Abstract
In this century, the rapid development of large data storage technologies, mobile network technology, and portable medical devices makes it possible to measure, record, store, and track analysis of large amount of data in human physiological signals. Entropy is a key metric for quantifying the irregularity contained in physiological signals. In this review, we focus on how entropy changes in various physiological signals in COPD. Our review concludes that the entropy change relies on the types of physiological signals under investigation. For major physiological signals related to respiratory diseases, such as airflow, heart rate variability, and gait variability, the entropy of a patient with COPD is lower than that of a healthy person. However, in case of hormone secretion and respiratory sound, the entropy of a patient is higher than that of a healthy person. For mechanomyogram signal, the entropy increases with the increased severity of COPD. This result should give valuable guidance for the use of entropy for physiological signals measured by wearable medical device as well as for further research on entropy in COPD.
Introduction
COPD is a major threaten to public health. Its diagnosis, assessment, and treatment vary based mostly on the severity of airflow limitation. The widely used method for objective assessment of functional limitation is to characterize lung function with spirometry, including indices such as forced expiratory volume in 1 second (FEV1), forced vital capacity (FVC), and FEV1/FVC ratio. However, this spirometry cannot be used for the assessment of a patient’s continuous biological dynamics. Therefore, researchers have studied the diagnosis, assessment, and treatment of COPD by continuously measuring the dynamics of physiological parameters of COPD patients.Citation1–Citation11
In this century, the rapid development of wearable, mobile, automatic, continuous, high-throughput medical device for measuring human physiological parameters heralds a new era – high-throughput phenotyping era.Citation12–Citation14 One of the key features that can be extracted from the data obtained by the high-throughput medical device is the entropy of physiological signals. Thus, entropy studies in various major diseases including respiratory diseases are arising as an important method to analyze these continuous-monitoring data measured by noninvasive medical devices.
The irregularity of physiological signals can be represented by entropy of biological dynamics contained in the physiological signals measured by continuous-monitoring medical devices. Entropy was proposed by Clausius in 1854 and statistical entropy was proposed by Boltzmann in 1886.Citation15 The initial entropy is applied to the physics of thermodynamics and statistical physics. In 1948, ShannonCitation16,Citation17 proposed an entropy (later known as Shannon entropy) that was then largely applied in information science. The Kolmogorov-Sinai entropyCitation17 and Renyi entropy,Citation18,Citation19 which were developed on the basis of Shannon’s entropy, are widely used in the nonlinear dynamics of the physical system. In physiological dynamic system, various extended concepts of entropy (such as approximate entropy [ApEn]),Citation20 cross-approximate entropy (Cross-ApEn),Citation21 sample entropy (SampEn),Citation22 and multi-scale entropyCitation23,Citation24 have been developed to quantify various physiological signals (eg, heart rate, airflow, pressure in airway, and sound signal).
It is well known that the entropy of heart rate is normally lower in patients with cardiovascular diseases than in healthy people.Citation25,Citation26 Does a patient with COPD have a lower value of entropy in each type of physiological signals than a healthy person? If not, what pattern does the entropy of each type of physiological signals have based on all the major studies that have been conducted for entropy analysis in COPD? So far, a number of studies have been conducted to investigate the entropy of physiological signals in patients with COPD.Citation1–Citation11 Hence, we conducted a systematic review on the available literature to address these questions on the entropy of physiological signals in COPD patients.
Methods
Search strategy and inclusion criteria
We searched EMBASE, PubMed, and Google Scholar for the application of entropy in respiratory diseases until June 2017. The keywords for searching were respiratory tract diseases, respiration disorders, pulmonary disease, chronic obstructive, and entropy. Articles resulting from these searches and relevant references cited in those articles were reviewed. After removal of duplicates, a total of 239 potentially relevant articles in the initial database search were identified. Finally, 12 studies met the selection criteria for this review ().
Trials included in this review met the following criteria: 1) use of entropy to analyze physiological signals, 2) patients suffered from COPD, and 3) published articles, excluding conference abstracts because the results of conference abstracts may not be reliable as they have not gone through rigorous peer review.
Data extraction and quality evaluation
We extracted the following characteristics and results from selected studies: physiologic signals, study, location, number of subjects, age in years, gender ratio, pulmonary function, and entropy result.
