Abstract
Purpose
Dilated cardiomyopathy (DCM) is a type of cardiomyopathy that can easily cause heart failure and has a high mortality rate. Therefore, there is an urgent need to study the underlying mechanism of action of dilated cardiomyopathy. In the present study, we aimed to explore potential miRNA–mRNA pairs and drugs related to DCM.
Methods
The Microarray data were collected from the Gene Expression Omnibus (GEO) database. Bioinformatics analysis differentially expressed miRNAs and mRNAs in each microarray were obtained. The target genes of miRNAs were obtained from the miRWalk 2.0 database, and the intersection of these two gene sets (miRNA target genes and differentially expressed mRNAs in the microarray) was obtained. Pathway and Gene Ontology (GO) enrichment analyses were performed in the KOBAS database. Cytoscape software was used to construct the miRNA–mRNA network, and the final hub genes were obtained. Furthermore, we predicted several candidate drugs related to hub genes using DSigDB database. To confirm the abnormal expression of hub genes, qRT-PCR was performed.
Results
In total, eight differentially expressed miRNAs and 92 differentially expressed mRNAs were identified. In addition, 47 differentially expressed miRNA target genes were identified. According to the analysis results of the miRNA-mRNA network, we identified hsa-miR-551b-3p, hsa-miR-770-5p, hsa-miR-363-3p, PIK3R1, DDIT4, and CXCR4 as hub genes in DCM. Several candidate drugs, which are related to the hug genes, were identified.
Conclusion
In conclusion, in our study, we identified several hub genes that may be involved in the pathogenesis of DCM. Several drugs related to these hub genes may be used as clinical therapeutic candidates.
Introduction
Dilated cardiomyopathy (DCM) is a primary myocardial disease with an undetermined cause. DCM is characterized by enlargement of the left or right ventricle or bilateral ventricles with a hypo-systolic ventricular function with or without congestive heart failure. Ventricular or atrial arrhythmias are common. DCM is progressive and can result in death at any stage of the disease.Citation1–Citation3 Because of the unclear mechanism underlying DCM, patients are not be treated in time, and the treatment approaches available are very limited.Citation4 Thus, it is necessary to study and identify novel biomarkers, which are of great interest in the diagnosis and preventive treatment of DCM.
MicroRNAs (miRNAs) are approximately 18–25 nucleotide-long endogenously expressed non-coding single-stranded RNAs that specifically regulate the expression of target genes.Citation5 miRNAs have been identified to be altered in cardiac disease.Citation6 It has also been reported that there is a specific relationship between the dysregulation of miRNAs and cardiac development and heart failure.Citation7–Citation9 Paweł Rubiś et al found that miR-133a is related to cardiovascular events (DCM).Citation10 Furthermore, Kenta Hirai et al confirmed that cardiosphere-derived exosomal miRNAs are related to DCM.Citation11 In previous studies, it was elucidated that the 3’-UTR of miRNAs can be bound by multiple RISC complexes, which may lead to synergistic co-repression of the target mRNA. Moreover, it has been found that complexes on the 3’-UTR of miRNAs may alter the structural organization of the target mRNAs, thereby affecting the progression of various Diseases.Citation12,Citation13
With the development of public databases, the gene chip microarray is an open-access database that was used to further investigate the biological process and function of various diseases. In the present study, 3 microarrays were chosen from the Gene Expression Omnibus (GEO) database to analyze the differentially expressed genes, gene-gene interaction, pathway, and GO enrichment in DCM. In addition, we also predicted several target genes and drugs for the treatment of DCM. The flow chart of the study is presented in .
In our study, we observed differentially expressed miRNAs (DEMis) and mRNAs (DEMs) in DCM tissues and normal tissues. Therefore, we hypothesized that DEMis and DEMs may be involved in the pathogenesis of DCM. To explore the underlying mechanism of DCM, difference analysis and functional analysis were performed. Experiments were performed to verify the differential expression of hub genes. The prediction of drugs in DCM may be a good reference in future studies. Thus, the data obtained in the present study may help find novel biomarkers and therapeutic targets for DCM.
Materials and Methods
Data Resources and Processing
Microarray data from miRNA (GSE112556) and mRNA (GSE29819 and GSE84796) were obtained from the public GEO database (http://www.ncbi.nlm.nih.gov/geo/). The miRNA dataset (GSE112556) contained 3 DCM tissues and 3 normal tissues, and the expression matrix was normalized by the robust multichip average (RMA) algorithm (Supplement Figure 1). The GSE29819 dataset contained 14 DCM tissues and 12 normal tissues was analyzed with MAS 5.0 using Affymetrix default analysis settings and global scaling as the normalization method (Supplement Figure 2). The GSE84796 dataset contained 10 DCM tissues and 7 normal tissues was analyzed and normalized using GeneSpring software (11.5.1) (Supplement Figure 3).
