Abstract
Purpose
Triple negative breast cancer (TNBC) is an intrinsic subtype of breast cancer with a poor prognosis, characterized by a lack of ER and PR expression and the absence of HER2 amplification. The aim of this study is to characterize hub genes (key genes in the molecular interaction network) expression in TNBC, which may serve as prognostic predictors for TNBC treatment.
Methods
Four transcriptome microarray datasets GSE27447, GSE39004, GSE43358 and GSE45827 were obtained from the Gene Expression Omnibus (GEO) database, and R package limma and RobustRankAggreg were employed to identify common differentially expressed genes (DEGs). Gene Ontology (GO) and Kyoto Encyclopedia of Genes and Genomes (KEGG) enrichment analyses were conducted by DAVID and KOBAS database. Thereafter, protein–protein interaction (PPI) network was constructed according to STRING online database. Functional modules and hub genes were screened by MCODE and cytohubba plug-ins, and the Cancer Genome Atlas (TCGA) survival analysis and qRT-PCR were utilized to validate the expression of these hub genes on TNBC.
Results
A total of 134 DEGs were identified by differential expression analysis, consisting of 88 up- and 46 down-regulated genes. GO and KEGG analyses showed that the terms and pathways enriched were mainly associated with cell adhesion, tumorigenesis and cellular immunity. From the PPI network, we identified six hub genes, including CD3D, CD3E, CD3G, FYN, GRAP2 and ITK. Survival analysis and the qRT-PCR results confirmed the robustness of the identified hub genes.
Conclusion
This study provides a new insight into the understanding of the molecular mechanisms associated with TNBC and suggested that the hub genes may serve as prognostic predictors.
Introduction
Breast cancer is one of the most common malignant tumors in women and a leading cause of cancer-associated deaths worldwide.Citation1 At present, breast cancer is categorized into four intrinsic molecular subtypes clinically, based on the presence of estrogen receptor (ER), progesterone receptor (PR), human epidermal growth factor receptor 2 (HER2) and ki67.Citation2 Triple negative breast cancer (TNBC) represents 10% to 20% of all breast cancer cases, and is characterized by the lack of ER and PR expression and the absence of HER2 amplification.Citation3 Compared with other molecular subtypes of breast cancer, TNBC is more commonly diagnosed in young women and is more prone to relapse and metastasis.Citation4–Citation6 Moreover, TNBC patients cannot benefit from endocrine or HER2 targeted therapy due to the absence of molecular targets, and this leads directly to poor clinical outcome. Therefore, it is necessary to further explore the molecular mechanisms underlying TNBC pathogenesis.
During the last decades, the clinical and molecular heterogeneity of breast cancer has been widely recognized. The development and widespread application of high-throughput technologies, including microarray and RNA-sequencing, has provided a novel understanding of the molecular complexity of this disease.Citation7–Citation9 Several studies have demonstrated that TNBC cells express genes characteristic of normal basal/myoepithelial cells, such as KRT5, KRT14, KRT17 and EGFR.Citation10,Citation11 Some molecular features of TNBC have been discovered, including a high frequency of TP53 mutations, aberrant activation of PI3K pathway, inactivation of BRCA1 and BRCA2, RB1 loss, and cyclin E1 amplification.Citation12 However, little is known about the etiological factors which promote the initiation and development of TNBC, and the molecular mechanisms underlying TNBC are far from elucidated.
Therefore, we carried out a series of transcriptome analysis using bioinformatics methods, trying to identify differentially expressed genes (DEGs) between TNBC and non-TNBC samples by analyzing several transcriptome microarray datasets from the Gene Expression Omnibus (GEO) database, and investigate their potential biological functions by performing Gene Ontology (GO) and Kyoto Encyclopedia of Genes and Genomes (KEGG) enrichment analyses. Furthermore, protein–protein interaction (PPI) network was constructed, and six hub genes, which play key roles in TNBC, were screened out. Our study provides a new insight into the understanding of the molecular mechanisms of TNBC, and the identified hub genes may serve as prognostic predictors.
