic analogs. Depending on whether a study uses causal or predictive models, a proper distinction between concepts of multimorbidity is important. It can be very difficult to separate complications of the index disease under study from comorbidity. In this context, use of comorbidity indices as confounding scores should be done with caution. Other methodologic issues are type, duration, severity, and number of comorbidities included in the ascertainment methods, as well as sources included in the research. Studies that recognize these challenges have the potential to yield valid estimates of the comorbidity burden and results that can be compared with other studies.
Multimorbidity
The major challenge facing modern health care systems is aging of the population in the context of significant pressure to contain costs. The proportion of people aged 60 years or more in the world population is expected to increase rapidly from 10% in 2000 to 21% in 2050.Citation1 Concurrently, the number of patients with multimorbidity, ie, coexistence of several chronic diseases, will increase dramatically. The prevalence of multimorbidity has been estimated at more than 80% among persons aged older than 85 years.Citation2 Up until now, clinical research has focused predominantly on single disease and episode, often with a focus on mortality as the main endpoint. Thus, one of the most important tasks in clinical medicine today is managing multimorbidity. This requires an evolution away from the single disease focus that has dominated medicine for centuries.Citation3 The aim of this commentary is to propose clear terminology for the clinical concepts describing different aspects of multimorbidity and to elucidate the relationship between these clinical concepts and their epidemiologic analogs.
Confusion concerning terminology used in clinical epidemiology
The concept of multimorbidity varies widely in the literature.Citation4,Citation5 It has been used to describe the number of morbidities, the number and severity of morbidities, and the number and severity of morbidities together with concurrent limitations in functional status or frailty. In addition, multimorbidity is often measured by the burden of comorbidity at time of diagnosis of an index disease.Citation4 The numerous definitions of multimorbidity include predefined medical conditions or unlimited numbers and types of medical conditions, chronic conditions, or both acute and chronic conditions, physical diseases alone, or physical and psychiatric conditions. Further, the various definitions include comorbidities diagnosed before or both before and concurrent with the index disease.Citation6–Citation14
Because of the existing confusion concerning terminology, we propose more stringent definition of five commonly used concepts. We suggest that the “index disease” describes the main condition under study, while “comorbidity” describes medical conditions that exist at the time of diagnosis of the index disease or later, but that are not a consequence of the index disease. In contrast, “multimorbidity” can be described as existence of two or more chronic diseases. “Complications” of an index disease are adverse events occurring after diagnosis of that disease. “Case-mix” refers most often to the mix of patient types treated at hospitals or departments, and the case-mix index is a measure of the complexity of illness used in health service research or in clinical medicine as, for example, a clinical prediction score.
In clinical epidemiology, these concepts are used in two main types of models with the purposes of control for confounding (causal models) or clinical prediction.
Causal models
These concepts can be translated into epidemiologic analogs in causal models with a well-defined exposure and outcome.Citation15 In this context, the index disease defines the study population or the exposure under study. The term “comorbidity” can have three roles in epidemiologic studies, depending on the exposure and endpoint. First, in some circumstances, comorbidity can be a part of the exposure complex under study. An example is the impact of comorbidities on mortality in patients with diabetes. Second, comorbidity can interact with the exposure and modify the association between that exposure and an endpoint. Third, in many studies of a defined index disease, comorbidity qualifies as a potential confounding factor in the association between an exposure and an endpoint, given that the burden of comorbidity varies for different patient populations based on characteristics such as age and lifestyle.Citation16 It is important to emphasize that there are three criteria for a confounding factor: a confounder must be associated with the disease (either as a cause or as a proxy for a cause but not as an effect of the disease); a confounder must be associated with the exposure; and a confounder must not be an effect of the exposure.Citation15
In contrast, “complications” of the index disease can arise after diagnosis of that disease and therefore qualify as an endpoint or an intermediate step in the pathway from exposure to a more distal endpoint in the clinical pathway. For example, multiple sclerosis and sarcoidosis can be comorbid conditions in diabetics, while retinopathy, cardiomyopathy, and nephropathy are well defined complications of diabetes.Citation17 Other comorbidities may modify the effect between the index disease and survival. Thus, cancer may modify the effect between diabetes and survival ().
