Abstract
Purpose
Glutamine and serine rich 1 (QSER1), as a DNA methylation modulator, play a crucial role in transforming tumor cells. Previous studies have shown that QSER1 plays a role in regulating the progression of various malignancies and that QSER1 dysfunction is connected with precancerous lesions of hepatocellular carcinoma (HCC) as well as HCC prognosis. However, little is known about the detailed contribution of QSER1 in HCC.
Patients and Methods
Various statistical methods such as Kaplan–Meier method, AUC analysis, GSEA, and immune-infiltration analysis were used to evaluate the relationship between QSER1 expression and clinical features, prognostic factors, and potential functional mechanisms of QSER1.
Results
QSER1 expression was negatively correlated with clinicopathological features (clinical stage, pathological grade, TP53 mutation, lymph node metastasis) and clinical outcome (overall survival versus recurrence). Functional enrichment analysis further suggested that QSER1 is involved in multiple pathways related to DNA replication and tumor immunity. TIMER analysis indicated that high QSER1 expression was significantly associated with higher macrophage infiltration and poorer macrophage-related outcomes. In particular, QSER1 was significantly more associated with M2 macrophages than M1 macrophages.
Conclusion
Overall, elevated QSER1 is a potential prognostic marker for HCC and is associated with immune infiltration in HCC.
Keywords:
Introduction
Liver cancer, more specifically hepatocellular carcinoma (HCC), is the second deadliest cancer, and its incidence is increasing worldwide every year.Citation1 According to statistics, nearly 85% of HCC cases occur in areas where medical resources are scarce, such as East Asia and sub-Saharan Africa.Citation2 Local ablation, surgical resection, or liver transplantation can lead to a clinical cure of early-stage HCC.Citation3 However, poor HCC surveillance causes most patients to lose the optimal treatment window.Citation3 For unresectable HCC, several targeted therapeutic agents, such as Sorafenib and regorafenib, have been approved for HCC treatment.Citation4 The overall therapeutic effect of these drugs is not satisfactory due to their side effects and drug resistance.Citation4 Therefore, novel and reliable diagnostic and therapeutic markers for HCC are urgently needed to improve patient prognosis.Citation5
Although the Human Genome Project has defined most of the gene functions, there are still some protein-coding genes whose functions are unknown.Citation6 Therefore, developing an easier understanding of disease mechanisms and elucidating the alterations and effects of these genes in disease states. Glutamine and serine-rich protein 1 (QSER1) is a gene of unknown function mutated in different cancer tissues.Citation7 Ovarian cancer studies have reported that high-frequency mutations in the QSER1 gene may influence the clinical outcome of ovarian cancer treatment.Citation8 QSER1 was found to be downregulated in gliomaCitation9 and non-small-cell lung cancer.Citation10 The mRNA expression level of QSER1 is connected with survival and recurrence in lung adenocarcinoma patients.Citation11 It has been shown that QSER1 is significantly mutated in high-grade dysplastic nodules, precancerous lesions of HCC.Citation12 Moreover, QSER1 was expressed at much higher levels in HCC cells than in normal hepatocytes and was linked with HCC recurrence.Citation13 Therefore, QSER1 may be a potential cancer marker. However, the mechanism of action of QSER1 in HCC needs to be further clarified.
In this study, we compared the expression levels of QSER1 in normal liver and HCC. We also broke down the relationship between QSER1 expression and clinicopathological features and further explored the prognostic value of QSER1 in patients’ overall survival (OS) and recurrence-free survival (RFS). Then, we investigated the potential relevance between QSER1 expression and immune infiltration. Our study showed that QSER1 is a novel premonitory biomarker in HCC patients and correlates with macrophage infiltration.
Materials and Methods
QSER1 Gene Expression Analysis
The Hepatocellular Carcinoma Comprehensive Molecular Database (HCCDB) is a gene expression atlas of HCC that contains 15 publicly available datasets.Citation14 The HCCDB helps researchers perform cross-platform differential expression analysis, predictive analysis, and tissue-specific network analysis and provides a visual presentation of the results for target genes. In this study, samples containing QSER1 expression data in the HCCDB were used to analyze the transcript levels of QSER1 in HCC and normal liver.
The Human Protein Atlas (HPA) is a protein atlas database based on human organ tissues.Citation15 The database provides a detailed analysis of approximately 20,000 protein-coding genes by integrating multiomics data. The entire database covers the distribution of proteins in all crucial tissues and organs in the human body. Additionally, it annotates proteins that are only expressed in specific tissues such as the brain, heart, or liver. As an open data resource, this database improves fundamental insights into human biology and is anticipated to help drive the development of new diagnostics and drugs. We compared the expression levels of QSER1 protein in human liver tissues with HCC tissues based on HPA atlases.
