Abstract
Introduction
Nasopharyngeal carcinoma (NPC) is endemic to Eastern and South-Eastern Asia, and, in 2020, 77% of global cases were diagnosed in these regions. Apart from its distinct epidemiology, the natural behavior, treatment, and prognosis are different from other head and neck cancers. With the growing trend of artificial intelligence (AI), especially deep learning (DL), in head and neck cancer care, we sought to explore the unique clinical application and implementation direction of AI in the management of NPC.
Methods
The search protocol was performed to collect publications using AI, machine learning (ML) and DL in NPC management from PubMed, Scopus and Embase. The articles were filtered using inclusion and exclusion criteria, and the quality of the papers was assessed. Data were extracted from the finalized articles.
Results
A total of 78 articles were reviewed after removing duplicates and papers that did not meet the inclusion and exclusion criteria. After quality assessment, 60 papers were included in the current study. There were four main types of applications, which were auto-contouring, diagnosis, prognosis, and miscellaneous applications (especially on radiotherapy planning). The different forms of convolutional neural networks (CNNs) accounted for the majority of DL algorithms used, while the artificial neural network (ANN) was the most frequent ML model implemented.
Conclusion
There is an overall positive impact identified from AI implementation in the management of NPC. With improving AI algorithms, we envisage AI will be available as a routine application in a clinical setting soon.
Introduction
According to the International Agency for Research on Cancer, nasopharyngeal carcinoma (NPC) is the twenty-third most common cancer worldwide. The global number of new cases and deaths in 2020 were 133,354 and 80,008, respectively.Citation1,Citation2 Although it is not uncommon, it has a distinct geographical distribution where it is most prevalent in Eastern and South-Eastern Asia, accounting for 76.9% of global cases. It was also found that almost half of the new cases occurred in China.Citation2 Because of its late symptoms and anatomical location, it makes it difficult to be detected in the early stages. Radiotherapy is the primary treatment modality, and concomitant/adjunctive chemotherapy is often needed for advanced locoregional disease.Citation3 Furthermore, there are many organs-at-risk (OARs) nearby that are sensitive to radiation; these include the salivary glands, brainstem, optic nerves, temporal lobes and the cochlea.Citation4 Hence, it is of interest whether the use of artificial intelligence (AI) can help improve the diagnosis, treatment process and prediction of outcomes for NPC.
With the advances of AI over the past decade, it has become pervasive in many industries playing both major and minor roles. This includes cancer treatment, where medical professionals search for methods to utilize it to improve treatment quality. AI refers to any method that allows algorithms to mimic intelligent behavior. It has two subsets, which are machine learning (ML) and deep learning (DL). ML uses statistical methods to allow the algorithm to learn and improve its performance, such as random forest and support vector machine. Artificial neural network (ANN) is an example of ML and is also a core part of DL.Citation5 DL can be defined as a learning algorithm that can automatically update its parameters through multiple layers of ANN. Deep neural networks such as convolutional neural network (CNN) and recurrent neural network are all DL architectures.
Besides histological, clinical and demographic information, a wide range of data ranging from genomics, proteomics, immunohistochemistry, and imaging must be integrated by physicians when developing personalized treatment plans for patients. This has led to an interest in developing computational approaches to improve medical management by providing insights that will enhance patient outcomes and workflow throughout a patient’s journey.
Given the increased use of AI in cancer care, in this systematic literature review, papers on AI applications for NPC management were compiled and studied in order to provide an overview of the current trend. Furthermore, possible limitations discussed within the articles were explored.
Methods
Search Protocol
A systematic literature search was conducted to retrieve all studies that used AI or its subfields in NPC management. Keywords were developed and combined using boolean logic to produce the resulting search phrase: (“artificial intelligence” OR “machine learning” OR “deep learning” OR “Neural Network”) AND (“nasopharyngeal carcinoma” OR “nasopharyngeal cancer”). Using the search phrase, a search of research articles from the past 15 years to March 2021 was performed on PubMed, Scopus and Embase. The results from the three databases were consolidated, and duplicates were removed. The Preferred Reporting Items for Systematic Review and Meta-Analyses (PRISMA) was followed where possible, and the PRISMA flow diagram and checklist were used as a guidelines to consider the key aspects of a systematic literature review.Citation6
Eligibility
Exclusion and inclusion criteria were determined to assess the eligibility of the retrieved publications. The articles were first checked to remove those that were not within the exclusion criteria. These included book chapters, conference reports, literature reviews, editorials, letters to the editors and case reports. In addition, articles in languages other than English or Chinese and papers with inaccessible full-texts were also excluded.
The remaining studies were then filtered by reading the title and abstract to remove any articles that were not within the inclusion criteria (applications of AI or its subfield and experiments on NPC). A full-text review was further performed to confirm the eligibility of the articles based on both these criteria. The process was conducted by two independent reviewers (B.B & H.C.).
Data Extraction
Essential information from each article was extracted and placed in a data extraction table (). These included the author(s), year of publication, country, sample type, sample size, AI algorithm used, application type, study aim, performance metrics reported, results, conclusion, and limitations. The AI model with the best performance metrics from each study was selected and included. Moreover, the performance results of models trained with the training cohort were obtained from evaluating the test cohort instead of the training cohort. This was to prevent overfitting by avoiding to train and test models with the same dataset.
