Abstract
Background
Cervical cancer, one of the leading causes of female deaths, remains a top cause of mortality in gynecologic oncology and tends to affect younger individuals. However, the pathogenesis of cervical cancer is still far from clear. Given the high incidence and mortality of cervical cancer, uncovering the causes and pathogenesis as well as identifying novel biomarkers are of great significance and are desperately needed.
Materials and methods
First, raw data were downloaded from the Gene Expression Omnibus database. The Robuse Multi-Array Average algorithm and combat function of the sva package were subsequently applied to preprocess and remove batch effects. Differentially expressed genes (DEGs) analyzed with the limma package were followed by gene ontology and pathway analysis, and a protein–protein interaction (PPI) network based on the STRING website and the Cytoscape software was constructed. Weighted Correlation Network Analysis (WGCNA) was utilized to build the coexpression network. Subsequently, UALCAN websites were employed to conduct survival analysis. Finally, the oncomine database was used to validate the expression of ANLN in other datasets.
Results
GSE29570 and GSE89657, including 49 cervical cancer tissues and 20 normal cervical tissues, were screened as the datasets. Three-hundred-twenty-four DEGs were identified and, among them, 123 were upregulated, while 201 were downregulated. The DEGs PPI network complex, contained 305 nodes and 4,962 edges, and 8 clusters were calculated according to k-core =2. Among them, cluster 1, which had 65 nodes and 1,780 edges, had the highest score in these clusters. In coexpression analysis, there were 86 hubgenes from the Brown modules that were chosen for further analysis. Sixty-one key genes were identified as the intersecting genes of the Brown module of WGCNA and DEGs. In survival analysis, only ANLN was a prognostic factor, and the survival was significantly better in the low-expression ANLN group.
Conclusion
Our study suggested that ANLN may be a potential tumor oncogene and could serve as a biomarker for predicting the prognosis of cervical cancer patients.
Introduction
Cervical cancer, one of the leading causes of female deaths, has an incidence of 7.4 per 100,000 women per year.Citation1 Despite the wide use of the Thinprep Cytologic Test and cervical cancer vaccine, cervical cancer remains a top cause of mortality in gynecologic oncology. There are ~250,000 women living with cervical cancer in America. Although there are many studies on the mechanism of cervical cancer, the pathogenesis of cervical cancer is still far from clear. Many factors are involved in the tumorigenesis and development of cervical cancer, including human papilloma virus infection,Citation2 oncogene activation, and antioncogene inactivation. Given the high incidence and mortality of cervical cancer, uncovering the causes and pathogenesis and identifying novel biomarkers are of great significance and are desperately needed.
Gene sequencing has been widely used with the rapid development of genomics, and numerous data have been stored in public databases, such as The Cancer Genome Atlas,Citation3 Gene Expression Omnibus (GEO) database, and ArrayExpress. Data can be freely accessed from these open platforms, and an integrated analysis of these data may pro- vide crucial clues for better understanding the mechanism of diseases, especially of cancers. Many bioinformatic methods were used to analyze the data. Among them, analysis of differentially expressed genes (DEGs) focuses on the upregulation and downregulation of genes between samples, while coexpression network analysis can be used to find modules of highly correlated genes.Citation4 As the genome is a complicated network and genes interact with each other in various ways, there is a tremendous need to analyze data from different perspectives.
In this study, raw data for GSE29570 and GSE89657 were obtained from GEO (available online: https://www.ncbi.nlm.nih.gov/geo),Citation5,Citation6,Citation7 from which there were a total of 49 cervical cancer cases and 20 normal cervical cases data available. DEGs were analyzed using the limma package with standard data processing and Gene Ontology (GO) term enrichment analysis, and Kyoto Encyclopedia of Genes and Genomes (KEGG) pathway analysis was developed using the clusterprofiler package.Citation8,Citation9 The protein–protein interaction (PPI) network was then executed on the STRING websiteCitation10 and Cytoscape software.Citation11 Subsequently, Weighted Correlation Network Analysis (WGCNA) was performed with the WGCNA package.Citation12 Finally, the Kaplan–Meier estimator was performed on the UALCAN website.Citation13,Citation14 An integrated analysis of cervical cancer, both on DEGs and WGCNA, will provide deep insight into the mechanism of cervical cancer.