We used the scoring system developed by Jadad et al to evaluate the quality of the publications.Citation27 Randomization, double blinding, and description of withdraw are considered. Possible scores range from 0 to 5. The recommendation for applying entropy to analyze the physiologic signals of COPD using the grading of evaluation system () was rated as very low, low, moderate, or high.Citation28,Citation29
Table 1 GRADE analysis: applied entropy to physiologic parameters of COPD
Entropy studies on COPD
For entropy studies on COPD, various physiological signals have been measured. These signals include heart rate variability (HRV), airflow, hormone secretion, gait variability, airway pressure, sound signal, and mechanomyogram (MMG) signal (). We should examine these physiological signals one by one in the entropy studies of COPD.
Table 2 Entropy studies for comparison between healthy people and patients with COPD
HRV
Both COPD and heart problems (specifically heart failure) have one serious symptom in common – difficulty in breathing, which has a high impact on heart activity. One common indication of heart activity is HRV which is the variation in time interval between heartbeats. HRV is also commonly referred as RR variability (where R is a point corresponding to the peak of the QRS complex of the ECG wave and RR is the interval between two successive R’s). Studies have demonstrated that COPD patients showed significant imbalances of heart autonomic regulation, with a reduction in HRV.Citation25,Citation26 Therefore, multiple studies on the entropy of HRV have been conducted in COPD patients with various degrees of severity. summarizes the studies on the entropy of HRV in the COPD patients.
Goulart et al conducted a cross-sectional study in 10 COPD patients affected by moderate to very severe disease. They recorded HRV using a polar cardio-frequency meter at rest in the sitting position (10 minutes) and during a respiratory sinus arrhythmia maneuver (RSA-M, 4 minutes).Citation1 They evaluated the HRV entropy using both ApEn and SampEn. They observed reduced entropy indicated by either entropy index during RSA-M. Mazzuco et al conducted a study on 16 sedentary males with COPD to investigate whether impairment of static lung volumes and lung diffusion capacity could be related to HRV indices in patients with moderate to severe COPD.Citation2 They used ApEn to assess irregularity of HRV and observed a significantly (p<0.05) decreased entropy of HRV in sitting RSA-M as compared to either supine standing or supine sitting position.
Borghi-Silva et al conducted a randomized controlled trial to investigate the potential effect of 6- versus 12-weeks of physical training on cardiac autonomic function and exercise capacity in COPD.Citation3 In this trial, 20 moderate to severe COPD patients were randomly assigned 10 each to either a training group or a control group. They assessed the HRV irregularity using SampEn at rest and during submaximal test. They observed significantly increased entropy in the training group after 6 weeks of physical training program as compared to its corresponding baseline. They concluded that the short-term rehabilitation for 6 weeks was beneficial to cardiac regulation in patients with COPD.
Airflow
Respiratory mechanics is known to be an important factor contributing to the genesis of the respiratory pattern. Also there are studies investigating the use of nonlinear dynamical analysis to characterize the entropy of respiratory patterns.Citation30 COPD may result in modifications in airflow pattern and its entropy.
Dames et al conducted an observational controlled study investigating the influence of airway obstruction in the entropy of airflow in COPD and its use as a marker of disease activity.Citation4 They used a bellows spirometer to perform simultaneous airflow and forced oscillation measurements in each of the following 88 subjects: 11 mild, 18 moderate, 16 severe, 14 very severe COPD patients, 13 NE subjects (ie, smoking subjects that presented a normal respiratory response to the spirometric exam), and 16 control subjects (ie, healthy individuals without a previous history of pulmonary or cardiac disease and smoking). They applied SampEn to quantify the entropy of airflow and found that the SampEn of airflow during resting breathing decreased significantly (p<0.0001) with increasing airway obstruction and is reduced in proportion to airway obstruction in COPD patients. Further using receiver operating curve analysis, they found that SampEn exhibited adequate values for diagnostic use in all COPD groups. They concluded that the entropy of airflow may serve as a novel respiratory biomarker to facilitate the diagnosis of respiratory abnormalities in children and older patients, as well as for home-based monitoring of lung diseases.
