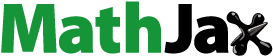
Abstract
Purpose
Clear cell renal cell carcinoma (ccRCC) is one of the most common cancers with high mortality worldwide. However, biomarkers for predicting prognosis in ccRCC are limited. In this study, we attempted to identify potential prognostic biomarkers of ccRCC.
Methods
Clinical information and the preprocessed ccRCC mature miRNA expression profiles in The Cancer Genome Atlas database were downloaded from UCSC Xena. The miRNAs differentially expressed between ccRCCs and matched normal tissues were analyzed using the “limma” package. A miRNA-based signature was constructed using the multivariate Cox regression model with prognosis index (PI) formula. Patients with ccRCC were divided into low-risk and high-risk subgroups according to median PI. The survival times were compared between the two groups using Kaplan–Meier analysis with log-rank test. The training set was used to construct a miRNA-based signature for predicting prognosis. The test set was used to verify the signature. Target gene prediction and functional enrichment analysis of the four miRNAs were performed using miRNet.
Results
We identified four miRNAs, miRNA-21-5p, miRNA-9-5p, miR-149-5p, and miRNA-30b-5p, as independent prognostic indicators. Next, we used these four miRNAs to construct a four-miRNA PI for each patient. Results revealed that patients in the high-risk group (n=119) had significantly shorter survival time than those in the low-risk group (n=118) (high-risk/low-risk group log-rank P=0.000). This four-miRNA signature is an independent prognostic factor compared with routine clinicopathological features in the test set. These miRNAs targeted 1,634 genes, and a miRNA-target gene network was constructed using miRNet. The target genes of these four miRNAs were involved in various pathways related to cancer.
Conclusion
Our observations suggest that the four-miRNA signature correlated with the survival of patients with ccRCC and can be used as a prognostic biomarker of ccRCC.
Introduction
Renal cell carcinoma (RCC) is a common malignant tumor of the urinary system, accounting for 2%–3% of adult malignancies,Citation1 and more than 100,000 people die of kidney cancer every year worldwide.Citation2 The most common subtype of RCC is clear cell renal cell carcinoma (ccRCC), which is associated with high morbidity and poor prognosis.Citation3
miRNAs were first identified by Lee et alCitation4 in Caenorhabditis elegans in 1993 as 19- to 24-nucleotide-long ncRNAs. It is estimated that miRNAs regulate the expression of >60% protein-coding genes. miRNAs are involved in various biological processes, such as cell growth, proliferation, differentiation, and apoptosis.Citation5 Owing to the tissue-specific expression of miRNAs, their expression profile has been associated with various diseases. Currently, abnormally expressed miRNAs have been detected in many human tumors, such as bladder cancer,Citation6 lung cancer,Citation7 prostate cancer,Citation8 pancreatic cancer,Citation9 gastric cancer,Citation10 liver cancer,Citation11 and other malignancies. Several recent studies have suggested that miRNA expression profiling can be used to predict the clinical outcome of patients with malignant tumors.Citation12,Citation13 Specific miRNAs have been used as potential diagnostic tools to distinguish the four subtypes of RCC (clear cell RCC, papillary RCC, chromophobe RCC, and benign oncocytomas).Citation14 However, studies on the association of miRNAs with ccRCC prognosis are limited. Currently, The Cancer Genome Atlas (TCGA) database (https://cancergenome.nih.gov/) can be used to analyze complicated clinical characteristics and cancer genomics. In this study, we screened the differentially expressed mature miRNAs between ccRCC tissues and matched normal tissues, and determined the association between these miRNAs and overall survival (OS). We constructed a four-miRNA signature that may be used as a potential prognostic biomarker of ccRCC.
Materials and methods
Data processing
The preprocessed ccRCC mature miRNA expression profiles in TCGA database, displayed as log2 converted reads per million (log2 (RPM + 1)), and clinical information, were downloaded from the UCSC Xena (https://xenabrowser.net/datapages/, version 09-08-2017). It contains miRNA expression data from two different platforms, including 311 samples (241 ccRCC tissues and 70 matched normal kidney tissues) based on the IlluminaHiSeq_miRNASeq platform (Illumina Inc., San Diego, CA, USA) and 259 ccRCC tissues based on the IlluminaGA_miRNASeq platform. The samples based on the IlluminaHiSeq_miRNASeq platform were used as the training set to identify differentially expressed miRNAs and to construct a miRNA-based signature for predicting prognosis. The samples based on IlluminaGA_miRNASeq platform were used as the test set to verify the signature. The mature miRNA sequencing data were processed using R language.
