Abstract
Background
Obesity is a public health problem all over the world, and dietary habits are considered one of the important reasons.
Methods
In this study, serum metabolites of mice fed a normal or high-fat diet (HFD) were analyzed using UPLC-QTOF-MS.
Results
A significant increase in body weight was noted in HFD mice. The HFD and control groups were significantly different from each other on OPLS-DA scores. The major metabolites contributing to obesity were lipid metabolites (phosphatidylcholines, phosphatidylethanolamine, and lysophosphatidylcholines). In addition, this study revealed that glycerophospholipid metabolism, α-linolenic acid metabolism, and linoleic acid metabolism were related to obesity and obesity-associated diseases.
Conclusion
These results can be used to better understand obesity and assess its risk, which will provide new ideas for treatment.
Introduction
Obesity is a strong risk factor of other metabolic diseases, including insulin resistance (IR), hypertension, fatty-liver disease, cardiovascular disease, and type 2 diabetes;Citation1,Citation2 therefore, it is becoming the most important public health problem across the world.Citation3 The causes of obesity are principally genetic and environmental contributions to a deregulation of balance between regular energy intake and energy expenditure.Citation4–Citation6 Animal experiments have shown acute inflammation and IR after 3 days on a high-fat diet (HFD), and lipid overload and lipotoxicity in white adipose tissue and muscle were observed at week 12.Citation7,Citation8 Much evidence has shown HFD-induced obesity alters the structure of gut microbiota and increases the ratio of Firmicutes to Bacteroidetes,Citation9 while the metabolic profile of liver, serum, urine are changed by obesity.Citation10 Therefore, HFD consumption may cause obesity and related diseases, including glucose intolerance, fat deposition in diverse tissue, and gut microbial dysbiosis.
Metabolomics is a comprehensive approach involving metabolites in systems biology,Citation11 and has been widely applied in pathophysiological pathway investigations, biomarker identification, and therapeutic response monitoring.Citation12,Citation13 Studies using LC-MS and GC-MS have identified metabolic characteristics associated with obesity, including branched-chain amino acids, glycerol, lysophosphatidylcholines, and glycerophosphocholines.Citation14–Citation17 Metabolomics may provide new insights into the molecular mechanisms of HFD-induced obesity, and identification of more metabolites related to obesity is necessary to further our understanding of obesity metabolism. In this study, we detected the metabolic profiling of serum from HFD-induced obese mice using UPLC-QTOF-MS, and the results were analyzed with Progenesis QI software and the MetaboAnalyst 5.0 platform to find metabolites and metabolic pathways that contribute to obesity, which will help researchers understand obesity and related diseases induced by HFD feeding, and give practicable therapeutic advice.
Methods
Chemicals and Reagents
HPLC-grade acetonitrile was obtained from Thermo Fisher Scientific (CAS A955-4, batch 135867). Formic acid and sodium hydroxide was purchased from Sigma-Aldrich (St. Louis, MO, USA). Ultrapure water was produced by a Milli-Q water-purification system (Millipore, Bedford, MA, USA).
Animals and Experimental Design
Five-week-old male C57BL/6J mice were obtained from Vital River Laboratories (Beijing, China). All animals were acclimatized under laboratory conditions at 22°C and 50% relative humidity and a 12/12-hour light/dark cycle for 1 week before experiments. After acclimation, ten mice were fed a standard chow diet and ten an HFD (D12492, 60% kcal, Research Diets, New Brunswick, NJ, USA). Body weight was recorded weekly for 10 weeks. At the end of the study, mice were anesthetized with diethyl ether, blood sampled from the portal and cava veins and centrifuged at 4,000 rpm for 15 minutes at 4°C, then sera were frozen at −80°C. After exsanguination, mice were euthanized by cervical dislocation. Subcutaneous adipose-tissue deposits, kidneys, and livers were precisely dissected, weighed, and stored at −80°C for further analysis. All animal experiments were approved by the Ethics Committee of Beijing Shijitan Hospital, Capital Medical University (permission sjtkyll-lx-2021Citation4), and performed in accordance with the Reporting of In Vivo Experiments guidelines and European Union Directive 2010/63/EU for animal experiments. All efforts were made to minimize animal suffering.