Screening of Differentially Expressed Genes (DEG)
The “limma” R package was used to identify the differentially expressed miRNAs and mRNAs.Citation14 P < 0.01 and |log2FC| > 1 were the screening criteria for the GSE 112556, and the adjusted P < 0.05 and |log2FC| > 1 were the screening criteria for GSE21819 and GSE84796. The differentially expressed genes volcano plots were visualized by the “ggplot2” R package and hierarchical clustering heatmaps were visualized by “Heatmap” R packages.Citation15
Pathway and Gene Ontology Enrichment Analysis
For Pathway and GO enrichment analysis, the mRNAs were imported into the KOBAS 3.0 database (http://kobas.cbi.pku.edu.cn/). The pathway analysis terms from the Kyoto Encyclopedia of Genes and Genomes (KEGG) database were summarized by the KOBAS platform. P < 0.05 was considered statistically significant.
Construction of the miRNA-mRNA Network
The differentially expressed miRNAs were uploaded to the miRWalk v2.0 database for predicting the potential targets of miRNAs (http://mirwalk.umm.uni-heidelberg.de/).Citation16 Then, 39 mRNAs were obtained by taking the intersection between up-regulated miRNA targets (downregulated miRNA targets) and up-regulated DEMs (downregulated DEMs). To construct the initial miRNA-mRNA network, the miRNA-mRNA pairs were imported into Cytoscape software. All mRNAs were uploaded to the STRING database (https://string-db.org/), which was used to construct a protein-protein interaction network (PPI). The initial miRNA-mRNA and PPI network were merged into the final network.
Identification of Hub Genes
The Cytohubba plugin of the Cytoscape software was used to screen the top 10 hub genes of the miRNA-mRNA network using 5 different algorithms. The 5 algorithms were degree, betweenness, closeness, stress, and Maximum Neighborhood Component (MCC), respectively. The final hub genes were obtained by taking the intersection of the results of the 5 algorithms. A Venn diagram was drawn to exhibit the overlapping hub genes of the results of the 5 algorithms.
Construction of the Cardiomyocyte Injury Model
H9c2 cells were derived from rat embryonic heart tissue and could maintain their cardiac myogenic cell characteristics. For the myocardial injury model, H9c2 cells were cultured in Dulbecco’s Modified Eagle’s Medium (DMEM) containing Doxorubicin (DOX) (2μM) (Solaribio, Life Science, Beijing, China) for 24 hours.Citation17 The cardiomyocyte injury model was used for the following experiments (qRT-PCR).
Real-Time Quantitative Reverse Transcription PCR
Total cell RNA was extracted by using the TRIzol reagent (Invitrogen, CA, USA). Reverse transcription and the PCR process were performed according to the manufacturer’s instructions (Applied Biosystem, ThermoFisher Scientific, Shanghai, China). The mRNA primers were designed and synthesized by Sangon Biotech Co. Ltd. (Shanghai, China), and miRNA primers were synthesized by RiboBio Co. Ltd. (Guangzhou, China). The primer sequences are presented in Supplementary Table 1. The target genes were quantified relative to internal control using the 2−ΔCT algorithm (GAPDH/U6), and the differences between the different groups were confirmed.
Statistical Analysis
The Student’s t-test was used to analyze the significant difference between the two groups. The Benjamini and Hochberg method was used to calculate the adjusted P-value for controlling the error rate. An adjusted P-value <0.05 was considered statistically significant.
Results
Because of the development of various public databases and microarray analysis, our study aimed to identify novel biomarkers. Therefore, to explore the underlying mechanisms in DCM, difference analysis and enrichment analysis were performed. Moreover, the hub gene-related drugs that were predicted may help find several novel treatments. Experiments were performed to increase the reliability of these analyses. Furthermore, our study may serve as a reference for advancing the development of DCM treatments.
Differentially Expressed miRNAs in Dilated Cardiomyopathy
The miRNA data was downloaded from the GEO database, which included 3 DCM tissues and 3 normal heart tissues. The screening criteria were described as before (|log2FC| > 1, P < 0.01). In addition, compared to normal heart tissues, 2 up-regulated (hsa-miR-770-5p and has-miR-21-5p) and 6 downregulated miRNAs (hsa-miR-144-5p, hsa-miR-9-3p, hsa-miR-144-3p, hsa-miR-451a, hsa-miR-363-3p, hsa-miR-551b-3p) were identified in the DCM tissues. The 8 miRNAs are listed in . A volcano plot was drawn to show the differentially expressed miRNAs (DEMis) (), and a hierarchical clustering heatmap was drawn to show the expression level of DEMis ().