Table 1 Data Extraction Table
Quality Assessment
The selected articles were assessed for risk of bias and applicability using the Quality Assessment of Diagnostic Accuracy Studies (QUADAS)-2 tool in .Citation7 Studies with more than one section rated “high” or “unclear” were eliminated. Further quality assessment was also completed to ensure the papers meet the required standard. This was performed using the guidelines for developing and reporting ML predictive models from Luo et al and Alabi et al ().Citation8,Citation9 The guideline was summarised, and a mark was given for each guideline topic followed. The threshold was set at half of the maximum marks, and the score was presented in .
Table 2 Quality Assessment via the QUADAS-2 Tool
Table 3 Quality Assessment Guidelines
Table 4 Quality Scores of the Finalized Articles
Results
Database Search
The selection process was performed using the PRISMA flow diagram in . 304 papers were retrieved from the three databases. After 148 duplicates were removed, one inaccessible article was rejected. The papers not meeting the inclusion (n=59) and exclusion (n=20) criteria were also filtered out. Moreover, two additional studies found in literature reviews were included after removing one for being duplicated and another that did not meet the exclusion criteria. Finally, 78 papers were then assessed for quality ().
Figure 1 PRISMA flow diagram 2020.
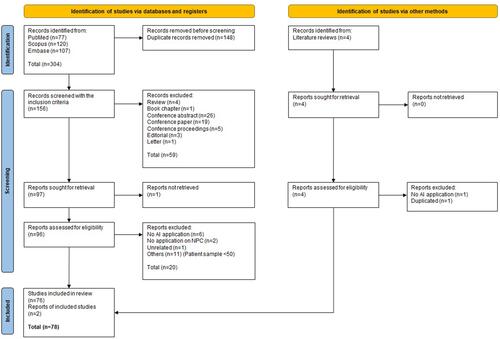
Quality Assessment
18 papers failed due to having more than one section with a “high” or “unclear” rating, leaving 60 studies to be further evaluated. The QUADAS-2 tool showed that 48.3% of articles showed an overall low risk of bias, while 98.3% of them had a low concern regarding applicability ().
An additional evaluation was performed based on , which was adapted from the guidelines by Luo et al and the modified version from Alabi et alCitation8,Citation9 Of the 60 relevant studies, 52 of them scored greater than 70% (). It should also be noted that 23 papers included the evaluation criteria items but did not fully follow the structure of the proposed guidelines.Citation10−Citation32 However, this only affects the ease of reading and extracting information from the articles, but not the content and quality of the papers.
Characteristics of Relevant Studies
The characteristics of the 7articles finally included in the current study were shown in . The articles were published in either English (n=57)Citation10−Citation66 or Chinese (n=3);Citation67−Citation69 3 studies examined sites other than the NPC.Citation10,Citation17,Citation34
When observing the origins of the studies, 45 were published in Asia, while Morocco and France contributed one study each. Furthermore, 13 papers were collaborated work from multiple countries. The majority of the studies were from the endemic regions.
The articles used various types of data to train the models. 66.7% (n=40) only used imaging data such as magnetic resonance imaging, computed tomography or endoscopic images.Citation15,Citation16,Citation18,Citation19,Citation21–Citation24,Citation26–Citation28,Citation30,Citation32,Citation34,Citation37–Citation39,Citation41–Citation43,Citation45–Citation56,Citation58–Citation63,Citation67,Citation69 There were also four studies that included clinicopathological data as well as images for training models,Citation25,Citation31,Citation36,Citation40 while three other studies developed models using images, clinicopathological data, and plasma Epstein-Barr virus (EBV) DNA.Citation29,Citation33,Citation35 Furthermore, 4 studies used treatment plans,Citation64–Citation66,Citation68 while proteins and microRNA expressions data were each extracted by one study.Citation10,Citation44 There were also four articles that trained with both clinicopathological and plasma EBV DNA/serology data,Citation12–Citation14,Citation17 while one article trained its model with clinicopathological and dosimetric data.Citation57 Risk factors (n=2), such as demographic, medical history, familial cancer history, dietary, social and environmental factors, were also used to develop AI models.Citation11,Citation20
The studies could be categorized into 4 domains, which were auto-contouring (n=21),Citation15,Citation16,Citation18,Citation22,Citation24,Citation30–Citation32,Citation45–Citation55,Citation67,Citation69 diagnosis (n=17),Citation10,Citation15,Citation16,Citation23,Citation26,Citation27,Citation49,Citation52,Citation54,Citation56–Citation63 prognosis (n=20)Citation12–Citation14,Citation17,Citation19,Citation25,Citation28,Citation29,Citation33–Citation44 and miscellaneous applications (n=7),Citation11,Citation20,Citation21,Citation64–Citation66,Citation68 which included risk factor identification, image registration and radiotherapy planning (). Five studies examined both diagnosis and auto-contouring simultaneously.Citation15,Citation16,Citation49,Citation52,Citation54
Figure 2 Comparison of studies on AI application for NPC management. (A) Application types of AI and its subfields on NPC; (B) Main performance metrics of application types on NPC.