Gait variability
Gait variability is a commonly used index as aimed to quantify the natural stride-to-stride fluctuations during walking. When compared with healthy people, patients with COPD may have an increased incidence of falls and demonstrate balance deficits while walking.Citation31 Therefore, the measurement of gait variability has been used as an indicator to calculate the risk of falling. Yentes et al investigated whether changes in gait variability are present in patients with COPD as compared to healthy controls. They recruited 20 patients with COPD and 28 healthy controls in this study.Citation32 Subjects were asked to walk on a treadmill at three speeds: their self-selected pace, 120%, and 80% of their self-selected pace. Then they used SampEn to measure the regularity within the time series of step length, step time, and step width. They found that the SampEn of gait variability in COPD patients was lower than that of healthy controls while walking. This decrease in regularity has a possible association with a loss of flexible adaptations, which suggests a reasonable explanation for the increased occurrence of falls in COPD patients.
Hormone secretion
Catabolic illnesses and low physical fitness are characteristic features of COPD, likewise predicts greater responsiveness of beta-endorphin and corticotropic (ACTH), adrenal (cortisol), and adrenal medullary hormones (Epi) to an exercise stimulus. Iranmanesh et al conducted a study investigating the impact of exercise level on hormone pattern irregularity (measured by ApEn) of sympathoadrenal outflow, namely the secretion of stress-adaptive noradrenergic hormone, Epi, ACTH, and cortisol.Citation5 This study involved 8 healthy men and 9 COPD male patients. Cross-ApEn quantifies the relative synchrony (joint regularity) of subpatterns in paired time series.Citation33,Citation34 They found that the entropy of hormone secretion in COPD patients was higher than that in healthy men during maximal exercise. Higher ApEn defines greater irregularity of hormone secretion pattern. It was also found that exercise benefits the regularity of the patterns (lower ApEn) of ACTH, cortisol, Epi, and noradrenergic hormone release.
Therefore, men with stable COPD fail to achieve normal exercise-induced corticotropic axis and adrenomedullary outflow.
Airway pressure
Rabarimanantsoa et al and Letellier et al conducted a study for the evaluation of patient-ventilator interactions during noninvasive ventilation.Citation6,Citation35 They applied pressure support noninvasive ventilation to 4 COPD patients, 4 obesity hypoventilation syndrome patients in stable state, and 4 healthy subjects during six successive 10-minute periods with various inspiratory pressure and recorded airflow and airway pressure using sensors located near the mask. They applied Shannon entropy to airway pressure and the total duration of the respiratory cycle. If these two entropies are <1, then it means that the quality of patient-ventilator interactions during noninvasive ventilation was high. They found that the Shannon entropy can evaluate patient-ventilator interactions objectively during noninvasive ventilation. Because airway pressure is a reflection of the interaction between a patient and a ventilator, not just a physiological signal of a patient, this study did not illustrate the difference in entropy between COPD patients and healthy people.
Respiratory sound
Sound signals produced by the airflow during inspiration and expiration can now be used to detect potential pulmonary dysfunctions. There are some entropy analysis methods proposed which showed valuable potential in the evaluation and discrimination of the complexity of respiratory sound signals in COPD patients. The respiratory sounds can be classified as breath sounds, abnormal breath sounds, and adventitious sounds.Citation36 Adventitious sounds are abnormal sounds that can indicate some type of respiratory disorders. These sounds include wheezes (continuous sounds), stridors, squawks, rhonchi, and crackles (discontinuous sounds). Wheezes are characterized by periodic waveforms with a dominant frequency (>100 Hz) and with a duration (>100 ms). Based on their total duration, crackles are commonly being classified as fine (<10 ms) or coarse (>10 ms).
Mendes et al tested 7 different features to identify the best subset of features that allows a robust detection of coarse crackles.Citation7 One of the features is local entropy, which is essentially Shannon entropy. For this entropy, respiratory sound signals are quantized into 6 levels first. Then the Shannon entropy is calculated based on the frequency of sound signals in each of the 6 levels. Mendes et al calculated the features including Shannon entropy in two datasets (ie, the first channel of the repository “Crackle a” and the repository “Crackle c”) available online. This is the expected type of crackles to be found in patients with COPD. They found that the Shannon entropy tends to be higher in presence of crackles. They also calculated the quartile for different processing algorithms to improve the robustness against outliers and to improve the performance of the detection of crackles. Shannon entropy was the best individual feature.