Materials and Methods
Downloading and Processing of Microarray Datasets
Microarray datasets (GSE27447,Citation13 GSE39004,Citation14 GSE43358Citation15 and GSE45827Citation16) were downloaded from GEO (URL: http://www.ncbi.nlm.nih.gov/geo) database for differential expression analysis. The inclusion criteria for the microarray datasets were as follows: (a) samples containing TNBC and non-TNBC tissues, (b) primary tumors with no adjuvant treatment, (c) the total RNA samples for transcriptome testing, (d) expression profiling by the array as the study type, and (e) Homo sapiens as the organism. The probe names were annotated to Entrez ID according to the corresponding GEO platform files. Detailed information of the four microarray datasets is shown in Supplementary Table 1.
Identification of DEGs
The differential expression analysis, for each of the selected GEO datasets, was performed using R package limma (URL: https://www.bioconductor.org/packages/release/bioc/html/limma.html), and the robust multi-array average (RMA) method was used to do the background correction. The PAM50 molecular subtyping was performed by using R package genefu (URL: https://www.bioconductor.org/packages/release/bioc/html/genefu.html). Furthermore, to screen out the common DEGs from all these datasets, we employed the robust rank aggregation (RRA) analysis by applying R package RobustRankAggreg (https://cran.r-project.org/web/packages/RobustRankAggreg/index.html). This algorithm is more credible for DEG identification from different datasets, since it is robust to outliers, noise and errors. Adjusted P-value (Adj.P) <0.05 and |log2 fold-change (FC)| > 1 were used as a threshold.
Functional and Pathway Enrichment Analyses
To further understand the biological functions of these DEGs, GO enrichment analysis of three categories, including biological process (BP), cellular component (CC) and molecular function (MF) were performed using the Database for Annotation, Visualization and Integrated Discovery (DAVID; version 6.8, URL: https://david.ncifcrf.gov/). KEGG pathway analysis was conducted using the KOBAS database (version 3.0, URL: http://kobas.cbi.pku.edu.cn/). False discovery rate (FDR) <0.05 was considered to be of statistical significance.
Construction of PPI Network and the Identification of Hub Genes
To investigate the interactions of proteins encoded by the identified DEGs, we constructed a PPI network by using Cytoscape (version 3.7.1) software, according to the Search Tool for the Retrieval of Interacting Genes (STRING; version 11.0, URL: https://string-db.org/) database. The combined score of 0.7 was considered as a threshold. Thereafter, MCODE (version 1.5, URL: http://apps.cytoscape.org/apps/mcode), a plug-in of Cytoscape, was used to screen significant functional modules from the PPI network, and another plug-in cytohubba (version 0.1, URL: apps.cytoscape.org/apps/cytohubba) was used for hub gene identification. In addition, maximal clique centrality (MCC) method was the algorithm for hub genes identification.
Survival Analysis Using Samples from TCGA Database
To validate the oncogenetic effect of the identified hub genes, we performed survival analyses of TCGA (URL: https://cancergenome.nih.gov/) samples by using the Gene Expression Profiling Interactive Analysis (GEPIA; URL: https://gepia.cancer-pku.cn/) online database. TNBC samples were derived into high- and low-expression groups for each hub gene. The median transcript per million (TPM) value was used as a cutoff. To further verify the association between the hub genes and breast cancer outcome, Cox regression analysis was performed in TCGA samples from multiple malignancies, including breast cancer (BRCA), cervical squamous cell carcinoma (CESC), liver hepatocellular carcinoma (LIHC), head and neck squamous cell carcinoma (HNSC), lung adenocarcinoma (LUAD), sarcoma (SARC) and skin cutaneous melanoma (SKCM). P-value <0.05 was considered statistically significant.
Clinical Samples Collection and Storage
Twenty TNBC and 20 non-TNBC clinical samples were collected from patients who were primarily diagnosed with breast cancer between 2013 and 2019 at the Affiliated Hospital of Jiangnan University. All clinical samples were diagnosed by senior pathologists, and the proportions of tumor cells were over 85%. The specimens were immediately transferred into the gas phase of liquid nitrogen after removal, and subsequently stored at −80°C until further processing. All the patients who were enrolled in the present study had signed informed consent.
RNA Extraction and Quantitative Reverse Transcriptional PCR (qRT-PCR)
Total RNA was extracted from clinical samples by using the E.Z.N.A Total RNA Kit I (Omega, USA) following the protocol. Reverse transcriptional PCR was performed to synthesis complementary DNA (cDNA) by using the Prime Script RT Reagent Kit (Takara Bio, Japan). CFX96 Touch Real-Time PCR Detection System (Bio-rad, USA) was employed to perform real-time PCR, and the comparative Ct method was used to measure the expression levels of the hub genes. Glyceraldehyde‐3‐phosphate dehydrogenase (GAPDH) was considered as a normalization control. The sequences of the primers are demonstrated in Supplementary Table 2.
