Abstract
Purpose
Although considerable progress has been made in basic and clinical research on nasopharyngeal carcinoma (NPC), the biomarkers of the progression of NPC have not been fully studied and described. This study was designed to identify potential novel biomarkers for NPC using integrated analyses and explore the immune cell infiltration in this pathological process.
Methods
Five GEO data sets were downloaded from gene expression omnibus database (GEO) and analysed to identify differentially expressed genes (DEGs), followed by Gene Ontology (GO) and Kyoto Encyclopedia of Genes and Genomes (KEGG) enrichment analyses. The four algorithms were adopted for screening of novel and key biomarkers for NPC, including random forest (RF) machine learning algorithm, least absolute shrinkage and selection operator (LASSO) logistic regression, support vector machine-recursive feature elimination (SVM-RFE), and weighted gene co-expression network analysis (WGCNA). Lastly, CIBERSORT was used to assess the infiltration of immune cells in NPC, and the correlation between diagnostic markers and infiltrating immune cells was analyzed.
Results
Herein, we identified 46 DEGs, and enrichment analysis results showed that DEGs and several kinds of signaling pathways might be closely associated with the occurrence and progression of NPC. DTL was recognized as NPC-related biomarker. DTL, also known as retinoic acid-regulated nuclear matrix-associated protein (RAMP), or DNA replication factor 2 (CDT2), is reported to be correlated with the cell proliferation, cell cycle arrest and cell invasion in hepatocellular carcinoma, breast cancer and gastric cancer. Immune infiltration analysis demonstrated that macrophages M0, macrophages M1 and T cells CD4 memory activated were linked to pathogenesis of NPC.
Conclusion
In summary, we adopted a comprehensive strategy to screen DTL as biomarkers related to NPC and explore the critical role of immune cell infiltration in NPC.
Graphical Abstract
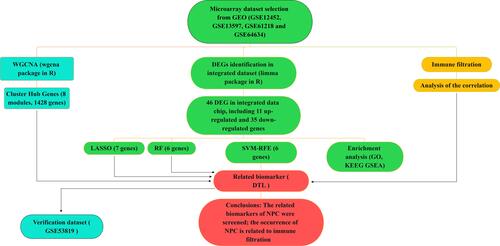
Introduction
Nasopharyngeal carcinoma (NPC) is a type of head and neck tumor with high invasion and metastasis originating from nasopharyngeal epithelial tissue. Although originating from similar cell or tissue lineages, NPC is significantly different from other epithelial head and neck tumors, characterized by early cervical lymph node metastasis and invasion of the base of the skull, with significant ethnic and geographic specificity, and the highest incidence of distant metastasis of NPC in head and neck tumors.Citation1–Citation3
Unfortunately, early-stage cancers can be asymptomatic, so biomarkers such as circulating cell-free Epstein–Barr virus (EBV) DNA are used to detect NPC in populations at risk for the disease.Citation4 Subjects with elevated plasma biomarkers are assessed by nasopharyngeal endoscopic examination. Those with an abnormality suspicious of NPC undergo endoscopic-guided biopsy for histological confirmation of NPC, whereas those without a suspicious abnormality are considered to have had a false-positive blood test. However, small tumors hidden in the pharyngeal recess, adenoid or beneath the mucosa can be missed on endoscopic examination and the number of such tumors in populations screened for NPC is unknown.Citation5–Citation8 Some studies had found that neoplastic spindle cells have features of epithelial mesenchymal transition (EMT) and cancer stem cells (CSCs), and should be considered as the more aggressive subtype in NPC, and the predictors of tumor cell dissemination and metastasis of patients.Citation9,Citation10 Although considerable progress has been achieved in basic and clinical research on NPC, the biomarkers of the progression of NPC is not fully studied and described. Thus, further investigation is beneficial, especially for identification of potential biomarkers to improve survival in patients for whom the NPC is in its early-stages.
With the development of sequencing technologies and microarray, we can easily screen the expression level of thousands of genes simultaneously in the human genome.Citation11 Comprehensive analysis of multiple datasets provides the capabilities to properly identify and assess the pathways and genes that mediate the biological processes associated with NPC. Machine learning (ML) is a rapidly advancing field of artificial intelligence (AI) that enables computer technology to learn from data to identify patterns and make predictions without explicit programming.Citation12 ML does not describe a single specific algorithm, but rather contains a variety of approaches that have to be modified to the addressed issue and data set. ML methods are typically classified as supervised learning, unsupervised learning, and reinforcement learning. The input file can be text, images, or anything that is digitally stored.Citation13 AI/ML techniques have been applied to various fields of biomedicine including novel target identification, understanding of target-disease associations, drug candidate selection, protein structure predictions, molecular compound design and optimization, understanding of disease mechanisms, development of new prognostic and predictive biomarkers, biometrics data analysis from wearable devices, imaging, precision medicine, and more recently clinical trial design, conduct, and analysis.Citation14,Citation15 To this end, we used microarray datasets of gene expression to assess the differentially expressed genes (DEGs) between NPC and normal nasopharyngeal tissue, then ML algorithm was used to screen biomarkers in DEGs for early identification of NPC.
