Abstract
Background
Endometrial carcinoma (EC) is one of the most common malignancies. Immunotherapy has shown promising effects in the treatment against specific subtypes of EC.
Methods
The RNA and clinical information of patients with EC were acquired from The Cancer Gene Atlas (TCGA) database. Firstly, the differentially expressed pyroptosis-related lncRNAs (PRLs) were screened between the tumor and normal control tissue. Secondly, the PRLs closely related to survival were identified by univariate and multivariate regression analysis, based on which, we evaluated the risk score for each EC patient to construct a risk signature. Moreover, we assessed the prognostic value, clinical relevance immunity, and immunotherapy based on this signature.
Results
We screened out 9 individual PRLs (AC087491.1, AL353622.1, AL035530.2, LINC02036, AL021578.1, AL390195.2, AC009097.2, AC004585.1, and AC244517.7) closely related to the prognosis of EC. Kaplan–Meier analyses showed a poorer prognosis for the patients in the high-risk FRLs signature (P < 0.001). The area under the curve (AUC) for 1 year, 2 years, 3 years was 0.693, 0.694, 0.750, respectively. Our risk model could be considered as an independent prognostic marker for EC (P < 0.001, HR:2.172, 95% CI:1.532–3.079). Moreover, immune functions and checkpoints were generally different in the 2 groups. Simulation analysis by termed immunophenoscores hinted that immunotherapy might bring optimal therapeutic effect in the low-risk group.
Conclusion
We successfully developed a novel signature with 9 lncRNAs related to pyroptosis, which may be used as biomarkers to evaluate the prognosis and immune treatment of EC.
Introduction
Endometrial carcinoma (EC) is the sixth most prevalent cancer in women of 185 countries, with 417,000 patients diagnosed and 97,000 died in the past 2020.Citation1 Patients at stage I have the favorable clinical outcome, whereas the survival rate for stage III– stage IV is very poor.Citation2 Considering the low survival rate for the patients at a late stage, we need to find proper prognostic biomarkers to prolong the survival time of patients with EC. HE4, alone or associated with CA125, may be used to evaluate prognosis and survival of EC.Citation3,Citation4 However, as far as we know, there is still a lack of accurate diagnostic and prediction models for EC.
Pyroptosis is a type of programmed cell death driven by inflammatory caspases, accompanied with an intact nucleus and the formation of plasma membrane pore.Citation5 It is associated with various cancers. Wang et al found up-regulated caspase-1, IL-1β, and IL-18 in the esophageal cancer tissues, which indicated that pyroptosis could be involved in the development of esophageal cancer.Citation6 In gastric cancer cells, the caspase-3 dependent apoptosis induced by 5-FU was converted to pyroptosis through gasdermin E.Citation7 Docosahexaenoic acid (DHA) could lead to pyroptosis in breast cancer cells and might be a useful addition in breast cancer treatment.Citation8 Extracellular signal-regulated kinases (ERK) activation is pivotal in cancer cell survival through the upregulation of anti-apoptotic proteins and inhibition of caspase activity. In ovarian cancer, the inhibition of the c-Jun N-terminal kinase (JNK) pathway by targeting ERK or MEK leads to the suppression of tumor growth. Indeed, poly (ADP-ribose) polymerase 1 (PARP1) inhibition causes a loss of ERK2 stimulation by decreasing the activity of critical pro-angiogenic factors.Citation9
The long non-coding RNAs (LncRNAs) are longer than 200 bp in the length of the transcription.Citation10 They do not encode proteins but play important roles in nearly every level of gene expression.Citation11 The expression levels of lncRNAs in tumor tissue possibly affect the tumor progression and metastasis.Citation12 At the same time, some special lncRNAs act in the pyroptosis of cancers. For example, the lncRNA RP1-85F18.6 put an impact on the proliferation, invasion, and pyroptosis of colorectal cancer cells by regulating the expression of ΔNp63.Citation13 The decreased long noncoding RNA growth arrest specific transcript 5 (lncRNA GAS5) was found accompanied with reduced pyroptosis in ovary cancer.Citation14
We notice that both pyroptosis and certain types of lncRNAs influence the occurrence and development of cancers. Recent studies have reported that a group of lncRNAs, such as FRMD6-AS2, AL161431.1, LINC01133, LINC01243, PCAT1, MALAT1, and CARLo-5 were associated with EC.Citation15–Citation20 We assumed that a risk model found based on pyroptosis-related lncRNAs might help us better identify EC and prolong the survival time of EC patients. Meanwhile, although a pyroptosis-related lncRNA signature showed potential predictive value in immune target therapy for head and neck squamous cell carcinoma,Citation21 the relationship between pyroptosis-related IncRNAs and immune target therapy in EC is still unknown. In our study, for the first time, we used The Cancer Gene Atlas (TCGA) data of patients with EC to identify a set of pyroptosis-related lncRNAs. Based on the lncRNAs, a risk signature was successfully constructed. We expect to use the risk model to judge prognosis and personalize the immune treatment of EC in the future.