Results
Identification of DEGs
In this study, we performed a multistep bioinformatic analysis to screen key genes of TNBC, and the flow diagram is shown in . Firstly, to verify the grouping of TNBC and non-TNBC samples in the four datasets (GSE27447, GSE39004, GSE43358 and GSE45827), we performed PAM50 subtyping, and the heatmaps of each dataset are shown in Supplementary Figure 1–4. According to the result, most of the TNBC samples were of basal-like subtype, and this confirmed the representativeness of the TNBC datasets. Subsequently, we performed a differential expression analysis on the datasets, which contain a total of 77 TNBC and 186 non-TNBC samples. The distribution of the DEGs in each dataset is shown in –. Furthermore, the common DEGs among the four datasets were identified by RRA method, including 88 up- and 46 down-regulated genes (). The expression heatmap of top 10 up- and down-regulated DEGs is demonstrated in .
Figure 2 Identification of DEGs in four TNBC microarray datasets from GEO.
Notes: (A–D) Volcano plots of differential expression analysis for GSE27447, GSE39004, GSE43358 and GSE45827. (E) Upset plot of DEGs overlapped in the four datasets. (F) Expression heatmap of top 10 up- and down-regulated genes.
Abbreviations: TNBC, triple negative breast cancer; GEO, Genome Expression Omnibus; DEGs, differentially expressed genes.
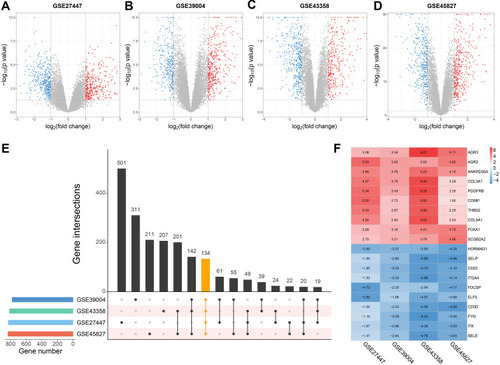
GO and KEGG Pathway Enrichment Analysis
GO and KEGG pathway enrichment analyses were performed to further explore the biological functions of the identified DEGs. The biological process category of the GO analysis results showed that up-regulated DEGs were significantly enriched in phosphatidylinositol 3-kinase signaling, mammary gland alveolus development and cell adhesion. For the cellular component category, these DEGs were enriched in the extracellular matrix, basement membrane and extracellular exosome. Moreover, the DEGs were significantly enriched in platelet-derived growth factor binding and extracellular matrix structural constituent under the molecular function category (). In addition, the most significantly enriched GO terms for down-regulated genes were cell adhesion and extracellular matrix organization (BP); plasma membrane and integrin complex (CC); T cell receptor binding and cell adhesion molecule binding (MF; ).
Figure 3 GO and KEGG pathway enrichment analyses for DEGs.
Notes: (A) Chord plot of GO enrichment analysis of up-regulated genes. (B) Chord plot of GO enrichment analysis of down-regulated genes. (C) Dot plot of KEGG enrichment analysis of up-regulated genes. (D) Dot plot of KEGG enrichment analysis of down-regulated genes.
Abbreviations: GO, Gene Ontology; KEGG, Kyoto Encyclopedia of Genes and Genomes.
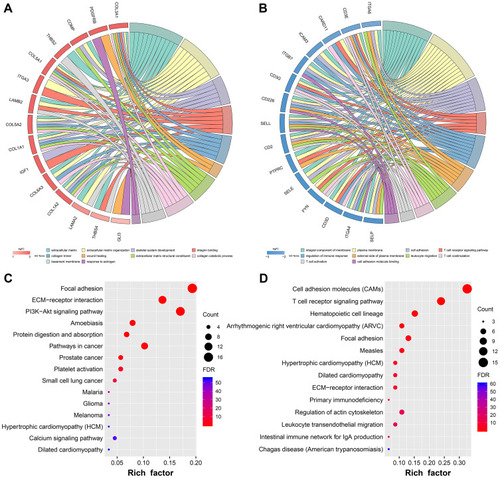
According to KEGG pathway enrichment analysis, the up-regulated DEGs were significantly enriched in focal adhesion, extracellular cell matrix (ECM)-receptor interaction and PI3K-AKT signaling pathway (). Downregulated DEGs were enriched in cell adhesion molecules (CAMs) and T cell receptor signaling pathway ().