Materials and Methods
Data Collection and Data Processing
Data sets of our study were all from the Gene Expression Omnibus (GEO) public database, and five sets of gene expression profiling Chips (GEPC) are selected, including GSE12452, GSE13597, GSE61218, GSE64634 and GSE53819Citation16–Citation22 (). NPC tissues and normal nasopharyngeal tissues were collected. GSE12452, GSE13597, GSE61218 and GSE64634 were used as training group data sets, GSE53819 was used as verification group data set. The need for further ethics approval was waived by the Ningbo First Hospital Ethics Committee.
Table 1 Characteristics of mRNA Expression Profiles of Nasopharyngeal Carcinoma (NPC)
Screening of Differentially Expressed Genes (DEGs)
For the microarray dataset (GSE12452, GSE13597, GSE61218 and GSE64634), background correction and normalization were performed by applying the combat algorithm. The limma packageCitation23 of R language was applied for standardization of expression matrix and screening of differential expressed genes (DEGs), and then the volcano plot and heatmap were drawn to present the differential expression of DEGs. The DEGs with an adjusted p < 0.05 and |log2FC| ≥2 were considered statistically significant.
Functional Enrichment Analysis
The GO (Gene Ontology) and KEGG (Kyoto Encyclopedia of Genes and Genomes) enrichment analysis of DEGs were implemented by the clusterProfiler package in R.Citation24 Gene set enrichment analysis (GSEA) was performed on the gene expression matrix through the “clusterProfiler” package and “c2.cp.kegg.v7.4.symbols.gmt” was selected as enrichment analysis gene set to run GSEA software.Citation25 Enrichment results with a p-value <0.05 and false discovery rate (FDR) <0.05 were considered statistically significant.
Screening Characteristic Related Biomarkers via the Comprehensive Strategy
The four algorithms were adopted for screening of novel and key biomarkers for NPC, including random forest (RF) machine learning algorithm,Citation26 least absolute shrinkage and selection operator (LASSO) logistic regression,Citation27 support vector machine-recursive feature eliminationCitation28 (SVM-RFE), and weighted gene co-expression network analysis (WGCNA). WGCNA is a systematic biological method used to describe the gene association modes among different samples, and it can be used to identify gene sets with highly synergistic variation and identify candidate biomarkers or therapeutic targets based on the coherence of gene sets and the correlation between gene sets and phenotypes.Citation29 The RF is widely used in medicine as a machine learning algorithm based on decision-tree theory for solving classification problems. RF produces randomly numerous independent tress as an ensemble to avoid overfitting and sensitivity to training data configuration, the predictive performance of RF has similar performance as the best-supervised learning algorithms, RF efficiently estimates the test error without incurring the cost of repeated model training associated with cross-validation, RF is flexible and has very high accuracy. SVM-RFE was a machine learning algorithm based on a support vector machine used to find the best variables by deleting feature vectors generated by SVM, SVM module was established to further identify the diagnostic value of these biomarkers in NPC by e1071 package.Citation30 Receiver operating characteristic (ROC) curves were established to evaluate the diagnostic significance of NPC-related biomarkers using the pROC package in R, and the area under the ROC curve (AUC) indicated the magnitude of diagnostic efficiency.Citation31 P<0.05 was considered to indicate a statistically significant difference. The input files of the ML model was the expression files of the differential genes in all samples. X-axis label was set to the expression level of the differential genes, y-axis set to the type of the sample. RF, LASSO and SVM were chosen as ML methods. The validation method was performed according to the cross validation. ML model parameters were set as follows: randomForest (ntree=500); LASSO cvfit=cv.glmnet (family=“binomial”, alpha=1, type.measure=“deviance”, nfolds=10); SVM=rfe (functions=caretFuncs, method=“cv”, methods=“svmRadial”). Characteristic genes with the minimum cross-validation error were used as output files.
Validation of the Diagnosis-Related Gene Signature
GSE53819 was used as verification group data set. To validate whether the candidate genes have important diagnostic value in patients with NPC, we also measured the candidate genes’ differential expression, ROC curve value and AUC value in the validation set.
Evaluation and Correlation Analysis of Infiltrating Immune Cells
The CIBERSORT algorithm was used to analyze the normalized gene expression data obtained previously, and the proportions of 22 kinds of immune cells were determined.Citation32 A correlation heatmap was produced to detect the associations of each of the immune cells with the others in NPC samples via the “corrplot” package.Citation33 The “ggstatsplot” package was used to perform the Spearman correlation analysis on diagnostic markers and infiltrating immune cells, and the “ggplot2” package was used to visualize the results.
