Abstract
Background
This study aimed to develop and validate a novel angiogenesis-related gene (ARG) signature and molecular subtypes by bioinformatics analysis.
Materials and Methods
The transcriptome data and clinical data were obtained from TCGA and ICGC database. We performed consensus clustering analysis to identify angiogenesis molecular subtypes for ccRCC. Univariate and multivariate Cox regression analyses were used to develop a novel ARG-related signature as a prognostic biomarker for ccRCC. Internal and external validation were then performed in TCGA and ICGC cohort, respectively.
Results
We identified a total of two angiogenesis molecular subtypes of ccRCC. The overall survival (OS) of subtype 1 ccRCC was significantly decreased compared with that of subtype 2 ccRCC (P=0.001). These two molecular subtypes have significantly different tumor microenvironment and immune checkpoint inhibitor sensitivities (P<0.05). Besides, we developed a novel signature based on three ARGs (including MSX1, TIMP1 and JAG2) for subtype 1 ccRCC. The difference in OS between high- and low-risk group was statistically significant in training cohort (P=0.009), test cohort (P=0.024), the whole type 1 cohort (P<0.001), and validation cohort (P=0.041). The AUC for one-year OS prediction was 0.732, 0.710, 0.725, and 0.645 in training cohort, test cohort, the whole type 1 cohort, and validation cohort, respectively. Independent prognostic analysis showed that this signature was an independent predictor for OS of subtype 1 ccRCC (P=0.028914). The power of this prognostic signature was superior to other signatures reported in previous studies.
Conclusion
We developed and successfully validated a novel ARG signature for predicting prognosis of subtype 1 ccRCC, which was superior to several previous signatures.
Introduction
Renal cell carcinoma (RCC) accounts for 90% of adult renal malignancies, and is regarded as the most lethal urological cancer.Citation1,Citation2 Approximately 70–80% of cases with RCC was clear cell RCC (ccRCC).Citation2,Citation3 Tumor heterogeneity leads to different survival outcomes in ccRCC patients.Citation4 At present, there are no consistent molecular subtypes and prognostic molecular characteristics of ccRCC.Citation5 It is urgent to explore effective biomarkers to establish risk stratification method and molecular subtypes to further investigate the potential molecular mechanisms of ccRCC.
It has been reported that tumor angiogenesis plays a vital role in the proliferation and metastasis of ccRCC, as well as antiangiogenic agents.Citation6 The expression of vascular endothelial growth factor receptors (VEGFRs) and hypoxia inducible factor (HIF) was significantly increased in ccRCC cells, which promotes tumor angiogenesis.Citation7 In recent years, the American Food and Drug Administration (FDA) approved tyrosine kinase inhibitors (TKI), including sorafenib, sunitinib, pazopanib etc., as vital anti-cancer targeted drugs by inhibiting VEGFRs.Citation8 TKI targeted therapy might promote the expression of major histocompatibility complex class I (MHC-I) and the infiltration of diverse immune cells, which might contribute to the therapeutic efficacy of immune checkpoint inhibitors.Citation9 Motzer et alCitation10 conducted an integrated evaluation of 823 advanced RCC patients and identified seven molecular subsets with distinct angiogenesis, immune, cell-cycle, metabolism, and stromal programs. These results would contribute to molecularly stratifying RCC patients, explaining improved prognosis of sarcomatoid tumors to immunotherapy versus antiangiogenics alone, and developing personalized therapies. Hence, there might be a very promising value to develop effective risk stratification tools and molecular subtypes for ccRCC patients by using angiogenesis-related genes (ARGs). However, to our knowledge, there are no published studies on developing angiogenesis related molecular subtypes and prognostic signatures of ccRCC from the perspective of ARGs.
In this study, we utilized consensus clustering analysis to identify two angiogenesis molecular subtypes and explored whether the molecular subtypes were associated with antitumor immunity. Besides, we developed a signature based on ARGs for predicting prognosis of subtype 1 ccRCC by using univariate and multivariate Cox regression analyses. Internal and external validation were then performed, and the correlations of the ARG-based signature with tumor microenvironment, immune cell infiltration and immunotherapy were investigated in particular.
