Abstract
Background
GLYATL1 is a member of the glycine-N-acyltransferase family, which catalyses acyl group transfer. The role of GLYATL1 in cancer is largely unknown; therefore, the potential value of GLYATL1 in clear cell renal cell carcinoma (ccRCC) was explored.
Methods
The ccRCC gene expression profiles and clinical data were obtained from the University of California Santa Cruz Xena platform. Differential expression and survival analysis were performed using R software. Samples from the TIMER public database and real-world were used for validation. The potential molecular mechanism of GLYATL1 in ccRCC was explored using gene set enrichment analysis (GSEA).
Results
GLYATL1 was downregulated, indicating a poor prognosis in ccRCC. Low expression of GLYATL1 was significantly associated with advanced stage and higher histological grade ccRCC. The differential expression of GLYATL1 was validated at the protein level using clinical samples and tissue microarray. The results of GSEA showed that multiple crucial signalling pathways including fatty acid metabolism, adipogenesis, oxidative phosphorylation and epithelial–mesenchymal transition were enriched.
Conclusion
This study demonstrated that GLYATL1 downregulation has an unfavourable impact on the survival of patients with ccRCC. The resulting data indicated that GLYATL1 could be a potential new target for ccRCC therapy, which may be helpful for the personalized treatment of such individuals.
Introduction
Renal cell carcinoma (RCC) is one of the most prevalent cancers of the urinary system. Clear cell renal cell carcinoma (ccRCC) is the major histological subtype of RCC, accounting for approximately 75% of all RCCs.Citation1 According to the 2022 cancer statistics data, 79,000 new cases and 13,920 fatality cases are reported in the United States annually.Citation2 Although surgery, targeted molecular therapy and immunotherapy have improved the overall survival (OS) of patients with ccRCC, therapeutic options are limited for those with metastatic ccRCC.Citation3,Citation4 Therefore, early diagnosis and identification of patients with poor prognoses are crucial to improving the survival outcomes of ccRCC. Increasing studies have revealed the various molecular aspects of ccRCC onset and progression. For instance, ccRCC is characterized by near-universal loss of chromosome 3p and relatively high mutation frequencies in several important genes residing on 3p (PBRM1, SETD2, BAP1, MLH1 and SETD2). Hence, chromosome 3p deletion was regarded as the initiating factor in ccRCC.Citation5 In addition, the loss or mutation of the VHL gene is generally considered to be one of the involved initiating steps in the progression of ccRCC, which can regulate the transcription of many genes involved in angiogenesis, metabolism, and chromatin remodeling via the pathway of HIF1α and HIF2α.Citation5 Therefore, understanding the molecular biology of ccRCC is vital.
Glycine-N-acyltransferase-like 1 (GLYATL1) is a member of the glycine-N-acyltransferase (GLYAT) family, mainly expressed in the kidney and liver. GLYATL1, first reported in 2007,Citation6 is located in 11q12.1 and comprises 1546 base pairs. It encodes a protein comprising 302 amino acids, which is involved in carboxylic acid conjugation to glycine and glutamine, like other members of the GLYAT family.Citation6 As various carboxylic acid derivatives are combined with an amino acid to increase water solubility and urine excretion in mammals, this conjugation is an important pathway in endogenous and exogenous acyl-CoA detoxification.Citation7 GLYATL1 is vital to not only normal physiological metabolism but also tumorigenesis. Furthermore, previous studies have reported that the expression and role of GLYATL1 differed among cancer types, with GLYATL1 expression significantly increasing in primary prostate cancer than that in benign prostatic tissue and metastatic prostate cancer.Citation8 Moreover, the knockdown of GLYATL1 inhibits the colony-forming ability of prostate cancer in vitro.Citation9 In breast cancer, GLYATL1 is overexpressed in oestrogen receptor-negative cancer tissue compared with oestrogen receptor-positive cancer tissue.Citation10 Additionally, studies have reported that GLYATL1 is downregulated in hepatocellular carcinoma, and its low expression is associated with poor OS, suggesting the prognostic value of GLYATL1 in hepatocellular carcinoma.Citation11,Citation12 However, the expression and value of GLYATL1 in ccRCC are yet to be investigated. This study explores the potential role of GLYATL1 in ccRCC using data accessed from The Cancer Genome Atlas (TCGA) database and real-world samples.
