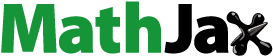
Abstract
Background
Although the incidence of tuberculosis (TB) has dropped substantially, it still is a serious threat to human health. And in recent years, the emergence of resistant bacilli and inadequate disease control and prevention has led to a significant rise in the global TB epidemic. It is known that the cause of TB is Mycobacterium tuberculosis infection. But it is not clear why some infected patients are active while others are latent.
Methods
We analyzed the blood gene expression profiles of 69 latent TB patients and 54 active pulmonary TB patients from GEO (Transcript Expression Omnibus) database.
Results
By applying minimal redundancy maximal relevance and incremental feature selection, we identified 24 signature genes which can predict the TB activation. The support vector machine predictor based on these 24 genes had a sensitivity of 0.907, specificity of 0.913, and accuracy of 0.911, respectively. Although they need to be validated in a large independent dataset, the biological analysis of these 24 genes showed great promise.
Conclusion
We found that cytokine production was a key process during TB activation and genes like CYBB, TSPO, CD36, and STAT1 worth further investigation.
Introduction
Tuberculosis (TB), a pulmonary infectious disease caused by Mycobacterium tuberculosis, is a serious threat to human health. In the early 20th century, TB had spread around the world, killing millions of people.Citation1 Later, with the progress of medical technology and improvement of sanitary conditions, the incidence of TB dropped substantially. However, in recent years the emergence of resistant bacilli and inadequate disease control and prevention has led to a significant rise in the global TB epidemic, which has now become the leading cause of deaths from infectious diseases.Citation2
Currently, about 90%–95% TB patients are with latent tuberculosis infection (LTBI).Citation3 LTBI is defined by persistent immune response caused by M. tuberculosis, but under conditions of a long-term asymptomatic infection.Citation4 During latent infection, body’s immune system can inhibit the growth of bacteria by blocking the replication of the bacteria,Citation5 but not to be completely eliminated. M. tuberculosis can stay dormant for decades, or even during the host’s lifetime.Citation6
However, the risk of developing active TB increases greatly when human immunity declines.Citation7 The main reason bacteria can survive in the body is because it contains high lipid content, which can prevent it from degradation and destruction within macrophages.Citation5
In addition, M. tuberculosis can also affect the normal function of CD8+ T-cells, natural killer cells, and complement membrane attack complex.Citation6 Immune responses to M. tuberculosis is primarily cell-mediated, regulated by the interaction between T-cells and infected macrophages and cytokines secreted by these cells.Citation8 In most cases, infection of M. tuberculosis transits to the dormant state accompanied by the formation of infective granuloma that are well separated from the surrounding tissue.Citation9 Saunders and Cooper indicated that the formation of granulomas is essential for limiting M. tuberculosis growth and tissue damage in TB infections, two major components of active TB.Citation10 Risk factors known for TB reactivation include malnutrition, HIV infection, anti-tumor necrosis factor treatment, insulin-dependent diabetes, alcoholism, and smoking. However, the mechanisms of reactivation of LTBI in most cases are still unknown.Citation11
Early diagnosis and preventive treatment of LTBI are an effective means to eliminate the bacteria and reduce the risk of TB in immunocompromised patients. Currently, the preferred diagnostic tools for LTBI remain tuberculin skin testing (TST) or interferon-γ release assay (IGRA).Citation12 IGRA, based on cellular immunity, was tested to confirm whether people infected with bacteria by detecting the release of IFN-γ to specific antigen of M. tuberculosis. Compared with traditional TST, IGRA has higher specificity and sensitivity, and has been widely used in clinical detection of TB.Citation13 However, they can only detect the infection of TB bacilli, but cannot reflect the risk of developing active TB.
Recently, evidence from researches has demonstrated the importance of genetic factors in the development of active TB,Citation14 but the mechanism of genetic susceptibility for TB remains largely unknown. Since humans are the natural hosts for M. tuberculosis, the precise etiology of infection cannot be studied in any other animal models.Citation15
To understand what actives TB, we analyzed the blood gene expression profiles of 69 latent TB patients and 54 active pulmonary TB (PTB) patients. With advanced feature selection methods, including minimal redundancy maximal relevance (mRMR) and incremental feature selection (IFS), 24 genes were identified as discriminative between latent and active TB patients. What is more, a support vector machine (SVM) classifier for active TB prediction was built based on these 24 genes and its sensitivity, specificity, and accuracy evaluated with leave-one out-cross validation (LOOCV) were 0.907, 0.913, and 0.911, respectively.