Mondal et al proposed an automatic lung status detection algorithm based on SampEn approach. This method consists of two approaches: Hilbert transformation and SampEn.Citation8 They used SampEn to value the frequency spectrum of lung sound. The lung sound recordings included normal and abnormal lungs conditions from 8 subjects. These pathological problems contained COPD, asthma, and interstitial lung disease (ILD). The SampEn in abnormal subjects was higher than that in normal subjects.Citation37 Then they conducted another study and extracted sound signals from 10 normal and 20 abnormal subjects to analyze. The SampEn index for abnormal subjects was found to be higher for normal cases due to the unstable condition of the respiratory system associated with the disease severity. They found that SampEn of respiratory sounds can reflect the pulmonary status. The authors suggested that this algorithm could be used as a diagnostic tool for its capability to assist the physicians in prognosis of the lung status of COPD patients.
Aydore et al developed a method to classify the wheeze and non-wheeze epochs within respiratory sound signals.Citation9 They chose four features for classification: kurtosis, Renyi entropy, f50/f90 ratio, and mean-crossing irregularity. The data used in this study were taken from the data of COPD and asthma patients, which were recorded by the 14-channel respiratory sound data acquisition system. Data obtained from 4 male and 3 female subjects at the age of 50±17 were used. The results showed that the Renyi entropy was higher in presence of wheezes.
Respiratory muscle MMG
Respiratory muscle dysfunction is a common problem in COPD patients. The MMG of respiratory muscles is a promising noninvasive technique to evaluate the respiratory muscular effort. MMG quantifies the low-frequency lateral oscillations of the muscle fibers during contraction. The time and frequency domain of the MMG signal can be used to assess a patient’s respiratory muscle function.Citation10,Citation11,Citation38–Citation40
Torres et al conducted a study to assess the respiratory muscular function in COPD patients.Citation10 The MMG signals from left and right hemidiaphragm were acquired using two capacitive accelerometers placed on both left and right sides of the costal wall surface in 6 patients with severe COPD while these patients carried out an inspiratory load respiratory test. The maximum inspiratory pressure (IPmax) and diaphragm MMG parameters were analyzed in 6 patients with severe COPD. Renyi entropy was used to quantify the amplitude change in the MMG signal. They found a positive correlation between the IPmax developed in a respiratory cycle and Renyi entropy with alpha =0.5 (left side: 0.73±0.11 and right side: 0.77±0.08). It was also found that the Renyi entropy of the MMG signal amplitude also increased when the inspiratory pressure increased.
In their previous works, Torres et al studied animal models (dogs) and found a positive correlation between amplitude parameters of the diaphragmatic MMG signal and the respiratory effort. They calculated the Shannon entropy of the MMG signal during the diaphragm muscle voluntary contraction.Citation41 Then they used Renyi entropy to analyze the spatiotemporal patterns of the MMG signal.Citation42 Their results showed an increase in Renyi entropy of the MMG signal with an increase in the respiratory effort. They demonstrated that Renyi entropy shows better performance than Shannon entropy and other metrics in all the MMG signals analyzed.
Sarlabous et al quantified the amplitude variations in biomedical signals by means of moving ApEn with fixed tolerance values.Citation43 Their MMG signals were acquired in animal models (dogs). The results showed that the information provided by MMG signals could be used to evaluate the respiratory effort and muscular efficiency in COPD patients. Then they conducted a study to noninvasively evaluate the mechanical activation of inspiratory muscles during tidal volume breathing in patients with severe to very severe COPD.Citation39 They investigated the IPmax and respiratory muscle MMG of 5 severe and 5 very severe COPD patients under both quiet breathing and maximal voluntary ventilation conditions.Citation38 They used moving SampEn with fixed tolerance values – fixed sample entropy (fSampEn) to estimate the respiratory muscle effort from MMG recordings. Then they calculated fSampEn for each inspiratory cycle of the averaged MMG. They found that the average fSampEn in very severe COPD patients was significantly higher (p<0.05) than that of severe COPD patients under either quiet breathing or maximal voluntary ventilation condition.
Through our review on the entropy studies, we obtained the following general results: regarding HRV, the entropy decreases during respiratory sinus arrhythmias; regarding airflow and gait variability, the entropy in a patient is lower than that in a healthy person; regarding hormone secretion, the entropy in COPD patients is higher than that in healthy people during maximal exercise; regarding sound signal, the entropy is higher in abnormal subjects; and regarding MMG signal, the entropy increases as the severity of the disease increases. These general results regarding HRV, airflow, and MMG are illustrated in .