Table 1 Differentially Expressed miRNAs (DEMis)
Figure 2 The screening and functional annotation of DEMis in DCM. (A) The 8 DEMis are shown in the volcano plot (|log2FC| > 1, P-value < 0.01). (B) The heat map shows the expression level of differentially expressed miRNA. (C) The transcription factors enrichment analysis of DEMis in DCM. (D–F) The GO functional annotation of DEMis in DCM.
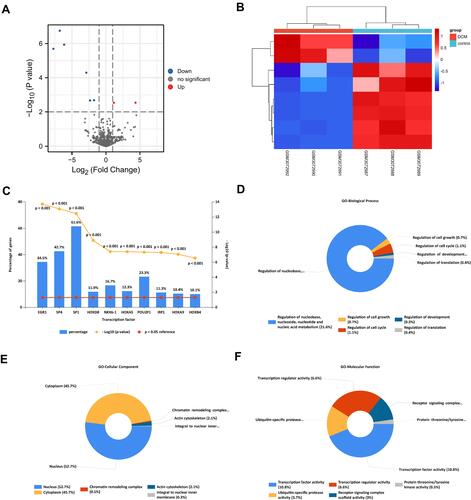
Functional and GO Annotation of DEMis
The 8 differentially expressed miRNAs (DEMis) were imported into FunRich 3.1.3 software. Next, transcription factors (TFs) enrichment analysis was performed to screen several TFs that were closely related to DEMis. The results showed that the top 10 TFs, including EGR1, SP4, SP1 HOXD8, NKX6-1, HOXA5, POU2F1, IRF1, HOXA9, and HOXB4 may have a regulatory relationship with DEMis (). Furthermore, GO enrichment analysis was performed to identify the biological functions of DEMis. The GO biological process terms indicated that miRNAs were mainly involved in the regulation of nucleobase, nucleoside, nucleotide and nucleic acid metabolism (21.6%) (). The GO cellular component terms showed that most miRNAs were located in the nucleus (52.7%) and the cytoplasm (45.7%) (). Furthermore, the GO Molecular Function terms indicated that miRNAs were mainly enriched in transcription factor activity (10.8%) ().
Differentially Expressed mRNAs in Dilated Cardiomyopathy
In this study, we chose two microarrays associated with DCM from the GEO database (GSE29819 and GSE84796). Then, the “limma” R package was used to analyze the different gene expressions (Supplement Tables 2 and 3). The screening criteria were as follows: |log2FC| > 1 and adjusted P < 0.05. As shown in , the volcano plot indicated that 209 up-regulated and 265 downregulated mRNAs were identified as differentially expressed RNAs in GSE29819. In addition, the volcano plot in was drawn to show the differentially expressed RNAs in GSE84796 (including 1059 up-regulated and 398 downregulated mRNAs). Moreover, the hierarchical clustering heatmap was drawn to show the expression level of DEMis ( and ). Finally, Venn diagrams were drawn to show the overlapping up-/down-regulated mRNAs (49 up-regulated and 43 downregulated mRNAs) between GSE29819 and GSE84796 ( and ).
Figure 3 The screening of DEMs in DCM. (A) The volcano plot was drawn to show the 209 up-regulated and 265 downregulated mRNAs in GSE29819 (|log2FC| > 1, adjusted P-value < 0.05). (B) The heatmap shows the expression level of DEMs in GSE29819. (C) The volcano plot was drawn to show the 1059 up-regulated and 398 downregulated mRNAs in GSE84796 (|log2FC| > 1, adjusted P-value < 0.05). (D) The heatmap shows the expression level of DEMs in GSE84796. (E and F) The Venn diagrams were drawn to show the overlapping up-/down-regulated mRNAs between GSE29819 and GSE84796.
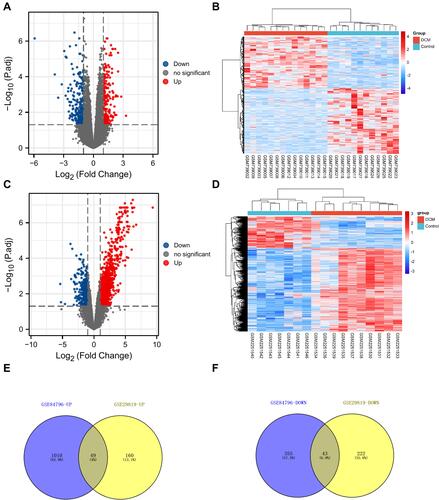
KEGG Pathway and GO Enrichment Analysis of DEMs
For investigating the underlying mechanism and function of DEMs, we uploaded 92 mRNAs to the KOBAS 3.0 database. For KEGG pathway enrichment analysis, we found that DEMs were mainly enriched in the neuroactive ligand-receptor interaction, estrogen signaling pathway, cytokine-cytokine receptor interaction, Jak-STAT signaling pathway, and pathways related to cancer, etc. A bubble plot was drawn to show the top 10 pathways from the results of KEGG analysis (). Using GO functional and enrichment analysis, DEMs were mainly located in the plasma membrane (GO:0005886), extracellular region (GO:0005576), cytoplasm (GO:0005737), extracellular space (GO:0005615), membrane (GO:0016020), and extracellular vesicles (GO:1903561). Besides, the biological process and molecular function of DEMs were mainly involved in protein binding (GO:0005515), collagen-containing extracellular matrix (GO:0062023), identical protein binding (GO:0042802), and G protein-coupled receptor signaling pathway (GO:0007186) ().