Materials and methods
Microarray data information
GEO is a public functional genomics data repository containing array- and sequence-based data, from which the gene expression profiles of GSE29570 and GSE89657 of cervical cancer and normal cervical tissue were obtained. These two series, including 49 cervical cancer tissues and 20 normal cervical tissues, were based on GPL6244 Platforms (Affymetrix Human Gene 1.0 ST Array; Affymetrix, Santa Clara, CA, USA). These two candidates were chosen for integrated analysis because the two datasets have the same platform and are crucial for combining data from different datasets.
The raw data for these two datasets were integrated for the analysis, and the Robust Multiarray Average was used to preprocess CEL files.Citation15 The combat function in the sva package was applied to remove the batch effects of these two datasets.Citation16 DEGs were identified using the limma package with the Empirical Bayes method,Citation8 and statistically significant DEGs were defined as p<0.05 and |logFC| ≥1.453. Coexpression network analysis was performed with WGCNA to reveal the correlation of genes and to search for significantly correlated gene modules. The soft thresholding power was set as 6; then, analyses of module–trait relationships, gene significance (GS), and module membership (MM) were used. Statistically significant modules were defined as p<0.05.
For validation, the GSE52903 and GSE7410 data were analyzed by GEO2R, which is an interactive web tool used to identify DEGs.Citation17 The data for Pyeon cervical cancer were obtained from oncomine,Citation18 which included 20 cancer cases and 8 normal cases.
GO term and KEGG pathway enrichment analyses
To better explore the biological significance of DEGs, enrichment of the functions and pathways was analyzed using clusterprofiler, a package with an analysis and visualization function to provide valuable information on the GO and KEGG analyses.Citation9 A p-value <0.05 was considered a significant enrichment.
Integration of the PPI network
The online database STRING was applied to construct a PPI network. The Cytoscape software was then employed to analyze the interactive relationship of the candidate proteins. The Molecular Complex Detection (MCODE), a plug-in used to score and find parameters that have been optimized to produce the best results for the network, was subsequently utilized to find clusters in the network.Citation19
Survival analysis
The key genes were identified as the intersecting genes of the Brown module of WGCNA and DEGs. The genes were then analyzed on UALCAN, a portal for facilitating tumor subgroup gene expression and survival analyses.Citation14 Cervical cancer samples were divided into two groups: 1) high ANLN expression (with TPM values above upper quartile) and 2) low/medium ANLN expression (with TPM values below upper quartile). The survival curves of samples with high gene expression and low/medium gene expression were compared by the log rank test.
p<0.05 was considered statistically significant.
Results
Identification of DEGs in cervical cancer and the enrichment of these genes
The DEGs of GSE29570 and GSE89657 were analyzed using the limma package following preprocessing and removing batch effects. Using p<0.05 and |logFC| ≥1.453 as the cutoff criteria, a total of 324 DEGs were identified, including 123 upregulated genes and 201 downregulated genes in cervical cancer tissues compared to normal cervical tissues (). The DEGs are shown in the volcano map, and the top 100 DEGs according to the value of |logFC| are also visualized on a heatmap (). The clusterprofiler package was used to compare gene clusters by their enriched biological processes, with a cutoff of p<0.05. In GO analysis, the upregulated genes that were significantly enriched included microtubule motor activity, ATPase activity, and microtubule binding. The downregulated genes were significantly enriched in sulfur compound binding, glycosaminoglycan binding, and heparin binding (). In the KEGG analysis, the upregulated genes were mainly enriched in the cell cycle, oocyte meiosis, and the p53 signaling pathway. The downregulated genes were mainly enriched in melanoma, breast cancer, and renin secretion (). The significantly enriched terms and pathways may help us further investigate the role of DEGs that play a role in cervical cancer.
Figure 1 DEGs identified in GSE29570 and GSE89657.
Notes: (A) Volcano map of differently expressed genes between cervical cancer tissues and normal cervical tissues. (B) Heatmap of the top 100 DEGs according to the value of |logFC|. (C) The top 20 GO terms in the enrichment analysis of the upregulated and downregulated genes. (D) The top 20 KEGG pathways in the enrichment analysis of upregulated and downregulated genes.
Abbreviations: DEG, differentially expressed genes; GO, Gene Ontology; HTLV-I, human T-cell leukemia virus type 1; KEGG, Kyoto Encyclopedia of Genes and Genomes ; Dw, down; NoDiff, no significant difference.