Figure 2 Entropy change in COPD.
Abbreviations: HRV, heart rate variability; MMG, mechanomyogram signal; RSA-M, respiratory sinus arrhythmia maneuver.
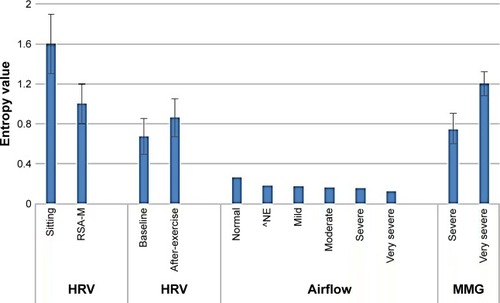
Discussion
With the rapid development of wearable, mobile, automatic, continuous, high-throughput medical device, continuous monitoring of physiological signals is becoming more and more important in the diagnosis and treatment of COPD.Citation12,Citation13 One of the keys in analyzing continuous-monitoring data is the entropy of physiological signals. Consequently, a number of studies have been conducted to investigate the entropy of physiological signals in patients with COPD. However, so far as we know, no one has published a sophisticated review specifically on the entropy change of COPD. To look for the pattern in the entropy change in COPD, we conducted a systematic review on the available literature.
The results from our review show that the pattern of entropy change in COPD depends on the physiological signals, which is summarized as follows. In case of COPD, in each of three physiological signals – HRV, airflow, and gait variability – the entropy in a patient is lower than that in a healthy person; it is lower in more severe patients; and exercise increases entropy. By contrast, in case of hormone secretion, the entropy in a patient is higher than that in a healthy person during maximal exercise; in case of respiratory sound, the entropy is higher in COPD patients with abnormal sound signals. In case of MMG, the entropy is also higher in more severe patients or in those with increased inspiratory pressure ().
These results can be effectively explained based on Goldberger hypothesis which states that increased regularity (ie, low entropy) of signals represents a “decomplexification” that is characteristic of illness.Citation44 Through our review, we note that in case of COPD, in each of three important physiological signals – airflow, HRV, and gait variability – the entropy in a patient is lower than in a healthy person and it is lower in a more severe patient.
The higher entropy of hormone secretion in a COPD patient during maximal exercise could be explained by the selective attenuation of pulsatile hormone secretion and failure to regularize ACTH secretion patterns. The impact of catabolic illness in COPD on corticotropin secretion is important (many studies have shown cross interaction between hormone secretion and catabolic disorders). And this pathway is impaired in patients with COPD.Citation5
It should be noted that Goldberger’s hypothesis could not explain few other physiological signals such as MMG and respiratory sound. In case of MMG signal, irrespective of the type of entropy calculation used (eg, Renyi entropy, Shannon entropy, ApEn, and SampEn), the entropy increases as the severity of COPD increases.Citation10,Citation38,Citation39 This may be explained by the fact that following the exacerbation of airflow obstruction, greater mechanical activation of respiratory muscle is required.Citation40 In case of respiratory sound, higher entropy in abnormal subject may be due to the fact that the sound signals of patients with wheeze or crackle are irregular or more complex in nature. These abnormal sound signals are produced by the unstable condition of the respiratory system associated with disease severity.Citation8
It is worth to mention that the analysis of MMG signal could be a useful alternative approach for assessing the function of respiratory muscles in patients with COPD.Citation41,Citation42 Respiratory muscle MMG reflects the mechanical counterpart of the neural activity measured by electromyography. The amplitude of the MMG signal is usually estimated by average rectified value (ARV) or root mean square (RMS). In these studies, they found that entropy analysis is robust against cardiac vibration interference. Although MMG vibrations are random in nature, the entropy is less influenced by cardiac vibrations than ARV and parameters. These are the advantages for the use of entropy as compared to ARV and RMS.
Currently, entropy analysis using entropy for discriminating the disease progression of COPD patients had not been broadly utilized according to the available literature. As shown in our review, despite the availability of various newly proposed entropy measures, none of them have been broadly accepted for classifying COPD-related physiological signals. Sample entropy, which has the biggest clinical potential to be a novel index in COPD, is gaining increasing popularity among all the other available entropy measures. However, the difficulty with sample entropy analysis lies in the requirement of multiple parameters adjustment such as tolerance and embedding dimension, because different results might be produced from different settings of the parameters. Therefore, entropy alone is inadequate to be used as a credible clinical analysis tool at present. Besides, our review shows that, to optimize the analysis result, for different physiological signals, there should be different entropy measures. Thus, the most practical way at present is to explore the comprehensive pattern of entropy change in different disease statuses as well as to compare the cons and pros of all proposed entropy measurements. It is hoped that in the future more stable entropy measures would be developed with broader clinical usage in various COPD-related physiological signals.