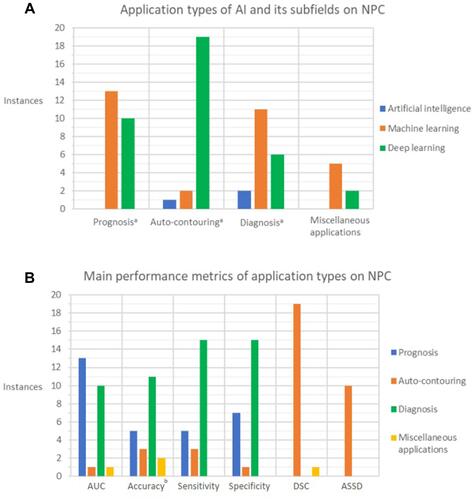
Analyses on the purpose of the application showed that, only in auto-contouring, DL is the most heavily used (with 19 out of 22 instances). For the rest of the categories (NPC diagnosis, prognosis and miscellaneous applications), ML is the most common technique (more than half of the publications in each category) (). In addition, studies applying DL models selected in this literature review were published from 2017 to 2021, where there was a heavier focus on experimenting with DL. It was observed that the majority of the papers applying DL models used various forms of CNN (n=30),Citation15,Citation18,Citation19,Citation21–Citation24,Citation28–Citation34,Citation36,Citation45–Citation53,Citation55,Citation56,Citation60,Citation65,Citation67,Citation69 while the main ML method used was ANN (n=12).Citation13,Citation16,Citation26,Citation42–Citation44,Citation54,Citation61–Citation64,Citation68
The primary metrics reported were the area under the receiver operating characteristic curve (AUC), accuracy, sensitivity, specificity, dice similarity coefficient (DSC) and average symmetric surface distance (ASSD), as shown in .
AUC was used to evaluate the models’ capabilities in 25 papers, with the majority measuring the prognostic (n=13)Citation12–Citation14,Citation19,Citation28,Citation33–Citation35,Citation37,Citation39,Citation40,Citation42,Citation44 and diagnostic abilities (n=10).Citation15,Citation23,Citation26,Citation27,Citation49,Citation56–Citation60 Similarly, accuracy was the parameter most frequently reported in the diagnosis and prognosis application: 11 and 5 out of 20 articles respectively.Citation10,Citation12,Citation15,Citation26–Citation28,Citation35,Citation43,Citation44,Citation49,Citation54,Citation56,Citation60–Citation63 Sensitivity was the most common studied parameter for diagnostic performance: 15 out of 23 papers.Citation10,Citation15,Citation16,Citation23,Citation26,Citation27,Citation49,Citation52,Citation54,Citation56,Citation59–Citation63 The specificity was only reported for prognosis (n=7)Citation12,Citation14,Citation28,Citation34,Citation39,Citation40,Citation43 and diagnosis (n=15).Citation10,Citation15,Citation16,Citation23,Citation26,Citation27,Citation49,Citation52,Citation54,Citation56,Citation59–Citation63 In addition, the DSC (n=20)Citation15,Citation18,Citation22,Citation24,Citation30–Citation32,Citation45–Citation53,Citation55,Citation65,Citation67,Citation69 and ASSD (n=10)Citation18,Citation22,Citation24,Citation31,Citation32,Citation45,Citation46,Citation48,Citation51,Citation69 were the primary metrics reported in studies on auto-contouring ().
Performance metrics with five or more instances of each application method were presented in a boxplot (). The median AUC, accuracy, sensitivity and specificity of prognosis were 0.8000, 0.8300, 0.8003 and 0.8070 respectively, while their range were 0.6330–0.9510, 0.7559–0.9090, 0.3440–0.9200 and 0.5200–1.000 respectively. For diagnosis, the AUC’s median was 0.9300, while the median accuracy was 0.9150. In addition, the median sensitivity and specificity were 0.9307 and 0.9413, respectively. The range for diagnosis’ AUC, accuracy, sensitivity and specificity were 0.6900–0.9900, 0.6500–0.9777, 0.0215–1.000 and 0.8000–1.000, respectively. The median DSC value for auto-contouring was 0.7530, while the range was 0.6200–0.9340. Furthermore, the median ASSD for auto-contouring was 1.7350 mm, and the minimum and maximum values found in the studies were 0.5330 mm and 3.4000 mm, respectively.
Figure 3 Performance metric boxplots of AI application types on NPC. (A) Prognosis and diagnosis: accuracy, AUC, sensitivity and specificity metric; (B) Auto-contouring: DSC metric; (C) Auto-contouring: ASSD metric.
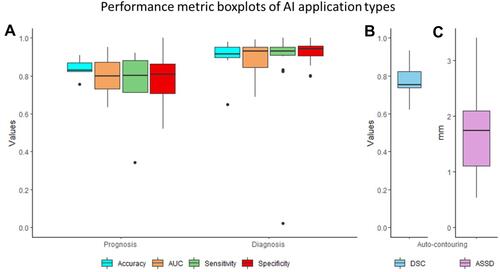
Auto-Contouring
Publications on auto-contouring experimented on segmenting gross tumor volumes, clinical target volume, OARs and primary tumor volumes. The target most delineated was the gross target volume (n=7),Citation30,Citation48,Citation49,Citation51,Citation53,Citation55,Citation69 while the second most were the OARs (n=3).Citation50,Citation52,Citation67 The clinical target volumes and the primary tumor volume were studied in two and one articles respectively.Citation46,Citation55,Citation56 However, nine articles did not mention the specific target volume contoured.Citation15,Citation16,Citation18,Citation22,Citation24,Citation31,Citation32,Citation47,Citation54 Two out of three articles reported that the DSC for delineating optic nerves was substantially lower than the other OARs.Citation52,Citation67 In contrast, for the remaining paper, although the segmentation of the optic nerve is not the worst, the paper reported that the three OARs it tested, which included optic nerves, were specifically more challenging to contour.Citation50 This is because of the low soft-tissue contrast in computed tomography images and their diverse morphological characteristics. When analyzing the OARs, automatic delineation of the eyes yielded the best DSC. Furthermore, apart from the spinal cord, optic nerve and optic chiasm, the AI models have a DSC value greater than 0.8 when contouring OARs.Citation50,Citation52,Citation67
Diagnosis
As for the detection of NPC, six papers compared the performance of AI and humans. Two of them found that AIs had better diagnostic capabilities than humans (oncologists and experienced radiologists),Citation15,Citation49 while another two reported that AIs had similar performances to ear, nose and throat specialists.Citation16,Citation62 However, the last two papers found that it depends on the experience of the person. For example, senior-level clinicians performed better than the AI, while junior level ones were worse.Citation23,Citation60 This is because of the variations in possible sizes, shapes, locations, and image intensities of NPC, making it difficult to determine the diagnosis. These factors make it challenging for clinicians with less experience, and it showed that AI diagnostic tools could support junior-level clinicians.