Materials and Methods
Data Extraction Using Online Databases
ccRCC gene expression profiles and clinical data of the TCGA cohort were obtained from the University of California Santa Cruz Xena platform (https://xena.ucsc.edu/).Citation13 The database provides data on 535 ccRCC RNA-sequence samples and 72 normal kidney samples. After matching the survival data and excluding patients with follow-ups less than one month, 518 patients were selected for survival analysis. Additionally, the TIMER database (https://cistrome.shinyapps.io/timer/) was used for validation studies.Citation14
Tissue Samples
The ccRCC samples from two cohorts were used to validate the GLYATL1 expression at the protein level using immunohistochemical (IHC) analysis. One cohort was from a tissue microarray (HKidE150CS03), which was purchased from Shanghai Outdo Biotech Co., Ltd. (http://www.superchip.com.cn/), containing 75 ccRCC samples and 75 paired normal renal samples. Another cohort was from the Meizhou People’s Hospital, comprising ten paraffin-embedded ccRCC samples and paired normal renal samples. Informed consent was obtained from each patient. Ethical approval for tissue microarray examinations was granted by the Ethics Committee of Outdo Biotech.
IHC Analysis
IHC staining was performed on the paraffin slides and tissue microarray following the provided protocol. The primary antibody was anti-GLYATL1 (1:500 dilution, Cat. No. GTX106956, GeneTex), and the secondary antibody was ab205718 (1:1000 dilution, Abcam, Cambridge, UK). Two independent pathologists evaluated and scored the IHC results after haematoxylin and eosin staining. The percentage of positively stained cells was scored as follows: 0 (< 1%), 1 (1–25%), 2 (26–50%), 3 (51–75%) and 4 (76–100%). The staining intensity was scored as follows: 0 (negative), 1 (weak), 2 (moderate) and 3 (strong). The final IHC score was obtained by multiplying the percentage and intensity scores, which ranged from 0 to 12. Detailed IHC analysis was performed as described previously.Citation15
Gene Set Enrichment Analysis (GSEA)
GSEA is a useful and commonly used method for functional enrichment analysis, which explores the potential mechanism of genes.Citation16 Firstly, the correlation coefficient and P-value was calculated between each gene and GLYATL1. Then, the genes was sorted from largest to smallest based on the correlation coefficient and the gene list was required when performing GSEA. Finally, GSEA was performed according to the gene list using the R package “clusterProfiler”. Thus, the pathways enriched by the GLYATL1 correlated genes could indicate the potential function of GLYATL1 to some extent. Additionally, the correlation between GLYATL1 expression and the significantly enriched pathways using single-sample GSEA was explored. The GO and KEGG analysis were also performed.
Statistics
R software v4.3.1 (R Foundation for Statistical Computing, Vienna, Austria) and Strawberry Perl v.5.30.1.1 were used for data processing and statistical analyses. Additionally, the chi-square test, t-test, Fisher’s exact test and ANOVA were used. The log-rank and Spearman tests were used for survival and correlation analysis, respectively. P < 0.05 was considered statistically significant.
Results
GLYATL1 Was Downregulated in ccRCC
RNA-sequence data from the 535 ccRCC and 72 normal renal samples revealed a significant decrease in GLYATL1 expression in ccRCC tissues compared with that in normal renal tissues (P = 0.00047, ). Additionally, data from the TIMER database was used, which validated the RNA sequence data results (). Moreover, GLYATL1 expression varied among different cancers. For example, compared with normal tissues, GLYATL1 was upregulated in breast invasive carcinoma, colon adenocarcinoma and lung adenocarcinoma; however, it was downregulated in cholangiocarcinoma, kidney chromophobe carcinoma and liver hepatocellular carcinoma ().