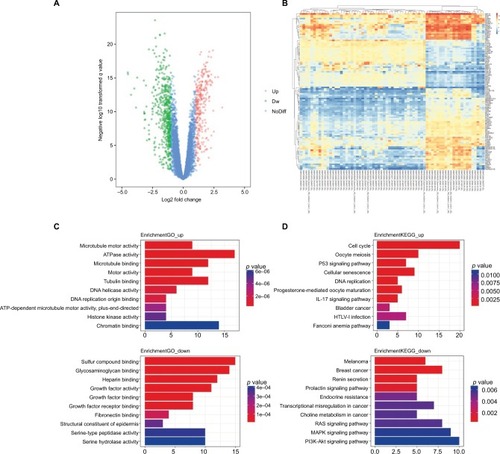
PPI network and cluster analysis
Using the STRING website, 305 DEGs were filtered into the DEGs PPI network complex, which contained 305 nodes and 4,962 edges (). The Cytoscape soft- ware was employed to analyze the interactive relationship of the candidate proteins. Afterward, the MCODE, a plug- in using scoring and finding parameters that have been optimized to produce the best results for the network, was utilized to find clusters in the network. Eight clusters were calculated according to k-core =2. Among them, cluster 1, which had 65 nodes and 1,780 edges, had the highest score in these clusters (). In this cluster, CEP55/ TYMS/ KIF15/TTK/ PTTG1/ CDKN3/ MKI67/ KIF23/ CCNB1/CDK1/KIFC1/KPNA2/CDC6/MCM4/MCM2/ SHCBP1/FOXM1/NUF2/RRM2/ANLN were the top 20 genes according to the mcode_score. This finding may indicate that the above mentioned genes play a critical role in cervical cancer.
Figure 2 Cluster analysis of the PPI network.
Notes: (A) Three-hundred-five DEGs were filtered into the DEGs PPI network complex that contained 305 nodes and 4,962 edges. (B) Cluster 1 consists of 65 nodes and 1,780 edges and has the highest score in those clusters.
Abbreviations: DEG, differentially expressed genes; PPI, protein–protein interaction.
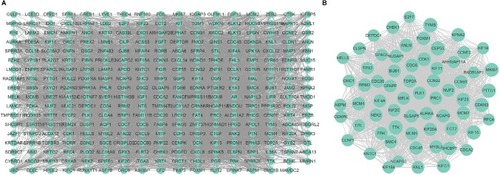
Coexpression analysis
The data underwent preprocessing and, after removing the batch effect, were analyzed by WGCNA to find the modules of highly correlated genes. After setting the power as 6, 13 modules were excavated (). Among the modules, module Brown and module Turquoise were the most relevant modules with cancer traits (), and 1,000 genes were selected at random for the heatmap (). Additionally, an intramodular analysis of GS and MM of the genes in the 13 modules was followed. As GS and MM exhibit a very significant correlation, this finding implies that the genes in the Brown module tend to be highly correlated with cancer (). The genes in this module were then selected for hubgenes with a cutoff of correlation ≥0.9. In total, there were 86 hubgenes from Brown modules that were chosen for further analysis.
Figure 3 WGCNA of GSE29570 and GSE89657.
Notes: (A) Six-thousand-sixty genes were assigned to one of 13 modules, including Module gray, with a cutoff of powers =6. The top image shows a gene dendrogram, and the bottom image shows the gene modules with different colors. (B) Correlation between modules and traits. The upper number in each cell refers to the correlation coefficient of each module in the trait, and the lower number is the corresponding p-value. Among them, the Brown and Turquoise modules were the most relevant modules with cancer traits. (C) A heatmap of 1,000 genes was selected at random. The intensity of the red color indicates the strength of the correlation between pairs of modules on a linear scale. (D) A scatter plot of GS for cervical cancer versus the MM in the Brown module. Intramodular analysis of the genes found in the Brown module, which contains genes that have a high correlation with cervical cancer, with p<1e-200 and correlation =0.92.
Abbreviations: GS, gene significance; MM, module membership; WGCNA, Weighted gene coexpression network analysis.