Biochemical Analyses
Levels of serum glucose, serum insulin, triglycerides, and total cholesterol were measured as previously described.Citation25 The homeostasis model assessment of IR (HOMA-IR) formula used was HOMA-IR = serum glucose levels (mmol/L) × insulin levels (mU/L)/22.5.
Sample Preparation
Serum samples were slowly dissolved at room temperature. Cold acetonitrile–methanol (4:1 v:v, 200 μL) was added to 50 μL sample to precipitate proteins. Subsequently, serum samples were vortexed for 1 minute after being on ice for 10 minutes. After centrifugation at 12,000 rpm for 20 minutes at 4°C, the supernatant was obtained for UPLC-Q-TOF analysis.
HPLC-QTOF/MS Analysis
Chromatographic analysis was performed using UPLC with an Acquity UPLC HSS T3 C18 column (2.1×100 mm, 1.7 μm). The mobile phase A consisted of 0.1% formic acid in H2O, and mobile phase B was 0.1% formic acid in ACN. The gradient used was: 1% B, 0–1 minute; 1%–40% B, 1–4 minutes; 40%–60% B, 4–7 minutes; 60%–75% B, 7–12 minutes; 75%–99% B, 12–14 minutes; 99% B, 14–16 minutes; 99%–1% B, 16–18 minutes. The column temperature was 30°C, flow rate 0.4 mL/min, and volume of injection 2 μL for each run. Metabolomic profiling analysis was performed on a Xevo G2-XS Q-TOF mass system and the MassLynx V14.1 workstation (Waters, Milford, MA, USA). Source-parameter settings in the positive or negative modes were as follows. The capillary voltage was set at 3 kV for positive mode and 2.5 kV for negative mode, sampling-cone voltage was set at 40 V, desolation-gas low rate was maintained at 600 L/h with cone-gas flow rate of 50 L/h, desolation temperature was set at 350°C for positive mode and 500°C for negative mode, source temperature was 100°C, and the TOF/MS full-scan range was 50–1,000 Da. To ensure precise and stable scanning, leucine encephalin (LE) was used as a lock-spray reference (m/z 556.2771 for positive mode and m/z 554.2615 for negative mode).
Metabolomic Data Processing and Metabolite Identification
The raw data were imported to Progenesis QI (Waters) for processing. The converted files were calculated for generation of alignment, peak picking, deconvolution, filter data, and identifying compounds. For identification of potential biomarkers, several online databases, such as the HMDB (http://www.hmdb.ca) were selected for metabolite identification based on exact mass measurement (mass error <10 ppm) obtained from UPLC-QTOF/MS. Other parameter settings were designed as defaults for data processing automatically. Pareto-scaling data sets were analyzed using multivariate statistical analysis on the MetaboAnalyst 5.0 platform (https://www.metaboanalyst.ca), including partial least square–discrimination analysis (PLS-DA), principal-component analysis (PCA), pathway analysis, heat map for hierarchical clustering analysis (HCA), and variable importance in projection (VIP) among the relative levels of significant metabolites.
Results
Parameters of Obesity Induced by High-Fat Diet in Mice
The body weight and the food accumulation of mice fed a normal diet or HFD are shown in –. In comparison with the control group, body weight in HFD mice had significantly increased after 2 weeks (). By the end of treatment, significant increases in LEE-index and kidney-index values were noted in HFD mice ( and ). However, there was no significant difference in food intake of the two groups or liver-index values ( ). Concerning glycolipid metabolism, mice fed the HFD showed significantly increased serum cholesterol, triacylglycerides, and HOMA-IR ( ).
Figure 1 Characteristics of mice fed a normal or high-fat diet: (A) body weight; (B) LEE index; (C) food accumulation; (D) liver and kidney index; (E) TC and TGs; (F) HOMA-IR. Data presented as means ± SEM. Ten mice per group. Statistical analysis was performed using one-way ANOVA. *P<0.05; **P<0.01; ***P<0.001.