Methods
Data Sources
The mRNA expression profiles and clinical information of patients with endometrial carcinoma (EC) were downloaded from The Cancer Gene Atlas (TCGA) database (https://tcga-data.nci.nih.gov/tcga/). We extracted pyroptosis-related genes from previous reviews.Citation22–Citation25 We got immune infiltration data from the tumor immune estimation resource (TIMER) database (http://timer.comp-genomics.org). Scores for immune treatment and microsatellite instability status of EC were downloaded from The Cancer Immunome Atlas (TCIA) database (https://tcia.at/).
Identification of Differentially Expressed Long Non-Coding RNAs (lncRNAs) Related to Pyroptosis
Firstly, we screened out the pyroptosis-related lncRNAs by co-expressing relationships between pyroptosis-related genes and lncRNAs. Secondly, we identified differentially expressed pyroptosis-related lncRNAs between the EC and control tissues (with false discovery rate (FDR) < 0.05 and |log2 FC|≥ 1). The expression data of the pyroptosis-related lncRNAs were merged with the survival data of EC. (By employing the “limma” R package)
Development of a Pyroptosis-Related lncRNAs Signature
First, we applied univariate and multivariate Cox analysis to look out the pyroptosis-related lncRNAs associated with the survival of patients with EC. Then a risk signature was further developed with the least absolute shrinkage and selection operator (LASSO) cox regression model using the “glmnet”, “survival”, and “survminer” R packages. Risk scores= (X: coefficient of each lncRNA, Y: expression of each lncRNA). Based on the median score, the EC patients were separated into low- and high-risk groups. The results were visualized by employing “pheatmap” R package. We used the Kaplan–Meier survival curve analysis and the receiver operating characteristic (ROC) curve to evaluate the sensitivity and specificity of the pyroptosis-related lncRNAs signature l (By employing the “timeROC”, “survival” and “survminer” R packages). Both univariate Cox regression analysis and multivariate Cox regression analysis were applied to assess the prognostic relationship between risk score, age, and grade. We used Cytoscape 3.8.2 software to construct the pyroptosis-related genes-lncRNAs regulatory network.
Immunity Analysis of Pyroptosis-Related lncRNAs Signature
According to the risk scores, EC Patients were separated into 2 risk groups. The immune-related functions and immune checkpoints were assessed by applying enrichment analysis (By employing the “limma”, “pheatmap” “GSVA”, “GSEABase”, “ggpubr”, and “reshape2” R packages).
Immunotherapy Evaluation of Pyroptosis-Related lncRNAs Signature
Charoentong et alCitation26 created quantification by termed immunophenoscores (IPS) for 20 solid cancers through machine learning, which could be used to predict the response of those cancers to anti-programmed cell death protein 1 (PD-1) and anti-cytotoxic T lymphocyte antigen-4 (CTLA-4). In this model, higher IPS mean a better response to corresponding immunotherapy. We evaluated the immunotherapy of EC by employing “ggpubr” and “ggplot2” R package.
Statistical Analyses
Statistical analysis was applied by R version 4.0.2 (Institute for Statistics and Mathematics, Vienna, Austria). We used the Wilcoxon-test to compare the expression levels of pyroptosis-related lncRNAs between EC and adjacent normal tissues. The Pearson correlation was used to contrast the categorical variables. We compared the overall survivals (OS) of patients between the low- and high-risk groups by applying the Kaplan-Meier curve. Univariate and multivariate Cox regression analyses were used to find out the independent factors related to survival rate. A P-value less than 0.05 was considered statistically significant.
Results
Differential Expression of Pyroptosis-Related Long Non-Coding RNAs (lncRNAs)
The RNA-seq data of 35 paracancerous tissues and 552 endometrial carcinoma (EC) tissues and the clinical information of EC patients were downloaded from The Cancer Gene Atlas (TCGA) cohort. The 33 genes related to pyroptosis were extracted from the published reviews (Supplementary Table 1). We initially identified 170 significantly differently expressed pyroptosis-related lncRNAs between the EC and control tissues (P < 0.05).