Materials and Methods
Data Acquisition and Processing
We downloaded the transcriptome, mutation, copy number variation and clinical data of ccRCC from TCGA database (https://portal.gdc.cancer.gov). There were 539 ccRCC patients with unabridged transcriptome data, 537 ccRCC patients with complete clinical data in TCGA database. The clinical data included age, gender, grade, T stage, N stage, M stage, and AJCC stage. There was a total of 475 ccRCC patients with complete clinical data and 91 ccRCC cases with unabridged transcriptome data in International Cancer Genome Consortium (ICGC) database (https://dcc.icgc.org/). After we merged transcriptome and survival information, there was a total of 90 ccRCC cases with unabridged transcriptome and survival data simultaneously in ICGC database, and 530 ccRCC cases with unabridged transcriptome and survival data simultaneously in TCGA database. All these data were combined into a matrix file using Perl language (http://www.perl.org/). Moreover, gene names of Ensemble IDs were converted into gene symbols utilizing the Ensemble database (http://asia.ensembl.org/signature.html).
The Molecular Signatures Database (MSigDB) (https://www.gsea-msigdb.org/gsea/msigdb) is regarded as a collection of annotated gene sets utilizing GSEA software. We obtained a total of 36 ARGs from HALLMARK ANGIOGENESIS gene set (H: hallmark gene sets; systematic name: M5944) in MSigDB database. All these ARGs were up-regulated during formation of blood vessels (angiogenesis). The detailed list of these ARGs was presented in Supplemental Document.
Identification of Angiogenesis-Related DEGs and Functional Enrichment
The mRNA expression matrix of 36 ARGs in TCGA cohort was extracted. We utilized the “limma” R package and Wilcoxon test to filter angiogenesis-related differentially expressed genes (DEARGs) between the normal samples and ccRCC samples in TCGA cohort. The cut-off value was false discovery rate (FDR) < 0.05 and log2 |fold change (FC)| > 1. The Metascape database (http://metascape.org/) was utilized for functional enrichment analysis of DEARGs. The mutation status and copy number variation of these 36 ARGs were also explored using TCGA data.
Identifying Angiogenesis Molecular Subtypes Using Consensus Clustering Analysis
Firstly, univariable Cox regression analysis was used to screen prognostic DEARGs that were associated with OS of ccRCC. We then performed consensus clustering analysis for identifying angiogenesis-related molecular subtypes of ccRCC based on DEARGs by using R package “ConsensusClusterPlus”. Next, we used R package “survival” and “survminer” to investigate the association between angiogenesis molecular subtypes and OS. R package “pheatmap” was used to show the association between angiogenesis molecular subtypes and clinicopathologic features (including T stage, N stage, M stage, AJCC stage, grade, gender, and age).
Development of a Novel Angiogenesis-Based Prognostic Signature for Subtype 1 ccRCC
All ccRCC patients with subtype 1 were randomly divided into training cohort and test cohort. We then performed univariate and multivariate Cox regression analyses in training cohort to establish an angiogenesis-based signature for predicting OS in subtype 1 ccRCC. Patients were divided into two groups, high-risk group and low-risk group, according to median value of the risk score. Survival analysis and time-dependent receiver operating characteristic (ROC) curve were performed to validate the performance of this angiogenesis-based signature. Univariate and multivariate independent prognostic analyses were used to investigate whether the angiogenesis-based prognostic signature was an independent indicator of OS of ccRCC.
Internal and External Validation of This Angiogenesis-Based Prognostic Signature
To perform internal and external validation for this novel angiogenesis-based prognostic signature, we calculated risk score for each case by risk formula in test cohort, subtype 2 cohort, and ICGC cohort. Patients in test cohort, subtype 2 cohort, and ICGC cohort were also divided into two groups, high-risk group and low-risk group, according to median value of the risk score.
Survival analysis and time-dependent ROC curve were also performed to validate the performance of angiogenesis-based signature. Moreover, the performance of this signature was also compared with previous signatures using ROC curve. The expression heatmap, the distribution of risk score and survival time of training cohort, test cohort, whole subtype 1 cohort, and ICGC cohort were presented using “pheatmap” R package.