Methods
The blood gene expression profiles of latent and active TB patients
To identify the key genes that activate TB, we downloaded the blood gene expression profiles of 69 latent TB patients and 54 active PTB patients from publicly available GEO (Transcript Expression Omnibus) database under accession number of GSE19491.Citation16 The 24 normal samples in the original GSE19491 dataset were excluded, only the 69 latent TB patients and 54 active PTB patients were analyzed to get the key genes that activate TB. These samples were collected from London, UK and Cape Town, South Africa.Citation16 We combined the samples from different cities to get a larger dataset. The age and gender information of these samples are listed in Table S1. Within the 123 samples, there were 64 male and 59 female patients. The average age was 32.5 with an SD of 13.6. The gene expression levels were measured using microarray of Illumina HumanHT-12 V3.0 expression beadchip. There were 48,803 probes corresponding to 25,153 genes. The probes corresponding to the same gene were averaged. The gene expression matrix was quantile normalized.
Identify the genes that are related to the activation of TB
As a basic problem in bioinformatics, many methods have been proposed to identify the phenotype-related genes or proteins. One of these methods, mRMR,Citation17 has been widely used and proved to be effective.Citation18–Citation20
The mRMR method is different from univariate statistical test. To explain its principle, let us use Ω to denote all the 25,153 genes, Ωs to denote the selected m genes, and Ωg to denote the to be selected n genes. First, the relevance of gene g from Ωg with the activeness of TB l was evaluated with mutual information (I) equation:
Then, the average redundancy of gene g with the already selected genes was
At last, to consider both maximum relevance and minimum redundancy, the goal of this algorithm was to find the best gene g from to maximize the function below in which the first part meant relevance and the second represented redundancy
After 25,153 rounds of optimization, all the 25,153 genes can be ranked as
In this gene list, the top ranked ones were discriminative. We considered the top 500 mRMR genes as relevant to the activeness of TB. The top 500 mRMR genes are listed in Table S2.
Find the signature genes for TB activation
The top 500 mRMR genes were related to TB activation, but the number of genes was too large to detect practically. Therefore, we adopted IFSCitation21–Citation27 to get the signature genes for TB activation. Hopefully, a small number of genes were enough to build an actionable predictor for TB activation. The widely used SVM was adopted to construct the classifiers.
During the IFS procedure, the genes were added sequentially based on the mRMR rank, starting with the first mRMR gene, ending with the 500th mRMR gene. In other words, 500 gene sets were selected and 500 SVM classifiers were constructed. Each classifier was evaluated with LOOCV and its sensitivity (Sn), specificity (Sp), and accuracy (ACC) were calculated
TP, TN, FP, and FN were the number of true active TB, true latent TB, false active TB, and latent TB patients.
Based on the IFS results, we can choose the right gene sets as signature and get acceptable prediction accuracy.
Results and discussion
The 500 genes that are related to the activation of TB
Using the mRMR method which considered both the relevance with TB activeness and the redundancy with other genes, we identified 500 genes that were related to the activation of TB. These 500 genes were ranked based on their discriminative ability of latent TB patients and active PTB patients.
The 24 signature genes for TB activation
To further narrow down the 500 genes that were related to the activation of TB and obtain a signature for TB activation, we applied the IFS analysis and plotted the IFS curve based on the prediction performances using different number of signature genes (Table S3). As shown in , when the top 51 mRMR genes were used, the LOOCV accuracy was the highest, 0.919. But when only 24 genes were used, the accuracy became stable. Therefore, we choose the 24 genes as signature genes for TB activation. The sensitivity, specificity, and accuracy of the 24 signature genes for TB activeness prediction were 0.907, 0.913, and 0.911, respectively. The 24 signature genes are given in and the confusion matrix of their prediction performance is shown in .
Table 1 The 24 signature genes for TB activation
Table 2 The confusion matrix of the predicted and actual TB activeness based on the 24 signature genes
Figure 1 The prediction performances for TB activation by using different numbers of signature genes.