Screening of differentially expressed miRNAs
In the training set, miRNAs that were not expressed in >10% samples were removed. The differentially expressed miRNAs between ccRCCs and matched normal tissues were analyzed using the “limma” packageCitation15 in R. The fold changes (FCs) in the expression of individual miRNAs were calculated, and differentially expressed miRNAs with |log2FC|>1.0 and P<0.05 were considered to be significant. We applied bidirectional hierarchical clustering to the differentially expressed miR-NAs based on Euclidean distance and displayed the results as a heat map.
Construction and validation of the miRNA-based prognostic signature for ccRCC
In the training set, the patients were separated into high- and low-level groups based on the median value of the differential expression of miRNAs, followed by univariate and multivariate Cox proportional hazards analyses. Finally, a miRNA signature-based prognosis index (PI) score was constructed on the basis of a linear combination of the expression level multiplied by a regression coefficient derived from the multivariate Cox regression model (β) using the following formula.
The “β” value is the estimated regression coefficient of miRNAs and is derived from the multivariate Cox regression analysis, and “M” indicates the expression profiles of the miRNAs. Patients with ccRCC were divided into low- and high-risk groups based on median PI. The survival times were compared between the two groups using Kaplan– Meier analysis with log-rank test at P-value<0.05. The test set was used to confirm the robustness and transferability of the miRNA-based prognostic signature. We conducted the univariate and multivariate Cox proportional hazards analyses in the training and test sets to compare the relative prognostic value of this four-miRNA signature with that of routine clinicopathological features.
Target gene prediction and functional enrichment analysis
Target gene prediction and functional enrichment analysis of the four miRNAs were performed using miRNet (http://www.mirnet.ca/).Citation16 miRNet is an easy-to-use web-based tool that offers statistical, visual, and network-based approaches to assist researchers understand miRNA function and regulatory mechanisms and construct a miRNA-target gene network. The Kyoto Encyclopedia of Genes and Genomes (KEGG) pathway enrichment analysis was subsequently performed for the target genes. P-value <0.05 was set as the cutoff criteria.
Statistical analysis
The chi-squared test was used for categorical data, and the unpaired Student’s t-test was used to screen differentially expressed miRNAs. Univariate/multivariate Cox proportional hazards analyses and Kaplan–Meier survival analysis were used to compare the two groups of patients. The chi-squared test and survival analysis were performed using IBM SPSS statistics software program version 22.0 (IBM, Armonk, NY, USA). All tests were two-sided, and P<0.05 was considered statistically significant.
Results
Differential expression of miRNAs between ccRCC and matched normal kidney tissues
The detailed clinical characteristics of patients with ccRCC, including gender, age at diagnosis, histological grade, and TNM stage, are shown in . The training set contained more patients with metastasis than the test set (16.88% vs 14.67%, chi-squared test, P=0.000). This also partially assisted us to test the prognostic value of this miRNA-based signature in different patients. According to the cutoff criteria (P<0.05 and |log2FC|>1), 138 miRNAs were differentially expressed between ccRCC and matched normal kidney tissues in the training set. These included 54 upregulated miRNAs and 84 downregulated miRNAs in ccRCC tissues. miRNA-21-5p was upregulated, whereas miRNA-9-5p, miR-149-5p, and miRNA-30b-5p were downregulated in ccRCC tissues. The results of the expression analysis are presented as a heat map (), and the results of hierarchical clustering showed that the expression patterns of these differentially expressed miRNAs can correctly distinguish ccRCC from normal kidney tissues.
Table 1 Summary of patient cohort information
Construction of miRNA-based signature with differentially expressed miRNAs
Four samples were removed because of lack of survival record in the training set. For each of the 138 differentially expressed miRNAs, we used the median expression level as a cutoff to stratify the remaining 237 patients into high-level and a low-level groups. The univariate Cox proportional hazards regression analysis revealed that eight miRNAs possessed prognostic value (). Next, we performed a multivariate Cox proportional hazards regression analysis and identified four miRNAs, namely, miRNA-21-5p (), miRNA-9-5p (), miR-149-5p (), and miRNA-30b-5p (), as independent prognostic indicators. Thus, we used these four miRNAs to construct a four-miRNA PI as follows:
Table 2 Univariate and multivariate analyses in ccRCC patients
Figure 2 Four miRNAs were associated with overall survival in ccRCC patients using Kaplan–Meier curves and log-rank tests.