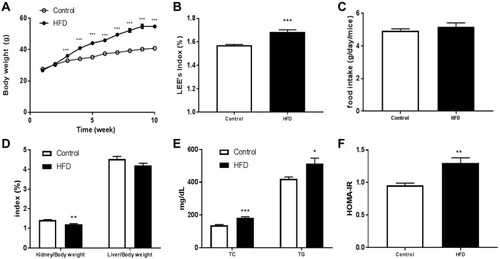
Analytical Assessment of Metabolomics
Total-ion chromatograms in positive- and negative-ion modes are shown in . One QC sample was inserted after every ten samples. The relative SD (RSD) of positive/negative ions in QC samples was calculated to evaluate the method’s stability. As shown in and , although 42.93% and 30.67% of ions displayed an RSD threshold of 10% in positive and negative models, and 79.66% and 81.57% of ions displayed an RSD threshold of 30%, which indicated that the UPLC-qTOF-MS system had relatively high reproducibility and stability.
Potential Serum Biomarkers Associated with HFD-Induced Obesity in Mice
Data processing for the groups was conducted using Progenesis QI software, and 1,862 and 2,495 ions were detected in the positive and negative models, respectively. OrthoPLS-DA models were constructed using these data on the MetaboAnalyst 5.0 platform, and a clear separation was observed between the control and HFD groups, which indicated that the OrthoPLS-DA models were reliable ( and ). In the volcano plot, 153 ion features in the positive model and 279 in the negative model met the criteria of P<0.05 and fold changes >1.5. After filtering out fragment and adduct ions, 134 of these metabolites were identified as differential ( and ). In , wherein HCA of the sample (top of the heat map) and the feature (left of the heat map) are displayed, we can observe metabolite patterns in these two groups were clearly clustered into two model types, which were also in agreement with OPLS-DA results.
Figure 4 PLS-DA score plots between the control group and HFD group in the positive (A) and negative (B) models; (C) VIP scores representing the most contributing metabolites involved in separation between controls and HFD-induced group.
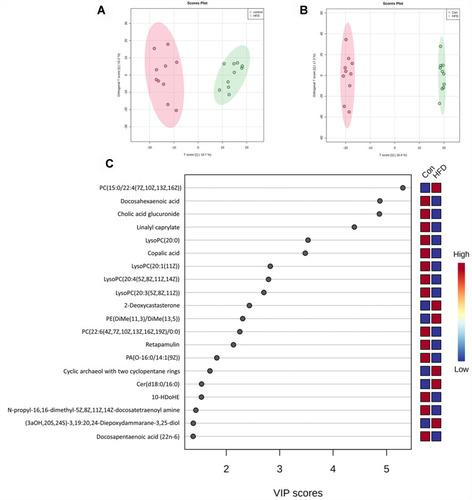
Figure 5 Metabolic characteristics of serum in HFD mice. (A) Volcano plot of Con vs HFD groups in positive (A) and negative (B) models; (C) heat map of normalized metabolites in serum samples. Columns represent the samples (Con and HFD groups), and rows represent the metabolites.
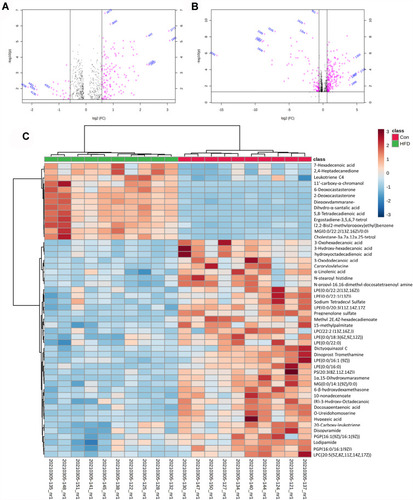
With the help of Progenesis QI and the HMDB database, 35 metabolites were identified with VIP >1 (), and 22 metabolites, including LPCs (LysoPC [20:1], LysoPC [20:4], LysoPC [20:3]), PC (22:6/0:0), and LysoPE (0:0/18:1 [11Z]), were significantly lower in the HFD group, while the other 13 metabolites, including PC (15:0/22:4 [7Z, 10Z, 13Z, and 16Z]) and PE (DiMe [11, 3]/DiMe [13,5]), were significantly higher than the control group. For pathway analysis, matched metabolic pathways were displayed based on P-values and pathway-impact values on hypergeometric tests and out-degree centrality. There were five pathways with an impact value >0.1 (): glycerophospholipid metabolism, α-linolenic acid metabolism, linoleic acid metabolism, sphingolipid metabolism, and glycosylphosphatidylinositol-anchor biosynthesis, among which glycosylphosphatidylinositol-anchor biosynthesis and sphingolipid metabolism had the most hits: four and three, respectively.