Construction of miRNA-mRNA Network and Identification of Hub Genes
In this study, we first identified 39 genes by taking the intersection between up-regulated miRNA targets (downregulated miRNA targets) and up-regulated DEMs (downregulated DEMs) (Supplement Figure 4). Next, the DEMis and DEMs were imported to construct the initial network. Moreover, DEMs were uploaded to the STRING database for constructing a protein-protein interaction network (PPI). The PPI network was also imported into the Cytoscape (version: 3.8.2) software. Subsequently, the initial network and PPI network were merged into the final miRNA-mRNA network using the Cytoscape software (). Hub genes were identified by using the Cytohubba plugin of the Cytoscape software. Next, we chose 5 algorithms to screen the hub genes of the PPI network. As shown in , the top 10 genes were identified by degree, MCC, betweenness, closeness, and stress. Lastly, 6 genes (hsa-miR-551b-3p, hsa-miR-770-5p, PIK3R1, DDIT4, CXCR4, and hsa-miR-363-3p) were identified as hub genes by taking the intersection of 5 algorithms in the Venn diagram () ().
Table 2 The Five Algorithmic Scores of Hub Genes
Figure 5 Construction of miRNA-mRNA network. (A) The network of DEMis and DEMs. (B) The top 10 hub genes from the results of Betweenness algorithms. (C) The top 10 hub genes from the results of Closeness algorithms. (D) The top 10 hub genes from the results of Degree algorithms. (E) The top 10 hub genes from the results of MCC algorithms. (F) The top 10 hub genes from the results of Stress algorithms. (G) The Venn diagram was drawn to show the overlapping hub genes in the results of 5 algorithms.
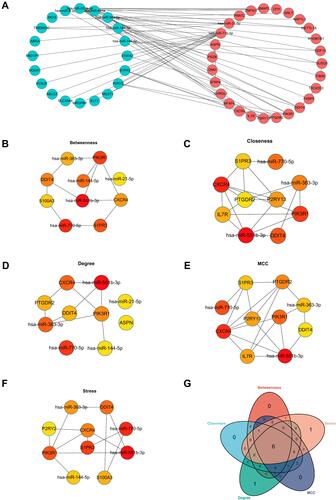
Prediction of Candidate Drugs
The DSigDB database was used to predict several drugs which are related to the 3 DEMs. The top 10 candidate drugs related to the DEMs were chosen according to the P-value and adjusted P-value. shows the top 10 candidate drugs from the DSigDB database and the 10 drugs are shown in . shows the structure of several drugs related to the hub gene.
Table 3 Suggested Top 10 Drugs Compounds for the DCM
Validation of Abnormal Hub Genes
To verify our results, we constructed a DOX-induced myocardial injury model of DCM and determined the expression of differential miRNAs using real-time PCR. The results are shown in . Compared with the control group, the expression of hsa-miR-363-3p and hsa-miR-551b-5p was down-regulated in DOX-treated H9c2 cells; however, the expression of hsa-miR-770-5p was significantly up-regulated (). In addition, the expression of Hub genes CXCR4, DDIT4 and PIK3R1 were significantly upregulated in H9c2 cells treated with DOX compared to the control group (). These findings were consistent with our predicted results.
Discussion
With the development of various public databases, microarray analysis has gradually become an optimal approach to identify differentially expressed genes that may affect the progression of various diseases.Citation18–Citation20 In recent years, there are an increasing number of studies related to gene chip analysis. This is because gene chip analysis not only helps to identify new biomarkers but also helps to predict treatments and prognostic factors in DCM.Citation21 To date, relatively few studies have been performed on DCM. By the time DCM is diagnosed, the disease is often at a stage when surgical treatment is necessary, so it is essential to identify predictive and diagnostic biomarkers for early diagnosis.