On the other hand, within the 17 papers experimenting on the diagnostic application of AI, three articles analyzed radiation-induced injury diagnosis.Citation27,Citation57,Citation58 Two of which were concerned with radiation-induced temporal lobe injury,Citation57,Citation58 while the remaining one predicted the fibrosis level of neck muscles after radiotherapy.Citation27 It was suggested that through early detection and prediction of radiation-induced injuries, preventive measures could be taken to minimize the side effects.
Prognosis
For studies on NPC prognosis, 11 out of 20 publications focused on predicting treatment outcomes, with the majority including disease-free survival as one of the study objectives.Citation12,Citation13,Citation17,Citation19,Citation29,Citation33,Citation36,Citation39–Citation42 The rest studied treatment response prediction (n=2),Citation35,Citation43 predicting patients’ risk of survival (n=5),Citation14,Citation25,Citation37,Citation38,Citation44 T staging prediction and the prediction of distant metastasis (n=2).Citation28,Citation34 Therefore, the versatility of AI in different functionalities was demonstrated. The performances of the models were reported in () and the main metric analyzed was AUC with 13 out of 25 articles ().
Miscellaneous Applications
In addition to the above aspects, AI was also used to study risk factor identification (n=2),Citation11,Citation20 image registration (n=1)Citation21 and dose/dose-volume histogram (DVH) distribution (n=4).Citation64–Citation66,Citation68 In particular, dose/DVH distribution prediction was frequently used for treatment planning. A better understanding of the doses given to the target and OARs can help clinicians give a more individualized treatment plan with better consistency and a lower planning duration. However, further development is required to obtain similar plan qualities as created by people. This is because one paper’s model showed the same quality as manual planning by an experienced physicist,Citation64 but another study using a different model was unable to achieve a similar plan quality designed by even a junior physicist.Citation68
Discussion
As evident in this systematic review, there is an exponential growth in interest to apply AI for the clinical management of NPC. A large proportion of the articles collected were published from 2019 to 2021 (n=45) compared to that from 2010 to 2018 (n=15).
A heavier focus is also placed on specific fields of AIs, such as ML and DL. There are only three reports on AI, while there are 31 studies on ML and 37 on DL. The choice of AI subfield sometimes depends on the task. For example, 86% of the papers focused on DL for NPC auto-contouring (n=19), while although the majority of the studies in the other applications used ML, they were more evenly distributed (). The reason why there is such a significant difference in the type of AI used in auto-contouring may be due to the capability of the algorithms and the nature of the data. The medical images acquired have many factors affecting the auto-contouring quality; these include the varying tumour sizes and shapes, image resolution, contrast between regions, noise and lack of consistency during data acquisition being collected from different institutions.Citation70 Because of these challenges, ML-based algorithms have difficulty in performing automated segmentation on NPC as image processing before training is required, which is time-consuming. Furthermore, handcrafted features are necessary to precisely contour each organ or tumour as there are significant variations in size and shape for NPC. On the other hand, DL does not have this issue as they can process the raw data directly without the need for handcrafted features.Citation70
ANN is the backbone of DL, as DL algorithms are ANNs with multiple (2 or more) hidden layers. In the development of AI applications for NPC, 80% of the studied articles incorporated either ANN or DL technique in their modelsCitation12,Citation13,Citation15–Citation19,Citation21–Citation26,Citation28–Citation34,Citation36,Citation38,Citation39,Citation42–Citation56,Citation60–Citation69 because neural networks are generally better for image recognition. However, one study cautioned that ANNs were not necessarily better than other ML models in NPC identification.Citation61 Hence, even though DL-based models and ANNs should be considered the primary development focus, other ML techniques should still not be neglected.
Based on the literature collected, the integration of AI applications in each category is beneficial to the practitioner. Automated contouring by AIs not only can make contouring less time-consuming for clinicians,Citation46,Citation51,Citation53,Citation64 it can also help to improve the user’s accuracy.Citation51 Similarly, AI can be used to reduce the treatment planning time for radiotherapy,Citation64 thus improve the efficiency and effectiveness of the radiotherapy planning process.
For some NPC studies, additional features from images and parameters were extracted to further improve the performances of models. However, it should be noted that not all features are suitable as some features have a more significant impact on the model’s performance than others.Citation40,Citation57,Citation58,Citation61 Therefore, feature selection should be considered where possible.
At its current state, AI cannot yet replace humans to perform the most complex and time-consuming tasks. This is because multiple articles which compared the performance of their developed model with medical professionals showed conflicting results. The reason for this is that the experience of the clinician is an important factor that affects the resulting comparison. The models developed by Chuang et al and Diao et al performed better than junior-level professionals, but performed worse when compared to more experienced clinicians.Citation23,Citation60 One article even showed that an AI model had a lower capability than a junior physicist.Citation68 Furthermore, the quality of the training data and the experience of the AI developers are critical.