PPI Network Construction and Hub Gene Identification
To investigate the interactions between proteins encoded by the DEGs, a PPI network was constructed according to STRING database, which involved 59 nodes and 142 edges (). Thereafter, A plug-in of Cytoscape, MCODE was employed to conduct clustering analysis. Four functional modules were screened out from the PPI network (–). To further understand the potential biological functions of these modules, we performed another KEGG pathway enrichment analyses. The results demonstrated that these modules play roles in cell adhesion, ECM–receptor interaction, pathways in cancer and T-cell immunity ().
Table 1 KEGG Enrichment Analysis of Top four Modules Identified from PPI Network
Figure 4 PPI network construction and hub genes identification.
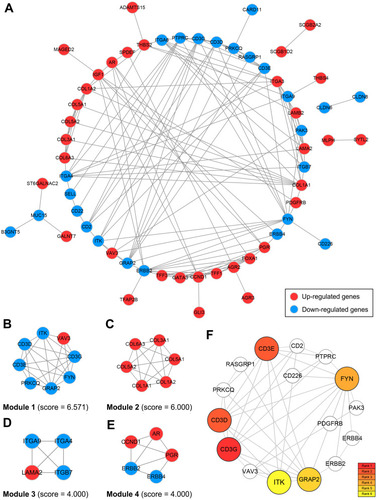
Subsequently, another plug-in of Cytoscape, cytohubba was used for hub genes identification. From the whole PPI network, six hub genes (CD3D, CD3E, CD3G, FYN, GRAP2 and ITK) were screened out according to the ranking by MMC method. The sub-network of the identified hub genes is shown in .
TCGA Survival Analysis and qRT-PCR Validation
To validate the association between identified hub genes expression and patients’ clinical outcomes, 135 TNBC samples from TCGA database were derived into high- and low-expression groups according to the median expression level of each hub gene, respectively. The online tool GEPIA2 was employed to conduct the survival analysis. Among the six hub genes, CD3D, CD3E, GRAP2 and ITK were significantly associated with the overall survival of TNBC (log-rank P < 0.05; –). Furthermore, a survival map of multiple malignancies is shown in . According to the result, the identified hub genes tended to increase the risk of tumor diseases.
Figure 5 Hub genes validation using TCGA data and clinical specimens.
Notes: (A) Kaplan–Meier survival analysis of CD3D. (B) Kaplan–Meier survival analysis of CD3E. (C) Kaplan–Meier survival analysis of GRAP2. (D) Kaplan–Meier survival analysis of ITK. (E) Survival map of the hub genes in multiple malignancies. (F) Expression levels of the hub genes in clinical specimens detected by qRT-PCR.
Abbreviations: TCGA, The Cancer Genome Atlas; qRT-PCR, quantitative reverse transcriptional polymerase chain reaction.
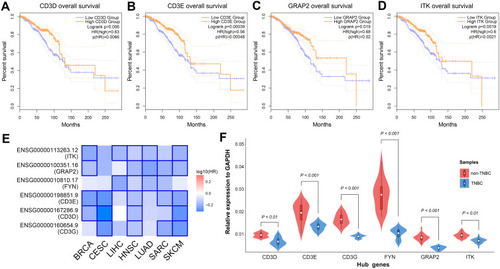
Finally, we validated the expression of the hub genes in clinical samples. As demonstrated in , the relative expression level of CD3D, CD3E, CD3G, FYN, GRAP2 and ITK in TNBC samples were significantly lower than in non-TNBC samples (P < 0.05), and this result was consistent with the differential expression analysis.
Discussion
In the present study, four TNBC datasets were downloaded from GEO, and a total of 134 DEGs (88 up- and 46 down-regulated genes) were identified. The results of GO and KEGG enrichment analyses showed that the DEGs were associated with various cancer-related functions and pathways, such as cell adhesion, ECM–receptor interaction, PI3K-AKT signaling pathway and T-cell immunity. Thereafter, PPI network of the identified DEGs was constructed based on STRING database, and six hub genes were screened out from it using MCC method. Survival analysis and qRT-PCR validation further supported the robustness of the above results.