Figure 1 GLYATL1 expression showed a decrease in ccRCC tissues compared with normal renal tissues. (A) The data of 535 tumours and 72 normal renal samples were obtained from The Cancer Genome Atlas. (B) GLYATL1 expression data in pan-cancer from the TIMER database (https://cistrome.shinyapps.io/timer/). (C–F) GLYATL1 expression shows a significant decrease with higher histological grade, T stage, distant metastasis and advanced TNM stages. *p < 0.05, **p < 0.01, ***p < 0.001.
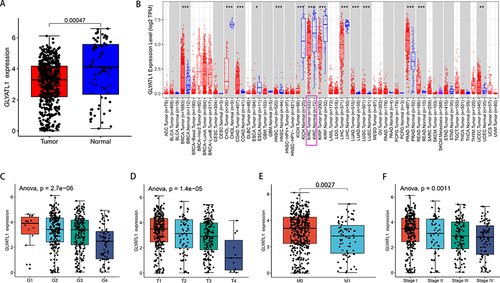
GLYATL1 Expression Correlated with Clinicopathological Parameters in ccRCC
After matching the clinicopathological and GLYATL1 expression data, the patients were divided into high- and low-GLYATL1 expression groups according to the median value of GLYATL1 expression. The association between GLYATL1 expression and clinicopathological characteristics of ccRCC cohort from TCGA is showed in . GLYATL1 expression was significantly associated with sex (P < 0.001), cancer status (P < 0.001), histological grade (P < 0.001), T stage (P < 0.001), M stage (P = 0.002) and TNM stage (P = 0.001). Further analysis revealed a significant decrease in GLYATL1 expression with increasing histological grade (P < 0.001), T stage (P < 0.001), distant metastasis (P = 0.0027) and TNM stage (P = 0.0011, ). These results indicate the negative association of GLYATL1 with ccRCC malignancy.
Table 1 Correlations Between the Expression of GLYATL1 and Clinicopathologic Characteristics in Clear Cell Renal Cell Carcinoma
The Protein Expression Level of GLYATL1 in Two Real-World Cohorts
GLYATL1 expression was validated at the protein level using IHC analysis in two real-world cohorts. IHC results showed that the cytoplasm and renal tubular cell membranes were primarily stained, with a high positively stained cell percentage and stronger staining intensity in normal renal tissues than that in ccRCC tissues in both cohorts (). Paired t-test revealed a significantly higher IHC score in normal renal samples compared with that in tumour samples (P < 0.001, ). Therefore, these results confirmed the downregulation of GLYATL1 in ccRCC.
Figure 2 Protein expression levels of GLYATL1 in ccRCC and normal renal tissues. (A) Representative images of immunohistochemically stained tumours and normal renal tissues. Case 1 represents a cohort of ten cases from the MZPH; case 2 represents a cohort of 75 cases derived from a tissue chip (magnification, ×200). (B) Comparison of the IHC scores of ccRCC and normal renal tissues.