Identification of 9 Pyroptosis-Related lncRNAs Prognostic Signature for EC
Based on the survival information of EC patients, univariate Cox regression was applied to screen the expression profiles of the 170 lncRNAs related to pyroptosis. Nineteen differentially expressed and survival-related lncRNAs were determined based on p < 0.05 (). By multiple Cox regression analysis, 9 lncRNAs (AC087491.1, AL353622.1, AL035530.2, LINC02036, AL021578.1, AL390195.2, AC009097.2, AC004585.1, and AC244517.7) were further identified for the prognostic signature (). The regulatory network for these pyroptosis-related lncRNAs and pyroptosis-related genes (including GSDMB, PJVK, SCAF11, NLRP3, and AIM2) was constructed and visualized in .
Table 1 The Expression Levels of These 9 lncRNAs
Constructions and Validations of a Risk Signature Based on Pyroptosis-Related lncRNAs
We used least absolute shrinkage and selection operator (LASSO)‐penalized cox regression model to construct a prognostic model based on the 9 pyroptosis-related lncRNAs. The risk score for each sample was calculated based on the expression levels of these 9 lncRNAs. Risk score = 0.27037 * AC087491.1 - 0.08116 * AL353622.1 + 1.17813 * AL035530.2 - 0.13151 * LINC02036 + 0.19490 * AL021578.1 - 0.74903 * AL390195.2 - 0.41496 * AC009097.2 - 0.43031 * AC004585.1 + 0.41271 * AL3 AC244517.7 (). 542 samples were defined as an entire cohort, which was randomly divided into a training cohort (379 samples) and a testing cohort (163 samples) according the ratio of 7:3. EC samples were divided into high- and low- groups according to the median risk score.
In the training cohort, compared with those in the high-risk group, patients in the low-risk group showed a lower rate of death and a higher rate of survival time (–). The survival probability of patients in the two groups showed a significant difference (, P < 0.001). We employed the time-dependent receiver operating characteristic (ROC) curve to evaluate the predictive role of risk scores on overall survival (OS). The area under the curve (AUC) for 1 year, 2 years, 3 years was 0.693, 0.694, 0.750, respectively (). We further used the multi-indicator ROC curve analysis to contrast the predictive efficacy between the risk score and the clinical features. We found that the predictive accuracy of our risk model was the best compared with age and tumor grade (AUC: 0.693, 0.576, and 0.657; respectively. ). We validated the prognostic value of this risk signature in both the testing and entire cohorts. The distributions of expression of 9 lncRNAs, risk score, and survival status in the testing and entire groups were shown in – and –. The survival probability of patients in the two risk groups showed a significant difference in both testing (P = 0.002) and entire cohorts (P < 0.001) ( and ). The ROC analysis showed satisfactory prognostic accuracy in the testing () and entire sets (). Additionally, our risk model behaved better in predictive accuracy than age and tumor grade in the testing () and entire sets ().
Figure 2 Identification of the pyroptosis-related lncRNA signature in the training cohort. (A) Distributions of related lncRNAs in the signature. (B) Distribution of patients based on the median risk score. (C) The survival status for each patient. (D) Kaplan–Meier curves for the EC of patients in the high- and low-risk groups. (E) The AUC for the prediction of 1, 3, 5-year survival rate of EC. (F) Multi-indicator ROC curves for risk score, age, and tumor grade.
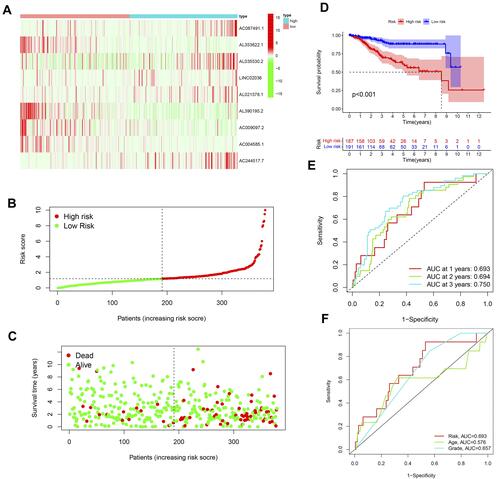
Figure 3 Identification of the pyroptosis-related lncRNA signature in the testing cohort. (A) Distributions of related lncRNAs in the signature. (B) Distribution of patients based on the median risk score. (C) The survival status for each patient. (D) Kaplan–Meier curves for the EC of patients in the high- and low-risk groups. (E) The AUC for the prediction of 1, 3, 5-year survival rate of EC. (F) Multi-indicator ROC curves for risk score, age, and tumor grade.