It has been reported that miRNAs are involved in various diseases, such as cancer,Citation22 cardiovascular diseases,Citation23–Citation25 and other diseases.Citation26–Citation28 We identified 3 miRNAs and 3 mRNAs as hub genes in DCM. Literature retrieved from PubMed showed several studies about the genes we identified before, but the underlying mechanisms of action and functions in DCM remain unclear. In previous studies, it has been shown that hsa-miR-551b may regulate the progression of lung adenocarcinoma and colorectal cancer.Citation29,Citation30 Feng et al found that hsa-miR-551b-5p was involved in diabetic cardiomyopathy.Citation31 Besides, the serum miR-551b-3p as a potential tumor suppressor was found to be downregulated in gastric cancer tissues, and it may predict the outcome of gastric cancer.Citation32,Citation33 Also, Chang et al revealed that miR-551b-3p inhibits the growth of cholangiocarcinoma cells via targeting Cyclin D1.Citation34 What’s more, Zhou et al reported that hsa-miR-363-3p can reduce endothelial cell inflammatory responses in coronary heart disease.Citation35 And has-miR-363-3p as an tumor suppressor affects the progression of multiple cancers, including osteosarcoma,Citation36 hepatocellular carcinoma,Citation37 papillary thyroid carcinoma,Citation38 and so on. All these studies suggest that has-miR-363-3p and hsa-miR-551b-3p have the function of inhibiting proliferation and affecting cell cycle. Therefore, these results provide some theoretical support for our study. And it reveals that the dysregulation of the two miRNAs may affect the iterative renewal of cells, leading to a bad phenotype of H9c2 cells. Then, Satake et al found that hsa-miR-770-5p was associated with hyperglycemia in patients with type 1 diabetes.Citation39 Lee et al also found that hsa-miR-770-5p can sensitize breast cancer to radiation.Citation40 And these two reports show that hsa-miR-770-5p may induce the death of cells. Therefore, studying miRNAs in DCM disease is feasible.
In addition to miRNAs, the hub mRNAs that we identified before have been studied in other diseases, which can be a reference for our research. The C-X-C motif chemokine receptor 4 (CXCR4) encodes a CXC chemokine receptor that is specific for stromal cell-derived factor-1 and can affect disease progression. Chu et al found that CXCR4 antagonism can reduce cardiac fibrosis and improve cardiac performance in DCM.Citation41 Besides, Damås et al elucidated that CXCR4 may enhance the progression of heart failure.Citation42 More importantly, Sara Beji found that CXCR4 expression is upregulated in DOX-induced cardiomyocyte injury.Citation43 DNA damage-inducible transcript 4 (DDIT4) was confirmed to promote gastric cancer and acute myeloid leukemia progression.Citation44,Citation45 Previous system analysis suggested that suppression of DDIT4 can preserve heart contraction.Citation46 Furthermore, DDIT4 also can control cell death in cardiac I/R injury by regulating several apoptosis-related signaling pathway.Citation47 In addition to cardiac diseases, relevant studies in other diseases have shown that DDIT4 mediates the apoptotic process, for example, Lynn M Knowles et al verified that increasing DDIT4 induces caspase-8 mediated tumor cell apoptosis via negatively regulating the mTOR signaling pathway.Citation48 And Li revealed that DDIT4 can promote the production of ROS in liver injury,Citation49 which suggests that the expression of DDIT4 is related to the injury of cells. The detailed functional mechanisms of DDIT4 in DCM remain unclear. DDIT4 as a novel mediator of cardiomyocyte apoptosis can be a good reference for our study and future studies. Phosphoinositide-3-kinase regulatory subunit 1 (PIK3R1) was identified as an oncogene in gastric cancer, breast cancer, and pancreatic cancer.Citation50–Citation52 Furthermore, PIK3R1 was found to be involved in the pathogenesis of coronary artery disease.Citation53 Yan et al have demonstrated that PIK3R1 can induce the suppression of invasiveness in breast cancer.Citation54 This means that the effect of PIK3R1 on the cells is controversial. And studies focused on PIK3R1 and DCM are still limited. However, Huang et al found that PIK3R1, which is up-regulated in DCM tissues, may be involved in the pathogenesis of DCM.Citation55 Therefore, the study of PIK3R1 in DCM may be an interesting and meaningful direction.
In the present study, we utilized bioinformatics analysis to predict potential biomarkers in DCM. Microarray analysis was used to screen DEMis and DEMs. Then, a miRNA- mRNA network was constructed, and hub genes were identified by using 5 algorithms. KEGG and GO enrichment analyses were performed to investigate the function and underlying mechanism of action of differentially expressed genes (DEGs). Moreover, the DSigDB database was used to predict several drugs, which are related to the DEGs.
Our study has some shortcomings. Due to the difficulty of obtaining samples in the short term, we only conducted simple cell-experimental validation, but we intend to conduct a further study at a later stage when we have sufficient funds and samples. Despite the shortcomings, the data obtained is of great significance for subsequent research.