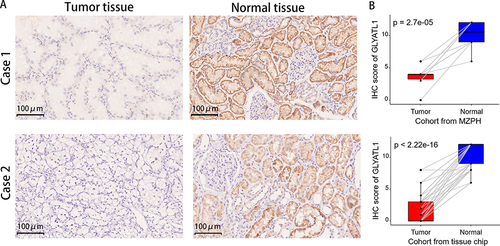
Independent Prognostic Value of GLYATL1 in ccRCC
The Kaplan–Meier analysis was used to explore the survival difference among patients with different GLYATL1 expressions in the TCGA cohort. The results showed that patients with lower GLYATL1 expression had significantly poorer OS and disease-free survival (DFS) than those with higher GLYATL1 expression (P < 0.001, and ). Furthermore, Cox regression was used for univariate and multivariate survival analyses to assess the independent prognostic value of GLYATL1 in ccRCC. The analyses revealed a significant difference in OS between the high- and low-GLYATL1 expression groups for both univariate (hazard ratio [HR], 0.774; 95% confidence interval [CI], 0.698–0.859; P < 0.001) and multivariate analyses (HR, 0.816; 95% CI, 0.729–0.913; P < 0.001, and ). For DFS, univariate (HR, 0.657; 95% CI, 0.577–0.747; P < 0.001) and multivariate (HR, 0.703; 95% CI, 0.607–0.813; P < 0.001, and ) analyses revealed similar results, which indicated the independent prognostic role of GLYATL1 in ccRCC. Additionally, age, histological grade and TNM stage were found to be independent prognostic factors for OS (), which required further stratified survival analysis. Compared to patients with high-GLYATL1, a significantly poorer OS was observed in patients with low-GLYATL1 expression for the following criteria: age < 60 years (P = 0.011) and ≥ 60 years (P < 0.001), grade 3/4 (P < 0.001) and stage I/II (P = 0.007), but not for grade 1/2 (P = 0.18) or stage III/IV (P = 0.052) (). However, a significant difference in DFS between the low- and high- GLYATL1 expression groups was observed in all stratified analyses based on age, histological grade and TNM stage ().
Figure 3 The prognostic value of GLYATL1 in ccRCC cohort from The Cancer Genome Atlas. (A and B) Decreased GLYATL1 expression was correlated with poor OS and DFS in patients with ccRCC. (C and D) Univariate and multivariate Cox regression analyses for OS. (E and F) Univariate and multivariate Cox regression analyses for DFS.
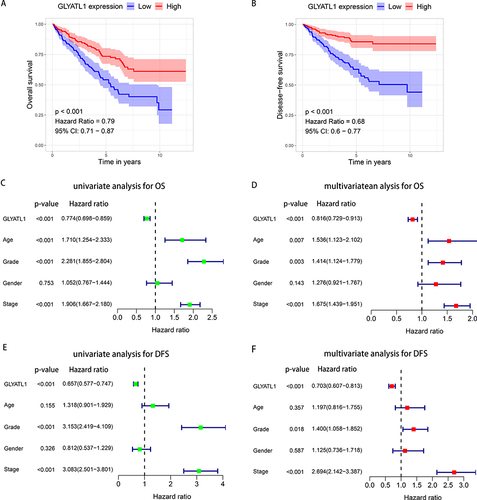
Figure 4 Stratified survival analysis for GLYATL1 expression in ccRCC cohort from The Cancer Genome Atlas. (A–C) Kaplan–Meier survival analysis for overall survival, which is stratified by age, grade, and stage. (D–F) Kaplan–Meier survival analysis for disease-free survival, which is stratified by age, grade, and stage.
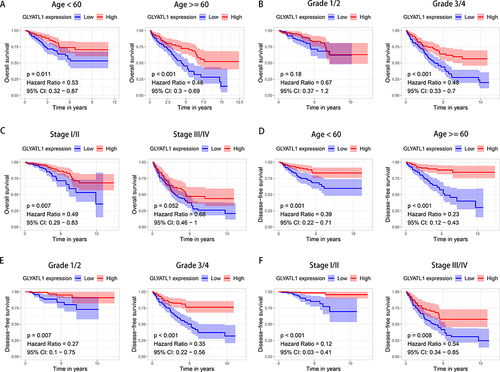
Constructing and Evaluating a GLYATL1-Based Predictive Nomogram
Nomogram is a common method utilized to predict the survival rate of individuals with cancer.Citation17 Calibration plots and concordance index (C-index) are commonly used to evaluate nomogram efficacy.Citation18,Citation19 The independent prognostic factors (age, grade, stage and GLYATL1 expression) were used to construct a nomogram that predicts the OS probability of patients with ccRCC (). The calibration plots revealed the well-predicted 1-, 3-, and 5-year OS probabilities using the nomogram, which were similar to the actual outcomes (). The C-index of the nomogram was 0.770, which was higher than that of any single independent prognostic factor (age, 0.565; grade, 0.672; stage, 0.732; GLYATL1 expression, 0.585). Moreover, the areas under the curve of the nomogram was 0.854, 0.809, and 0.749 for the 1-, 3-, and 5-year OS of patients with ccRCC, respectively, which was higher than any single independent prognostic factor (). These results indicate good predictability of the nomogram for the OS of patients with ccRCC.