Notes: The x-axis is the number of genes in the gene set while y-axis is the prediction accuracy of the SVM classifier evaluated with LOOCV. The peak of the IFS curve had an accuracy of 0.919 when 51 genes were used. But when 24 genes were used, the accuracy has already become stable. Therefore, we choose these 24 genes as signature genes of TB activation. The sensitivity, specificity, and accuracy of the 24 signature genes for TB activeness prediction were 0.907, 0.913, and 0.911, respectively.
Abbreviations: LOOCV, leave-one out-cross validation; IFS, incremental feature selection; SVM, support vector machine; TB, tuberculosis.
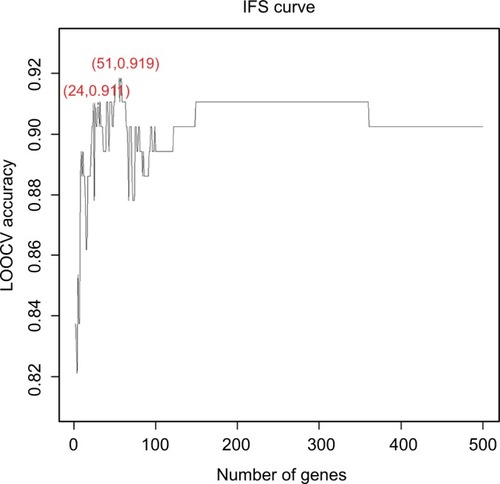
To explore the expression levels of these 24 signature genes in latent TB patients and active PTB patients, we plotted their heatmap in . It can be seen that most of the latent and active TB patients were clustered into the right groups. TMEM51, TSPO, CD36, CYBB, LOXL3, CYB561, GPR63, LYRM1, and STAT1 were highly expressed in active TB patients while SLC9A3R1, TUSC4, ZNF91, HNRNPD, PRKCQ, QSOX2, BANK1, EPHA4, C15orf15, NELL2, ANAPC1, FNBP4, TRIB2, CA5B, and KIAA1370 were highly expressed in latent TB patients.
Figure 2 The heatmap of the 24 signature genes latent and active TB patients.
Notes: The rows represent genes while the columns represent patients. The green and red columns represent latent and active TB patients, respectively. It can be seen that the latent and active TB patients were clustered into different groups.
Abbreviation: TB, tuberculosis.
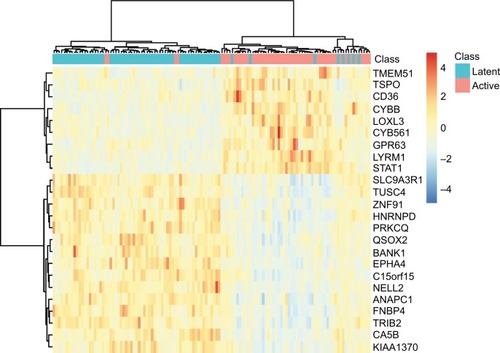
The biological analysis of the signature genes for TB activation
We did Gene Ontology (GO) enrichment analysis of these 24 signature genes and the results with false discovery rate <0.10 are given in . The enriched GO biological processes were GO:0001817 regulation of cytokine production, GO:0001816 cytokine production, GO:0051172 negative regulation of nitrogen compound metabolic process, GO:0042592 homeostatic process, GO:0031324 negative regulation of cellular metabolic process, GO:0010243 response to organonitrogen compound, and GO:0010605 negative regulation of macromolecule metabolic process. There have been many reports of the important roles of cytokine in responding to mycobacteria during the activation of TB.Citation28,Citation29
Table 3 The enriched GO biological processes for the 24 signature genes
The following genes from showed great promise and were discussed.