UALCAN is an easy-to-use, interactive portal that allows a thorough analysis of TCGA level 3 RNA-seq and clinical data for 31 cancers.Citation16 UALCAN can evaluate the association of transcript levels or methylation levels of target genes with clinicopathological features. Links to other databases such as HPRD, GeneCards, PubMed, TargetScan, DRUGBANK, open targets, and GTEx are also available to provide additional information about the selected genes. Specifically, in this study, we used UALCAN to analyze the mRNA expression levels of QSER1 in different clinicopathological subgroups of the TCGA-LIHC cohort.
Survival Prognostication
Currently, as an online survival analysis website,Citation17 the Kaplan–Meier Plotter database presents 54,675 genes and 18,674 cancer samples for study, covering breast cancer, lung cancer, etc.; data types involve microarray data, high-throughput sequencing data, containing mRNA and miRNA, and are continually being concentrated. Here, we evaluated the ability of QSER1 to predict overall survival (OS) and progression-free survival (PFS) in HCC patients (TCGA-LIHC cohort) using the Kaplan–Meier mapper. We also evaluated the predictive ability of QSER1 for prognosis in different HCC clinicopathological subgroups. We separated the TCGA-LIHC cohort into two groups based on the best cutoff value of QSER1. The Kaplan–Meier plotter was automatically selected for the Log rank test in the analysis. The results of the subgroup analysis were displayed as forest plots in R (R package: forest plot, version 1.10.1).Citation18
Function Exploration of QSER1
In the TCGA-LIHC cohort, by calculating the correlation between QSER1 and all differentially expressed genes, we screened the 50 genes with the strongest associations with QSER1. GO, and KEGG analyses of QSER1 and differentially associated genes were implemented, and we also explored the possible pathways and mechanisms of regulation involved in QSER1 and its associated genes. Pathways with P<0.05 were considered expressively concentrated. The GSCALite tool presented the role of QSER1 in ten classical tumor-associated tracks in the form of pie charts.Citation19 Based on the meta-analysis of the HCCDB database, we finally constructed a gene coexpression network of QSER1 in normal liver, paraneoplastic and hepatocellular carcinoma tissues.Citation20,Citation21
Correlation Analysis of QSER1 and Immune Cell Infiltration
The elements and enrichment of immune cells in the tumor microenvironment can forcefully influence tumor progression and the effectiveness of immunotherapy.Citation4 However, elucidating the complex tumor-immune interactions poses significant computational and experimental challenges.Citation22 Given the gene expression profiles of more than 10,000 samples in the TCGA database, the TIMER database provides a systematic analysis of tumor-infiltrating immune cells (TIICs) for 32 cancer types with a user-friendly portal.Citation22 TIMER identifies the enrichment of TIICs according to a statistical analysis of gene expression profiles.Citation22 We also analyzed the correlation with QSER1 gene expression levels and the abundance of other infiltrating immune cells according to the expression of specific marker genes for HCC.Citation23 The extent of all correlations was further validated in the Gene Expression Profile Interaction Analysis (GEPIA) database.Citation24
Statistical Analysis
The gene expression correlations were assessed in the TIMER and GEPIA databases using Spearman correlation analysis, and if not explicitly stated, p<0.05 was considered statistically significant.
Results
QSER1 Expression Increased in HCC
Previous studies have confirmed QSER1 as a gene affecting tumorigenesis. Therefore, we detected the expression pattern of QSER1 in HCC in depth. QSER1 was expressively accelerated in HCC tumor tissues compared to normal tissues (). Immunohistochemical results of tumor tissues and adjacent normal tissues revealed that QSER1 protein expression was also expressively increased in HCC tissues (). To further demonstrate the specificity of QSER1 in HCC, we integrated various clinical factors such as gender, race, age, weight, alpha-fetoprotein level, fibrosis ishak score, TP53 mutation status, lymph node metastasis status, cancer stage and tumour grade in hepatocellular carcinoma (LIHC) samples in the TCGA database to compare the transcript levels of QSER1 in each group. The results showed that QSER1 remained at a higher transcript level in HCC patients compared to normal subjects ( and Supplementary Figure 1). Interestingly, QSER1 expression showed a progressive upward trend with an increasing grade stage, while no similar trend was observed in the TNM stage. Overall, QSER1 was highly expressed in HCC.