Due to the development and popularization of the next-generation sequencing technologies, multiple transcriptomics studies of TNBC have been carried out. For instance, in a study based on the original TNBC dataset GSE76275 from GEO,Citation17 207 DEGs were identified, and SOX8, AR, C9orf152, NRK and RAB30 were proposed as hub genes. Another bioinformatics analysis on TNBC transcriptome involved three GEO datasets (GSE38959, GSE45827, and GSE65194), and CCND1 was considered as the potential key gene associated with TNBC prognosis.Citation18 Comparing with these published similar studies, the present study has some advantages: First, we employed the RRA method to analyze the overlapping DEGs from the four datasets. To the best of our knowledge, this algorithm has not been used for TNBC vs non-TNBC transcriptomic expression analysis. The RRA method is parameter-free and robust to outliers, noise and errors. Secondly, the present study involved 77 TNBC and 186 non-TNBC samples from four microarray datasets, and this sample size is larger than most of the other similar studies. These features ensured the credibility of our results.
In the present study, we screened out six hub genes from the whole PPI network. According to the existing studies, the hub genes play key roles in multiple cancer-related biological processes. CD3D, CD3E and CD3G are located in the same cluster on chromosome 11, and encode a group of polypeptide named CD3-delta, CD3-gamma and CD3 epsilon. These polypeptides, along with the T-cell receptor alpha/beta and gamma/delta heterodimers, form the T-cell receptor/CD3 complex (TCR/CD3 complex) which plays an important role in coupling antigen recognition to several intracellular signalling pathways.Citation19,Citation20 It was reported that CD3D, CD3E and CD3G were associated with improved OS in five cancer types, including breast cancer.Citation21 According to the survival analysis of TCGA data, this is consistent with our result. Another immune-associated hub gene, ITK, encodes an essential intracellular tyrosine kinase expressed in T-cells, which is thought to play a role in T-cell proliferation and differentiation.Citation22,Citation23 Research of ibrutinib, an inhibitor of both ITK and BTK, demonstrated that inhibiting ITK could shift the balance between Th1 and Th2 T cells and potentially enhance antitumor immune responses.Citation24 However, the effect of ITK on breast cancer has not been elucidated so far.
GRAP2 locates in chromosome 22q13.1, and the protein it encodes is an adaptor-like protein involved in leukocyte-specific protein-tyrosine kinase signaling.Citation25 FYN encodes a membrane-associated tyrosine kinase that has been implicated in the control of cell growth, and it has been recognized as an oncogene in various tumor diseases, including melanoma, glioblastoma, squamous cell carcinoma, prostate and breast cancers.Citation26–Citation28 Interestingly, according to the survival and expression analysis performed in our study, we observed that FYN was down-regulated in TNBC comparing with non-TNBC, while the expression of FYN was not associated with TNBC prognosis. Further study is still required to validate the bio-functions of the hub genes in vitro and in vivo.
Our study identified six hub genes and various signalling pathways that were strongly associated with T-cell immunity. Accumulating evidence has demonstrated that immuno-biomarkers are of prognostic significance in many tumor types, including breast cancer.Citation29 Unlike other intrinsic subtypes of breast cancer, TNBC is characterized by a higher degree of tumor-infiltrating lymphocytes (TILs),Citation30 and multiple series of investigations have shown that higher TILs are associated with improved overall survival and higher response rate.Citation31–Citation34 In combination with the existing studies, our findings further indicated the important role of immune-related factors in TNBC.
Conclusion
The present study has identified several key genes (CD3D, CD3E, CD3G, FYN, GRAP2 and ITK) which might be considered as novel and potential biomarkers of TNBC. Survival analysis and qRT-PCR validation supported the strong and robust association between the hub genes and TNBC. These results may provide a novel understanding of TNBC tumorigenesis, and further study is urgently needed to elucidate the underlying molecular mechanisms.
Ethics Approval and Consent to Participate
All procedures performed in studies involving human participants were in accordance with the 1964 Helsinki declaration and its later amendments or comparable ethical standards. Each patient enrolled in this study has signed informed consent, and this study was approved by the ethical committee of the Affiliated Hospital of Jiangnan University (Approval No. LS2019052).