Exploration of the Associations of this Angiogenesis-Related Signature with Tumor Microenvironment, Immune Cell Infiltration and Immune Functions
The ESTIMATE algorithm was applied to access tumor microenvironment (TME) scores while the CIBERSORT method was utilized to evaluate the score of 22 types of immune infiltrating cells.Citation11,Citation12 Then, we calculated the infiltrating score of 22 immune cells using CIBERSORT method and tumor microenvironment (TME) scores using ESTIMATE algorithm. The associations of angiogenesis molecular subtypes with immune cell infiltration and tumor microenvironment were explored. Generally, the expression levels of immune checkpoint gene are closely related to the sensitivity of immunotherapy. We investigated the association of the angiogenesis molecular subtypes with expression level of PD-L1 gene to predict immunotherapy sensitivity. P value < 0.05 was considered statistically significant.
Besides, we calculated the infiltrating score of 16 immune cells and the activity of 13 immune-related pathways of TCGA cohort using single-sample gene set enrichment analysis (ssGSEA) in the “gsva” R package. Then, the associations of the angiogenesis-based signature with immune cells infiltration and immune-related pathways activity were explored.
Validation of Hub Biomarkers in Multi-Database
Survival analysis based on GEPIA database (http://gepia.cancer-pku.cn/index.html) was performed to evaluate the prognostic value of these three hub biomarkers. UALCAN database (http://ualcan.path.uab.edu/) is a portal for facilitating tumor subgroup gene expression and survival analyses. Expression levels and promoter methylation levels of hub genes were revealed using UALCAN database.
Results
Angiogenesis-Related DEGs and Functional Enrichment
The clinicopathologic data of TCGA cohort and ICGC cohort were demonstrated in . There was a total of 36 ARGs (Supplemental Document). The mutation status and copy number variation of these 36 ARGs were presented in . A total of 17 DEARGs were finally identified, including 3 downregulated genes and 14 upregulated genes (). The results of functional enrichment analysis of these DEARGs were presented in . These 17 DEARGs were mainly enriched in extracellular structure organization, response to wounding, response to growth factor, NABA PROTEOGLYCANS, integrin-mediated signaling pathway, post-translational protein phosphorylation, platelet degranulation, regulated exocytosis, positive regulation of cytokine production, cartilage development, HTLV-I infection, transmembrane receptor protein serine/threonine kinase signaling pathway, and embryonic morphogenesis.
Table 1 Clinicopathologic Data of TCGA Cohort and ICGC Cohort
Table 2 Angiogenesis-Related Differentially Expressed Genes
Identifying Two Angiogenesis Molecular Subtypes of ccRCC
Univariable Cox regression analysis revealed that there was a total of nine angiogenesis-related DEGs associated with OS, including COL5A2, JAG2, LPL, LUM, MSX1, NRP1, PF4, PRG2, TIMP1. We identified a total of two angiogenesis molecular subtypes of ccRCC, including 213 cases of subtype 1 and 317 cases of subtype 2 ( ). The OS of subtype 1 ccRCC was significantly decreased compared with that of subtype 2 ccRCC (P=0.001, ). The correlation heatmap between the angiogenesis molecular subtypes and clinicopathologic features was presented in . As indicated by heatmap, these two molecular subtypes have significantly different AJCC stage and T stage.
Figure 2 Consensus clustering analysis for identifying two angiogenesis molecular subtypes of ccRCC (A and B). Comparison of OS between these two subtypes (C). The correlation heatmap between this angiogenesis molecular subtypes and clinicopathologic features (D). The expression level of PD-L1 between these two subtypes (E). The expression correlation of PD-L1 with nine angiogenesis-related prognostic DEGs (F). **P<0.01; *P<0.05.