The review revealed that AI at its current state still has several limitations. The first concern was the uncertainty regarding the generalizability of the models, because datasets of many studies are retrospective and single institutional in nature.Citation15,Citation19,Citation28,Citation33,Citation35–Citation38,Citation41,Citation48,Citation57–Citation59 The dataset may not represent the true population and may only represent a population subgroup or a region. Hence, this reduces the applicability of the models and affects their performance when applied to other datasets. Another reason was the difference in scan protocol between institutions. Variations in tissue contrasts or field of views may affect the performance as the model was not trained for the same condition.Citation45,Citation56 Therefore, consistency of scan protocols among different institutions is important to facilitate AI model training and validation.
Another limitation was the small amount of data used to train the models. 33% (n=20) of the articles chosen had ≤150 total samples for both training and testing the model. The reason for this was not only were the articles usually based on single-centre data, but also because NPC is less common compared to other cancers. This particularly affects DL-based models as they are more reliant on a much larger dataset to achieve their potential when compared to ML models; over-fitting will likely occur when there is only limited data; thus, data augmentation is often used to increase the dataset size. In addition, some studies had patient selection bias, while others had concerns about not implementing multi-modality inputs into the training model ().
Future work should address these issues when developing new models. Possible solutions include incorporating other datasets or cooperating with other institutions for external validation or to expand the dataset, which were lacking in most of the analysed papers in this review. The former suggestion can boost generalizability and avoid any patient selection bias, while the latter method can increase the capability of the AI models by providing more training samples. Other methods to expand dataset have also been explored, one of which is by using big data which can be done at a much larger scale. Big data can be defined as the vast data generated by technology and the internet of things, allowing easier access to information.Citation71 In the healthcare sector, it will allow easier access to an abundance of medical data which will facilitate AI model training. However, with the large collection of data, privacy protection becomes a serious challenge. Therefore, future studies are required to investigate how to implement it.
The performances of the AI models could also be improved by increasing the amount of data and diversifying it with data augmentation techniques which were performed in some of the studies. However, it should be noted that with an increase in training samples, more data labelling will be required, making the process more time-consuming. Hence, one study proposed the use of continual learning, which it found to boost the model’s performance while reducing the labelling effort.Citation47 However, continual learning is susceptible to catastrophic forgetting, which is a long-standing and highly challenging issue.Citation72 Thus, further investigation into methods to resolve this problem would be required to make it easier to implement in other research settings.
There are several limitations in this literature review. The metric performance results extracted from the publications were insufficient to perform a meta-analysis. Hence, the insight obtained from this review is not comprehensive enough. The quality of the included studies was also not consistent, which may affect the analysis performed.
Conclusion
There is growing evidence that AI can be applied in various situations, particularly as a supporting tool in prognostic, diagnostic and auto-contouring applications and to provide patients with a more individualized treatment plan. DL-based algorithm was found to be the most frequently used AI subfield and usually obtained good results when compared to other methods. However, limited dataset and generalizability are key challenges that need to be overcome to further improve the performances and accessibility of AI models. Nevertheless, studies on AI demonstrated highly promising potential in supporting medical professionals in the management of NPC; therefore, more concerted efforts in swift development is warranted.
Disclosure
Dr Nabil F Saba reports personal fees from Merck, GSk, Pfizer, Uptodate, and Springer, outside the submitted work; and Research funding from BMS and Exelixis. Professor Raymond KY Tsang reports non-financial support from Atos Medical Inc., outside the submitted work. The authors report no other conflicts of interest in this work.
References
- Sung H, Ferlay J, Siegel RL, et al. Global cancer statistics 2020: GLOBOCAN estimates of incidence and mortality worldwide for 36 cancers in 185 countries. CA Cancer J Clin. 2021;71(3):209–249. doi:10.3322/caac.21660
- Ferlay J, Ervik M, Lam F, et al. Global cancer observatory: cancer today; 2020. Available from: https://gco.iarc.fr/today. Accessed June 4, 2021.