Disclosure
The authors report no conflicts of interest in this work.
References
- Siegel RL, Miller KD, Jemal A. Cancer statistics, 2019. CA Cancer J Clin. 2019;69(1):7–34. doi:10.3322/caac.2155130620402
- Savci-Heijink CD, Halfwerk H, Hooijer GK, et al. Retrospective analysis of metastatic behaviour of breast cancer subtypes. Breast Cancer Res Treat. 2015;150(3):547–557. doi:10.1007/s10549-015-3352-025820592
- Kumar P, Aggarwal R. An overview of triple-negative breast cancer. Arch Gynecol Obstet. 2016;293(2):247–269. doi:10.1007/s00404-015-3859-y26341644
- Haffty BG, Yang Q, Reiss M, et al. Locoregional relapse and distant metastasis in conservatively managed triple negative early-stage breast cancer. J Clin Oncol. 2006;24(36):5652–5657. doi:10.1200/JCO.2006.06.566417116942
- Carey LA, Dees EC, Sawyer L, et al. The triple negative paradox: primary tumor chemosensitivity of breast cancer subtypes. Clin Cancer Res. 2007;13(8):2329–2334. doi:10.1158/1078-0432.CCR-06-110917438091
- Malorni L, Shetty PB, De Angelis C, et al. Clinical and biologic features of triple-negative breast cancers in a large cohort of patients with long-term follow-up. Breast Cancer Res Treat. 2012;136(3):795–804. doi:10.1007/s10549-012-2315-y23124476
- Banerji S, Cibulskis K, Rangel-Escareno C, et al. Sequence analysis of mutations and translocations across breast cancer subtypes. Nature. 2012;486(7403):405–409. doi:10.1038/nature1115422722202
- Shah SP, Roth A, Goya R, et al. The clonal and mutational evolution spectrum of primary triple-negative breast cancers. Nature. 2012;486(7403):395–399. doi:10.1038/nature1093322495314
- Curtis C, Shah SP, Chin SF, et al. The genomic and transcriptomic architecture of 2,000 breast tumours reveals novel subgroups. Nature. 2012;486(7403):346–352. doi:10.1038/nature1098322522925
- Parker JS, Mullins M, Cheang MCU, et al. Supervised risk predictor of breast cancer based on intrinsic subtypes. J Clin Oncol. 2009;27(8):1160–1167. doi:10.1200/JCO.2008.18.137019204204
- Foulkes WD, Smith IE, Reis-Filho JS. Triple-negative breast cancer. N Engl J Med. 2010;363(20):1938–1948. doi:10.1056/NEJMra100138921067385
- Anders CK, Abramson V, Tan T, et al. The evolution of triple-negative breast cancer: from biology to novel therapeutics. Am Soc Clin Oncol Educ Book. 2016;35:34–42. doi:10.1200/EDBK_15913527249684
- Yang L, Wu X, Wang Y, et al. FZD7 has a critical role in cell proliferation in triple negative breast cancer. Oncogene. 2011;30(43):4437–4446. doi:10.1038/onc.2011.14521532620
- Terunuma A, Putluri N, Mishra P, et al. MYC-driven accumulation of 2-hydroxyglutarate is associated with breast cancer prognosis. J Clin Invest. 2014;124(1):398–412. doi:10.1172/JCI7118024316975
- Fumagalli D, Blanchet-Cohen A, Brown D, et al. Transfer of clinically relevant gene expression signatures in breast cancer: from Affymetrix microarray to Illumina RNA-sequencing technology. BMC Genomics. 2014;15(1):1008. doi:10.1186/1471-2164-15-100825412710
- Gruosso T, Mieulet V, Cardon M, et al. Chronic oxidative stress promotes H2AX protein degradation and enhances chemosensitivity in breast cancer patients. EMBO Mol Med. 2016;8(5):527–549. doi:10.15252/emmm.20150589127006338
- Dong P, Yu B, Pan L, et al. Identification of key genes and pathways in triple-negative breast cancer by integrated bioinformatics analysis. Biomed Res Int. 2018;2018:2760918. doi:10.1155/2018/276091830175120