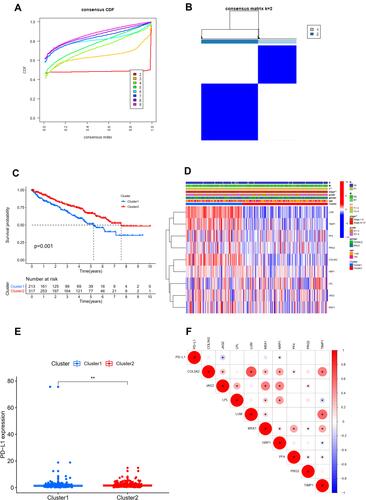
The expression level of PD-L1 was significantly decreased in subtype 1 group in comparison with that in subtype 2 group (P <0.01, ). The expression correlation of PD-L1 with these nine angiogenesis-related prognostic DEGs (including COL5A2, JAG2, LPL, LUM, MSX1, NRP1, PF4, PRG2, TIMP1) was demonstrated in . The results showed that the expression level of PD-L1 was significantly associated with the expression level of JAG2 and NRP. The immune score, stromal score, and ESTIMATE score in subtype 1 group was significantly increased compared with that in subtype 2 group while the tumor purity score in subtype 1 group was significantly decreased compared with that in subtype 2 group (P<0.05, –). The infiltrating proportions of naive B cells, regulatory T cells, M0 macrophages, M2 macrophages in subtype 1 group were significantly increased compared with that in subtype 2 group while the infiltrating proportions of CD8 T cells, T follicular helper cells, monocytes, M1 macrophages, resting dendritic cells in subtype 1 group were significantly decreased compared with that in subtype 2 group (P<0.05, ).
Figure 3 Relationship between angiogenesis molecular subtypes and tumor immune microenvironment. Correlation of angiogenesis molecular subtypes with ESTIMATE score (A), tumor purity (B), immune score (C), stromal score (D). Correlation of angiogenesis molecular subtypes with tumor-infiltrating immune cells (E).
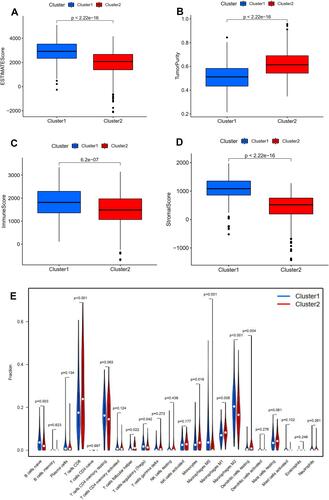
Development and Validation of a Novel Angiogenesis-Based Signature for Prognosis Prediction of Subtype 1 ccRCC
There was a total of 213 cases of type 1 ccRCC, including 108 in the training cohort and 105 in test cohort. A total of 90 ccRCC cases in ICGC database were used as an external validation cohort. We performed multivariate Cox regression analysis to develop a novel angiogenesis-based signature using three ARGs in training cohort for prognosis prediction of subtype 1 ccRCC. The calculation formula of risk score is shown as follows: risk score = 0.202457231804962 * MSX1 + 0.000522859217901573 * TIMP1 - 0.109529744114114 * JAG2 ().
Table 3 Univariate and Multivariate Cox Regression Analysis to Develop Angiogenesis-Based Prognostic Signature of Subtype 1 ccRCC
The difference in OS between high-risk and low-risk group was statistically significant in training cohort (P = 0.009), test cohort (P = 0.024), whole type 1 cohort (P < 0.001), and ICGC cohort (P = 0.041), respectively. High-risk score was associated with significantly poor OS in comparison with low-risk score in training cohort, test cohort, whole type 1 cohort and ICGC cohort, respectively. The area under ROC curve (AUC) for one year OS prediction was 0.732, 0.710, 0.725 and 0.645 in training cohort, test cohort, whole type 1 cohort and ICGC cohort, respectively, suggesting the potential power of this prognostic signature for prognosis prediction of subtype 1 ccRCC (). The expression heatmap, the distribution of risk score and survival time of training cohort, test cohort, whole subtype 1 cohort and ICGC cohort were demonstrated in .
Figure 4 Internal and external validation of a novel angiogenesis-based prognostic signature in subtype 1 ccRCC. The survival analysis between high and low risk group and corresponding area under ROC curve in training cohort (A and B), test cohort (C and D), the whole type 1 cohort (E and F) and validation cohort (G and H).