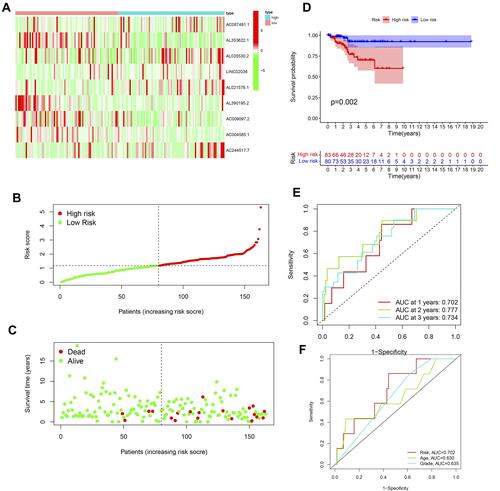
Figure 4 Identification of the pyroptosis-related lncRNA signature in the entire cohort. (A) Distributions of related lncRNAs in the signature. (B) Distribution of patients based on the median risk score. (C) The survival status for each patient. (D) Kaplan–Meier curves for the EC of patients in the high- and low-risk groups. (E) The AUC for the prediction of 1, 3, 5-year survival rate of EC. (F) Multi-indicator ROC curves for risk score, age, and tumor grade.
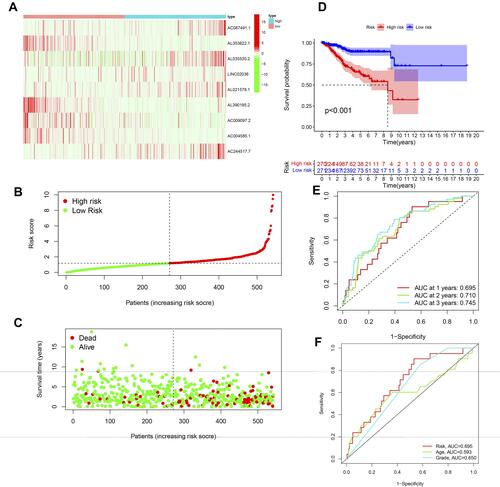
Independent Prognostic Value of the 9-lncRNAs Signature
The risk score was a reliable independent risk factor connected with OS (P < 0.001, HR:2.172, 95% CI:1.532–3.079) ( and ). The result was validated in both the testing ( and ) and entire ( and ) cohorts.
Figure 5 Cox regression analysis was used to assess the independent prognostic value of the risk score. Training cohort: (A) univariate cox regression analysis, (B) multivariate Cox regression analysis. Testing cohort: (C) univariate cox regression analysis, (D) multivariate cox regression analysis. Entire cohort: (E) univariate cox regression analysis, (F) multivariate cox regression analysis.
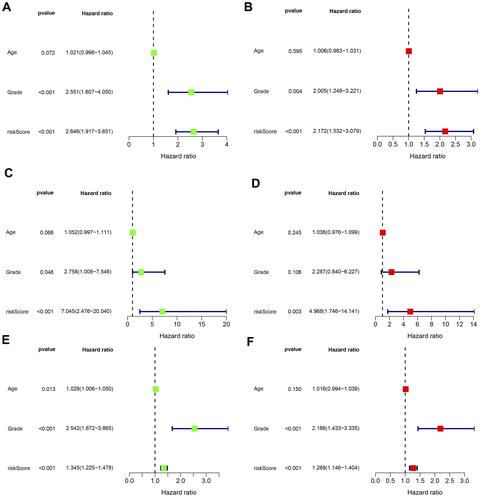
Immunity Expression Based on the Risk Model
There was a significant difference between the 2 groups in the expression of immune checkpoints (). Correlation analysis between immune cell subpopulations and related functions based on ssGSEA showed that co-stimulation antigen-presenting cells (APC), chemokine receptors (CCR), checkpoint, cytolytic-activity, human leukocyte antigen (HLA), regulation of inflammation, co-stimulation and co-inhibition of T cells, type I and type II IFN response were significantly different between the low-risk and high-risk groups ().