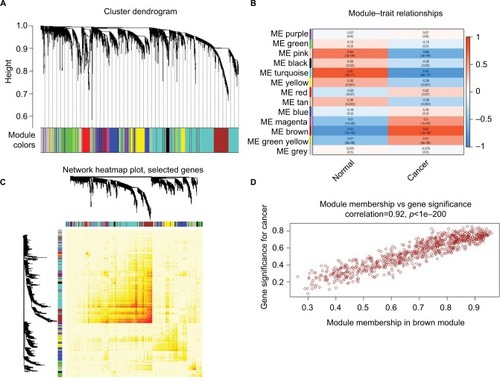
Key genes identified both from hubgenes and DEGs
To better extract valuable clues from these data, key genes were discovered both from hubgenes in the Brown module and the DEGs. In total, 61 key genes were identified (). Further survival analyses on these key genes were employed to evaluate their effects on the survival of cervical cancer. Briefly, only one gene, ANLN, was clearly related to the prognosis of patients (). Patients whose tissues displayed a higher expression of ANLN had significantly shorter overall survival compared to those with lower expression. Three other datasets were utilized to validate the expression of this gene. The results showed that ANLN expression was significantly higher in cervical cancer tissues compared to that of normal tissues (p<0.05) (). Together with the bioinformatics analyses, the PPI network based on ANLN was analyzed. MKI67 and FOXM1 have a close relationship with ANLN, a link that is also crucial in cervical cancer ().
Figure 4 ANLN is a biomarker and prognostic factor in cervical cancer.
Notes: (A) A Venn diagram of the DEGs and hubgenes in the Brown module revealed 61 key genes. (B) Survival analysis indicated that ANLN is a poor prognosis factor in cervical cancer, while patients with a higher expression of ANLN have significantly shorter overall survival compared to those with lower expression (p=0.013). (C–E) Validation of ANLN expression both from the oncomine database (C) and GEO databases (D, E). Three datasets showed higher expression of ANLN in cervical cancer tissues compared with normal cervical tissues (p<0.05). (F) PPI network of ANLN based on the STRING website. Many genes involved in the progression of cervical cancer have interactions with ANLN, including MKI67 and FOXM1. ***p<0.001.
Abbreviations: DEG, differentially expressed genes; GEO, Gene Expression Omnibus; PPI, protein–protein interaction.
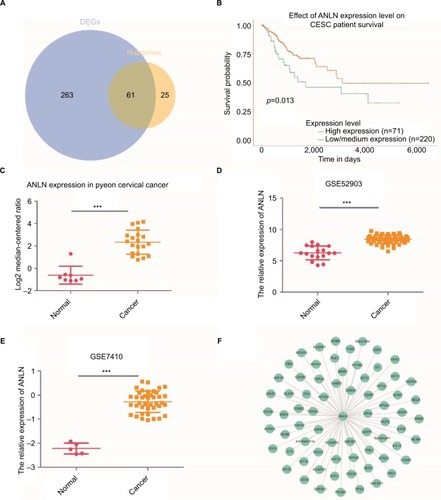
Discussion
In this study, we collected two series from GEO datasets and performed an integrated analysis from both DEG and WGCNA to deeply study the data to try to find valuable clues. A total of 324 DEGs were identified, including 123 upregulated genes and 201 downregulated genes. Enrichment analyses of GO and KEGG were subsequently performed to further analyze the function of these genes. The PPI network was constructed on the DEGs utilized on the STRING website and Cytoscape software. In this step, 8 clusters were found for the cutoff of a k-core of 2. Cluster 1, which had the highest score, contained 65 nodes and 1,780 edges in this subnetwork. In WGCNA, genes were clustered into 13 modules, and among them modules Brown and Turquoise were the most relevant modules to cancer traits. As the genes in the Brown module had the highest correlation with cancer traits, the genes in this module were further selected for hubgenes with a cutoff of correlation ≥0.9. Key genes were identified from both the hubgenes selected in the Brown module and DEGs. Briefly, 61 key genes were excavated and chosen for survival analysis. Interestingly, ANLN was clearly related to the prognosis of patients, with a p-value of 0.013.
ANLN is a protein-coding gene and has higher expression levels in the brain, placenta, and testis, but lower expression levels in the heart, kidney, liver, lung, pancreas, prostate, and spleen.Citation20 Many studies have shown that ANLN is overexpressed in many carcinomas, including breast cancer, colorectal cancer, hepatic cancer, and pancreatic cancer.Citation21–Citation26 Interestingly, overexpression of ANLN is a poor prognosis factor for colorectal cancer, gastric cancer, and breast cancer, and inhibiting the expression of ANLN may deregulate cell growth and migration in breast cancer, this indicated ANLN may be used as the target for the precision treatment of cervical cancer. However, in renal cell carcinoma, cytoplasmic anillin expression is a marker of favorable prognosis.Citation27 Additionally, in upper urinary tract urothelial carcinoma, overexpression of ANLN in the nucleus is a poor prognosis factor, while low expression of ANLN in the cytoplasm is a poor prognosis maker.Citation28 In non-small-cell lung cancer, ANLN was reduced only by carbon beam irradiation. This suggests that ANLN may act as a potential marker for evaluating the effect of radiotherapy in patients with lung cancer.Citation29 As radiation therapy has been established as a standard treatment for cervical cancer and has improved survival of the patients, ANLN may serve as a potential marker for evaluating the effect of radiotherapy in patients with cervical cancer.