Conclusion
The summarized result obtained through our review should give valuable guidance for further research on the entropy in COPD and provide basis for the use of entropy for physiological signals measured by wearable medical device.
Acknowledgments
This work was supported by the Start-up Research Grant (SRG2016-00083-FHS) at the University of Macau.
Disclosure
The authors report no conflicts of interest in this work.
References
- Goulart CdaLSimonJCSchneiders PdeBRespiratory muscle strength effect on linear and nonlinear heart rate variability parameters in COPD patientsInt J Chron Obstruct Pulmon Dis2016111671167727555757
- MazzucoAMedeirosWMSperlingMPRelationship between linear and nonlinear dynamics of heart rate and impairment of lung function in COPD patientsInt J Chron Obstruct Pulmon Dis2015101651166126316739
- Borghi-SilvaAMendesRGTrimerRPotential effect of 6 versus 12-weeks of physical training on cardiac autonomic function and exercise capacity in chronic obstructive pulmonary diseaseEur J Phys Rehabil Med201551221122124594853
- DamesKKLopesAJde MeloPLAirflow pattern complexity during resting breathing in patients with COPD: effect of airway obstructionRespir Physiol Neurobiol2014192394724334010
- IranmaneshARochesterDFLiuJVeldhuisJDImpaired adrenergic-and corticotropic-axis outflow during exercise in chronic obstructive pulmonary diseaseMetabolism201160111521152921632072
- RabarimanantsoaHAchourLLetellierCMuirJFCuvelierAObjective evaluation of patient-ventilator interactions during noninvasive ventilation (NIV)Eur Respir Rev2008171072223
- MendesLCarvalhoPTeixeiraCAPaivaRPHenriquesJRobust features for detection of crackles: an exploratory studyConf Proc IEEE Eng Med Biol Soc201420141473147625570247
- MondalABhattacharyaPSahaGDetection of lungs status using morphological complexities of respiratory soundsSci World J20142014182938
- AydoreSSenIKahyaYPMihcakMClassification of respiratory signals by linear analysisConf Proc IEEE Eng Med Biol Soc200920092617262019965225
- TorresASarlabousLFizJANoninvasive measurement of inspiratory muscle performance by means of diaphragm muscle mechanomyographic signals in COPD patients during an incremental load respiratory testConf Proc IEEE Eng Med Biol Soc201020102493249621096168
- SarlabousLTorresAFizJAJaneREvidence towards improved estimation of respiratory muscle effort from diaphragm mechanomyographic signals with cardiac vibration interference using sample entropy with fixed tolerance valuesPLoS One201492e8890224586436
- KvedarJCFogelALElenkoEZoharDDigital medicine’s march on chronic diseaseNat Biotechnol201634323924626963544
- ElenkoEUnderwoodLZoharDDefining digital medicineNat Biotechnol201533545646125965750
- BarangerMChaos, Complexity, and EntropyCambridgeNew England Complex Systems Institute2000
- ChakrabartiCGDeKBoltzmann entropy: generalization and applicationsJ Biol Phys199723316317023345658
- ShannonCECommunication theory of secrecy systems. 1945MD Comput199815157649458664
- GrassbergerPProcacciaIEstimation of the Kolmogorov entropy from a chaotic signalPhys Rev A198328425912593
- Gonzalez AndinoSLGrave de Peralta MenendezRThutGMeasuring the complexity of time series: an application to neuro-physiological signalsHum Brain Mapp2000111465710997852
- SlomczynskiWKwapienJZyczkowskiKEntropy computing via integration over fractal measuresChaos200010118018812779373
- PincusSMApproximate entropy as a measure of system complexityProc Natl Acad Sci U S A19918862297230111607165
- PincusSSingerBHRandomness and degrees of irregularityProc Natl Acad Sci U S A19969352083208811607637
- RichmanJSMoormanJRPhysiological time-series analysis using approximate entropy and sample entropyAm J Physiol Heart Circ Physiol20002786H2039H204910843903
- CostaMDGoldbergerALGeneralized multiscale entropy analysis: application to quantifying the complex volatility of human heartbeat time seriesEntropy (Basel)20151731197120327099455