Results
Although previous studies have reported biomarkers associated with NPC, the relationship between the immune infiltration characteristics and these biomarkers of NPC remains unclear. In this study, we performed a comprehensive analysis of ML algorithms to screen potential biomarkers associated with NPC, including RF, LASSO, SVM-RFE, WGCNA. By using CIBERSORT algorithm, we found the difference of immune infiltration between cancer and normal tissue of 22 subpopulations of immune cells in NPC. Ultimately, DTL has been screened as candidate NPC-related biomarker and immune infiltration characteristics of DTL were analyzed.
Screening of DEGs in Different Datasets
The DEGs of integrated data chip (GSE12452, GSE13597, GSE61218 and GSE64634) were identified by limma package. According to the criteria (adjusted p-value < 0.05 and |log2FC| > 2), a total of 46 DEGs were identified in the integrated data chip, including 11 up-regulated and 35 down-regulated genes. The DEGs data were processed by “pheatmap” and “ggrepel” packages in the R program to draw a heatmap and volcano plot of the significantly changed genes ( and ).
Figure 1 DEGs in the integrated dataset of NPC. (A) The volcano plots of DEGs, the red and green dots represent up-regulated and down-regulated genes, respectively. (B) The heatmap of DEGs.
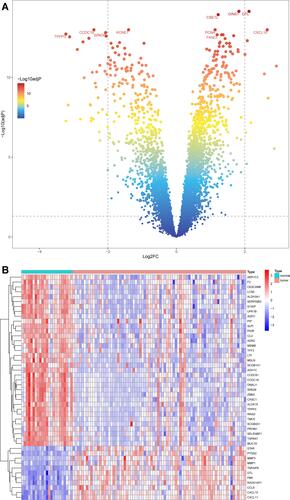
Figure 2 Functional enrichment analysis of DEGs. (A) Results of GO functional enrichment analysis of the DEGs, including BP, MF and CC. (B) KEEG enrichment analysis revealed signaling pathways highly associated with NPC. (C) The top five signaling pathways in normal nasopharyngeal tissue based on GSEA are shown. (D) GSEA showed that the top five signaling pathways were most related to NPC.
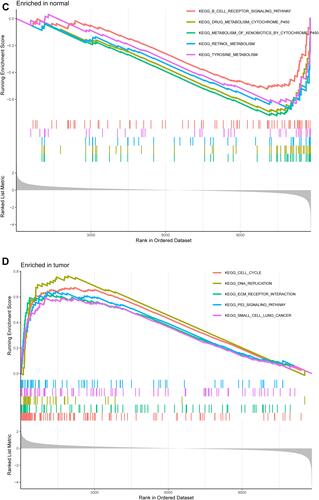
Functional Enrichment Analyses of DEGs
GO enrichment analysis shows the top five GO terms. Biological process (BP) enrichment showed that the common DEGs were enriched in neutrophil degranulation, neutrophil activation involved in immune response, neutrophil mediated immunity, antimicrobial humoral response, and neutrophil activation. The cellular component (CC) part is mainly enriched in secretory granule lumen, cytoplasmic vesicle lumen, vesicle lumen, specific granule lumen and microvillus membrane. GO molecular function (MF) showed that the up-regulated DEGs were remarkably enriched in glycosaminoglycan binding, chemokine activity, serine-type endopeptidase activity, chemokine receptor binding and heparin binding (). KEGG pathway analysis revealed that the DEGs were mainly enriched in the IL-17 signaling pathway, viral protein interaction with cytokine and cytokine receptor, ovarian steroidogenesis, arachidonic acid metabolism and TNF signaling pathway were highly related to NPC pathology (). The GSEA analysis results showed that B cell receptor signaling pathway, metabolism of xenobiotics by cytochrome P450, retinol metabolism, tyrosine metabolism and drug metabolism cytochrome P450 were highly active in normal nasopharyngeal tissue, while cell cycle, DNA replication, small cell lung cancer, ECM receptor interaction and P53 signaling pathway were highly active in NPC tissue ( and ).
Screening Characteristic-Related Biomarkers via the Comprehensive Strategy
We utilized LASSO logistic regression algorithm to identify 7 genes from DEGs as biomarkers for NPC (). Six genes were recognized as vital biomarkers with RF algorithm ( and ). Six genes were detected from DEGs using the SVM-RFE algorithm as diagnostic markers (). To identify sets of genes that are highly correlated in their expression modules, we performed hierarchical clustering on a batch-controlled, rlog transformed expression data using WGCNA. The soft threshold power 5 was chosen to define the adjacency matrix based on the criterion of approximately scale-free topology. Then, we set MEDissThres as 0.25 to merge similar modules, and a total of 8 modules were identified. The hub genes in brown and turquoise module were highly expressed in tumor samples (). Finally, we obtained DTL that was significantly associated with NPC by the four algorithms were overlapped ( and ).
Figure 3 Screening characteristic related biomarkers via comprehensive strategy. (A) The LASSO logistic regression algorithm was performed to retain the most predictive features. (B) Screening biomarkers based on random forest (RF) machine learning algorithm. (C) Results of screening biomarkers based on RF. (D) Results of screening biomarkers based on sSVM-RFE algorithm.