Immunotherapy Assessment of the Pyroptosis-Related lncRNAs Signature
In our risk model, the relative probabilities of response to anti-PD-1 and anti-CTLA-4 treatments in the low-risk group were higher compared with those in the high-risk group (p < 0.05) (–). The risk scores in the high-frequency microsatellite instability (MSI-H) group were lower than that in the microsatellite stability (MSS) group (p < 0.05) (). No significant difference was found between neither the low-frequency microsatellite instability (MSI-L) group and MSS group nor the MSI-H group and MSI-L group ().
Figure 7 The immunotherapy evaluation based on the risk score. (A) With negative CTLA4 and positive PD-1, (B) with positive CTLA4 and negative PD-1, (C) with positive CTLA4 and positive PD-1, (D) risk scores in different microsatellite statuses.
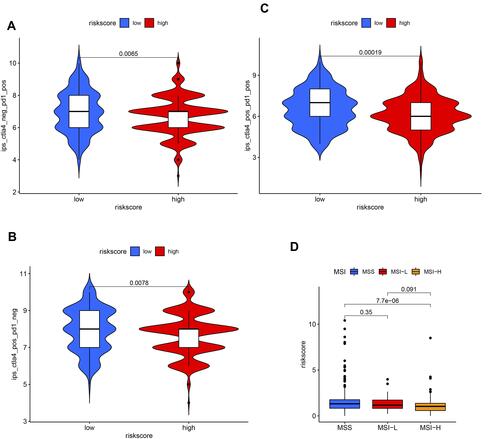
Discussion
In the present study, a prognostic signature was developed based on long non-coding RNAs (lncRNAs) related to pyroptosis using The Cancer Gene Atlas (TCGA) dataset. This signature consisted of 9 pyroptosis-related lncRNAs. Among the 9 lncRNAs, AC004585.1 has been reported to predict the outcomes of patients with breast cancer.Citation27 However, there is a lack of detailed information for other lncRNAs.
The development of risk models might be beneficial to predict the prognosis of various cancers. More and more studies try to establish signatures based on lncRNAs and improve the clinical outcome of related diseases. For example, lncRNAs can provide valuable information in diagnosis and prognosis and offers predictive value in melanoma. In particular, levels of UCA1 and MALAT-1 are significantly higher in patients with melanoma and are correlated to the stage of the disease.Citation28 In a pan-cancer study, a signature based on 5 lncRNAs was found to behave well in predicting survival outcomes of cancers based on the TCGA, TARGET, and National Cancer Institute cohorts.Citation29 After exploring the lncRNAs in colorectal cancer, HOXA11-AS, MEG3, SLCO4A1-AS1, SPINT1-AS1, and DANCR lncRNAs were discovered strong power for the diagnosis.Citation30 Four-methylated LncRNAs were considered as biomarkers for predicting the survival of osteosarcoma.Citation31 The prognosis of diffuse large B‐cell lymphoma might be predicted by a signature based on 6 lncRNAs.Citation32 In bladder cancer of TCGA cohort, 7 lncRNAs related to immune could be used to predict the prognosis of patients with bladder cancer, and the immune statuses were different in 2 risk groups.Citation33 Besides, up-regulated lncRNA ZFAS1 was connected with increased cell proliferation and epithelial-mesenchymal transition, and it could be a poor prognostic indicator for endometrial carcinoma (EC).Citation34 Similarly, the risk model based on 9 pyroptosis-related lncRNAs could be considered as an independent prognostic marker for the prognosis of EC, and the predictive accuracy of the risk model was superior to age and tumor grade.
The tumor microenvironment (TME) is comprised of extracellular matrix, fibroblasts, endothelial cells, neurons, and multiple immune cells.Citation35 Among them, the Interaction between lymphoma cells and the TME is essential for the survival and proliferation of a large number of tumors.Citation36 In recent years, people have been trying to find novel treatment methods for EC. To our delight, immunotherapy brings new insight into it. Growing evidence shows that the status of tumor-infiltrating lymphocytes (TILs) plays important roles in the prognostic and has potentially predictive significance for various tumor types. A chronic inflammatory reaction represented by TILS and plasma cells is associated with an improved prognosis of the malignant mesothelioma that could be responsive to immunotherapy.Citation37 Clinical outcomes of EC were found improved with the increased tumor-infiltrating of CD8(+), FoxP3(+), and CD45R0(+) T-lymphocytes.Citation38 The infiltrated number of intraepithelial CD8(+) T, or CD3(+) lymphocytes at the invasive border was considered an independent prognostic factor of survival for EC patients.Citation39,Citation40 Identification of immune cells in the EC is beneficial for prognosis and clinical improvement. In the present risk model, different prognoses were found and various cellular components were shown in the high-and low-risk groups, which might be applied to guide clinical diagnosis and treatment.