Figure 1 The expression level of QSER1 in HCC. (A) The mRNA expression of QSER1 in 9 of 10 clinical cohorts was significantly different between HCC and normal liver tissues; (B) Representative IHC images from the HPA database; (C) The expression level of QSER1 in different subgroups of HCC. The Student’s t-test, *p< 0.05; **p< 0.01; ***p< 0.001.
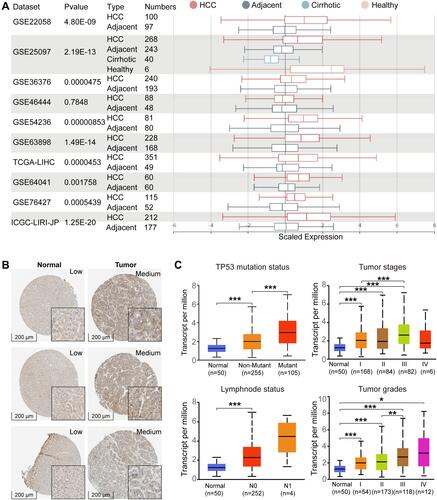
Higher Expression of QSER1 Was Relevant to Poor Prognosis
To estimate the influence of elevated QSER1 expression on the clinical prognosis of HCC, we evaluated the effect of high QSER1 expression on overall survival (OS) and progression-free survival (PFS). Additionally, we analyzed the predictive efficacy of QSER1 transcript levels on 1-, 3-, and 5-year survival outcomes. The outcomes showed that high QSER1 expression was expressively associated with low survival. As shown in and , increased QSER1 was markedly related to poor OS (HR = 1.47[1.04–2.09], P = 0.029) and PFS (HR = 1.65 [1.23–2.22], P = 0.00084). Moreover, time-dependent ROC analysis showed that QSER1 had high sensitivity for predicting early postoperative outcomes in HCC. The areas under the one-year curve for OS and PFS were 0.701 and 0.639, respectively.
Figure 2 The clinical value of QSER1. (A) Overall survival analysis of QSER1 in the TCGA-LIHC cohort. (B) AUC curve evaluating the diagnostic efficacy of QSER1 in identifying the overall survival outcome of HCC. (C) Progression-free survival analysis of QSER1 in the TCGA-LIHC cohort. (D) AUC curve evaluates the diagnostic efficacy of QSER1 in identifying the overall recurrence of HCC. (E) Analysis of the role of QSER1 in the outcome events in different subgroups.
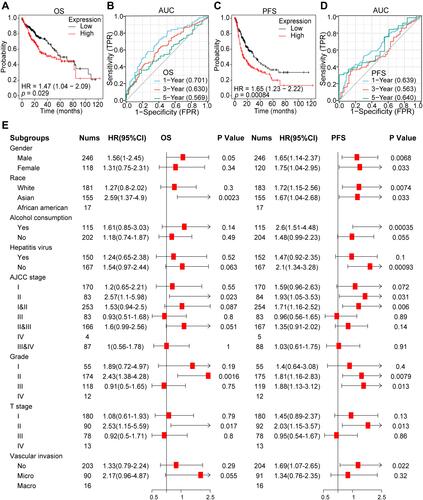
According to the differential expression of QSER1 observed in HCC and the significant prognostic values associated with its expression, we investigated the association with QSER1 expression and different clinicopathological features of HCC using the Kaplan–Meier mapper database. High expression of QSER1 was associated with Asian sex (OS: HR = 2.59, p = 0.0023; PFS: HR = 1.67, p = 0.033), AJCC stage II (OS: HR = 2.57, p = 0.023; PFS: HR = 1.93, p = 0.031), Grade II (OS: HR = 2.43, p = 0.0016; PFS: HR = 1.81, p = 0.0079) and T II (OS: HR = 2.53, p = 0.042; PFS = 0.017; PFS: HR = 2.03, p = 0.013) and was expressively connected with poorer prognosis. However, QSER1 expression was not related to AJCC-T stage III and grade III patients (). These findings suggest that QSER1 expression levels can impact the prognosis of HCC patients with different clinicopathological factors, peculiarly in the early stage of disease.