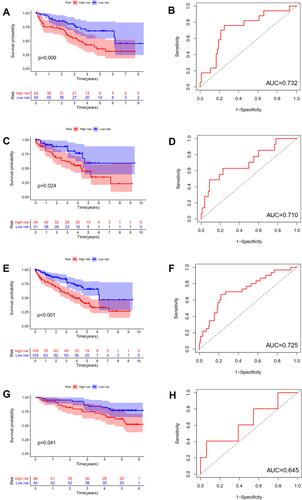
Independent Prognostic Analysis and Comparison with Previous Models
Univariate and multivariate independent prognostic analyses showed that this signature was an independent predictor for subtype 1 ccRCC, indicating the great performance of this signature (P < 0.05, ). Besides, the difference in OS between high-risk and low-risk group of subtype 2 ccRCC in TCGA cohort was also statistically significant (). The area under ROC curve (AUC) of our signature for OS prediction was higher in comparison with YangSu’s signature, YueheGuo’s signature, JingminZhou’s signature, YueWu’s signature, suggesting that the power of this prognostic signature was superior to other signatures reported in previous studies (). Stage III–IV, grade 3–4 and T3-4 were significantly associated with higher risk score compared with stage I–II, grade 1–2 and T1-2, respectively (P < 0.05, –). The correlation heatmap among this signature, clinicopathologic features, angiogenesis molecular subtypes and immune score was presented in .
Table 4 Univariate and Multivariate Independent Prognostic Analysis
Association of the Angiogenesis-Related Signature with Immune Cell Infiltration and Immune Function
We quantified the immune cell infiltration and immune functions using ssGSEA to further explore the association of this angiogenesis-based signature with immune status. Interestingly, the infiltrating proportions of aDCs, macrophages, Tfh, Th1 cells and Th2 cells in high-risk group were significantly increased compared with that in low-risk group, while the infiltrating proportions of mast cells in high-risk group were significantly decreased compared with that in low-risk group (P<0.05, ). Moreover, the results also showed that patients with high-risk score have higher scores of immune function or pathways than those with low risk, including APC co-inhibition, CCR, para-inflammation, T cell co-inhibition, T cell co-stimulation. However, patients with high risk had a lower score of Type II IFN response than those with low risk (P<0.05, ).
Validation of Hub Biomarkers in Multi-Database
It was also found that expression levels of MSX1, TIMP1 and JAG2 were significantly increased in ccRCC tissues compared with that in normal tissues. Using ULCAN database, we indicated that MSX1 has significantly higher levels of promoter methylation in ccRCC tissues in comparison with that in normal tissue while the promoter methylation level of JAG2 was significantly lower in ccRCC tissues compared with that in normal tissue. However, the promoter methylation level of TIMP1 between ccRCC and normal tissue was not statistically significant (). The survival analysis revealed that high expression of TIMP1 was significantly associated with poor OS and disease-free survival (DFS) of ccRCC patients, while high expression of JAG2 was significantly associated with higher OS of ccRCC patients. The expression level of MSX1 was not associated with OS and DFS of ccRCC patients. The expression level of JAG2 was not associated with DFS of RCC patients ().
Discussion
It has been confirmed that paracrine and autocrine factors promoting angiogenesis is vital in balancing cell proliferation, orientation, migration, and elongation, which might result in micro-vessels’ formation and establishment.Citation13 Increasing evidence indicates that aberrant angiogenesis in tumor microenvironment is of great significance in processes of carcinogenesis, progression, and metastasis of ccRCC.Citation14 However, there is no published study focusing on the correlation of angiogenesis with prognosis evaluation and molecular subtypes of ccRCC. In this study, we identified a total of two angiogenesis molecular subtypes of ccRCC using consensus clustering analysis. The OS of subtype 1 ccRCC was significantly decreased compared with that of subtype 2 ccRCC. Further investigation showed that these two molecular subtypes had significantly varying tumor microenvironments. The expression level of PD-L1 was significantly decreased in subtype 1 group in comparison with that in subtype 2 group.