ECs are genetically divided into DNA polymerase epsilon (POLE), high-frequency microsatellite instability (MSI-H), copy number high, and copy number low groups.Citation41 In 27 types of cancers, EC was found with the highest microsatellite instability (MSI) by using an MSI-calling software.Citation42 Overexpressed PD-1 and PD-L1 were found in ultramutated polymerase e and microsatellite instability (MSI) ECs, which were accompanied by high neoantigen loads and a large number of tumor-infiltrating lymphocytes.Citation43 That makes ECs with POLE-mutation and MSI suitable for immunotherapies. Immune checkpoint therapy (ICT) has been developed as a novel treatment method, including monotherapy, combination with cytotoxic chemotherapy, other immunotherapy, or targeted agents.Citation44 The immune tolerance in ECs with a high mutation rate or a high mutation burden is less likely to occur, which makes they are more easily recognized and targeted by ICT-induced T cells. In our risk signature, the relative probabilities of response to ICT treatment in the low-risk group were higher than those in the high-risk group. At the same time, the risk scores in the high-frequency microsatellite instability (MSI-H) group were lower than those in the microsatellite stability (MSS) group. The results implied that patients with EC in the low-risk group might be with ideal targets for Immunotherapy.
There are some limits to the present study. First, our signature needs further validation by prospective large-scale randomized controlled studies with more clinical samples in the future. Second, work to explore the potential functions of pyroptosis-related lncRNAs is needed to explain the mechanisms of those lncRNAs in EC.
Conclusion
In summary, 9 pyroptosis-related lncRNAs were found useful in predicting the prognosis and immunotherapy of EC. The risk signature based on the 9 pyroptosis-related lncRNAs could well class EC patients and may be applied in guiding immunotherapy for patients with EC.
Data Sharing Statement
All data can be acquired from the corresponding author.
Ethics Approval and Consent to Participate
Our study had been reviewed by the Ethics Committee of the First Affiliated Hospital of Chongqing Medical University. Ethical approval and informed consent were waived.
Disclosure
The authors declare that they have no competing interests.
Additional information
Funding
References
- Sung H, Ferlay J, Siegel RL, et al. Global cancer statistics 2020: GLOBOCAN estimates of incidence and mortality worldwide for 36 cancers in 185 countries. CA Cancer J Clin. 2021;71(3):209–249. doi:10.3322/caac.21660
- Lewin SN, Herzog TJ, Barrena Medel NI, et al. Comparative performance of the 2009 international Federation of gynecology and obstetrics’ staging system for uterine corpus cancer. Obstet Gynecol. 2010;116(5):1141–1149. doi:10.1097/AOG.0b013e3181f39849
- Degez M, Caillon H, Chauviré-Drouard A, et al. Endometrial cancer: a systematic review of HE4, REM and REM-B. Clin Chim Acta. 2021;515:27–36. doi:10.1016/j.cca.2020.12.029
- Espiau Romera A, Coronado Martín PJ, Chóliz Ezquerro M, Cuesta Guardiola T, Adiego Calvo I, Baquedano Mainar L. Value of preoperative HE4 as predictor of advanced disease in endometrioid endometrial cancer. Int J Gynaecol Obstet. 2021;153(1):64–70. doi:10.1002/ijgo.13473
- Jorgensen I, Miao EA. Pyroptotic cell death defends against intracellular pathogens. Immunol Rev. 2015;265(1):130–142. doi:10.1111/imr.12287
- Wang F, Li G, Ning J, et al. Alcohol accumulation promotes esophagitis via pyroptosis activation. Int J Biol Sci. 2018;14(10):1245–1255. doi:10.7150/ijbs.24347