- Li MX, Jin LT, Wang TJ, et al. Identification of potential core genes in triple negative breast cancer using bioinformatics analysis. Onco Targets Ther. 2018;11:4105–4112. doi:10.2147/OTT.S16656730140156
- Ngoenkam J, Schamel WW, Pongcharoen S. Selected signalling proteins recruited to the T-cell receptor-CD3 complex. Immunology. 2018;153(1):42–50. doi:10.1111/imm.1280928771705
- Dahlén E, Veitonmäki N, Norlén P. Bispecific antibodies in cancer immunotherapy. Ther Adv Vaccines Immunother. 2018;6(1):3–17. doi:10.1177/251513551876328029998217
- Wang Q, Li P, Wu W. A systematic analysis of immune genes and overall survival in cancer patients. BMC Cancer. 2019;19(1):1225. doi:10.1186/s12885-019-6414-631842801
- Lo HY. Itk inhibitors: a patent review. Expert Opin Ther Pat. 2010;20(4):459–469. doi:10.1517/1354377100367440920218931
- Tillman BF, Pauff JM, Satyanarayana G, et al. Systematic review of infectious events with the Bruton tyrosine kinase inhibitor ibrutinib in the treatment of hematologic malignancies. Eur J Haematol. 2018;100(4):325–334. doi:10.1111/ejh.1302029285806
- Sagiv-Barfi I, Kohrt HE, Czerwinski DK, et al. Therapeutic antitumor immunity by checkpoint blockade is enhanced by ibrutinib, an inhibitor of both BTK and ITK. Proc Natl Acad Sci U S A. 2015;112(9):E966–E972. doi:10.1073/pnas.150071211225730880
- Billadeau DD. T cell activation at the immunological synapse: vesicles emerge for LATer signaling. Sci Signal. 2010;3(121):pe16. doi:10.1126/scisignal.3121pe1620460646
- Noble M, Mayer-Pröschel M, Li Z, et al. Redox biology in normal cells and cancer: restoring function of the redox/Fyn/c-Cbl pathway in cancer cells offers new approaches to cancer treatment. Free Radic Biol Med. 2015;79:300–323.25481740
- Lee CG, Koo JH, Kim SG. Phytochemical regulation of Fyn and AMPK signaling circuitry. Arch Pharm Res. 2015;38(12):2093–2105. doi:10.1007/s12272-015-0611-x25951818
- Elias D, Ditzel HJ. Fyn is an important molecule in cancer pathogenesis and drug resistance. Pharmacol Res. 2015;100:250–254. doi:10.1016/j.phrs.2015.08.01026305432
- Stovgaard ES, Nielsen D, Hogdall E, et al. Triple negative breast cancer – prognostic role of immune-related factors: a systematic review. Acta Oncol. 2018;57(1):74‐82. doi:10.1080/0284186X.2017.1400180
- Vikas P, Borcherding N, Zhang W. The clinical promise of immunotherapy in triple-negative breast cancer. Cancer Manag Res. 2018;10:6823–6833. doi:10.2147/CMAR.S18517630573992
- Loi S, Michiels S, Salgado R, et al. Tumor infiltrating lymphocytes are prognostic in triple negative breast cancer and predictive for trastuzumab benefit in early breast cancer: results from the FinHER trial. Ann Oncol. 2014;25(8):1544–1550. doi:10.1093/annonc/mdu11224608200
- Adams S, Goldstein LJ, Sparano JA, et al. Tumor infiltrating lymphocytes (TILs) improve prognosis in patients with triple negative breast cancer (TNBC). Oncoimmunology. 2015;4(9):e985930. doi:10.4161/2162402X.2014.98593026405612
- Loi S, Sirtaine N, Piette F, et al. Prognostic and predictive value of tumor-infiltrating lymphocytes in a Phase III randomized adjuvant breast cancer trial in node-positive breast cancer comparing the addition of docetaxel to doxorubicin with doxorubicin-based chemotherapy: BIG 02-98. J Clin Oncol. 2013;31(7):860–867. doi:10.1200/JCO.2011.41.090223341518
- Dieci MV, Criscitiello C, Goubar A, et al. Prognostic value of tumor-infiltrating lymphocytes on residual disease after primary chemotherapy for triple-negative breast cancer: a retrospective multicenter study. Ann Oncol. 2014;25(3):611–618. doi:10.1093/annonc/mdt55624401929