Figure 1 Simple epidemiological models illustrating the association between the exposure variable and the outcome under study.
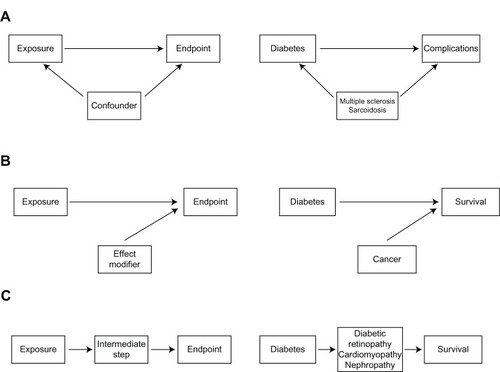
Risk prediction models
While causal models are used in the research setting to evaluate the causal role of one or more exposures while simultaneously controlling for possible confounding factors,Citation15 risk or prognosis prediction models may be useful tools in several clinical settings taking multiple clinical variables into consideration. The American Society for Anesthesiology score, for example, is used in acute medicine to evaluate the physical status of a patient and the impact of the index disease, comorbidity, and complications on mortality.Citation18 The Acute Physiology and Chronic Health Evaluation scale is used in intensive care to evaluate the burden of morbidity from the index disease, comorbidity, and acute clinical status.Citation19,Citation20
In health service management, the Diagnosis-Related Group system is used as a way to classify hospital cases into one of 467 original groups (now 745). This system of classification was developed by Fetter and Thompson.Citation21 Their intention was to identify the “products” that a hospital provides. Diagnosis-Related Groups are assigned by a “grouper” program based on International Classification of Diseases (ICD) diagnoses, procedures, age, gender, discharge status, and the presence of complications or comorbidities.Citation22
In practical clinical epidemiology, it might be difficult to distinguish complications from comorbidities. Such evaluation might most often require data information outside the actual study.Citation23 Evidence from particular experimental studies and theory, for example, must be considered.
Complications versus comorbidity in epidemiologic research
Failure to separate complications from comorbidities can have a serious impact on clinical epidemiology research. A very broad definition of comorbidity must be used with caution to avoid misclassifying complications as comorbidities. As shown in , complications are endpoints or intermediate steps in the pathway from an exposure to an endpoint. Therefore, they must be considered separately from comorbidities. Otherwise, the total comorbidity burden would be overestimated and misclassification of information about comorbidity would be introduced. If complications are regarded as comorbidities and handled as confounders, some of the effect between the exposure and outcome is masked, resulting in distorted estimates of association.Citation24 At the same time, a more restrictive definition of comorbidities could misclassify comorbidities as complications, and therefore result in underestimation of the comorbidity burden, potentially leading to residual confounding if comorbidity is a confounder in the study.
Correct classification of medical conditions as comorbidities or complications is necessary to avoid inaccurate estimation of the comorbidity burden. As described above, in examining the association between diabetes and survival, diseases such as multiple sclerosis or sarcoidosis are not known to be related to diabetes. Therefore, these diseases should be clearly defined as comorbidities in patients with diabetes as an index disease. Other diseases and conditions may not clearly meet the criteria of either comorbidities or complications of diabetes. Hypertension may be a common complication of diabetes as a result of vascular changes, but may also arise independently. This illustrates the complexity of separating medical conditions into comorbidities and complications, but also stresses its importance. Directed acyclic graphs may help clarify the role of different variables in a study.Citation24
Comorbidity scores and indices
Comorbidity scores or indices combine information about several comorbidities into one score. The idea behind a confounder summarization, for example, is to define a single continuous variable that pulls together relevant information on the confounding properties of all variables.Citation25 Several indices have been developed to account for comorbidity as a confounding factor in research studies. Frequently used indices include the Charlson Comorbidity Index, the Cumulative Illness Rating Scale, the Index of Co-existing Disease, and the Kaplan–Feinstein Index.Citation7,Citation9,Citation12–Citation14 These indices are based on information about severity or number and severity of comorbid conditions, defined by organ systems and severity of diverse aspects of each comorbid disease, or on the degree of pathologic changes of the comorbid condition defined by organ systems. These indices incorporate available information about comorbid conditions into an aggregate index, which precludes estimation of effects of individual comorbid diseases. In addition, the definition of a comorbid condition and its role in the index varies for different indices.