Conclusion
In summary, we comprehensively obtained several differentially expressed genes (hsa-miR-551b-5p, hsa-miR-770-5p, hsa-miR-363-3p, CXCR4, DDIT4, and PIK3R1) and several drugs that may be associated with the pathogenesis of DCM.
Acknowledgment
We acknowledge GEO and other public databases for providing their platforms and contributors for uploading their meaningful datasets.
Disclosure
The authors report no conflicts of interest in this work.
Additional information
Funding
References
- Kayvanpour E, Sedaghat-Hamedani F, Amr A, et al. Genotype-phenotype associations in dilated cardiomyopathy: meta-analysis on more than 8000 individuals. Clin Res Cardiol. 2017;106:127–139. doi:10.1007/s00392-016-1033-6
- Mestroni L, Maisch B, McKenna WJ, et al. Guidelines for the study of familial dilated cardiomyopathies. Collaborative Research Group of the European human and capital mobility project on familial dilated cardiomyopathy. Eur Heart J. 1999;20:93–102. doi:10.1053/euhj.1998.1145
- Hershberger RE, Cowan J, Morales A, et al. Progress with genetic cardiomyopathies: screening, counseling, and testing in dilated, hypertrophic, and arrhythmogenic right ventricular dysplasia/cardiomyopathy. Circ Heart Fail. 2009;2:253–261. doi:10.1161/circheartfailure.108.817346
- Hunt SA, Abraham WT, Chin MH, et al. Focused update incorporated into the ACC/AHA 2005 guidelines for the diagnosis and management of heart failure in adults a report of the American College of Cardiology Foundation/American Heart Association task force on practice guidelines developed in collaboration with the international society for heart and lung transplantation. J Am Coll Cardiol. 2009;53:e1–e90. doi:10.1016/j.jacc.2008.11.013
- Bartel DP. MicroRNAs: genomics, biogenesis, mechanism, and function. Cell. 2004;116:281–297. doi:10.1016/s0092-8674(04)00045-5
- van Rooij E, Sutherland LB, Liu N, et al. A signature pattern of stress-responsive microRNAs that can evoke cardiac hypertrophy and heart failure. Proc Natl Acad Sci U S A. 2006;103:18255–18260. doi:10.1073/pnas.0608791103
- Cordes KR, Srivastava D. MicroRNA regulation of cardiovascular development. Circ Res. 2009;104:724–732. doi:10.1161/circresaha.108.192872
- van Rooij E, Olson EN. Searching for miR-acles in cardiac fibrosis. Circ Res. 2009;104:138–140. doi:10.1161/circresaha.108.192492
- Latronico MV, Condorelli G. MicroRNAs and cardiac pathology. Nat Rev Cardiol. 2009;6:419–429. doi:10.1038/nrcardio.2009.56
- Rubiś P, Totoń-żurańska J, Wiśniowska-śmiałek S, et al. The relationship between myocardial fibrosis and myocardial microRNAs in dilated cardiomyopathy: a link between mir-133a and cardiovascular events. J Cell Mol Med. 2018;22:2514–2517. doi:10.1111/jcmm.13535
- Hirai K, Ousaka D, Fukushima Y, et al. Cardiosphere-derived exosomal microRNAs for myocardial repair in pediatric dilated cardiomyopathy. Sci Transl Med. 2020;12. doi:10.1126/scitranslmed.abb3336
- Filipowicz W, Bhattacharyya SN, Sonenberg N. Mechanisms of post-transcriptional regulation by microRNAs: are the answers in sight? Nat Rev Genet. 2008;9:102–114. doi:10.1038/nrg2290
- Brennecke J, Stark A, Russell RB, et al. Principles of microRNA-target recognition. PLoS Biol. 2005;3:e85. doi:10.1371/journal.pbio.0030085
- Ritchie ME, Phipson B, Wu D, et al. limma powers differential expression analyses for RNA-sequencing and microarray studies. Nucleic Acids Res. 2015;43:e47. doi:10.1093/nar/gkv007
- Gu Z, Eils R, Schlesner M. Complex heatmaps reveal patterns and correlations in multidimensional genomic data. Bioinformatics. 2016;32:2847–2849. doi:10.1093/bioinformatics/btw313
- Dweep H, Gretz N. miRWalk2.0: a comprehensive atlas of microRNA-target interactions. Nat Methods. 2015;12:697. doi:10.1038/nmeth.3485
- Chen C, Jiang L, Zhang M, et al. Isodunnianol alleviates doxorubicin-induced myocardial injury by activating protective autophagy. Food Funct. 2019;10:2651–2657. doi:10.1039/c9fo00063a