Most importantly, we developed a novel prognostic signature related to angiogenesis for subtype 1 ccRCC. Univariate and multivariate Cox regression analyses revealed that this novel prognostic signature was a vital independent predictor for OS of ccRCC. Internal and external validation indicated the potential role of this angiogenesis-related signature for subtype 1 ccRCC. There have been several prognostic models reported previously. Chu et alCitation15 constructed a novel seven protein signature for predicting prognosis. Xing et al utilized glycolysis-related genes to successfully establish and externally verify a novel signature for predicting OS of ccRCC.Citation16 Wang et alCitation17 successfully constructed a prognostic model for ccRCC based on apoptosis-related genes, which would help clinicians make the prognostic assessment. However, there has been no study developing prognostic signature of ccRCC from the point of angiogenesis and molecular subtypes. In this study, we developed a novel angiogenesis-related prognostic signature for subtype 1 ccRCC for the first time. Most importantly, both internal validation and external validation suggested the satisfactory predictive power of this signature. Furthermore, we interestingly found that the area under ROC curve (AUC) of our signature for OS prediction was higher in comparison with YangSu’s signature,Citation18 YuheGuo’s signature,Citation19 JingminZhou’s signature,Citation20 YueWu’s signature,Citation21 suggesting that the power of this prognostic signature might be superior to other signatures reported in previous studies. Furthermore, we demonstrated that stage III–IV, grade 3–4 and T3-4 were significantly associated with higher risk score compared with stage I–II, grade 1–2 and T1-2, respectively, and that high and low-risk score ccRCC patients have significantly varying immune cell infiltration and immune function.
These three vital ARGs (including MSX1, TIMP1 and JAG2), which were used to construct angiogenesis-related prognostic signature, could be considered as candidate markers of ccRCC. Dias et alCitation22 found that extracellular vesicle-derived TIMP1 in plasma could be used as an adverse potential prognostic biomarker of ccRCC. Armstrong et alCitation23 detected 23 types of angiokines in ccRCC patients in a randomized open-label Phase II trial, and found that the expression level of TIMP1 increased with progression on everolimus or sunitinib treatment. He et alCitation24 reported that RUNX3 played a vital role in inhibiting ccRCC proliferation and metastasis of cRCC cells by regulating TIMP1. Yang et alCitation25 considered that IL-8 could recruit tumor associated neutrophils and induce the expression of JAG2 in tumor associated neutrophils, which play an important role in regulating immune evasion microenvironment in epithelial ovarian cancer. Hatano et al revealed that JAG2 overexpression has potential prognostic value in oral squamous cell carcinoma.Citation26 He et alCitation27 demonstrated that interaction of JAG2 and PRAF2 facilitates colorectal carcinoma cells’ migration and invasion through non-canonical Notch and non-EMT-dependent pathways. MSX1 could inhibit angiogenesis to suppress tumorigenesis.Citation28 Son et alCitation28 showed that the interaction of PIASy and MSX1 mediated the inhibition of tumor angiogenesis. Yue et alCitation29 reported that msh homeobox 1 (MSX1) could suppress breast tumorigenesis through arresting G1/S cell cycle and apoptosis, and the methylation of MSX1 might be regarded as an epigenetic biomarker for early diagnosis of breast cancer. However, there has been no study focusing on the role of MSX1 and JAG2 in ccRCC. In this study, we found that expression levels of MSX1, TIMP1 and JAG2 were significantly increased in RCC tissues compared with normal tissues. High expression of TIMP1 was significantly associated with poor OS and DFS of ccRCC patients while high expression of JAG2 was significantly associated with higher OS of ccRCC patients. Besides, we revealed that MSX1 has significantly higher levels of promoter methylation in ccRCC tissues in comparison with normal tissue, while the promoter methylation level of JAG2 was significantly lower in ccRCC tissues compared with normal tissue.
However, we have to acknowledge that several limitations in our study were inescapable. First of all, retrospective design with limited sample size would result in selection bias. Larger prospective studies should be performed for generalizability. Secondly, all of the work in this study was purely based on bioinformatics analysis and did not achieve any mechanistic explanation. Further in vitro and in vivo experiments into the underlying mechanism are warranted.
In this study, we identified two angiogenesis-related molecular subtypes for ccRCC. These two molecular subtypes have significantly different prognosis, tumor microenvironment, and immune checkpoint inhibitor sensitivities. Besides, we developed and successfully validated a novel angiogenesis-related gene signature for predicting prognosis of subtype 1 ccRCC, which was superior to several previous signatures. The angiogenesis-based molecular subtypes and prognostic signature would contribute to further understanding of molecular mechanism in ccRCC.