Enrichment Analysis of QSER1-Associated Co-Expressed Genes in HCC
To explore the feasible molecular mechanisms of QSER1. We performed GO terminology and KEGG analyses on QSER1 and related differentially expressed genes. The outcomes suggested that the significantly differentially expressed genes associated with QSER1 were primarily involved in fatty acid metabolism, factor biosynthesis, humoral immune response, and acute inflammatory response (). In addition, KEGG pathway enrichment analysis proved that these genes were primarily concentrated in the IGF protein kinase B signaling cascade, T cell activation, and DNA replication-related pathways (). We also found that the QSER1 gene plays a role in all ten classical pathways. qSER1 predominantly accounted for the largest share of cell cycle activation (). Eventually, we also analyzed the coexpression network, and the described outcomes performed that the QSER1 coexpression network was utterly different in HCC tissues, adjacent tissues, and normal liver tissues ().
High QSER1 Expression Correlated with M2 Macrophage Polarization
We analyzed the association between QSER1 expression and the enrichment of immune infiltrates by TIMER. As revealed in the correlation plot (), QSER1 expression showed a moderately positive correlation with the infiltration enrichment of suppressive immune cells, such as neutrophils, DCs, and macrophages (r > 0.3, p < 0.05). Other immune cell subsets, including CD4+ T cells and CD8+ T cells were moderately associated with QSER1 (r > 0.1, p < 0.05). These results indicate that QSER1 influences the immune response by affecting immune cell infiltration in the immune microenvironment of hepatocellular carcinoma.
Figure 4 Relationship between QSER1 gene expression and immune infiltration. (A) Correlation between QSER1 and six kinds of immune cell infiltration in the TIMER database. (B) The effects of macrophages on the prognosis of HCC. (C) The effects of dendritic cells on the prognosis of HCC. (D) The impact of QSER1 gene expression and macrophage infiltration on OS. (E) The impact of QSER1 gene expression and macrophage infiltration on RFS. (F) Correlation analysis between QSER1 and M1/M2 macrophage markers. (G) The degree of correlation between QSER1 and M2 macrophage markers (TGFB1 and CSF1R).
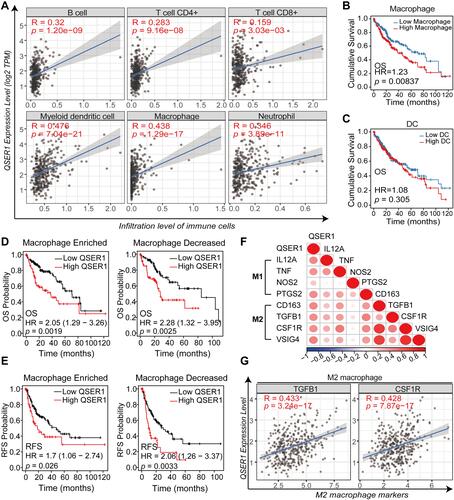
In the next step, we accomplished Kaplan–Meier survival analysis to assess OS in HCC patients with different immune cell infiltrations. Of the immune cells most associated above (DCs and macrophages), only high enrichment of macrophages was expressively correlated with poorer cumulative survival in HCC ( and ); based on a 5-year follow-up analysis, other lymphocytes had no marked effect on the survival result (data not shown). Further survival analysis showed that ascending QSER1 expression was related to a significantly poor prognosis in patients with macrophage infiltration ( and ). When assessing the association between QSER1 and biomarkers of both macrophage types, scatter plots showed a high positive correlation between QSER1 and molecular biomarkers of M2 macrophages (CD163, TGFB1, CSF1R, and VSIG4; and ), while M1 macrophage biomarkers (IL12A, TNF, NOS2, and PTGS2) suggested only low or no correlation (). Overall, our findings suggest that QSER1 upregulation is associated with M2 macrophage polarization, contributing to HCC development.
Discussion
In this study, we managed to identify the potential of QSER1 as a feasible biomarker in HCC patients. Our study is the first to analyze the differential expression of QSER1 in HCC tissues using different public datasets and investigate its role in cancer-related signaling pathways to determine its possible biological importance in HCC carcinogenesis. Using TCGA, HCCDB, GEO, and HPA datasets, we found that QSER1 was expressively upregulated in HCC tissues, and its expression level was negatively related to the OS and PFS of HCC patients. Using the TIMER algorithm, we demonstrated that the expression level of QSER1 was associated with the degrees of infiltration of multiple immune cell types. In addition, we found that QSER1 may lead to M2 macrophage polarization to influence the development and progression of HCC.