Figure 5 A nomogram based on GLYATL1 expression to predict the overall survival of patients with ccRCC. (A) The nomogram for predicting overall survival. (B) Calibration plots show the association between the nomogram-predicted overall survival and actual overall survival. The Y-axis represents the actual survival while the X-axis represents the nomogram-predicted survival. The solid and dotted lines are close, which suggests the efficient predictability of the nomogram. (C) Comparison of the predictive power of the nomogram and other independent prognostic factors for the overall survival of patients with ccRCC.
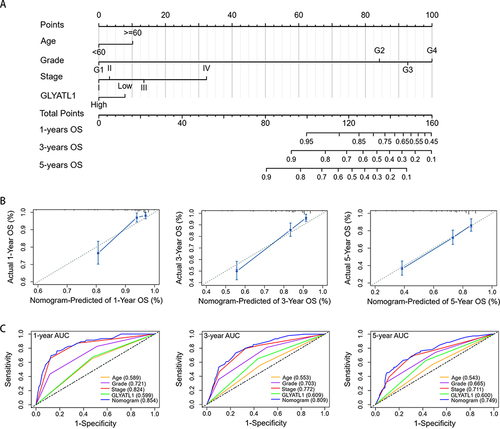
Functional Enrichment Analysis
GLYATL1 expression is closely associated with the clinicopathological features and survival of ccRCC. Hence, the potential molecular mechanism of GLYATL1 was explored through GSEA in the ccRCC cohort acquired from TCGA. The results showed the possible association of GLYATL1 with fatty acid metabolism, adipogenesis, oxidative phosphorylation and epithelial–mesenchymal transition (EMT) (). Consistently, the results of GO and KEGG analysis also showed that GLYATL1 was associated with small molecule metabolic process including carboxylic acid, amino acid, and fatty acid ( and ). Owing to the vital role of fatty acid metabolism reprogramming and EMT in cancer progression, its relationship with GLYATL1 was further explored. It revealed that GLYATL1 expression was significantly negatively correlated with EMT in several cancer types, such as prostate adenocarcinoma, kidney renal papillary cell carcinoma and brain lower grade glioma (). In addition, a positively correlation was observed between GLYATL1 expression and fatty acid metabolism in various cancer types, such as liver hepatocellular carcinoma, cholangiocarcinoma and pancreatic adenocarcinoma ().
Figure 6 The results of functional enrichment analysis. (A) GSEA of GLYATL1 in ccRCC. (B) The results of KEGG analysis. (C) The results of GO analysis. (D) Correlation analysis of GLYATL1 expression and hallmark EMT pathways in pan-cancer analysis. (E) Correlation analysis of GLYATL1 expression and fatty acid metabolism pathways in pan-cancer analysis.