Data Sharing Statement
All data generated or analyzed during the present study were downloaded from The Cancer Genome Atlas (TCGA) database (https://portal.gdc.cancer.gov), International Cancer Genome Consortium (ICGC) database (https://dcc.icgc.org/), and Molecular Signatures Database (MSigDB) (https://www.gsea-msigdb.org/gsea/msigdb).
Ethics Approval and Consent to Participate
This study was conducted in accordance with the Ethical Standards of the Institutional Ethics Committee of First Affiliated Hospital of Fujian Medical University and with the 1964 Helsinki declaration and its later amendments or comparable Ethical Standards.
Author Contributions
All authors contributed to data analysis, drafting or revising the article, gave final approval of the version to be published, agreed to the submitted journal, and agreed to be accountable for all aspects of the work.
Disclosure
All authors declare no conflicts of interest.
Additional information
Funding
References
- Gao S, Gao L, Wang S, et al. ATF3 suppresses growth and metastasis of clear cell renal cell carcinoma by deactivating EGFR/AKT/GSK3β/β-catenin signaling pathway. Front Cell Dev Biol. 2021;9:618987.
- Rossi SH, Klatte T Usher-Smith J, et al. Epidemiology and screening for renal cancer. World J Urol. 2018;36(9):1341–1353. doi:10.1007/s00345-018-2286-7
- Liu Y, Huang Z, Cheng G, et al. Development of a four-gene prognostic model for clear cell renal cell carcinoma based on transcriptome analysis. Genomics. 2021;113(4):1816–1827.
- Lv Z, Qi L, Hu X, et al. Identification of a novel glycolysis-related gene signature correlates with the prognosis and therapeutic responses in patients with clear cell renal cell carcinoma. Front Oncol. 2021;11:633950. doi:10.3389/fonc.2021.633950
- Marchioni M, Rivas JG, Autran A, et al. Biomarkers for renal cell carcinoma recurrence: state of the art. Curr Urol Rep. 2021;22(6):31. doi:10.1007/s11934-021-01050-0
- Zheng W, Zhang S, Guo H, et al. Multi-omics analysis of tumor angiogenesis characteristics and potential epigenetic regulation mechanisms in renal clear cell carcinoma. Cell Commun Signal. 2021;19(1):39. doi:10.1186/s12964-021-00728-9
- Lai Y, Zhao Z, Zeng T, et al. Crosstalk between VEGFR and other receptor tyrosine kinases for TKI therapy of metastatic renal cell carcinoma. Cancer Cell Int. 2018;18(1):31. doi:10.1186/s12935-018-0530-2
- Roskoski R Jr. Vascular endothelial growth factor (VEGF) and VEGF receptor inhibitors in the treatment of renal cell carcinomas. Pharmacol Res. 2017;120:116–132. doi:10.1016/j.phrs.2017.03.010
- Rassy E, Flippot R, Albiges L. Tyrosine kinase inhibitors and immunotherapy combinations in renal cell carcinoma. Ther Adv Med Oncol. 2020;12:1758835920907504. doi:10.1177/1758835920907504
- Motzer RJ, Banchereau R, Hamidi H, et al. Molecular subsets in renal cancer determine outcome to checkpoint and angiogenesis blockade. Cancer Cell. 2020;38(6):803–817.e4. doi:10.1016/j.ccell.2020.10.011
- Xu N, Ke Z-B, Lin X-D, et al. Development and validation of a molecular prognostic index of bladder cancer based on immunogenomic landscape analysis. Cancer Cell Int. 2020;20(1):302. doi:10.1186/s12935-020-01343-3
- Ke ZB, Wu YP, Huang P, et al. Identification of novel genes in testicular cancer microenvironment based on ESTIMATE algorithm-derived immune scores. J Cell Physiol. 2021;236(1):706–713.