Gp91phox, encoded by CYBB ranked second in , is an essential subunit of NADPH oxidase complex. Alterations in macrophage function, such as defects in NADPH oxidaseCitation30 and the vitamin D receptor,Citation31 are known as risk factors for mycobacterial infection. Several studies have shown that mutations in CYBB could result in X-linked chronic granulomatous disease with much higher risk of TB,Citation32–Citation34 which is an immunodeficiency caused by defective activity of NADPH oxidase in phagocytes.Citation33,Citation35 Liu et al have also demonstrated the significant correlation between CYBB polymorphisms and decreased risk of TB, particularly among male smokers.Citation36
TSPO ranked third in serves as a trans-mitochondrial membrane channel that transports cholesterol and other endogenous ligands.Citation37 It has been reported that the expression of TSPO is highest in steroidogenic tissues, lung, and immune cells like macrophages.Citation38 Immunofluorescence studies made by Foss et al indicated that TSPO was highly expressed in phagocytic cells and CD68(+) macrophages within TB lesions.Citation39 The increased expression of TSPO will lead to macrophages activation, which is a pivotal component of TB-associated inflammation.Citation39 In PTB, a synthetic ligand for TSPO, radioiodinated DPA-713, is found to be upregulated in activated macrophages.Citation40
CD36 ranked 23rd in is a membrane glycoprotein that exist in various cells, including macrophages, monocytes, adipocytes, and platelets.Citation41 It has been implicated in multiple cellular processes and defined as a multiligand scavenger receptor that mediates fatty acid transport, phagocytosis, and inflammation in response to a variety of pathogens, including mycobacteria.Citation42 CD36 facilitates surfactant lipid uptake which can be exploited by M. tuberculosis for growth.Citation43 Lao et al suggested that rs1194182 and rs10499859, two SNPs of CD36, may reduce the risk of PTB, indicating CD36 as an important biomarker for PTB.Citation44 Hawkes et al observed that deficiency of CD36 reduces the susceptibility of mice to mycobacterial infection. In addition, CD36 deficiency of macrophages inhibits the growth of many mycobacterial species in vitro, demonstrating that deficiency of CD36 plays a role in the resistance to mycobacterial infection.Citation45
STAT1 ranked 24th in , is a member of the STAT protein family and thought to be an important mediator in response to IFN-γ and host defense against M. tuberculosis.Citation46,Citation47 IFN-γ activates macrophages to kill multiple pathogens but cannot activate macrophages to kill M. tuberculosis.Citation48 Much recent evidence shows that M. tuberculosis infection inhibited IFN-γ signaling via blocking several responses to IFN-γ, such as induction of FcγRI,Citation49 and the dysfunction of macrophages in response to IFN-γ depends on an altered regulatory mechanism in STAT1 signaling pathway.Citation50 Sugawara et al discovered that STAT1 knockout mice have higher susceptibility to pulmonary mycobacterial infection in mice, indicating that STAT1 appears to be a key transcription factor in resisting mycobacterial infection.Citation51
The proteomics pattern of the signature genes
Marakalala et al measured the granulomas proteomes of TB patients using mass spectrometry and confocal microscopy.Citation52 They identified 4,406 proteins that were expressed in caseous granuloma caseum, cavitary granuloma cells, cavitary granuloma caseum, and solid granuloma cells. We mapped our signatures onto their proteomics results. Within the 24 signature genes, 6 of them were detected by Marakalala et al. Their log2 label-free quantification intensities in caseous granuloma caseum, cavitary granuloma cells, cavitary granuloma caseum, and solid granuloma cells were shown in . HNRNPD, CYBB, TSPO, SLC9A3R1, FNBP4, and STAT1 are important on both mRNA and protein levels.
Table 4 The log2 label-free quantification intensity of signature genes in caseous granuloma caseum, cavitary granuloma cells, cavitary granuloma caseum, and solid granuloma cells
Conclusion
As a pulmonary infectious disease caused by M. tuberculosis, TB is a serious threat and can spread through coughing, sneezing, or other ways. But not all infected patients exhibit symptoms. Some patients were active while others were latent. Which factors or genes trigger TB activation is key to understand TB. By analysis, the blood gene expression profiles of 69 latent TB patients and 54 active PTB patients, we found 24 signature genes that can predict TB activeness. In-depth analysis of these 24 genes suggested that cytokine production was a key process during TB activation. Signature genes including CYBB, TSPO, CD36, and STAT1 worth to be further investigated.
Acknowledgments
This study was supported by The Key Discipline of Jiaxing Respiratory Medicine Construction Project, Zhejiang North Regional Anesthesia Special Disease Center, Talent Cultivation in Science and Technology Innovation Project of The First Hospital of Jiaxing (No. 2016-CX-04).
Disclosure
The authors report no conflicts of interest in this work.