- Lee AWM, Ma BBY, Ng WT, Chan ATC. Management of nasopharyngeal carcinoma: current practice and future perspective. J Clin Oncol. 2015;33(29):3356–3364. doi:10.1200/JCO.2015.60.9347
- Chan JW, Parvathaneni U, Yom SS. Reducing radiation-related morbidity in the treatment of nasopharyngeal carcinoma. Future Oncology. 2017;13(5):425–431. doi:10.2217/fon-2016-0410
- Shimizu H, Nakayama KI. Artificial intelligence in oncology. Cancer Sci. 2020;111(5):1452–1460. doi:10.1111/cas.14377
- Page MJ, McKenzie JE, Bossuyt PM, et al. The PRISMA 2020 statement: an updated guideline for reporting systematic reviews. BMJ. 2021;372:n71. doi:10.1136/bmj.n71
- Whiting PF, Rutjes AWS, Westwood ME. QUADAS-2: a revised tool for the quality assessment of diagnostic accuracy studies. Ann Intern Med. 2011;155(8):529–536. doi:10.7326/0003-4819-155-8-201110180-00009
- Luo W, Phung D, Tran T, et al. Guidelines for developing and reporting machine learning predictive models in biomedical research: a multidisciplinary view. J Med Internet Res. 2016;18(12):e323. doi:10.2196/jmir.5870
- Alabi RO, Youssef O, Pirinen M, et al. Machine learning in oral squamous cell carcinoma: current status, clinical concerns and prospects for future—A systematic review. Artif Intell Med. 2021;115:102060. doi:10.1016/j.artmed.2021.102060
- Wang HQ, Zhu HL, Cho WCS, Yip TTC, Ngan RKC, Law SCK. Method of regulatory network that can explore protein regulations for disease classification. Artif Intell Med. 2010;48(2):119–127. doi:10.1016/j.artmed.2009.07.011
- Aussem A, de Morais SR, Corbex M. Analysis of nasopharyngeal carcinoma risk factors with Bayesian networks. Artif Intell Med. 2012;54(1):53–62. doi:10.1016/j.artmed.2011.09.002
- Kumdee O, Bhongmakapat T, Ritthipravat P. Prediction of nasopharyngeal carcinoma recurrence by neuro-fuzzy techniques. Fuzzy Sets Syst. 2012;203:95–111. doi:10.1016/j.fss.2012.03.004
- Ritthipravat P, Kumdee O, Bhongmakap T. Efficient missing data technique for prediction of nasopharyngeal carcinoma recurrence. Inf Technol J. 2013;12:1125–1133. doi:10.3923/itj.2013.1125.1133
- Jiang R, You R, Pei X-Q, et al. Development of a ten-signature classifier using a support vector machine integrated approach to subdivide the M1 stage into M1a and M1b stages of nasopharyngeal carcinoma with synchronous metastases to better predict patients’ survival. Oncotarget. 2016;7(3):3645–3657. doi:10.18632/oncotarget.6436
- Li C, Jing B, Ke L, et al. Development and validation of an endoscopic images-based deep learning model for detection with nasopharyngeal malignancies. Cancer Commun. 2018;38(1):59. doi:10.1186/s40880-018-0325-9
- Mohammed MA, Abd Ghani MK, Arunkumar N, Mostafa SA, Abdullah MK, Burhanuddin MA. Trainable model for segmenting and identifying Nasopharyngeal carcinoma. Comput Electr Eng. 2018;71:372–387. doi:10.1016/j.compeleceng.2018.07.044
- Jing B, Zhang T, Wang Z, et al. A deep survival analysis method based on ranking. Artif Intell Med. 2019;98:1–9. doi:10.1016/j.artmed.2019.06.001
- Ma Z, Zhou S, Wu X, et al. Nasopharyngeal carcinoma segmentation based on enhanced convolutional neural networks using multi-modal metric learning. Phys Med Biol. 2019;64(2):025005. doi:10.1088/1361-6560/aaf5da
- Peng H, Dong D, Fang M-J, et al. Prognostic value of deep learning PET/CT-based radiomics: potential role for future individual induction chemotherapy in advanced nasopharyngeal carcinoma. Clin Cancer Res. 2019;25(14):4271–4279. doi:10.1158/1078-0432.CCR-18-3065
- Rehioui H, Idrissi A. On the use of clustering algorithms in medical domain. Int J Artifi Intell. 2019;17:236.
- Zou M, Hu J, Zhang H, et al. Rigid medical image registration using learning-based interest points and features. Comput Mater Continua. 2019;60(2):511–525. doi:10.32604/cmc.2019.05912
- Chen H, Qi Y, Yin Y, et al. MMFNet: a multi-modality MRI fusion network for segmentation of nasopharyngeal carcinoma. Neurocomputing. 2020;394:27–40. doi:10.1016/j.neucom.2020.02.002
- Chuang W-Y, Chang S-H, Yu W-H, et al. Successful identification of nasopharyngeal carcinoma in nasopharyngeal biopsies using deep learning. Cancers (Basel). 2020;12(2):507. doi:10.3390/cancers12020507
- Guo F, Shi C, Li X, Wu X, Zhou J, Lv J. Image segmentation of nasopharyngeal carcinoma using 3D CNN with long-range skip connection and multi-scale feature pyramid. Soft Comput. 2020;24(16):12671–12680. doi:10.1007/s00500-020-04708-y
- Jing B, Deng Y, Zhang T, et al. Deep learning for risk prediction in patients with nasopharyngeal carcinoma using multi-parametric MRIs. Comput Methods Programs Biomed. 2020;197:105684. doi:10.1016/j.cmpb.2020.105684
- Mohammed MA, Abd Ghani MK, Arunkumar N, et al. Decision support system for nasopharyngeal carcinoma discrimination from endoscopic images using artificial neural network. J Supercomput. 2020;76(2):1086–1104. doi:10.1007/s11227-018-2587-z
- Wang J, Liu R, Zhao Y, et al. A predictive model of radiation-related fibrosis based on the radiomic features of magnetic resonance imaging and computed tomography. Transl Cancer Res. 2020;9(8):4726–4738. doi:10.21037/tcr-20-751
- Yang Q, Guo Y, Ou X, Wang J, Hu C. Automatic T staging using weakly supervised deep learning for nasopharyngeal carcinoma on MR images. Journal of Magnetic Resonance Imaging. 2020;52(4):1074–1082. doi:10.1002/jmri.27202
- Zhong L-Z, Fang X-L, Dong D, et al. A deep learning MR-based radiomic nomogram may predict survival for nasopharyngeal carcinoma patients with stage T3N1M0. Radiother Oncol. 2020;151:1–9. doi:10.1016/j.radonc.2020.06.050