It is well known that the levels of AFP, a routine biomarker widely used in clinical practice, correlate with the pathological grading, progression and prognosis of HCC.Citation25–Citation27 However, there are few studies on the prognostic indicators of AFP-negative HCC.Citation27 In the present study, QSER1 expression levels were found to be strongly correlated with serum AFP levels and postoperative outcome of HCC. This suggests that QSER1 may also be a valid predictive target for outcome in AFP-negative HCC patients. TP53 mutations are among the most common genetic changes in HCC.Citation28 It is clinically important to tailor a specific prognostic approach to TP53 mutant HCCs and to explore additional treatment options.Citation28 Our results suggest that QSER1 may be a potential therapeutic target for patients with TP53-mutated HCC. Also, cirrhosis is associated with reduced survival and increased incidence of HCC.Citation29 Advanced fibrosis is an independent predictor of HCC progression.Citation30 The Ishak score, a complex staging system, provides the most accurate assessment of the extent of cirrhosis in HCC patients.Citation31 However, our results suggest that QSER1 may not significantly correlate with the cirrhosis level in HCC. Finally, although TNM stage is an important predictor for HCC outcome,Citation32 Insufficient sample size may have contributed to the insignificant statistical difference in Stage IV (N=6).
As QSER1 is an essential paralog of PRR12, the functions of QSER1 and PRR12 are similar.Citation33 PRR12 is aberrantly expressed in gliomas and is involved in the development and progression of gliomas.Citation34 Similarly, QSER1 is downregulated by miR-181b targeting and may be associated with enhanced sensitivity of glioma cells to temozolomide.Citation9 The long-term progression from chronic liver disease to a confirmed diagnosis of HCC involves multiple steps.Citation35 Genetic mutations can induce normal cell carcinogenesis and promote tumor progression.Citation36 High-grade dysplastic nodules (HGDNs) are precancerous lesions that precede hepatocellular carcinoma (HCC), and approximately 20% of HGDNs eventually progress to HCC.Citation37 Lee et al found that QSER1 presents an HGDN-specific mutation that is the genetic basis for the progression of HGDN to malignant disease.Citation12
Interestingly, recent reports suggest that QSER1 interacts with TET1 to protect transcriptional and developmental procedures from DNMT3-mediated de novo methylation.Citation38 In addition, QSER1 has been reported to interact with the NANOG and TET1 proteins that induce pluripotent stem cells.Citation39 Hepatocellular carcinoma stem cells are currently considered a specific subpopulation with significant tumorigenic potential and play a vital role in the development and recurrence of HCC.Citation40,Citation41 In addition, Das et al proved that QSER1 was expressively overexpressed in HCC and was related to HCC recurrence.Citation13 Overall, these reports are consistent with our results. We found that QSER1 expression levels increased with disease progression and were associated with worse survival situations.
Dysfunction of the immune system is a crucial factor in cancer development and progression.Citation42 Tumor immune escape is mainly facilitated by an immunosuppressive tumor microenvironment, which is associated with a high accumulation of cells negatively regulating immune activity, such as regulatory T cells (Tregs), suppressor B cells, and M2-polarized tumor-associated macrophages (TAMs) in the TME.Citation43 Targeted immune checkpoint therapy has been widely used for cancer treatment.Citation44 A study found that QSER1 is overexpressed in pathological cardiomyopathy and is associated with B-lymphocyte infiltration.Citation45 Our study revealed that QSER1 was associated with the immune response and T-cell activation. Specifically, our outcomes also suggested that QSER1 may take part in the polarization of M2 macrophages.
In HCC, it has been reported that macrophages can promote tumor progression at many stages and induce immunosuppression, metastasis, and chemoresistance, subsequently resulting in decreased survival.Citation46,Citation47 Furthermore, macrophages are highly vital and heterogeneous within and between tumors, ranging from antitumor (M1) to protumor (M2) states.Citation48 We demonstrate that QSER1 is closely associated with M2 macrophage infiltration and positively correlates with specific biomarkers of M2 while connecting insignificantly with M1 markers. Previous studies have proven that M2 macrophages can promote angiogenesis, metastasis, and, most significantly, immunosuppression of hepatocellular carcinoma cells.Citation49 We discovered that macrophages were expressively connected with a poorer prognosis in HCC. Moreover, our survival analysis showed that patients with high macrophage infiltration and facilitated QSER1 expression had poor overall results. Hence, the poor prognosis associated with increased QSER1 may be due, at least to some degree, to the polarization of M2 macrophages. In conclusion, the current study provides rudimentary evidence that QSER1 may be involved in inducing and maintaining the recruitment of M2 macrophages. In other words, targeting QSER1 may represent a promising method to re-program macrophages into an antitumor M1 phenotype.