References
- MjidMCherifJBen SalahNEpidemiology of tuberculosisRev Pneumol Clin2015712–36772 French25131367
- SubbianSPandeyRSoteropoulosPRodriguezGMVaccination with an attenuated ferritin mutant protects mice against virulent Mycobacterium tuberculosisJ Immunol Res20152015112
- SubbianSBandyopadhyayNTsenovaLEarly innate immunity determines outcome of Mycobacterium tuberculosis pulmonary infection in rabbitsCell Commun Signal20131116023958185
- CheeCBSesterMZhangWLangeCDiagnosis and treatment of latent infection with Mycobacterium tuberculosisRespirology201318220521623107218
- Hernandez-PandoROrozcoHAguilarDFactors that deregulate the protective immune response in tuberculosisArch Immunol Ther Exp (Warsz)200957535536719707720
- MuñozLStaggHRAbubakarIDiagnosis and management of latent tuberculosis infectionCold Spring Harb Perspect Med2015511a01783026054858
- SalgamePGeadasCCollinsLJones-LópezEEllnerJJLatent tuberculosis infection—revisiting and revising conceptsTuberculosis (Edinb)201595437338426038289
- O’GarraARedfordPSMcNabFWBloomCIWilkinsonRJBerryMPThe immune response in tuberculosisAnnu Rev Immunol201331147552723516984
- UlrichsTKaufmannSHNew insights into the function of granulomas in human tuberculosisJ Pathol2006208226126916362982
- SaundersBMCooperAMRestraining mycobacteria: role of granulomas in mycobacterial infectionsImmunol Cell Biol200078433434110947857
- LillebaekTDirksenABaessIStrungeBThomsenVØAndersenABMolecular evidence of endogenous reactivation of Mycobacterium tuberculosis after 33 years of latent infectionJ Infect Dis2002185340140411807725
- EsmailHBarryCEWilkinsonRJUnderstanding latent tuberculosis: the key to improved diagnostic and novel treatment strategiesDrug Discov Today2012179–1051452122198298
- ThillaiMPollockKPareekMLalvaniAInterferon-gamma release assays for tuberculosis: current and future applicationsExpert Rev Respir Med201481677824308653
- CookeGSHillAVGenetics of susceptibility to human infectious diseaseNat Rev Genet200121296797711733749
- AroraGMisraRSajidAModel systems for pulmonary infectious diseases: paradigms of anthrax and tuberculosisCurr Top Med Chem201717182077209928137237
- BerryMPGrahamCMMcNabFWAn interferon-inducible neutrophil-driven blood transcriptional signature in human tuberculosisNature2010466730997397720725040
- PengHLongFDingCFeature selection based on mutual information criteria of max-dependency, max-relevance, and min-redundancyIEEE Trans Pattern Anal Mach Intell20052781226123816119262
- LiJHuangTPredicting and analyzing early wake-up associated gene expressions by integrating GWAS and eQTL studiesBiochim Biophys Acta Mol Basis Dis201818646 Pt B2241224629109033
- CaiLHuangTSuJImplications of newly identified brain eQTL genes and their interactors in schizophreniaMol Ther Nucleic Acids20181243344230195780
- ChenLPanXHuXGene expression differences among different MSI statuses in colorectal cancerInt J Cancer Epub2018426
- ZhangNHuangTCaiYDDiscriminating between deleterious and neutral non-frameshifting indels based on protein interaction networks and hybrid propertiesMol Genet Genomics2015290134335225248637
- ShuYZhangNKongXHuangTCaiY-DPredicting A-to-I RNA editing by feature selection and random forestPLoS One2014910e11060725338210
- LiBQYouJHuangTCaiYDClassification of non-small cell lung cancer based on copy number alterationsPLoS One201492e8830024505469
- JiangYHuangTChenLGaoY-FCaiYChouK-CSignal propagation in protein interaction network during colorectal cancer progressionBioMed Res Int20132013119
- ZhangPWChenLHuangTZhangNKongXYCaiYDClassifying ten types of major cancers based on reverse phase protein array profilesPLoS One2015103e012314725822500
- HuangTShuYCaiYDGenetic differences among ethnic groupsBMC Genomics2015161109326690364
- ChenLLiJZhangYHIdentification of gene expression signatures across different types of neural stem cells with the Monte-Carlo feature selection methodJ Cell Biochem201811943394340329130544
- EtnaMPGiacominiESeveraMCocciaEMPro- and anti-inflammatory cytokines in tuberculosis: a two-edged sword in TB pathogenesisSemin Immunol201426654355125453229