- CostaMGoldbergerALPengCKMultiscale entropy analysis of biological signalsPhys Rev E2005712 Pt 1021906
- VolterraniMScalviniSMazzueroGDecreased heart rate variability in patients with chronic obstructive pulmonary diseaseChest19941065143214377956396
- CorboGMInchingoloRSguegliaGALanzaGValenteSC-reactive protein, lung hyperinflation and heart rate variability in chronic obstructive pulmonary disease – a pilot studyCOPD201310220020722946790
- JadadARMooreRACarrollDAssessing the quality of reports of randomized clinical trials: is blinding necessary?Control Clin Trials19961711128721797
- BalshemHHelfandMSchünemannHJGRADE guidelines: 3. Rating the quality of evidenceJ Clin Epidemiol201164440140621208779
- AtkinsDEcclesMFlottorpSSystems for grading the quality of evidence and the strength of recommendations I: critical appraisal of existing approaches The GRADE Working GroupBMC Health Serv Res200443815615589
- El-KhatibMFA diagnostic software tool for determination of complexity in respiratory pattern parametersComput Biol Med200737101522152717403521
- YentesJMRennardSIBlankeDStergiouNPatients with COPD walk with a more periodic step width pattern as compared to healthy controlsAm J Respir Crit Care Med2014189A2643
- YentesJMRennardSISchmidKKBlankeDStergiouNPatients with chronic obstructive pulmonary disease walk with altered step time and step width variability as compared with healthy control subjectsAnn Am Thorac Soc201714685886628267374
- LiuPYPincusSMKeenanDMRoelfsemaFVeldhuisJDAnalysis of bidirectional pattern synchrony of concentration-secretion pairs: implementation in the human testicular and adrenal axesAm J Physiol Regul Integr Comp Physiol20052882R440R44615486096
- VeldhuisJDKeenanDMPincusSMMotivations and methods for analyzing pulsatile hormone secretionEndocr Rev200829782386418940916
- LetellierCRabarimanantsoaHAchourLCuvelierAMuirJFRecurrence plots for dynamical analysis of non-invasive mechanical ventilationPhilos Trans A Math Phys Eng Sci2008366186562163417698467
- SovijarviACharacteristics of breath sounds and adventitious respiratory soundsEur Respir Rev200010591596
- MondalABhattacharyatPSahaGDiagnosing of the lungs status using morphological anomalies of the signals in transformed domainPaper presented at: Intelligent Human Computer Interaction (IHCI), 4th International Conference27–29 December; 2012Kharagpur, India
- SarlabousLTorresAFizJAGeaJMartinez-LlorensJMJaneREfficiency of mechanical activation of inspiratory muscles in COPD using sample entropyEur Respir J20154661808181126493808
- SarlabousLTorresAFizJAGeaJMartinez-LlorensJMJaneREvaluation of the respiratory muscular function by means of diaphragmatic mechanomyographic signals in COPD patientsConf Proc IEEE Eng Med Biol Soc200920093925392819964322
- TorresASarlabousLFizJANoninvasive measurement of inspiratory muscle performance by means of diaphragm muscle mechanomyographic signals in COPD patients during an incremental load respiratory testConf Proc IEEE Eng Med Biol Soc201020102493249621096168
- TorresAFizJAGaldizJBGeaJMoreraJJaneRInspiratory pressure evaluation by means of the entropy of respiratory mechanomyographic signalsConf Proc IEEE Eng Med Biol Soc200615735573817947166
- TorresAFizJAJaneRRenyi entropy and Lempel-Ziv complexity of mechanomyographic recordings of diaphragm muscle as indexes of respiratory effortConf Proc IEEE Eng Med Biol Soc200820082112211519163113
- SarlabousLTorresAFizJAInterpretation of the approximate entropy using fixed tolerance values as a measure of amplitude variations in biomedical signalsConf Proc IEEE Eng Med Biol Soc201020105967597021096950
- GoldbergerALFractal variability versus pathologic periodicity: complexity loss and stereotypy in diseasePerspect Biol Med19974045435619269744