- Ivandic BT, Mastitsky SE, Schönsiegel F, et al. Whole-genome analysis of gene expression associates the ubiquitin-proteasome system with the cardiomyopathy phenotype in disease-sensitized congenic mouse strains. Cardiovasc Res. 2012;94:87–95. doi:10.1093/cvr/cvs080
- Ceylan H. Identification of hub genes associated with obesity-induced hepatocellular carcinoma risk based on integrated bioinformatics analysis. Med Oncol. 2021;38:63. doi:10.1007/s12032-021-01510-0
- Ceylan H. Integrated bioinformatics analysis to identify alternative therapeutic targets for alzheimer’s disease: insights from a synaptic machinery perspective. J Mol Neurosci. 2021. doi:10.1007/s12031-021-01893-9
- Barth AS, Kuner R, Buness A, et al. Identification of a common gene expression signature in dilated cardiomyopathy across independent microarray studies. J Am Coll Cardiol. 2006;48:1610–1617. doi:10.1016/j.jacc.2006.07.026
- Rupaimoole R, Slack FJ. MicroRNA therapeutics: towards a new era for the management of cancer and other diseases. Nat Rev Drug Discov. 2017;16:203–222. doi:10.1038/nrd.2016.246
- Vegter EL, van der Meer P, de Windt LJ, et al. MicroRNAs in heart failure: from biomarker to target for therapy. Eur J Heart Fail. 2016;18:457–468. doi:10.1002/ejhf.495
- Zhou SS, Jin JP, Wang JQ, et al. miRNAS in cardiovascular diseases: potential biomarkers, therapeutic targets and challenges. Acta Pharmacol Sin. 2018;39:1073–1084. doi:10.1038/aps.2018.30
- Barwari T, Joshi A, Mayr M. MicroRNAs in cardiovascular disease. J Am Coll Cardiol. 2016;68:2577–2584. doi:10.1016/j.jacc.2016.09.945
- Simion V, Nadim WD, Benedetti H, et al. Pharmacomodulation of microRNA Expression in neurocognitive diseases: obstacles and future opportunities. Curr Neuropharmacol. 2017;15:276–290. doi:10.2174/1570159x14666160630210422
- Liu T, Zhang Q, Zhang J, et al. EVmiRNA: a database of miRNA profiling in extracellular vesicles. Nucleic Acids Res. 2019;47:D89–d93. doi:10.1093/nar/gky985
- Baskara-Yhuellou I, Tost J. The impact of microRNAs on alterations of gene regulatory networks in allergic diseases. Adv Protein Chem Struct Biol. 2020;120:237–312. doi:10.1016/bs.apcsb.2019.11.006
- Kim MH, Cho JS, Kim Y, et al. Discriminating between terminal- and non-terminal respiratory unit-type lung adenocarcinoma based on MicroRNA profiles. PLoS One. 2016;11:e0160996. doi:10.1371/journal.pone.0160996
- Han B, Feng D, Yu X, et al. Identification and interaction analysis of molecular markers in colorectal cancer by integrated bioinformatics analysis. Med Sci Monit. 2018;24:6059–6069. doi:10.12659/msm.910106
- Feng Y, Xu W, Zhang W, et al. LncRNA DCRF regulates cardiomyocyte autophagy by targeting miR-551b-5p in diabetic cardiomyopathy. Theranostics. 2019;9:4558–4566. doi:10.7150/thno.31052
- Bai SY, Ji R, Wei H, et al. Serum miR-551b-3p is a potential diagnostic biomarker for gastric cancer. Turk J Gastroenterol. 2019;30:415–419. doi:10.5152/tjg.2019.17875
- Yuan H, Chen Z, Bai S, et al. Molecular mechanisms of lncRNA SMARCC2/miR-551b-3p/TMPRSS4 axis in gastric cancer. Cancer Lett. 2018;418:84–96. doi:10.1016/j.canlet.2018.01.032
- Chang W, Wang Y, Li W, et al. MicroRNA-551b-3p inhibits tumour growth of human cholangiocarcinoma by targeting Cyclin D1. J Cell Mol Med. 2019;23:4945–4954. doi:10.1111/jcmm.14312
- Zhou T, Li S, Yang L, et al. microRNA-363-3p reduces endothelial cell inflammatory responses in coronary heart disease via inactivation of the NOX4-dependent p38 MAPK axis. Aging. 2021;13:11061–11082. doi:10.18632/aging.202721
- Wang K, Yan L, Lu F. miR-363-3p inhibits osteosarcoma cell proliferation and invasion via targeting SOX4. Oncol Res. 2019;27:157–163. doi:10.3727/096504018x15190861873459
- Wang J, Tang Q, Lu L, et al. LncRNA OIP5-AS1 interacts with miR-363-3p to contribute to hepatocellular carcinoma progression through up-regulation of SOX4. Gene Ther. 2019;27:495–504. doi:10.1038/s41434-020-0123-2
- Liu J, Li Q, Li R, et al. MicroRNA-363-3p inhibits papillary thyroid carcinoma progression by targeting PIK3CA. Am J Cancer Res. 2017;7:148–158.