Note: (A) miRNA-21-5p; (B) miRNA-9-5p; (C) miRNA-149-5p; (D) miRNA-30b-5p.
Abbreviation: ccRCC, clear cell renal cell carcinoma.
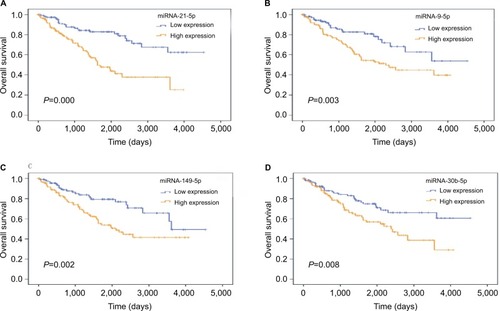
A PI was calculated for each patient in the training set. Then, 237 patients were separated into low- and high-risk groups according to median PI. Survival analysis was performed using the Kaplan–Meier method with log-rank test. Results revealed that patients in the high-risk group (n=119) had significantly shorter survival time than those in the low-risk group (n=118) (high-risk/low-risk group log-rank P=0.000; ). This four-miRNA signature is an independent prognostic factor compared with routine clinicopathological features ().
Table 3 Univariate and multivariate analyses of routine clinicopathological features and four-miRNA prognostic signature PI in the training set
Verification of the four-miRNA signature in the test set
Similar to that observed in the training set, patients in the test set were divided into low- and high-risk groups according to median PI, and Kaplan–Meier analysis was used to compare the patient’s OS. The results of survival analysis revealed that patients in the high-risk group (n=130) had significantly shorter survival time than those in the low-risk group (n=129) (high-risk/low-risk group log-rank P=0.000; ). The four-miRNA signature is an independent prognostic factor compared with routine clinicopathological features in the test set (). Overall, these results are consistent with that in the training set.
Table 4 Univariate and multivariate analyses of routine clinicopathological features and four-miRNA prognostic signature PI in the test set
Target gene prediction and functional enrichment analysis
To investigate the potential biological functions of these four miRNAs, we predicted their target genes using miRNet. We observed that 1,634 genes were targeted by these four miRNAs and a miRNA-target gene network was constructed using miRNet (). KEGG pathway enrichment analyses of the target genes revealed that they were involved in various pathways related to cancer, such as the MAPK, p53, and Wnt signaling pathways, cell cycle, and RNA transport ().
Figure 4 The miRNA-target genes network constructed using the miRNet and the corresponding target genes involved in KEGG Pathways.
Note: (A) The total network of four miRNAs and their targets; (B) pathways in cancer, (C) p53 signaling pathway; (D) cell cycle pathway; (E) renal cell carcinoma; (F) Wnt signaling pathway.
Abbreviation: KEGG, Kyoto Encyclopedia of Genes and Genomes.
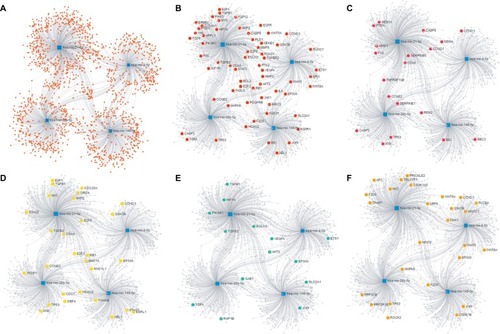
Discussion
ccRCC is one of the most common renal malignancies associated with high mortality and morbidity.Citation17 However, clinical tools for predicting patient outcome utilize traditional clinical parameters. Therefore, accurate identification of predictive factors from data obtained from analysis of ccRCC specimens is clinically challenging. Identification and validation of novel biomarkers form an important part of practical studies on ccRCC. During tumorigenesis, miRNAs act as oncogenes or tumor suppressors; hence, the biological behavior of tumors can be inhibited by regulating miRNA levels for therapeutic purposes. Identification of RNA profiles and selective targets is the basis for individualized treatment of different tumors. Previous studies have shown that some specific miRNAs were aberrantly expressed in RCC and participate in its development.Citation18–Citation21 However, detailed analyses of the associations between miRNA expression and prognosis of patients with ccRCC remain limited.