Discussion
Due to changes in lifestyles, such as high-fat/calorie diet and lack of physical activity, obesity has become an important threat to human health recently. We observed short-term HFD treatment in mice led to weight gain and dyslipidemia, and later IR was also observed. Meanwhile, we investigated serum nontargeted metabolomics of HFD-induced obese mice using UPLC-QTOF-MS, and their metabolic profiling were compared with normal mice by multivariate statistical analysis. One of the most differential identifiable markers was PC (15:0/22:4 [7Z, 10Z, 13Z, and 16Z]). PC is the most component for hepatic very low–density lipoprotein secretion, and in a physiological state, it will transform to triglycerides. PE (DiMe [11, 3]/DiMe [13, 5]) was also significantly higher in HFD mice, related to energy intake and inflammatory. These findings were in accord with data from lipidomic analyses of plasma from healthy overweight and obese individuals.Citation18,Citation19 On pathway analysis, it was found that glycerophospholipid metabolism changed significantly. Glycerophospholipids are the most abundant phospholipids and a source of physiologically active compounds participating in the regulation of many cellular processes.
Linoleic acid and α-linolenic acid are polyunsaturated fatty acids and associated with significantly lower diabetes risk.Citation20 Conjugated linoleic acid has been considered to have a good antiobesity effect.Citation21,Citation22 In our study, there were significant differences in α-linolenic acid metabolism and linoleic acid metabolism between the groups, and combined with the results of differential metabolite analysis, we found that linoleic acid was higher in the sera of normal mice. Previous studies have shown sphingolipid metabolism is involved in IR, which suggests inhibition of ceramide synthesis could markedly improve glucose tolerance and prevent the onset of diabetes in obese mice.Citation23 Hammerschmidt et al showed that ceramide (C16:0) can inhibit β-oxidation reactions of mitochondria in liver and brown adipose tissue and that MFF (mitochondrial fission factor) and CerS6 specifically regulate fatty acid–induced mitochondrial fragmentation, thereby improving high fat–induced obesity.Citation24
In conclusion, a nontargeted UPLC-qTOF-MS serum metabolomic method was applied to investigate the metabolic characteristics of obese mice. We observed many important metabolites, mainly related to glycerophospholipid metabolism, α-linolenic acid metabolism, and linoleic acid metabolism. Our study will contribute to better understanding of the mechanism of obesity induced by an HFD.
Disclosure
The authors declare no competing interests.
Additional information
Funding
References
- Petrie JR , Guzik TJ , Touyz RM . Diabetes, hypertension, and cardiovascular disease: clinical insights and vascular mechanisms. Can J Cardiol . 2018;34:575–584. doi:10.1016/j.cjca.2017.12.005 29459239
- Kaur J . A Comprehensive review on metabolic syndrome. Cardiol Res Pract . 2014;2014:943162. doi:10.1155/2014/943162 24711954
- Chen JQ , Brown TR , Russo J . Regulation of energy metabolism pathways by estrogens and estrogenic chemicals and potential implications in obesity associated with increased exposure to endocrine disruptors. Biochimi Biophys Acta . 2009;1793:1128–1143. doi:10.1016/j.bbamcr.2009.03.009
- David LA , Maurice CF , Carmody RN , et al. Diet rapidly and reproducibly alters the human gut microbiome. Nature . 2014;505:559–563. doi:10.1038/nature12820 24336217
- Carmody RN , Gerber GK , Luevano JM , et al. Diet dominates host genotype in shaping the murine gut microbiota. Cell Host Microbe . 2015;17:72–84. doi:10.1016/j.chom.2014.11.010 25532804
- Yatsunenko T , Rey FE , Manary MJ , et al. Human gut microbiome viewed across age and geography. Nature . 2012;486:222–227. doi:10.1038/nature11053 22699611