- Bai X, Hu Y, Gong G, Yin Y, Xia Y. A deep learning approach to segmentation of nasopharyngeal carcinoma using computed tomography. Biomed Signal Process. 2021;64:102246. doi:10.1016/j.bspc.2020.102246
- Cai M, Wang J, Yang Q, et al. Combining images and t-staging information to improve the automatic segmentation of nasopharyngeal carcinoma tumors in MR images. IEEE Access. 2021;9:21323–21331. doi:10.1109/ACCESS.2021.3056130
- Tang P, Zu C, Hong M, et al. DA-DSUnet: dual attention-based dense SU-net for automatic head-and-neck tumor segmentation in MRI images. Neurocomputing. 2021;435:103–113. doi:10.1016/j.neucom.2020.12.085
- Zhang L, Wu X, Liu J, et al. MRI-based deep-learning model for distant metastasis-free survival in locoregionally advanced Nasopharyngeal carcinoma. J Magn Reson Imaging. 2021;53(1):167–178. doi:10.1002/jmri.27308
- Wu X, Dong D, Zhang L, et al. Exploring the predictive value of additional peritumoral regions based on deep learning and radiomics: a multicenter study. Med Phys. 2021;48(5):2374–2385. doi:10.1002/mp.14767
- Zhao L, Gong J, Xi Y, et al. MRI-based radiomics nomogram may predict the response to induction chemotherapy and survival in locally advanced nasopharyngeal carcinoma. Eur Radiol. 2020;30(1):537–546. doi:10.1007/s00330-019-06211-x
- Zhang F, Zhong L-Z, Zhao X, et al. A deep-learning-based prognostic nomogram integrating microscopic digital pathology and macroscopic magnetic resonance images in nasopharyngeal carcinoma: a multi-cohort study. Ther Adv Med Oncol. 2020;12:1758835920971416. doi:10.1177/1758835920971416
- Xie C, Du R, Ho JWK, et al. Effect of machine learning re-sampling techniques for imbalanced datasets in 18F-FDG PET-based radiomics model on prognostication performance in cohorts of head and neck cancer patients. Eur J Nucl Med Mol Imaging. 2020;47(12):2826–2835. doi:10.1007/s00259-020-04756-4
- Liu K, Xia W, Qiang M, et al. Deep learning pathological microscopic features in endemic nasopharyngeal cancer: prognostic value and protentional role for individual induction chemotherapy. Cancer Med. 2020;9(4):1298–1306. doi:10.1002/cam4.2802
- Cui C, Wang S, Zhou J, et al. Machine learning analysis of image data based on detailed MR image reports for nasopharyngeal carcinoma prognosis. Biomed Res Int. 2020;2020:8068913. doi:10.1155/2020/8068913
- Du R, Lee VH, Yuan H, et al. Radiomics model to predict early progression of nonmetastatic nasopharyngeal carcinoma after intensity modulation radiation therapy: a multicenter study. Radiology. 2019;1(4):e180075. doi:10.1148/ryai.2019180075
- Zhang B, Tian J, Dong D, et al. Radiomics features of multiparametric MRI as novel prognostic factors in advanced nasopharyngeal carcinoma. Clin Cancer Res. 2017;23(15):4259–4269. doi:10.1158/1078-0432.CCR-16-2910
- Zhang B, He X, Ouyang F, et al. Radiomic machine-learning classifiers for prognostic biomarkers of advanced nasopharyngeal carcinoma. Cancer Lett. 2017;403:21–27. doi:10.1016/j.canlet.2017.06.004
- Liu J, Mao Y, Li Z, et al. Use of texture analysis based on contrast-enhanced MRI to predict treatment response to chemoradiotherapy in nasopharyngeal carcinoma. J Magn Reson Imaging. 2016;44(2):445–455.
- Zhu W, Kan X, Calogero RA. Neural network cascade optimizes MicroRNA biomarker selection for nasopharyngeal cancer prognosis. PLoS One. 2014;9(10):e110537. doi:10.1371/journal.pone.0110537
- Wong LM, Ai QYH, Mo FKF, Poon DMC, King AD. Convolutional neural network in nasopharyngeal carcinoma: how good is automatic delineation for primary tumor on a non-contrast-enhanced fat-suppressed T2-weighted MRI? Jpn J Radiol. 2021;39(6):571–579. doi:10.1007/s11604-021-01092-x
- Xue X, Qin N, Hao X, et al. Sequential and iterative auto-segmentation of high-risk clinical target volume for radiotherapy of nasopharyngeal carcinoma in planning CT images. Front Oncol. 2020;10:1134. doi:10.3389/fonc.2020.01134
- Men K, Chen X, Zhu J, et al. Continual improvement of nasopharyngeal carcinoma segmentation with less labeling effort. Phys Med. 2020;80:347–351. doi:10.1016/j.ejmp.2020.11.005
- Wang X, Yang G, Zhang Y, et al. Automated delineation of nasopharynx gross tumor volume for nasopharyngeal carcinoma by plain CT combining contrast-enhanced CT using deep learning. J Radiat Res Appl Sci. 2020;13(1):568–577. doi:10.1080/16878507.2020.1795565
- Ke L, Deng Y, Xia W, et al. Development of a self-constrained 3D DenseNet model in automatic detection and segmentation of nasopharyngeal carcinoma using magnetic resonance images. Oral Oncol. 2020;110:104862. doi:10.1016/j.oraloncology.2020.104862
- Zhong T, Huang X, Tang F, Liang S, Deng X, Zhang Y. Boosting-based cascaded convolutional neural networks for the segmentation of CT organs-at-risk in nasopharyngeal carcinoma. Med Phys. 2019;46(12):5602–5611. doi:10.1002/mp.13825
- Lin L, Dou Q, Jin Y-M, et al. Deep learning for automated contouring of primary tumor volumes by MRI for nasopharyngeal carcinoma. Radiology. 2019;291(3):677–686. doi:10.1148/radiol.2019182012
- Liang S, Tang F, Huang X, et al. Deep-learning-based detection and segmentation of organs at risk in nasopharyngeal carcinoma computed tomographic images for radiotherapy planning. Eur Radiol. 2019;29(4):1961–1967. doi:10.1007/s00330-018-5748-9
- Li S, Xiao J, He L, Peng X, Yuan X. The tumor target segmentation of nasopharyngeal cancer in CT images based on deep learning methods. Technol Cancer Res Treat. 2019;18:1533033819884561. doi:10.1177/1533033819884561
- Mohammed MA, Abd Ghani MK, Hamed RI, Ibrahim DA, Abdullah MK. Artificial neural networks for automatic segmentation and identification of nasopharyngeal carcinoma. J Comput Sci. 2017;21:263–274.