- Mayer-BarberKDSherACytokine and lipid mediator networks in tuberculosisImmunol Rev2015264126427525703565
- LauYLChanGCHaSYHuiYFYuenKYThe role of phagocytic respiratory burst in host defense against Mycobacterium tuberculosisClin Infect Dis19982612262279455564
- SunYPCaiQSVitamin D receptor FokI gene polymorphism and tuberculosis susceptibility: a meta-analysisGenet Mol Res20151426156616326125816
- KhanTAKalsoomKIqbalAA novel missense mutation in the NADPH binding domain of CYBB abolishes the NADPH oxidase activity in a male patient with increased susceptibility to infectionsMicrob Pathog201610016316927666509
- BustamanteJAriasAAVogtGGermline CYBB mutations that selectively affect macrophages in kindreds with X-linked predisposition to tuberculous mycobacterial diseaseNat Immunol201112321322121278736
- BareseCCopelliSZandomeniROleastroMZelazkoMRivasEMX-linked chronic granulomatous disease: first report of mutations in patients of ArgentinaJ Pediatr Hematol/Oncol20042610656660
- IshibashiFMizukamiTKanegasakiSImproved superoxide-generating ability by interferon gamma due to splicing pattern change of transcripts in neutrophils from patients with a splice site mutation in CYBB geneBlood200198243644111435314
- LiuQWangJSandfordAJAssociation of CYBB polymorphisms with tuberculosis susceptibility in the Chinese Han populationInfect Genet Evol20153316917525929165
- RupprechtRPapadopoulosVRammesGTranslocator protein (18 kDa) (TSPO) as a therapeutic target for neurological and psychiatric disordersNat Rev Drug Discov201091297198821119734
- ZavalaFHaumontJLenfantMInteraction of benzodiazepines with mouse macrophagesEur J Pharmacol198410635615666151510
- FossCAHarperJSWangHPomperMGJainSKNoninvasive molecular imaging of tuberculosis-associated inflammation with radioiodinated DPA-713J Infect Dis2013208122067207423901092
- TuckerEWPokkaliSZhangZMicroglia activation in a pediatric rabbit model of tuberculous meningitisDis Model Mech20169121497150627935825
- ParkYMCD36, a scavenger receptor implicated in atherosclerosisExp Mol Med2014466e9924903227
- FebbraioMHajjarDPSilversteinRLCD36: a class B scavenger receptor involved in angiogenesis, atherosclerosis, inflammation, and lipid metabolismJ Clin Invest2001108678579111560944
- DoddCEPyleCJGlowinskiRRajaramMVSchlesingerLSCD36-mediated uptake of surfactant lipids by human macrophages promotes intracellular growth of Mycobacterium tuberculosisJ Immunol2016197124727473527913648
- LaoWKangHJinGEvaluation of the relationship between MARCO and CD36 single-nucleotide polymorphisms and susceptibility to pulmonary tuberculosis in a Chinese Han populationBMC Infect Dis201717148828693442
- HawkesMLiXCrockettMCD36 deficiency attenuates experimental mycobacterial infectionBMC Infect Dis201010129920950462
- MerazMAWhiteJMSheehanKCTargeted disruption of the STAT1 gene in mice reveals unexpected physiologic specificity in the JAK-STAT signaling pathwayCell19968434314428608597
- DurbinJEHackenmillerRSimonMCLevyDETargeted disruption of the mouse STAT1 gene results in compromised innate immunity to viral diseaseCell19968434434508608598
- FlynnJLChanJTrieboldKJDaltonDKStewartTABloomBRAn essential role for interferon gamma in resistance to Mycobacterium tuberculosis infectionJ Exp Med19931786224922547504064
- TingLMKimACCattamanchiAAErnstJDMycobacterium tuberculosis inhibits IFN-gamma transcriptional responses without inhibiting activation of STAT1J Immunol199916373898390610490990
- Esquivel-SolísHQuiñones-FalconiFZarain-HerzbergAAmieva-FernándezRILópez-VidalYImpaired activation of STAT1 and c-Jun as a possible defect in macrophages of patients with active tuberculosisClin Exp Immunol20091581455419737230
- SugawaraIYamadaHMizunoSSTAT1 knockout mice are highly susceptible to pulmonary mycobacterial infectionTohoku J Exp Med20042021415014738323
- MarakalalaMJRajuRMSharmaKInflammatory signaling in human tuberculosis granulomas is spatially organizedNat Med201622553153827043495