Although this research has provided us with a better understanding of the oncogenic role of QSER1 in the progression and development of hepatocellular carcinoma, there are still some limiting factors. First, all cohorts of hepatocellular carcinoma patients used were retrospective, as this type of study design may lead to information bias. The clinical value of QSER1 remains to be further recognized in prospectively designed studies, which may reduce the bias due to uncontrollable factors. Moreover, although genomic studies have emerged as one of the most effective approaches to accelerate the translation of cancer research, correlation analyses based on bioinformatics algorithms can only provide preliminary evidence of this relationship. They cannot establish a causal correlation between QSER1 and the immune response. Because of the complexity of immune regulation in cancer pathogenesis and progression, the precise molecular mechanisms of QSER1-mediated immune responses need to be further investigated.
Conclusion
In conclusion, the expression of QSER1 is highly increased in HCC and is related to poorer clinicopathological features and poorer prognosis. QSER1 may influence HCC progression through M2 macrophage recruitment and polarization and mediate immunosuppression in the tumor immune microenvironment. Our study provides good insights into the premonitory and predictive biomarker role of HCC and offers a new precision immunotherapeutic method to combat HCC.
Abbreviations
HCC, hepatocellular carcinoma; QSER1, glutamine and serine rich 1; TCGA, the Cancer Genome Atlas; ICGC, International Cancer Genome Consortium; GEO, Gene Expression Omnibus; OS, overall survival; RFS, recurrence-free survival; HCCDB, Hepatocellular Carcinoma Comprehensive Molecular Database; HPA, Human Protein Atlas; AUC, area under curve; HR, hazard ratio; TIMER, Tumor Immune Estimation Resource.
Data Sharing Statement
This article contains data to support the results of this study.
Ethics Approval and Consent to Participate
This research obtained approval from the Ethics Committee of Xiangya Hospital Central South University (approval number, 202009493). Since the clinical data of this study is publicly obtained from the TCGA and GEO program, the approval of the local ethics committee was not needed.
Author Contributions
All authors made a significant contribution to the work reported, whether that is in the conception, study design, execution, acquisition of data, analysis and interpretation, or in all these areas; took part in drafting, revising or critically reviewing the article; gave final approval of the version to be published; have agreed on the journal to which the article has been submitted; and agree to be accountable for all aspects of the work.
Disclosure
The authors report no conflicts of interest in this work.
Additional information
Funding
References
- Sung H, Ferlay J, Siegel RL, et al. Global cancer statistics 2020: GLOBOCAN estimates of incidence and mortality worldwide for 36 cancers in 185 countries. CA Cancer J Clin. 2021;71:209–249. doi:10.3322/caac.21660
- Tang A, Hallouch O, Chernyak V, Kamaya A, Sirlin CB. Epidemiology of hepatocellular carcinoma: target population for surveillance and diagnosis. Abdom Radiol. 2018;43:13–25. doi:10.1007/s00261-017-1209-1
- Kudo M. Management of hepatocellular carcinoma in Japan as a world-leading model. Liver Cancer. 2018;7:134–147. doi:10.1159/000484619
- Llovet JM, Castet F, Heikenwalder M, et al. Immunotherapies for hepatocellular carcinoma. Nat Rev Clin Oncol. 2021. doi:10.1038/s41571-021-00573-2
- Wang C, Cao Y, Yang C, Bernards R, Qin W. Exploring liver cancer biology through functional genetic screens. Nat Rev Gastroenterol Hepatol. 2021;18:690–704. doi:10.1038/s41575-021-00465-x
- Lander ES, Linton LM, Birren B, et al. Initial sequencing and analysis of the human genome; 2001.