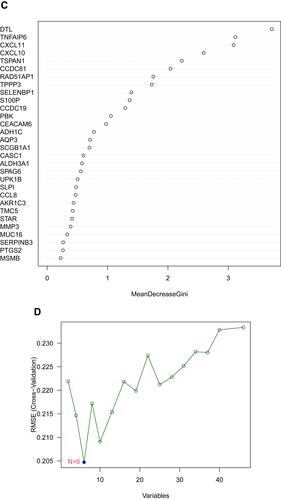
Figure 4 (A) The cluster dendrogram of genes in independent data sets. Branches of the cluster dendrogram of the most connected genes gave rise to eight gene coexpression modules. (B) Relationships of consensus modules with samples. Different color represents a specific module, containing a cluster of highly correlated genes. (C) Soft-threshold power determination for WGCNA by analysis of the scale-free fit index and mean connectivity for various soft-threshold powers.
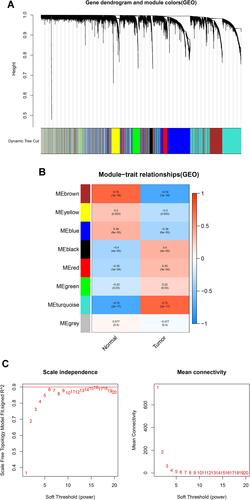
Validation of the Diagnosis-Related Gene Signature
In order to further verify the potentials of DTL as diagnostic markers of NPC, we conducted ROC analysis of these genes in the expression data set GSE53819 and drew the ROC curve (AUC>0.900, P<0.01) ( and ).
Figure 6 Validation of the diagnosis-related gene signature. (A) The expression of DTL in GSE53819. (B) ROC curves of DTL in GSE53819.
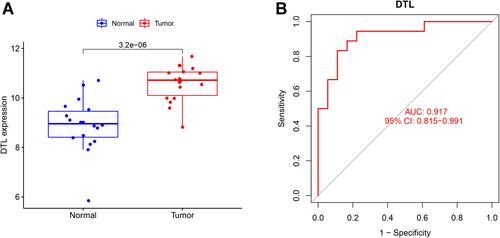
Figure 7 Immune cells infiltration analysis. (A) Pattern of infiltration of 22 kinds of immune cells in normal and tumor groups. (B) The violin plot showed the difference in 22 infiltrating immune cells between NPC and normal nasopharyngeal tissue. (C) The correlation heatmap was drawn to display the correlations of 22 types of infiltrated immune cells. The size of color square represents correlation intensity, red represents the positive correlation, and blue represents the negative correlation.
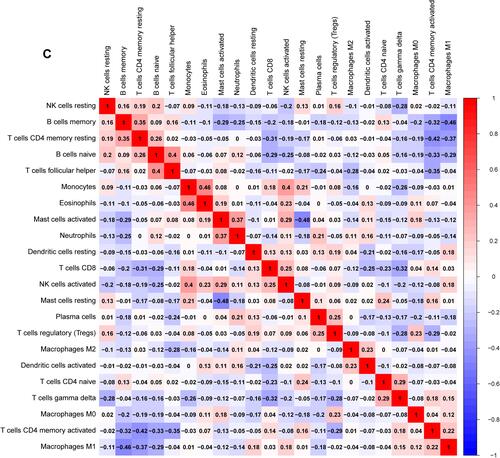
Analysis of Infiltrating Immune Cells
The infiltration abundance matrix of 22 kinds of immune cells in integrated data sets was calculated using CIBERSORT algorithm (). The violin plot showed that the immune infiltration of macrophages M0, macrophages M1 and T cells CD4 memory activated was more, while that of B cells naive, B cells memory and T cells CD4 memory resting was less (). Correlation heatmap of the 22 types of immune cells revealed that monocytes and eosinophils had a significant positive correlation. B cells naive were positively correlated with T cells follicular helper, and NK cells activated and monocytes also positively correlate. While mast cells resting were negatively associated with mast cells activated, macrophages M1 and B cells memory also negatively correlate ().
Correlation Analysis Between Related Biomarkers and Infiltrating Immune Cells
Correlation analysis showed that DTL was positively correlated with macrophages M1 (r = 0.461, p < 0.01), neutrophils (r = 0.289, p < 0.01) and T cells CD4 memory activated (r = 0.402, p < 0.01). DTL was negatively correlated with B cells memory (r = −0.606, p < 0.01) and T cells CD4 memory resting (r = −0.367, p < 0.01) ().
Discussion
Early diagnosis of some NPC patients is very difficult, and the number of candidate biomarkers for NPC is very few according to current studies. Therefore, further study on biomarkers for the diagnosis of NPC is important. In this study, we identified DTL as candidate NPC-related biomarker based on ML method and immune cells differentially distributed between NPC tissue and normal nasopharyngeal tissue. Furthermore, we explored the correlations between DTL and immune cells.