In this study, we identified 138 miRNAs that were differentially expressed between ccRCC and normal kidney tissues. The univariate Cox proportional hazards regression analysis revealed that eight miRNAs possessed prognostic value. We confirmed that the four-miRNA (miRNA-21-5p, miRNA-9-5p, miRNA-149-5p, and miRNA-30b-5p) signature can be regarded as an independent predictor of prognostic OS after considering the various variables, including gender, age, histology, and stages. Previous studies have also identified these four miRNAs, and several studies have investigated the relationship between miRNA expression patterns and cancer. Kowalczyk et alCitation22 reported that special AT-rich sequence binding protein 1 (SATB1) may be a potential prognostic marker for ccRCC, as low SATB1 expression in ccRCC may result from overexpression of miR-21-5p. SATB1 downregulation and miR-21-5p upregulation were associated with shorter patient survival. At present, the role of miRNA-9-5p in tumors has not been clarified. Certain studies show that downregulation of miR-9-5p expression can reverse the effect of miRNA-9-5p on proliferation, colony formation, cell cycle arrest, and apoptosis in osteosarcoma cells.Citation23 Okato et alCitation24 demonstrated that dual strands of pre-miR-149 (miRNA-149-5p and miRNA-149-3p) acted as antitumor miRNAs by targeting FOXM1, which was shown to be associated with survival of patients with ccRCC. Liu et alCitation25 suggested that miR-30b-5p acts as a novel tumor suppressor to regulate RCC cell proliferation, metastasis, and epithelial to mesenchymal transition by downregulating GNA13 expression. In other words, miR-30b-5p may be considered a potential biomarker for RCC diagnosis. However, studies demonstrating that the four differentially expressed miRNAs were predictors of ccRCC were lacking.
In this study, we constructed a four-miRNA signature, and the PI of this signature was calculated for each patient, which successfully separated patients into low- and high-risk groups. Specifically, patients considered high-risk by our four-miRNA signature had significantly poor prognosis than those in the low-risk group (P<0.001). We confirmed that the four-miRNA signature is an independent predictor of OS in patients with ccRCC. Similar to that observed in the training set, patients in the test set were divided into low- and high-risk groups based on the risk score of individual patients, and Kaplan–Meier analysis was used to compare patient survival differences. Statistically significant differences (P<0.0001) were observed between high- and low-risk groups. This confirmed that our four-miRNA signature is an independent and universal predictor of ccRCC.
It is well known that miRNAs modulate gene expression. Hence, we screened the target genes of these four miRNAs and used bioinformatics to predict the pathways and biological functions associated with their targets. The target genes were significantly enriched in multiple cancer-associated pathways, such as MAPK, p53, and Wnt signaling pathways. Abnormal regulation of these signaling pathways is involved in the development of various human cancers, such as breast cancer, hepatocellular carcinoma, hematological cancer, and lung cancer.Citation26–Citation28 Thus, abnormal regulation of signaling pathways may play a crucial role in the pathogenesis and progression of ccRCC. Further molecular investigations may provide new therapeutic targets for ccRCC.
Limitations
However, our study has few limitations. First, the ccRCC tissues were more than normal kidney tissues. Second, the miRNA expression profiles in the test set were not based on the same platform. Therefore, this four-miRNA signature has to be verified in a larger independent cohort of patients.
Conclusion
A comprehensive analysis of differentially expressed miRNA profiles and corresponding clinical information suggested that a four-miRNA signature was an independent and universal prognostic factor in patients with ccRCC. These miRNAs modulated genes associated with multiple cancer-associated pathways. However, further studies are required to verify our observations and establish the molecular mechanism underlying the interplay of miRNAs, their target genes, and ccRCC progression.
Acknowledgments
This study is funded by the Key Planning Development Research Program of Guangxi (grant no. guikeAB16380215) and the Guangxi Medical Health Appropriate Technology Development application project (no. 201634).
Author contributions
All authors contributed toward data analysis, drafting, and critically revising the paper, gave final approval of the version to be published, and agree to be accountable for all aspects of the work.
Disclosure
The authors report no conflicts of interest in this work.