- Wang Y, Yin B, Li D, Wang G, Han X, Sun X. GSDME mediates caspase-3-dependent pyroptosis in gastric cancer. Biochem Biophys Res Commun. 2018;495(1):1418–1425. doi:10.1016/j.bbrc.2017.11.156
- Pizato N, Luzete BC, Kiffer L, et al. Omega-3 docosahexaenoic acid induces pyroptosis cell death in triple-negative breast cancer cells. Sci Rep. 2018;8(1):1952. doi:10.1038/s41598-018-20422-0
- Boussios S, Karathanasi A, Cooke D, et al. PARP inhibitors in ovarian cancer: the route to “Ithaca”. Diagnostics. 2019;9(2):55. doi:10.3390/diagnostics9020055
- Wang KC, Chang HY. Molecular mechanisms of long noncoding RNAs. Mol Cell. 2011;43(6):904–914. doi:10.1016/j.molcel.2011.08.018
- Wapinski O, Chang HY. Long noncoding RNAs and human disease. Trends Cell Biol. 2011;21(6):354–361. doi:10.1016/j.tcb.2011.04.001
- Wang X, Ren Y, Yang X, et al. miR-190a inhibits epithelial-mesenchymal transition of hepatoma cells via targeting the long non-coding RNA treRNA. FEBS Lett. 2015;589(24 Pt B):4079–4087. doi:10.1016/j.febslet.2015.11.024
- Ma Y, Chen Y, Lin C, Hu G. Biological functions and clinical significance of the newly identified long non‑coding RNA RP1‑85F18.6 in colorectal cancer. Oncol Rep. 2018;40(5):2648–2658. doi:10.3892/or.2018.6694
- Li J, Yang C, Li Y, Chen A, Li L, You Z. LncRNA GAS5 suppresses ovarian cancer by inducing inflammasome formation. Biosci Rep. 2018;38(2). doi:10.1042/bsr20171150
- Chen G, Zhang M, Liang Z, et al. Association of polymorphisms in MALAT1 with the risk of endometrial cancer in Southern Chinese women. J Clin Lab Anal. 2020;34(4):e23146. doi:10.1002/jcla.23146
- Gu ZR, Liu W. The lncRNA AL161431.1 targets miR-1252-5p and facilitates cellular proliferation and migration via MAPK signaling in endometrial carcinoma. Eur Rev Med Pharmacol Sci. 2020;24(5):2294–2302. doi:10.26355/eurrev_202003_20495
- Wang J, Li Z, Wang X, Ding Y, Li N. The tumor suppressive effect of long non-coding RNA FRMD6-AS2 in uteri corpus endometrial carcinoma. Life Sci. 2020;243:117254. doi:10.1016/j.lfs.2020.117254
- Yang W, Yue Y, Yin F, Qi Z, Guo R, Xu Y. LINC01133 and LINC01243 are positively correlated with endometrial carcinoma pathogenesis. Arch Gynecol Obstet. 2021;303(1):207–215. doi:10.1007/s00404-020-05791-0
- Zhang C, Shao S, Zhang Y, et al. LncRNA PCAT1 promotes metastasis of endometrial carcinoma through epigenetical downregulation of E-cadherin associated with methyltransferase EZH2. Life Sci. 2020;243:117295. doi:10.1016/j.lfs.2020.117295
- Zhao X, Zhang C, Wei X, et al. CARLo-5 as an oncogenic gene in endometrial carcinoma. Environ Mol Mutagen. 2020;61(2):256–265. doi:10.1002/em.22340
- Zhu W, Ye Z, Chen L, Liang H, Cai Q. A pyroptosis-related lncRNA signature predicts prognosis and immune microenvironment in head and neck squamous cell carcinoma. Int Immunopharmacol. 2021;101(Pt B):108268. doi:10.1016/j.intimp.2021.108268
- Karki R, Kanneganti TD. Diverging inflammasome signals in tumorigenesis and potential targeting. Nat Rev Cancer. 2019;19(4):197–214. doi:10.1038/s41568-019-0123-y
- Man SM, Kanneganti TD. Regulation of inflammasome activation. Immunol Rev. 2015;265(1):6–21. doi:10.1111/imr.12296
- Wang B, Yin Q. AIM2 inflammasome activation and regulation: a structural perspective. J Struct Biol. 2017;200(3):279–282. doi:10.1016/j.jsb.2017.08.001
- Xia X, Wang X, Cheng Z, et al. The role of pyroptosis in cancer: pro-cancer or pro-”host”? Cell Death Dis. 2019;10(9):650. doi:10.1038/s41419-019-1883-8
- Charoentong P, Finotello F, Angelova M, et al. Pan-cancer immunogenomic analyses reveal genotype-immunophenotype relationships and predictors of response to checkpoint blockade. Cell Rep. 2017;18(1):248–262. doi:10.1016/j.celrep.2016.12.019