We identified 46 significant DEGs using limma package, including 11 up-regulated genes and 35 down-regulated genes. GO analysis showed that DEGs were mainly concentrated in antimicrobial humoral response, neutrophil degranulation, neutrophil activation involved in immune response, neutrophil-mediated immunity, and neutrophil activation. The KEGG analysis results showed that IL-17 signaling pathway was highly related to NPC pathology. The interleukin-17 (IL-17) family is a subset of cytokines consisting of IL-17A-F that play crucial roles in autoimmune disease and tumor progression. IL-17A has been demonstrated to be upregulated in a wide variety of biologically distinct cancers, including kidney cancer, gastric cancer, breast cancer, cervical cancer and lung cancer.Citation34–Citation36 IL-17A has been reported to control various processes involved in the malignant transformation of cells, such as cell proliferation, one of the major causes of mortality in cancer.Citation37,Citation38 IL17A stimulation increased the proliferation of human NPC cells in vitro.Citation39 Besides, the top five KEGG terms with inverted gene set enrichment included viral protein interaction with cytokine and cytokine receptor, ovarian steroidogenesis, arachidonic acid metabolism and TNF signaling pathway were also related to NPC pathology. The enrichment pathways of GSEA showed that cell cycle, DNA replication, ECM receptor interaction and P53 signaling pathway were highly active in NPC tissue, and the hyperactivity of these pathways may be associated with the development and progression of NPC.
WGCNA is a prevalent systems biology tool used to construct gene co-expression networks, which can be used to detect disease-associated gene clusters and identify therapeutic targets. In order to improve the usability of NPC-related biomarkers for pre-screening purposes, several different approaches were used, including RF, LASSO logistic regression and SVM-RFE. We performed explorative LASSO logistic regression, which performs automatic variable selection and penalizes regression coefficients to decrease overfitting. RF can deal with classification problems with unbalanced, multiclass, and small sample data. Variable selection is performed by means of Support Vector Machine Recursive Feature Elimination (SVM-RFE) for non-linear kernels. To develop biomarkers associated with diagnosis of NPCS, we combined the intersection of four algorithms.Citation40 Finally, DTL was selected as biomarkers to identify NPC.
DTL, also known as retinoic acid-regulated nuclear matrix-associated protein (RAMP), or DNA replication factor 2 (CDT2), is reported to be correlated with the cell proliferation, cell cycle arrest and cell invasion in hepatocellular carcinoma, breast cancer and gastric cancer.Citation41 DTL is a substrate receptor for the CRL4 ubiquitin ligase, serving as a key regulator of the cell cycle and genomic stability. Along with the substrate receptor DTL, the CRL4 ubiquitin ligase promotes the ubiquitin-dependent degradation of several proteins essential for cell cycle progression as well as for DNA replication and repair.Citation42 The expression level of DTL was found to be elevated in human malignancies including breast cancer and ovarian cancer. Besides, its potential as a prognostic biomarker in gastric cancer and Ewing sarcoma has been reported. Furthermore, data from TCGA revealed that patients with melanoma with higher DTL expression exhibit shorter disease-free survival (DFS) and overall survival (OS).Citation43–Citation46 Previous studies have shown that DTL might make cancer cells become addicted. This phenomenon has been termed “non-oncogene addiction” in reference to the increased dependence of cancer cells on the normal cellular functions of certain genes, which themselves are not classical oncogenes. Research has demonstrated that DTL depletion can induce apoptosis in different cancer cell lines without affecting non-cancer cell lines. Consequently, the “non-oncogene addiction” feature facilitates DTL signalling as a potential therapeutic target.Citation47–Citation49
To quantify the relative proportions of infiltrating immune cells from the gene expression profiles in NPC, a bioinformatics algorithm called CIBERSORT was used to calculate immune cell infiltration. CIBERSORT has been increasingly used to estimate the infiltration of immune cells due to its favourable performance.Citation50,Citation51 We used CIBERSORT to further evaluate the immune infiltration of NPC to explore the role of immune cell infiltration in NPC, and analyzed the correlation between related biomarker and infiltrating immune cells. We discovered that the expression of DTL was positively correlated with macrophages M1, neutrophils and T cells CD4 memory activated levels in NPC group. While was negatively correlated with B cells memory and T cells CD4 memory resting. In addition, we found higher immune infiltration levels of macrophages M0, macrophages M1 and T cells CD4 memory activated in NPC group. Although studies have shown that changes in immune microenvironment are closely related to the occurrence and development of NPC, the specific mechanism remains unclear,Citation52,Citation53 4-mRNA signature (U2AF1L5, TMEM265, GLB1L and MLF1), immune subtypes and constitutive activation of the NF-κB inflammatory pathways were considered as possible mechanisms.Citation54–Citation56 Although more research is needed, we speculated that changes in immune microenvironment caused by overexpression of DTL might be one of the mechanisms of NPC based on the results of this study. The limitation of this study is that the conclusion has not been verified by immunohistochemistry. In the future study, we will scrupulously design experiments and collect nasopharyngeal cancer samples for immunohistochemistry to verify the conclusion of this study.