- Viallard C, Larrivée B. Tumor angiogenesis and vascular normalization: alternative therapeutic targets. Angiogenesis. 2017;20(4):409–426. doi:10.1007/s10456-017-9562-9
- Seles M, Hutterer GC, Foßelteder J, et al. Long non-coding RNA PANTR1 is associated with poor prognosis and influences angiogenesis and apoptosis in clear-cell renal cell cancer. Cancers. 2020;12(5):1200. doi:10.3390/cancers12051200
- Chu G, Xu T, Zhu G, et al. Identification of a novel protein-based signature to improve prognosis prediction in renal clear cell carcinoma. Front Mol Biosci. 2021;8:623120. doi:10.3389/fmolb.2021.623120
- Xing QW, Zeng TY, Liu SY, et al. A novel 10 glycolysis-related genes signature could predict overall survival for clear cell renal cell carcinoma. BMC Cancer. 2021;21(1):381. doi:10.1186/s12885-021-08111-029
- Wang Y, Chen Y, Zhu B, et al. A novel nine apoptosis-related genes signature predicting overall survival for kidney renal clear cell carcinoma and its associations with immune infiltration. Front Mol Biosci. 2021;8:567730. doi:10.3389/fmolb.2021.567730
- Su Y, Zhang T, Tang J, et al. Construction of competitive endogenous RNA network and verification of 3-key LncRNA signature associated with distant metastasis and poor prognosis in patients with clear cell renal cell carcinoma. Front Oncol. 2021;11:640150. doi:10.3389/fonc.2021.640150
- Guo Y, Li X, Zheng J, et al. Identification of a novel immune-related microRNA prognostic model in clear cell renal cell carcinoma. Transl Androl Urol. 2021;10(2):888–899. doi:10.21037/tau-20-1495
- Zhou J, Liu G, Wu X, et al. A risk score model based on nine differentially methylated mRNAs for predicting prognosis of patients with clear cell renal cell carcinoma. Dis Markers. 2021;2021:8863799. doi:10.1155/2021/8863799
- Wu Y, Wei X, Feng H, et al. Transcriptome analyses identify an RNA binding protein related prognostic model for clear cell renal cell carcinoma. Front Genet. 2020;11:617872. doi:10.3389/fgene.2020.617872
- Dias F, Teixeira AL, Nogueira I, et al. Plasma extracellular vesicle-derived TIMP-1 mRNA as a prognostic biomarker in clear cell renal cell carcinoma: a Pilot Study. Int J Mol Sci. 2020;21(13):4624.
- Armstrong AJ, Nixon AB, Carmack A, et al. Angiokines associated with outcomes after sunitinib or everolimus treatment in patients with non-clear cell renal cell carcinoma. Clin Cancer Res. 2021;27(12):3503–3503.
- He L, Zhao X, Wang H, et al. RUNX3 mediates suppression of tumor growth and metastasis of human CCRCC by regulating cyclin related proteins and TIMP-1. PLoS One. 2012;7(3):e32961. doi:10.1371/journal.pone.0032961
- Yang M, Zhang G, Wang Y, et al. Tumour-associated neutrophils orchestrate intratumoural IL-8-driven immune evasion through Jagged2 activation in ovarian cancer. Br J Cancer. 2020;123(9):1404–1416. doi:10.1038/s41416-020-1026-0
- Hatano K, Saigo C, Kito Y, et al. Overexpression of JAG2 is related to poor outcomes in oral squamous cell carcinoma. Clin Exp Dent Res. 2020;6(2):174–180. doi:10.1002/cre2.267
- He W, Tang J, Li W, et al. Mutual regulation of JAG2 and PRAF2 promotes migration and invasion of colorectal cancer cells uncoupled from epithelial–mesenchymal transition. Cancer Cell Int. 2019;19(1):160. doi:10.1186/s12935-019-0871-5
- Son MJ, Rho SB, Kim K, et al. Homeoprotein Msx1-PIASy interaction inhibits angiogenesis. Cells. 2020;9(8):1854. doi:10.3390/cells9081854
- Yue Y, Yuan Y, Li L, et al. Homeobox protein MSX1 inhibits the growth and metastasis of breast cancer cells and is frequently silenced by promoter methylation. Int J Mol Med. 2018;41(5):2986–2996.