- Lai J, Chen B, Zhang G, Li X, Mok H, Liao N. Molecular characterization of breast cancer: a potential novel immune-related lncRNAs signature. J Transl Med. 2020;18(1):416. doi:10.1186/s12967-020-02578-4
- Revythis A, Shah S, Kutka M, et al. Unraveling the wide spectrum of melanoma biomarkers. Diagnostics. 2021;11(8):1341. doi:10.3390/diagnostics11081341
- Bao G, Xu R, Wang X, et al. Identification of lncRNA signature associated with pan-cancer prognosis. IEEE J Biomed Health Inform. 2021;25(6):2317–2328. doi:10.1109/jbhi.2020.3027680
- Ghafouri-Fard S, Hussen BM, Gharebaghi A, Eghtedarian R, Taheri M. LncRNA signature in colorectal cancer. Pathol Res Pract. 2021;222:153432. doi:10.1016/j.prp.2021.153432
- Deng Y, Yuan W, Ren E, Wu Z, Zhang G, Xie Q. A four-methylated LncRNA signature predicts survival of osteosarcoma patients based on machine learning. Genomics. 2021;113(1 Pt 2):785–794. doi:10.1016/j.ygeno.2020.10.010
- Gao H, Wu B, Jin H, Yang W. A 6-lncRNA signature predicts prognosis of diffuse large B-cell lymphoma. J Biochem Mol Toxicol. 2021;35(6):1–12. doi:10.1002/jbt.22768
- Wang J, Shen C, Dong D, Zhong X, Wang Y, Yang X. Identification and verification of an immune-related lncRNA signature for predicting the prognosis of patients with bladder cancer. Int Immunopharmacol. 2021;90:107146. doi:10.1016/j.intimp.2020.107146
- Sun Y, Gao X, Li P, Song L, Shi L. LncRNA ZFAS1, as a poor prognostic indicator, promotes cell proliferation and epithelial-mesenchymal transition in endometrial carcinoma. Per Med. 2021;18(1):43–53. doi:10.2217/pme-2020-0014
- Hessmann E, Buchholz SM, Demir IE, et al. Microenvironmental determinants of pancreatic cancer. Physiol Rev. 2020;100(4):1707–1751. doi:10.1152/physrev.00042.2019
- Menter T, Tzankov A, Dirnhofer S. The tumor microenvironment of lymphomas: insights into the potential role and modes of actions of checkpoint inhibitors. Hematol Oncol. 2021;39(1):3–10. doi:10.1002/hon.2821
- Boussios S, Moschetta M, Karathanasi A, et al. Malignant peritoneal mesothelioma: clinical aspects, and therapeutic perspectives. Ann Gastroenterol. 2018;31(6):659–669. doi:10.20524/aog.2018.0305
- de Jong RA, Leffers N, Boezen HM, et al. Presence of tumor-infiltrating lymphocytes is an independent prognostic factor in type I and II endometrial cancer. Gynecol Oncol. 2009;114(1):105–110. doi:10.1016/j.ygyno.2009.03.022
- Čermáková P, Melichar B, Tomšová M, et al. Prognostic significance of CD3+ tumor-infiltrating lymphocytes in patients with endometrial carcinoma. Anticancer Res. 2014;34(10):5555–5561.
- Kondratiev S, Sabo E, Yakirevich E, Lavie O, Resnick MB. Intratumoral CD8+ T lymphocytes as a prognostic factor of survival in endometrial carcinoma. Clin Cancer Res. 2004;10(13):4450–4456. doi:10.1158/1078-0432.Ccr-0732-3
- Kandoth C, Schultz N, Cherniack AD, et al. Integrated genomic characterization of endometrial carcinoma. Nature. 2013;497(7447):67–73. doi:10.1038/nature12113
- Bonneville R, Krook MA, Kautto EA, et al. Landscape of microsatellite instability across 39 cancer types. JCO Precis Oncol. 2017;2017. doi:10.1200/po.17.00073
- Howitt BE, Shukla SA, Sholl LM, et al. Association of polymerase e-mutated and microsatellite-instable endometrial cancers with neoantigen load, number of tumor-infiltrating lymphocytes, and expression of PD-1 and PD-L1. JAMA Oncol. 2015;1(9):1319–1323. doi:10.1001/jamaoncol.2015.2151
- Green AK, Feinberg J, Makker V. A review of immune checkpoint blockade therapy in endometrial cancer. Am Soc Clin Oncol Educ Book. 2020;40:1–7. doi:10.1200/edbk_280503