Conclusions
In summary, we found that DTL was biomarker associated with NPC. Macrophages M0, macrophages M1 and T cells CD4 memory activated are related to NPC occurrence. Further research on biomarkers of NPC will help us to understand the internal mechanism of the occurrence and development of NPC, while help us to diagnose NPC early so that more NPC patients can obtain a better prognosis.
Acknowledgments
We acknowledge GEO database for providing their platforms and contributors for uploading their meaningful datasets.
Disclosure
The authors report no conflicts of interest in this work.
Additional information
Funding
References
- Chen YP, Chan AT, Le QT, et al. Nasopharyngeal carcinoma. Lancet. 2019;394(10192):64–80. doi:10.1016/S0140-6736(19)30956-0
- Peng L, Liu JQ, Xu C, et al. The prolonged interval between induction chemotherapy and radiotherapy is associated with poor prognosis in patients with nasopharyngeal carcinoma. Radiat Oncol. 2019;14(1):9. doi:10.1186/s13014-019-1213-4
- Mao YP, Xie FY, Liu LZ, et al. Re-evaluation of 6th edition of AJCC staging system for nasopharyngeal carcinoma and proposed improvement based on magnetic resonance imaging. Int J Radiat Oncol Biol Phys. 2009;73(5):1326–1334. doi:10.1016/j.ijrobp.2008.07.062
- Chan KC, Hung EC, Woo JK, et al. Early detection of nasopharyngeal carcinoma by plasma Epstein-Barr virus DNA analysis in a surveillance program. Cancer. 2013;119(10):1838–1844. doi:10.1002/cncr.28001
- King AD, Woo JK, Ai QY, et al. Complementary roles of MRI and endoscopic examination in the early detection of nasopharyngeal carcinoma. Ann Oncol. 2019;30(6):977–982. doi:10.1093/annonc/mdz106
- Liu ZW, Ji MF, Huang QH, et al. Two Epstein-Barr virus-related serologic antibody tests in nasopharyngeal carcinoma screening: results from the initial phase of a cluster randomized controlled trial in Southern China. Am J Epidemiol. 2013;177(3):242–250. doi:10.1093/aje/kws404
- Coghill AE, Hsu W-L, Pfeiffer RM, et al. Epstein–Barr virus serology as a potential screening marker for nasopharyngeal carcinoma among high-risk individuals from multiplex families in Taiwan. Cancer Epidemiol Biomarkers Prev. 2014;23(7):1213–1219. doi:10.1158/1055-9965.EPI-13-1262
- Lee AW, Ng WT, Chan L, et al. Evolution of treatment for nasopharyngeal cancer–success and setback in the intensity-modulated radiotherapy era. Radiother Oncol. 2014;110(3):377–384. doi:10.1016/j.radonc.2014.02.003
- Luo WR, Chen XY, Li SY, et al. Neoplastic spindle cells in nasopharyngeal carcinoma show features of epithelial-mesenchymal transition. Histopathology. 2012;61(1):113–122. doi:10.1111/j.1365-2559.2012.04205.x
- Luo WR, Yao KT. Molecular characterization and clinical implications of spindle cells in nasopharyngeal carcinoma: a novel molecule-morphology model of tumor progression proposed. PLoS One. 2013;8(12):e83135. doi:10.1371/journal.pone.0083135
- Han BA, Yang XP, Zhang P, et al. DNA methylation biomarkers for nasopharyngeal carcinoma. PLoS One. 2020;15(4):e0230524. doi:10.1371/journal.pone.0230524
- Baştanlar Y, Ozuysal M. Introduction to machine learning. Methods Mol Biol. 2014;1107:105–128.
- Barbounaki S, Vivilaki VG. Intelligent systems in obstetrics and midwifery: applications of machine learning. Eur J Midwifery. 2021;5:58. doi:10.18332/ejm/143166
- Dai CX, Sun BW, Wang RZ, et al. The application of artificial intelligence and machine learning in pituitary adenomas. Front Oncol. 2021;11:784819. doi:10.3389/fonc.2021.784819
- Kolluri S, Lin JC, Liu R, et al. Machine learning and artificial intelligence in pharmaceutical research and development: a review. AAPS J. 2022;24(1):19. doi:10.1208/s12248-021-00644-3
- Dodd LE, Sengupta S, Chen IH, et al. Genes involved in DNA repair and nitrosamine metabolism and those located on chromosome 14q32 are dysregulated in nasopharyngeal carcinoma. Cancer Epidemiol Biomarkers Prev. 2006;15(11):2216–2225. doi:10.1158/1055-9965.EPI-06-0455
- Sengupta S, den Boon JA, Chen IH, et al. Genome-wide expression profiling reveals EBV-associated inhibition of MHC class I expression in nasopharyngeal carcinoma. Cancer Res. 2006;66(16):7999–8006. doi:10.1158/0008-5472.CAN-05-4399
- Hsu WL, Tse KP, Liang S, et al. Evaluation of human leukocyte antigen-A (HLA-A), other non-HLA markers on chromosome 6p21 and risk of nasopharyngeal carcinoma. PLoS One. 2012;7(8):e42767. doi:10.1371/journal.pone.0042767
- Bose S, Yap LF, Fung M, et al. The ATM tumour suppressor gene is down-regulated in EBV-associated nasopharyngeal carcinoma. J Pathol. 2009;217(3):345–352. doi:10.1002/path.2487
- Fan C, Wang J, Tang Y, et al. Upregulation of long non-coding RNA LOC284454 may serve as a new serum diagnostic biomarker for head and neck cancers. BMC Cancer. 2020;20(1):917.