- Patel SJ, Sanjana NE, Kishton RJ, et al. Identification of essential genes for cancer immunotherapy. Nature. 2017;548:537–542. doi:10.1038/nature23477
- Dufresne J, Bowden P, Thavarajah T, et al. The plasma peptides of ovarian cancer. Clin Proteomics. 2018;15:41. doi:10.1186/s12014-018-9215-z
- Wang J, Sai K, Chen FR, Chen ZP. miR-181b modulates glioma cell sensitivity to temozolomide by targeting MEK1. Cancer Chemother Pharmacol. 2013;72:147–158. doi:10.1007/s00280-013-2180-3
- Kim Y, Pierce CM, Robinson LA. Impact of viral presence in tumor on gene expression in non-small cell lung cancer. BMC Cancer. 2018;18:843. doi:10.1186/s12885-018-4748-0
- Yi M, Li T, Qin S, et al. Identifying tumorigenesis and prognosis-related genes of lung adenocarcinoma: based on weighted gene coexpression network analysis. Biomed Res Int. 2020;2020:4169691. doi:10.1155/2020/4169691
- Lee M, Kim K, Kim SY, et al. Genomic structures of dysplastic nodule and concurrent hepatocellular carcinoma. Hum Pathol. 2018;81:37–46. doi:10.1016/j.humpath.2018.06.026
- Das T, Diamond DL, Yeh M, et al. Molecular signatures of recurrent hepatocellular carcinoma secondary to hepatitis C virus following liver transplantation. J Transplant. 2013;2013:878297. doi:10.1155/2013/878297
- Lian Q, Wang S, Zhang G, et al. HCCDB: a database of hepatocellular carcinoma expression atlas. Genomics Proteomics Bioinformatics. 2018;16:269–275. doi:10.1016/j.gpb.2018.07.003
- Thul PJ, Lindskog C. The human protein atlas: a spatial map of the human proteome. Protein Sci. 2018;27:233–244. doi:10.1002/pro.3307
- Chandrashekar DS, Bashel B, Balasubramanya SAH, et al. UALCAN: a portal for facilitating tumor subgroup gene expression and survival analyses. Neoplasia. 2017;19:649–658. doi:10.1016/j.neo.2017.05.002
- Győrffy B. Survival analysis across the entire transcriptome identifies biomarkers with the highest prognostic power in breast cancer. Comput Struct Biotechnol J. 2021;19:4101–4109. doi:10.1016/j.csbj.2021.07.014
- Gordon M, Lumley T, Gordon MM Package ‘forestplot’. Advanced forest plot using ‘grid’graphics The Comprehensive R Archive Network, Vienna; 2019.
- Liu C-J, Hu F-F, Xia M-X, Han L, Zhang Q, Guo A-Y. GSCALite: a web server for gene set cancer analysis. Bioinformatics. 2018;34:3771–3772. doi:10.1093/bioinformatics/bty411
- Ju G, Zhou T, Zhang R, Pan X, Xue B, Miao S. DUSP12 regulates the tumorigenesis and prognosis of hepatocellular carcinoma. PeerJ. 2021;9:e11929. doi:10.7717/peerj.11929
- Xu M, Zhu S, Xu R, Lin N. Identification of CELSR2 as a novel prognostic biomarker for hepatocellular carcinoma. BMC Cancer. 2020;20:313. doi:10.1186/s12885-020-06813-5
- Li T, Fu J, Zeng Z, et al. TIMER2.0 for analysis of tumor-infiltrating immune cells. Nucleic Acids Res. 2020;48:W509–W514. doi:10.1093/nar/gkaa407
- Jiang Y, Chen S, Li Q, et al. TANK-binding kinase 1 (TBK1) serves as a potential target for hepatocellular carcinoma by enhancing tumor immune infiltration. Front Immunol. 2021;12:612139. doi:10.3389/fimmu.2021.612139
- Tang Z, Li C, Kang B, Gao G, Li C, Zhang Z. GEPIA: a web server for cancer and normal gene expression profiling and interactive analyses. Nucleic Acids Res. 2017;45:W98–W102. doi:10.1093/nar/gkx247
- Xu J, Liu C, Zhou L, et al. Distinctions between clinicopathological factors and prognosis of alpha-fetoprotein negative and positive hepatocellular carcinoma patients. Asian Pac J Cancer Prev. 2012;13:559–562. doi:10.7314/APJCP.2012.13.2.559
- Zhao T, Jia L, Li J, et al. Heterogeneities of site-specific N-glycosylation in HCC tumors with low and high AFP concentrations. Front Oncol. 2020;10:496. doi:10.3389/fonc.2020.00496
- Liu Z, Pu Y, Bao Y, He S. Investigation of potential molecular biomarkers for diagnosis and prognosis of AFP-negative HCC. Int J Gen Med. 2021;14:4369–4380. doi:10.2147/IJGM.S323868
- Yang C, Huang X, Li Y, Chen J, Lv Y, Dai S. Prognosis and personalized treatment prediction in TP53-mutant hepatocellular carcinoma: an in silico strategy towards precision oncology. Brief Bioinform. 2021;22:baa164.