- Satake E, Pezzolesi MG, Md Dom ZI, et al. Circulating miRNA profiles associated with hyperglycemia in patients with Type 1 diabetes. Diabetes. 2018;67:1013–1023. doi:10.2337/db17-1207
- Lee HC, Her NG, Kang D, et al. Radiation-inducible miR-770-5p sensitizes tumors to radiation through direct targeting of PDZ-binding kinase. Cell Death Dis. 2017;8:e2693. doi:10.1038/cddis.2017.116
- Chu PY, Joshi MS, Horlock D, et al. CXCR4 antagonism reduces cardiac fibrosis and improves cardiac performance in dilated cardiomyopathy. Front Pharmacol. 2019;10:117. doi:10.3389/fphar.2019.00117
- Damås JK, Eiken HG, Oie E, et al. Myocardial expression of CC- and CXC-chemokines and their receptors in human end-stage heart failure. Cardiovasc Res. 2000;47:778–787. doi:10.1016/s0008-6363(00)00142-5
- Beji S, Milano G, Scopece A, et al. Doxorubicin upregulates CXCR4 via miR-200c/ZEB1-dependent mechanism in human cardiac mesenchymal progenitor cells. Cell Death Dis. 2017;8:e3020. doi:10.1038/cddis.2017.409
- Du F, Sun L, Chu Y, et al. DDIT4 promotes gastric cancer proliferation and tumorigenesis through the p53 and MAPK pathways. Cancer Commun. 2018;38:45. doi:10.1186/s40880-018-0315-y
- Cheng Z, Dai Y, Pang Y, et al. Up-regulation of DDIT4 predicts poor prognosis in acute myeloid leukaemia. J Cell Mol Med. 2020;24:1067–1075. doi:10.1111/jcmm.14831
- Nishi M, Ogata T, Cannistraci CV, et al. Systems network genomic analysis reveals cardioprotective effect of MURC/Cavin-4 deletion against ischemia/reperfusion injury. J Am Heart Assoc. 2019;8:e012047. doi:10.1161/jaha.119.012047
- Eltzschig HK, Eckle T. Ischemia and reperfusion–from mechanism to translation. Nat Med. 2011;17:1391–1401. doi:10.1038/nm.2507
- Knowles LM, Yang C, Osterman A, et al. Inhibition of fatty-acid synthase induces caspase-8-mediated tumor cell apoptosis by up-regulating DDIT4. J Biol Chem. 2008;283:31378–31384. doi:10.1074/jbc.M803384200
- Li Z, Zhao Q, Lu Y, et al. DDIT4 S-Nitrosylation aids p38-MAPK signaling complex assembly to promote hepatic reactive oxygen species production. Adv Sci. 2021;8:e2101957. doi:10.1002/advs.202101957
- Huang X, Li Z, Zhang Q, et al. Circular RNA AKT3 upregulates PIK3R1 to enhance cisplatin resistance in gastric cancer via miR-198 suppression. Mol Cancer. 2019;18:71. doi:10.1186/s12943-019-0969-3
- Kong Y, Li Y, Luo Y, et al. circNFIB1 inhibits lymphangiogenesis and lymphatic metastasis via the miR-486-5p/PIK3R1/VEGF-C axis in pancreatic cancer. Mol Cancer. 2020;19:82. doi:10.1186/s12943-020-01205-6
- Kim S, Lee E, Jung J, et al. microRNA-155 positively regulates glucose metabolism via PIK3R1-FOXO3a-cMYC axis in breast cancer. Oncogene. 2018;37:2982–2991. doi:10.1038/s41388-018-0124-4
- Miao L, Yin RX, Zhang QH, et al. Integrated DNA methylation and gene expression analysis in the pathogenesis of coronary artery disease. Aging. 2019;11:1486–1500. doi:10.18632/aging.101847
- Yan LX, Liu YH, Xiang JW, et al. PIK3R1 targeting by miR-21 suppresses tumor cell migration and invasion by reducing PI3K/AKT signaling and reversing EMT, and predicts clinical outcome of breast cancer. Int J Oncol. 2016;48:471–484. doi:10.3892/ijo.2015.3287
- Huang K, Wen S, Huang J, et al. Integrated analysis of hub genes and miRNAs in dilated cardiomyopathy. Biomed Res Int. 2020;2020:8925420. doi:10.1155/2020/8925420