- Bo H, Gong Z, Zhang W, et al. Upregulated long non-coding RNA AFAP1-AS1 expression is associated with progression and poor prognosis of nasopharyngeal carcinoma. Oncotarget. 2015;6(24):20404–20418. doi:10.18632/oncotarget.4057
- Bao YN, Cao X, Luo DH, et al. Urokinase-type plasminogen activator receptor signaling is critical in nasopharyngeal carcinoma cell growth and metastasis. Cell Cycle. 2014;13(12):1958–1969. doi:10.4161/cc.28921
- Ritchie ME, Phipson B, Wu D, et al. limma powers differential expression analyses for RNA-sequencing and microarray studies. Nucleic Acids Res. 2015;43(7):e47. doi:10.1093/nar/gkv007
- Yu G, Wang LG, Han Y, et al. clusterProfiler: an R package for comparing biological themes among gene clusters. OMICS. 2012;16(5):284–287. doi:10.1089/omi.2011.0118
- Subramanian A, Tamayo P, Mootha VK, et al. Gene set enrichment analysis: a knowledge-based approach for interpreting genome-wide expression profiles. Proc Natl Acad Sci U S A. 2005;102(43):15545–15550. doi:10.1073/pnas.0506580102
- Alhamzawi R, Ali HTM. The Bayesian adaptive lasso regression. Math Biosci. 2018;303:75–82. doi:10.1016/j.mbs.2018.06.004
- Alakwaa FM, Chaudhary K, Garmire LX. Deep learning accurately predicts estrogen receptor status in breast cancer metabolomics data. J Proteome Res. 2018;17(1):337–347. doi:10.1021/acs.jproteome.7b00595
- Lin X, Yang F, Zhou L, et al. A support vector machine-recursive feature elimination feature selection method based on artificial contrast variables and mutual information. J Chromatogr B Analyt Technol Biomed Life Sci. 2012;910:149–155. doi:10.1016/j.jchromb.2012.05.020
- Liao R, Ma QZ, Zhou CY, et al. Identification of biomarkers related to Tumor-Infiltrating Lymphocytes (TILs) infiltration with gene co-expression network in colorectal cancer. Bioengineered. 2021;12(1):1676–1688. doi:10.1080/21655979.2021.1921551
- Huang ML, Hung YH, Lee WM, et al. SVM-RFE based feature selection and Taguchi parameters optimization for multiclass SVM classifier. Sci World J. 2014;2014:795624. doi:10.1155/2014/795624
- Robin X, Turck N, Hainard A, et al. pROC: an open-source package for R and S+ to analyze and compare ROC curves. BMC Bioinform. 2011;12(1):77. doi:10.1186/1471-2105-12-77
- Xue G, Hua L, Zhou N, et al. Characteristics of immune cell infiltration and associated diagnostic biomarkers in ulcerative colitis: results from bioinformatics analysis. Bioengineered. 2021;12(1):252–265. doi:10.1080/21655979.2020.1863016
- Serang S, Jacobucci R, Brimhall KC, et al. Exploratory mediation analysis via regularization. Struct Equ Modeling. 2017;24(5):733–744. doi:10.1080/10705511.2017.1311775
- Xu C, Yu L, Zhan P, et al. Elevated pleural effusion il-17 is a diagnostic marker and outcome predictor in lung cancer patients. Eur J Med Res. 2014;19(1):23. doi:10.1186/2047-783X-19-23
- Song Y, Yang JM. Role of interleukin (IL)-17 and T-helper (Th)17 cells in cancer. Biochem Biophys Res Commun. 2017;493(1):1–8. doi:10.1016/j.bbrc.2017.08.109
- Li J, Mo HY, Xiong G, et al. Tumor microenvironment macrophage inhibitory factor directs the accumulation of interleukin-17-producing tumor-infiltrating lymphocytes and predicts favorable survival in nasopharyngeal carcinoma patients. J Biol Chem. 2012;287(42):35484–35495. doi:10.1074/jbc.M112.367532
- Wang LX, Ma RX, Di LL, et al. Correlation between IL-17A expression in nasopharyngeal carcinoma tissues and cells and pathogenesis of NPC in endemic areas. Eur Arch Otorhinolaryngol. 2019;276(11):3131–3138. doi:10.1007/s00405-019-05608-0
- Roy LD, Sahraei M, Schettini JL, et al. Systemic neutralization of IL-17A significantly reduces breast cancer associated metastasis in arthritic mice by reducing CXCL12/SDF-1 expression in the metastatic niches. BMC Cancer. 2014;14:225. doi:10.1186/1471-2407-14-225
- Cai K, Wang B, Dou H, et al. IL-17A promotes the proliferation of human nasopharyngeal carcinoma cells through p300-mediated Akt1 acetylation. Oncol Lett. 2017;13(6):4238–4244.