- Williams LM , Campbell FM , Drew JE , et al. The development of diet-induced obesity and glucose intolerance in C57Bl/6 mice on a high-Fat diet consists of distinct phases. PlosOne . 2014;9(8):e106159. doi:10.1371/journal.pone.0106159
- Parikh D , Riascos-Bernal DF , Egana-Gorrono L , et al. Allograft inflammatory factor-1-like is not essential for age dependent weight gain or HFD-induced obesity and glucose insensitivity. Sci Rep . 2020;10:3594. doi:10.1038/s41598-020-60433-4 32107417
- Ley RE , Bäckhed F , Turnbaugh P , et al. Obesity alters gut microbial ecology. Proc Natl Acad Sci USA . 2005;102:11070–11075. doi:10.1073/pnas.0504978102 16033867
- Kim HJ , Kim JH , Noh S , et al. Metabolomic analysis of livers and serum from high-fat diet induced obese mice. J Proteome Res . 2011;10:722–731. doi:10.1021/pr100892r 21047143
- Wishart DS . Emerging applications of metabolomics in drug discovery and precision medicine. Nat Rev Drug Discov . 2016;15:473–484. doi:10.1038/nrd.2016.32 26965202
- Wang TJ , Larson MG , Vansan RS . Metabolite profiles and the risk of developing diabetes. Nat Med . 2011;17:448–453. doi:10.1038/nm.2307 21423183
- Newgard CB . Interplay between lipids and branched chain amino acids in development of insulin resistance. Cell Metab . 2012;15:606–614. doi:10.1016/j.cmet.2012.01.024 22560213
- Butte NF , Liu Y , Zakeri IF , et al. Global metabolomics profiling targeting childhood obesity in the Hispanic population. Am J Clin Nutr . 2015;102:256–267. doi:10.3945/ajcn.115.111872 26085512
- Chen HH , Tseng YJ , Wang SY , et al. The metabolome profiling and pathway analysis in metabolic healthy and abnormal obesity. Int J Obes . 2015;39:1241–1248. doi:10.1038/ijo.2015.65
- Ho JE , Larson MGM , Ghorbani A , et al. Metabolomic profiles of body mass index in the Framingham Heart Study reveal distinct cardiometabolic phenotypes. PLoS One . 2016;11:e0148361. doi:10.1371/journal.pone.0148361 26863521
- Piening BD , Zhou W , Contrepois K , et al. Integrative personal omics profiles during periods of weight gain and loss. Cell Syst . 2018;6:157–170. doi:10.1016/j.cels.2017.12.013 29361466
- Holčapek M , Ovčačíková M , Lísa M , et al. Continuous comprehensive two-dimensional liquid chromatography-electrospray ionization mass spectrometry of complex lipidomic samples. Anal Bioanal Chem . 2015;407:5033–5043. doi:10.1007/s00216-015-8528-2 25694148
- Wallace M , Morris C , O’Grada CM , et al. Relationship between the lipidome, inflammatory markers and insulin resistance. Mol Biosyst . 2014;10:1586–1595. doi:10.1039/C3MB70529C 24714806
- Lankinen MA , Stancakova A , Uusitupa M , et al. Plasma fatty acids as predictors of glycaemia and type 2 diabetes. Diabetologia . 2015;58:2533–2544. doi:10.1007/s00125-015-3730-5 26277381
- Terpstra AH , Beynen AC , Everts H , et al. The decrease in body fat in mice fed conjugated linoleic acid is due to increases in energy expenditure and energy loss in the excreta. J Nutr . 2002;132:940–945. doi:10.1093/jn/132.5.940 11983818
- Ostrowska E , Muralitharan M , Cross RF , et al. Dietary conjugated linoleic acids increase lean tissue and decrease fat deposition in growing pigs. J Nutr . 1999;129:2037–2042. doi:10.1093/jn/129.11.2037 10539781
- Holland WL , Brozinick JT , Wang LP , et al. Inhibition of ceramide synthesis ameliorates glucocorticoid-, saturated-fat-, and obesity-induced insulin resistance. Cell Metab . 2007;5:167–179. doi:10.1016/j.cmet.2007.01.002 17339025
- Hammerschmidt P , Ostkotte D , Nolte H , et al. CerS6-derived sphingolipids interact with Mff and promote mitochondrial fragmentation in obesity. Cell . 2019;177:1536–1552. doi:10.1016/j.cell.2019.05.008 31150623
- Wang K , Bao L , Ma K , et al. A novel class of alpha-glucosidase and HMG-CoA reductase inhibitors from Ganoderma leucocontextum and the antidiabetic properties of ganomycin I in KK-Ay mice. Eur J Med Chem . 2017;127:1035–1046. doi:10.1016/j.ejmech.2016.11.015 27839787