References
- BrugarolasJMolecular genetics of clear-cell renal cell carcinomaJ Clin Oncol201432181968197624821879
- GuoXZhangQThe emerging role of histone demethylases in renal cell carcinomaJ Kidney Cancer VHL20174215
- GremelGDjureinovicDNiinivirtaMA systematic search strategy identifies cubilin as independent prognostic marker for renal cell carcinomaBMC Cancer2017171928052770
- LeeRCFeinbaumRLAmbrosVThe C. elegans heterochronic gene lin-4 encodes small RNAs with antisense complementarity to lin-14Cell19937558438548252621
- Esquela-KerscherASlackFJOncomirs – microRNAs with a role in cancerNat Rev Cancer20066425926916557279
- WangRWuYHuangWChenWMicroRNA-940 targets INPP4A or GSK3β and activates the Wnt/β-catenin pathway to regulate the malignant behavior of bladder cancer cellsOncol Res201826114515528337959
- XuGShaoGPanQMicroRNA-9 regulates non-small cell lung cancer cell invasion and migration by targeting eukaryotic translation initiation factor 5A2Am J Transl Res20179247848828337276
- El BezawyRCominettiDFendericoNmiR-875-5p counteracts epithelial-to-mesenchymal transition and enhances radiation response in prostate cancer through repression of the EGFR-ZEB1 axisCancer Lett2017395536228274892
- JamiesonNBMorranDCMortonJPMicroRNA molecular profiles associated with diagnosis, clinicopathologic criteria, and overall survival in patients with resectable pancreatic ductal adenocarcinomaClin Cancer Res201218253454522114136
- HeLQuLWeiLChenYSuoJReduction of miR-132-3p contributes to gastric cancer proliferation by targeting MUC13Mol Med Rep20171553055306128339011
- JiangJZhangYYuCLiZPanYSunCMicroRNA-492 expression promotes the progression of hepatic cancer by targeting PTENCancer Cell Int20141419525253996
- ZengFCZengMQHuangLDownregulation of VEGFA inhibits proliferation, promotes apoptosis, and suppresses migration and invasion of renal clear cell carcinomaOnco Targets Ther201691213127110129
- LiangBZhaoJWangXA three-microRNA signature as a diagnostic and prognostic marker in clear cell renal cancer: an in silico analysisPLoS One2017126e018066028662155
- YoussefYMWhiteNMGrigullJAccurate molecular classification of kidney cancer subtypes using microRNA signatureEur Urol201159572173021272993
- RitchieMEPhipsonBWuDlimma powers differential expression analyses for RNA-sequencing and microarray studiesNucleic Acids Res2015437e4725605792
- FanYSiklenkaKAroraSKRibeiroPKimminsSXiaJmiR-Net – dissecting miRNA-target interactions and functional associations through network-based visual analysisNucleic Acids Res201644W1W135W14127105848
- SiegelRLMillerKDJemalACancer statistics, 2018CA Cancer J Clin201868173029313949
- SzabóZSzegediKGombosKExpression of miRNA-21 and miRNA-221 in clear cell renal cell carcinoma (ccRCC) and their possible role in the development of ccRCCUrol Oncol20163412533.e21533.e27
- KurozumiAGotoYOkatoAIchikawaTSekiNAberrantly expressed microRNAs in bladder cancer and renal cell carcinomaJ Hum Genet2017621495627357429
- ZhaoJJChenPJDuanRQLiKJWangYZLiYmiR-630 functions as a tumor oncogene in renal cell carcinomaArch Med Sci201612347347827279836
- GaoCPengFHPengLKMiR-200c sensitizes clear-cell renal cell carcinoma cells to sorafenib and imatinib by targeting heme oxygenase-1Neoplasma201461668068925150313
- KowalczykAEKrazinskiBEGodlewskiJSATB1 is down-regulated in clear cell renal cell carcinoma and correlates with miR-21-5p overexpression and poor prognosisCancer Genomics Proteomics201613320927107063
- XieCHCaoYMHuangYLong non-coding RNA TUG1 contributes to tumorigenesis of human osteosarcoma by sponging miR-9-5p and regulating POU2F1 expressionTumour Biol20163711150311504127658774
- OkatoAAraiTYamadaYDual strands of pre-miR-149 inhibit cancer cell migration and invasion through targeting FOXM1 in renal cell carcinomaInt J Mol Sci2017189
- LiuWLiHWangYMiR-30b-5p functions as a tumor suppressor in cell proliferation, metastasis and epithelial-to-mesenchymal transition by targeting G-protein subunit α-13 in renal cell carcinomaGene201762627528128536082
- de LeeuwRMcNairCSchiewerMJMAPK reliance via acquired CDK4/6 inhibitor resistance in cancerClin Can Res2018241742014214
- WangZSunYTargeting p53 for novel anticancer therapyTransl Oncol20103111220165689
- AsemMBuechlerSWatesRMillerDStackMWnt5a signaling in cancerCancers20168979