- Sanz H, Valim C, Vegas E, et al. SVM-RFE: selection and visualization of the most relevant features through non-linear kernels. BMC Bioinform. 2018;19(1):432. doi:10.1186/s12859-018-2451-4
- Pan HW, Chou HY, Liu SH, et al. Role of L2DTL, cell cycle-regulated nuclear and centrosome protein, in aggressive hepatocellular carcinoma. Cell Cycle. 2006;5(22):2676–2687. doi:10.4161/cc.5.22.3500
- Kobayashi H, Komatsu S, Ichikawa D, et al. Overexpression of denticleless E3 ubiquitin protein ligase homolog (DTL) is related to poor outcome in gastric carcinoma. Oncotarget. 2015;6(34):36615–36624. doi:10.18632/oncotarget.5620
- Abbas T, Dutta A. CRL4Cdt2: master coordinator of cell cycle progression and genome stability. Cell Cycle. 2011;10(2):241–249. doi:10.4161/cc.10.2.14530
- Mackintosh C, Ordóñez JL, García DJ, et al. 1q gain and CDT2 overexpression underlie an aggressive and highly proliferative form of Ewing sarcoma. Oncogene. 2012;31(10):1287–1298. doi:10.1038/onc.2011.317
- Pan WW, Zhou JJ, Yu C, et al. Ubiquitin E3 ligase CRL4(CDT2/DCAF2) as a potential chemotherapeutic target for ovarian surface epithelial cancer. J Biol Chem. 2013;288:29680–29691
- Benamar M, Guessous F, Du KP, et al. Inactivation of the CRL4-CDT2-SET8/p21 ubiquitylation and degradation axis underlies the therapeutic efficacy of pevonedistat in melanoma. EBioMedicine. 2016;10:85–100. doi:10.1016/j.ebiom.2016.06.023
- Luo J, Solimini NL, Elledge SJ. Principles of cancer therapy: oncogene and non-oncogene addiction. Cell. 2009;136(5):823–837. doi:10.1016/j.cell.2009.02.024
- Olivero M, Dettori D, Arena S, et al. The stress phenotype makes cancer cells addicted to CDT2, a substrate receptor of the CRL4 ubiquitin ligase. Oncotarget. 2014;5(15):5992–6002. doi:10.18632/oncotarget.2042
- Yang L, Dai J, Ma M, et al. Identification of a functional polymorphism within the 3’-untranslated region of denticleless E3 ubiquitin protein ligase homolog associated with survival in acral melanoma. Eur J Cancer. 2019;118:70–81. doi:10.1016/j.ejca.2019.06.006
- Luo MS, Huang GJ, Liu BX. Immune infiltration in nasopharyngeal carcinoma based on gene expression. Medicine. 2019;98(39):e17311. doi:10.1097/MD.0000000000017311
- Becht E, Giraldo NA, Lacroix L, et al. Estimating the population abundance of tissue-infiltrating immune and stromal cell populations using gene expression. Genome Biol. 2016;17(1):218. doi:10.1186/s13059-016-1070-5
- Jin SZ, Li RY, Chen MY, et al. Single-cell transcriptomic analysis defines the interplay between tumor cells, viral infection, and the microenvironment in nasopharyngeal carcinoma. Cell Res. 2020;30(11):950–965. doi:10.1038/s41422-020-00402-8
- Wang YQ, Liu X, Xu C, et al. Spatial heterogeneity of immune infiltration predicts the prognosis of nasopharyngeal carcinoma patients. Oncoimmunology. 2021;10(1):1976439. doi:10.1080/2162402X.2021.1976439
- Zhao S, Dong X, Ni XG, et al. Exploration of a novel prognostic risk signature and its effect on the immune response in nasopharyngeal carcinoma. Front Oncol. 2021;11:709931. doi:10.3389/fonc.2021.709931
- Chen YP, Lv JW, Mao YP, et al. Unraveling tumour microenvironment heterogeneity in nasopharyngeal carcinoma identifies biologically distinct immune subtypes predicting prognosis and immunotherapy responses. Mol Cancer. 2021;20(1):14. doi:10.1186/s12943-020-01292-5
- Bruce JP, To KF, Lui WY, et al. Whole-genome profiling of nasopharyngeal carcinoma reveals viral-host co-operation in inflammatory NF- κB activation and immune escape. Nat Commun. 2021;12(1):4193. doi:10.1038/s41467-021-24348-6