Conclusion
ANLN acts as a substrate for the anaphase-promoting complex/cyclosome. ANLN may control the spatial contractility of myosin and play a crucial role during cytokinesis.Citation30 Several studies have shown that ANLN activates RHOA through the PI3K/AKT pathway in lung cancer as a responsive gene of Wnt/β-catenin in gastric cancer.Citation31,Citation32 ANLN forms complexes with p190RhoGAP-A and may regulate the RhoA-GTP levels in the cytokinetic furrow to ensure the progression of cell division.Citation33 In this study, according to the PPI network, many genes involved in the progression of cervical cancer have interactions with ANLN, including MKI67 and FOXM1. Several studies have snow that MKI67, also known as Ki-67, has a strong relationship with tumor grade, disease-free survival, and overall survival in cervical cancer, and MKI67 has been used as a prognostic factor in clinical diagnosis and treatment.Citation34 The protein encoded by FOXM1 is a transcriptional activator in cell proliferation and regulates the expression of cell cycle genes, such as CCNB1 and CCND1.Citation35 FOXM1 has been reported to promote cell invasion and is highly correlated with a poor prognosis in early-stage cervical cancer.Citation36 These findings suggest that ANLN may be a potential tumor oncogene and may serve as a biomarker for predicting the prognosis of cervical cancer patients. Many studies have been carried out to investigate the pathogenesis of cervical cancer over the past few years, and little is known about the formation and progression of cervical cancer. Cervical cancer still remains the top cause of death in gynecologic oncology, especially in younger patients. Most of these bioinformatics studies are focused on single data and utilized a single method to analyze DEGs. In this study, raw data were collected from different series to increase the sample size. Numerous tools were applied to deeply reanalyze the data to provide diverse perspectives from different angles. After integrating the results of the DEGs and WGCNA, survival analysis was performed to screen the key candidate genes to reveal valuable ideas. Here, for the first time, we found that ANLN was upregulated in cervical cancer tissues. In addition, expression of ANLN is a maker of poor prognosis in patients with cervical cancer, which may be a therapeutic target for precision medicine in cervical cancer. Further studies are still needed to explore the biological functions and understand the underlying molecular mechanism by which ANLN plays a role in cervical cancer.
Acknowledgments
This work was supported by National Key Research and Development Program of China (grant no. 2016YFC1303100).
Disclosure
The authors report no conflicts of interest in this work.
References
- FerlayJSoerjomataramIDikshitRCancer incidence and mortality worldwide: sources, methods and major patterns in GLO- BOCAN 2012Int J Cancer20151365E359E38625220842
- BrintonLAHerreroRReevesWCde BrittonRCGaitanETenorioFRisk factors for cervical cancer by histologyGynecol Oncol19935133013068112636
- Cancer Genome Atlas Research NetworkWeinsteinJNCollissonEAThe Cancer Genome Atlas Pan-Cancer analysis projectNat Genet201345101113112024071849
- JeongHMasonSPBarabásiALOltvaiZNLethality and centrality in protein networksNature20014116833414211333967
- EdgarRDomrachevMLashAEGene Expression Omnibus: NCBI gene expression and hybridization array data repositoryNucleic Acids Res20023012071011752295
- Guardado-EstradaMMedina-MartínezIJuárez-TorresEThe Amerindian mtDNA haplogroup B2 enhances the risk of HPV for cervical cancer: de-regulation of mitochondrial genes may be involvedJ Hum Genet201257426927622357541
- Marrero-RodríguezDLa CruzHATaniguchi-PoncianoKKrüppel like factors family expression in cervical cancer cellsArch Med Res201748431432229157672
- RitchieMEPhipsonBWuDlimma powers differential expression analyses for RNA-sequencing and microarray studiesNucleic Acids Res2015437e4725605792
- YuGWangLGHanYHeQYclusterProfiler: an R package for comparing biological themes among gene clustersOMICS201216528428722455463
- SzklarczykDMorrisJHCookHThe STRING database in 2017: quality-controlled protein-protein association networks, made broadly accessibleNucleic Acids Res201745D3626827924014