The Charlson Comorbidity Index is frequently used in clinical epidemiology studies to quantify the level of comorbidity. This index is based on 19 comorbid diseases weighted according to adjusted one-year cumulative mortality risk,Citation7 and has been validated as a prognostic marker of comorbidity for several index diseases.Citation26–Citation32 However, the Charlson Comorbidity Index has several limitations. It does not include psychiatric diseases, which can confer substantial morbidity, even in patients with physical index diseases. The Charlson Comorbidity Index also evaluates disease severity only for a few diseases and to a very limited extent. Diabetes and cancer, for example, are categorized into only two severity groups, although the prognostic impact of disease severity can be more finely parsed. The prognostic impact of disease duration varies for different diseases. For instance, it increases with duration for diabetes, but may decrease for successfully treated ulcer disease and cancer.
Limitations of confounding indices
The burden of comorbidity is measured by extracting data from medical records or medical databases, physical examination, personal interview, or questionnaires.Citation33 These methods have many weaknesses and there is no gold standard. First, the sensitivity and specificity of comorbid diagnoses, whether they come from medical files, databases, or patient report, are never complete. Therefore, there will be residual confounding in a study where comorbidity is a confounding factor. Due to variation in sensitivity and specificity for different comorbid diagnoses and potential failure to account for disease severity and duration, which may be highly correlated with an exposure and endpoint, comorbidity indices cannot accurately measure the comorbidity burden for each patient, thus leading to residual confounding. Any underestimation of the comorbidity burden, for example, by using restrictive definitions of comorbidity, may also introduce residual confounding into a research study. In view of these limitations, all confounding score indices must be used with caution.Citation14
Conclusion
Research on multimorbidity is urgently needed to understand the clinical course of disease in detail in order to improve clinical outcomes. Depending on whether a study uses causal or prediction models, a proper distinction between concepts of multimorbidity is important. It can be very difficult to separate complications of the index disease under study from comorbidity. In this context, use of comorbidity indices as confounding scores should be undertaken with caution. Other methodologic issues are type, duration, severity, and number of comorbidities included in the ascertainment methods, as well as sources included in the research. Studies that recognize these challenges have the potential to yield valid estimates of the comorbidity burden and results that can be compared with those from other studies.
Acknowledgment
This commentary was supported by the Danish Agency for Science, Technology and Innovation (10-084581).
Disclosure
The authors declare no conflicts of interest in this work.
References
- United Nations, Department of Economic and Social Affairs. Population DivisionWorld Population Ageing 1950–2050New York, NYUnited Nations2002
- SaliveMEMultimorbidity in older adultsEpidemiol Rev1312013 [Epub ahead of print.]