- Men K, Chen X, Zhang Y, et al. Deep deconvolutional neural network for target segmentation of nasopharyngeal cancer in planning computed tomography images. Front Oncol. 2017;7:315. doi:10.3389/fonc.2017.00315
- Wong LM, King AD, Ai QYH, et al. Convolutional neural network for discriminating nasopharyngeal carcinoma and benign hyperplasia on MRI. Eur Radiol. 2021;31(6):3856–3863. doi:10.1007/s00330-020-07451-y
- Wen D-W, Lin L, Mao Y-P, et al. Normal tissue complication probability (NTCP) models for predicting temporal lobe injury after intensity-modulated radiotherapy in nasopharyngeal carcinoma: a large registry-based retrospective study from China. Radiother Oncol. 2021;157:99–105. doi:10.1016/j.radonc.2021.01.008
- Zhang B, Lian Z, Zhong L, et al. Machine-learning based MRI radiomics models for early detection of radiation-induced brain injury in nasopharyngeal carcinoma. BMC Cancer. 2020;20(1):502. doi:10.1186/s12885-020-06957-4
- Du D, Feng H, Lv W, et al. Machine learning methods for optimal radiomics-based differentiation between recurrence and inflammation: application to nasopharyngeal carcinoma post-therapy PET/CT images. Mol Imaging Biol. 2020;22(3):730–738. doi:10.1007/s11307-019-01411-9
- Diao S, Hou J, Yu H, et al. Computer-aided pathologic diagnosis of nasopharyngeal carcinoma based on deep learning. Am J Pathol. 2020;190(8):1691–1700. doi:10.1016/j.ajpath.2020.04.008
- Abd Ghani MK, Mohammed MA, Arunkumar N, et al. Decision-level fusion scheme for nasopharyngeal carcinoma identification using machine learning techniques. Neural Comput Appl. 2020;32(3):625–638. doi:10.1007/s00521-018-3882-6
- Mohammed MA, Abd Ghani MK, Arunkumar N, Hamed RI, Abdullah MK, Burhanuddin MA. A real time computer aided object detection of nasopharyngeal carcinoma using genetic algorithm and artificial neural network based on Haar feature fear. Future Gener Comput Syst. 2018;89:539–547. doi:10.1016/j.future.2018.07.022
- Wang Y-W, Wu C-S, Zhang G-Y, et al. Can parameters other than minimal axial diameter in MRI and PET/CT further improve diagnostic accuracy for equivocal retropharyngeal lymph nodes in nasopharyngeal carcinoma? PLoS One. 2016;11(10):e0163741–e0163741. doi:10.1371/journal.pone.0163741
- Bai P, Weng X, Quan K, et al. A knowledge-based intensity-modulated radiation therapy treatment planning technique for locally advanced nasopharyngeal carcinoma radiotherapy. Radiat Oncol. 2020;15(1):188. doi:10.1186/s13014-020-01626-z
- Liu Z, Fan J, Li M, et al. A deep learning method for prediction of three-dimensional dose distribution of helical tomotherapy. Med Phys. 2019;46(5):1972–1983. doi:10.1002/mp.13490
- Jiao S-X, Chen L-X, Zhu J-H, Wang M-L, Liu X-W. Prediction of dose-volume histograms in nasopharyngeal cancer IMRT using geometric and dosimetric information. Phys Med Biol. 2019;64(23):23NT04. doi:10.1088/1361-6560/ab50eb
- Yang X, Li X, Zhang X, Song F, Huang S, Xia Y. Segmentation of organs at risk in nasopharyngeal cancer for radiotherapy using a self-adaptive Unet network. Nan Fang Yi Ke Da Xue Xue Bao. 2020;40(11):1579–1586. doi:10.12122/j.issn.1673-4254.2020.11.07
- Chen X, Yang J, Yi J, Dai J. Quality control of VMAT planning using artificial neural network models for nasopharyngeal carcinoma. Chin J Radiol Med Prot. 2020;40(2):99–105.
- Xue X, Hao X, Shi J, Ding Y, Wei W, An H. Auto-segmentation of high-risk primary tumor gross target volume for the radiotherapy of nasopharyngeal carcinoma. J Image Graph. 2020;25(10):2151–2158.
- Rizwan I, Haque I, Neubert J. Deep learning approaches to biomedical image segmentation. Inf Med Unlocked. 2020;18:100297. doi:10.1016/j.imu.2020.100297
- Leclerc B, Cale J. Big Data. Milton, UK: Taylor & Francis Group; 2020.
- Parisi GI, Kemker R, Part JL, Kanan C, Wermter S. Continual lifelong learning with neural networks: a review. Neural Netw. 2019;113:54–71. doi:10.1016/j.neunet.2019.01.012