- SuGMorrisJHDemchakBBaderGDBiological network exploration with Cytoscape 3Curr Protoc Bioinformatics2014478.13.18.13.2425199793
- LangfelderPHorvathSWGCNA: an R package for weighted correlation network analysisBMC Bioinformatics2008955919114008
- ChandrashekarDSBashelBBalasubramanyaSAHUALCAN: A Portal for Facilitating Tumor Subgroup Gene Expression and Survival AnalysesNeoplasia201719864965828732212
- ChandrashekarDSBashelBBalasubramanyaSAHUALCAN: a portal for facilitating tumor subgroup gene expression and survival analysesNeoplasia201719864965828732212
- IrizarryRAHobbsBCollinFExploration, normalization, and summaries of high density oligonucleotide array probe level dataBiostatistics20034224926412925520
- LeekJTJohnsonWEParkerHSJaffeAEThe sva package for removing batch effects and other unwanted variation in high-throughput experimentsBioinformatics201228688288322257669
- CloughEBarrettTThe gene expression omnibus databaseMethods Mol Biol201614189311027008011
- RhodesDRYuJShankerKONCOMINE: a cancer microarray database and integrated data-mining platformNeoplasia2004611615068665
- BaderGDHogueCWAn automated method for finding molecular complexes in large protein interaction networksBMC Bioinformatics20034212525261
- OegemaKSavoianMSMitchisonTJFieldCMFunctional analysis of a human homologue of the Drosophila actin binding protein anillin suggests a role in cytokinesisJ Cell Biol2000150353955210931866
- ZhouWWangZShenNKnockdown of ANLN by lentivirus inhibits cell growth and migration in human breast cancerMol Cell Biochem20153981–2111925223638
- O’LearyPCPennySADolanRTSystematic antibody generation and validation via tissue microarray technology leading to identification of a novel protein prognostic panel in breast cancerBMC Cancer20131317523547718
- MagnussonKGremelGRydénLANLN is a prognostic biomarker independent of Ki-67 and essential for cell cycle progression in primary breast cancerBMC Cancer201616190427863473
- WangGShenWCuiLChenWHuXFuJOverexpression of Anillin (ANLN) is correlated with colorectal cancer progression and poor prognosisCancer Biomark201616345946527062703
- KimHKimKYuSJDevelopment of biomarkers for screening hepatocellular carcinoma using global data mining and multiple reaction monitoringPLoS One201385e6346823717429
- OlakowskiMTyszkiewiczTJarzabMNBL1 and anillin (ANLN) genes over-expression in pancreatic carcinomaFolia Histochem Cytobiol200947224925519995712
- RonkainenHHirvikoskiPKauppilaSVaaralaMHAnillin expression is a marker of favourable prognosis in patients with renal cell carcinomaOncol Rep201125112913321109967
- LiangPIChenWTLiCFSubcellular localisation of anillin is associated with different survival outcomes in upper urinary tract urothelial carcinomaJ Clin Pathol201568121026103226135313
- AkinoYTeshimaTKiharaACarbon-ion beam irradiation effectively suppresses migration and invasion of human non-small-cell lung cancer cellsInt J Radiat Oncol Biol Phys200975247548119735871
- ZhaoWMFangGAnillin is a substrate of anaphase-promoting complex/cyclosome (APC/C) that controls spatial contractility of myosin during late cytokinesisJ Biol Chem200528039335163352416040610
- SuzukiCDaigoYIshikawaNANLN plays a critical role in human lung carcinogenesis through the activation of RHOA and by involvement in the phosphoinositide 3-kinase/AKT pathwayCancer Res20056524113141132516357138
- PandiNSManimuthuMHarunipriyaPMurugesanMAshaGVRajendranSIn silico analysis of expression pattern of a Wnt/β-catenin responsive gene ANLN in gastric cancerGene20145451232924809965
- ManukyanALudwigKSanchez-ManchinellySParsonsSJStukenbergPTA complex of p190RhoGAP-A and anillin modulates RhoA-GTP and the cytokinetic furrow in human cellsJ Cell Sci20151281506025359885
- AncuţaEAncuţaCCozmaLGTumor biomarkers in cervical cancer: focus on Ki-67 proliferation factor and E-cadherin expressionRom J Morphol Embryol200950341341819690767
- XueLChiangLHeBZhaoYYWinotoAFoxM1, a forkhead transcription factor is a master cell cycle regulator for mouse mature T cells but not double positive thymocytesPLoS One201052e922920169079
- HeSYShenHWXuLFOXM1 promotes tumor cell invasion and correlates with poor prognosis in early-stage cervical cancerGynecol Oncol2012127360161022943878