- Wang Q, Blank S, Fiel MI, et al. The severity of liver fibrosis influences the prognostic value of inflammation-based scores in hepatitis B-associated hepatocellular carcinoma. Ann Surg Oncol. 2015;22(Suppl 3):S1125–S1132. doi:10.1245/s10434-015-4598-9
- Wang Q, Fiel MI, Blank S, et al. Impact of liver fibrosis on prognosis following liver resection for hepatitis B-associated hepatocellular carcinoma. Br J Cancer. 2013;109:573–581. doi:10.1038/bjc.2013.352
- Everhart JE, Wright EC, Goodman ZD, et al. Prognostic value of Ishak fibrosis stage: findings from the hepatitis C antiviral long-term treatment against cirrhosis trial. Hepatology. 2010;51:585–594. doi:10.1002/hep.23315
- Kinoshita A, Onoda H, Fushiya N, Koike K, Nishino H, Tajiri H. Staging systems for hepatocellular carcinoma: current status and future perspectives. World J Hepatol. 2015;7:406–424. doi:10.4254/wjh.v7.i3.406
- Wang X, Tang H, Bowers JE, Feltus FA, Paterson AH. Extensive concerted evolution of rice paralogs and the road to regaining independence. Genetics. 2007;177:1753–1763.
- Mao Y, Shen G, Su Z, Du J, Xu F, Yu Y. RAD21 inhibited transcription of tumor suppressor MIR4697HG and led to glioma tumorigenesis. Biomed Pharmacother. 2020;123:109759. doi:10.1016/j.biopha.2019.109759
- Schütte K, Bornschein J, Malfertheiner P. Hepatocellular carcinoma–epidemiological trends and risk factors. Dig Dis. 2009;27:80–92. doi:10.1159/000218339
- Villanueva A, Llovet JM. Mutational landscape of HCC—the end of the beginning. Nat Rev Clin Oncol. 2014;11:73–74. doi:10.1038/nrclinonc.2013.243
- Kobayashi M, Ikeda K, Hosaka T, et al. Dysplastic nodules frequently develop into hepatocellular carcinoma in patients with chronic viral hepatitis and cirrhosis. Cancer. 2006;106:636–647. doi:10.1002/cncr.21607
- Dixon G, Pan H, Yang D, et al. QSER1 protects DNA methylation valleys from de novo methylation. Science. 2021;373:372. doi:10.1126/science.373.6553.372
- Costa Y, Ding J, Theunissen TW, et al. NANOG-dependent function of TET1 and TET2 in establishment of pluripotency. Nature. 2013;495:370–374. doi:10.1038/nature11925
- Mishra L, Banker T, Murray J, et al. Liver stem cells and hepatocellular carcinoma. Hepatology. 2009;49:318–329. doi:10.1002/hep.22704
- Ajani JA, Song S, Hochster HS, Steinberg IB. Cancer stem cells: the promise and the potential. Seminars in oncology; 2015; Elsevier; S3–S17.
- Schreiber RD, Old LJ, Smyth MJ. Cancer immunoediting: integrating immunity’s roles in cancer suppression and promotion. Science. 2011;331:1565–1570. doi:10.1126/science.1203486
- Rabinovich GA, Gabrilovich D, Sotomayor EM. Immunosuppressive strategies that are mediated by tumor cells. Annu Rev Immunol. 2007;25:267–296. doi:10.1146/annurev.immunol.25.022106.141609
- Sangro B, Sarobe P, Hervás-Stubbs S, Melero I. Advances in immunotherapy for hepatocellular carcinoma. Nat Rev Gastroenterol Hepatol. 2021;18:525–543. doi:10.1038/s41575-021-00438-0
- Galindo CL, Skinner MA, Errami M, et al. Transcriptional profile of isoproterenol-induced cardiomyopathy and comparison to exercise-induced cardiac hypertrophy and human cardiac failure. BMC Physiol. 2009;9:23. doi:10.1186/1472-6793-9-23
- Eggert T, Wolter K, Ji J, et al. Distinct functions of senescence-associated immune responses in liver tumor surveillance and tumor progression. Cancer Cell. 2016;30:533–547. doi:10.1016/j.ccell.2016.09.003
- Li X, Yao W, Yuan Y, et al. Targeting of tumour-infiltrating macrophages via CCL2/CCR2 signalling as a therapeutic strategy against hepatocellular carcinoma. Gut. 2017;66:157–167. doi:10.1136/gutjnl-2015-310514
- Prieto J, Melero I, Sangro B. Immunological landscape and immunotherapy of hepatocellular carcinoma. Nat Rev Gastroenterol Hepatol. 2015;12:681–700. doi:10.1038/nrgastro.2015.173
- Shi W, Zhang C, Ning Z, et al. CMTM8 as an LPA1-associated partner mediates lysophosphatidic acid-induced pancreatic cancer metastasis. Ann Transl Med. 2021;9:42. doi:10.21037/atm-20-1013