- SalisburyCMultimorbidity: redesigning health care for people who use itLancet201238098367922579042
- ValderasJMStarfieldBSibbaldBSalisburyCRolandMDefining comorbidity: implications for understanding health and health servicesAnn Fam Med20097435736319597174
- MarengoniAAnglemanSMelisRAging with multimorbidity: a systematic review of the literatureAgeing Res Rev201110443043921402176
- ElixhauserASteinerCHarrisDRCoffeyRMComorbidity measures for use with administrative dataMed Care19983618279431328
- CharlsonMEPompeiPAlesKLMacKenzieCRA new method of classifying prognostic comorbidity in longitudinal studies: development and validationJ Chronic Dis19874053733833558716
- FeinsteinARThe pre-therapeutic classification of co-morbidity in chronic diseaseJ Chronic Dis1970237455468
- KaplanMHFeinsteinARThe importance of classifying initial comorbidity in evaluation the outcome of diabetes mellitusJ Chronic Dis1974277–83874044436428
- ParkersonGRJrBroadheadWETseCKThe Duke Severity of Illness Checklist (DUSOI) for measurement of severity and comorbidityJ Clin Epidemiol19934643793938483003
- VerbruggeLMLepkowskiJMImanakaYComorbidity and its impact on disabilityMilbank Q1989673–44504842534562
- LinnBSLinnMWGurelLCumulative illness rating scaleJ Am Geriatr Soc19681656226265646906
- GreenfieldSApoloneGMcNeilBJClearyPDThe importance of co-existent disease in the occurrence of postoperative complications and one-year recovery in patients undergoing total hip replacement. Comorbidity and outcomes after hip replacementMed Care19933121411548433577
- de GrootVBeckermanHLankhorstGJBouterLMHow to measure comorbidity: a critical review of available methodsJ Clin Epidemiol200356322122912725876
- RothmanKJEpidemiology: An Introduction2nd edOxford, UKOxford University Press2012
- SchaferIHansenHSchonGThe influence of age, gender and socio-economic status on multimorbidity patterns in primary care. First results from the Multicare Cohort StudyBMC Health Serv Res2012128922471952
- NathanDMLong-term complications of diabetes mellitusN Engl J Med199332823167616858487827
- OwensWDFeltsJASpitznagelELJrASA physical status classifications: a study of consistency of ratingsAnesthesiology1978494239243697077
- KnausWADraperEAWagnerDPZimmermanJEAPACHE II: a severity of disease classification systemCrit Care Med198513108188293928249
- KnausWWagnerDDraperEAPACHE III study design: analytic plan for evaluation of severity and outcome in intensive care unit patients. Development of APACHECrit Care Med19891712 Pt 2S181S1852512055
- FetterRBShinYFreemanJLAverillRFThompsonJDCase mix definition by diagnosis-related groupsMed Care198018Suppl 2iii1537188781
- GoldfieldNThe evolution of diagnosis-related groups (DRGs): from its beginnings in case-mix and resource use theory, to its implementation for payment and now for its current utilization for quality within and outside the hospitalQual Manag Health Care201019131620042929
- RothmanKJGreenlandSLashTValidity in epidemiologic studiesModern Epidemiology3rd edPhiladelphia, PAWolter Kluwer2008128147
- RothmanKJGreenlandSLashTCausal diagramsModern Epidemiology3rd edPhiladelphia, PAWolter Kluwer2008
- MiettinenOSStratification by a multivariate confounder scoreAm J Epidemiol19761046609620998608
- BaldwinLMKlabundeCNGreenPBarlowWWrightGIn search of the perfect comorbidity measure for use with administrative claims data: does it exist?Med Care200644874575316862036
- PosesRMMcClishDKSmithWRBekesCScottWEPrediction of survival of critically ill patients by admission comorbidityJ Clin Epidemiol19964977437478691223
- GoldsteinLBSamsaGPMatcharDBHornerRDCharlson Index comorbidity adjustment for ischemic stroke outcome studiesStroke20043581941194515232123
- LeeDSDonovanLAustinPCComparison of coding of heart failure and comorbidities in administrative and clinical data for use in outcomes researchMed Care200543218218815655432
- MyersRPQuanHHubbardJNShaheenAAKaplanGGPredicting in-hospital mortality in patients with cirrhosis: results differ across risk adjustment methodsHepatology200949256857719085957
- QuachSHennessyDAFarisPFongAQuanHDoigCA comparison between the APACHE II and Charlson Index Score for predicting hospital mortality in critically ill patientsBMC Health Serv Res2009912919643010
- HemmelgarnBRMannsBJQuanHGhaliWAAdapting the Charlson Comorbidity Index for use in patients with ESRDAm J Kidney Dis200342112513212830464
- LashTLMorVWielandDFerrucciLSatarianoWSillimanRAMethodology, design, and analytic techniques to address measurement of comorbid diseaseJ Gerontol A Biol Sci Med Sci200762328128517389725