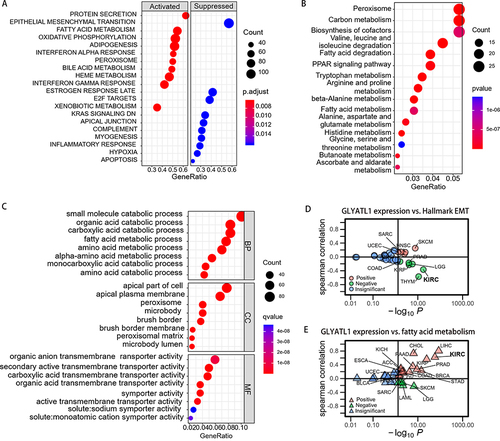
Discussion
Although ccRCC therapeutics have improved, the survival outcomes remain unsatisfactory. The lack of useful prognostic biomarkers can be considered a contributing factor to this unsatisfactory outcome. Therefore, it is crucial to identify novel prognostic biomarkers for patients with ccRCC. GLYATL1 is a potential candidate owing to its involvement in the catalysis of acyl group transfer. Therefore, this study analysed the RNA-sequence data of ccRCC, which were validated using IHC on two real-world cohort samples. The data analyses revealed that GLYATL1 expression was significantly decreased in the ccRCC tumor tissue compared with normal kidney tissue, which may help differentiate ccRCC from normal tissues. In addition, decreased GLYATL1 expression significantly correlated with poorer OS and DFS, which indicated the potential involvement of GLYATL1 as a prognostic biomarker in ccRCC. Hence, GLYATL1 could enable the identification of patients with poor survival and aid in selecting the appropriate therapeutic measures to improve the survival of these individuals.
GLYATL1 encodes a type of glycine-N-acyltransferase, which catalyses the acyl group transfer from acyl-CoA to glycine and regulates the production of adenosine triphosphate; availability of CoASH, glycine, and the detoxification of various organic acids.Citation7,Citation20,Citation21 Apart from normal physiological metabolism, GLYATL1 also plays a significant role in cancers. Guan et al reported that decreased GLYATL1 expression was correlated with poor OS in liver cancer.Citation11 However, Eich et al reported that GLYATTL1 was upregulated and positively correlated with histological grade in prostate cancer.Citation8 In this study, decreased GLYATL1 expression was correlated with malignant clinicopathological features, such as a higher histological grade and an advanced TNM stage. Moreover, univariate and multivariate analyses indicated the independent prognostic value of GLYATL1 for OS and DFS in ccRCC, which was validated by the stratified analysis results based on age, histological grade and TNM stage. However, no significant difference was observed in OS among patients with histological grade 1/2 or TNM stage III/IV, which can be attributed to inadequate follow-up time or small sample sizes. Additionally, the GLYATL1-based nomogram predicted the survival rates of patients with ccRCC, which can help to personalise clinical evaluation and therapeutic strategies.
Previous studies have reported on a close association between glycine metabolism and cancer cell proliferation.Citation22 As an important enzyme in glycine metabolism, GLYATL1 can inhibit glycine uptake and biosynthesis and impair cancer proliferation.Citation23 Additionally, GLYAT, another member of the GLYAT family, was downregulated and associated with poor survival outcomes in breast cancer. Moreover, GLYAT can promote tumour growth and EMT through the PI3K pathway.Citation24 The results of GSEA in this study also suggested that GLYATL1 was significantly associated with EMT pathways in various cancer types including ccRCC.
ccRCC is a known metabolic disease characterized by the reprogramming of energy production processes including the glucose and lipid metabolism.Citation25–27 Additionally, RCC is also one of the most immune-infiltrated tumors.Citation28,Citation29 An increasing body of evidence indicates the crucial crosstalk among metabolism reprogramming, immune infiltration, inflammatory response, and tumor microenvironment.Citation30–32 For example, fatty acids metabolism reprogramming can affect the alternative polarization of immune cells and further influence the therapeutic effect of anti-PD-1/PD-L1 in tumor immune microenvironment in ccRCC.Citation33 Our study revealed that GLYATL1 might play a vital role in fatty acid metabolism and could modulate inflammatory response. This observation aligns with the functions observed in other members of the GLYAT family, including GLYATL2 and GLYATL3.Citation34,Citation35
Although this study elucidates the potential significance of GLYATL1 in ccRCC, several limitations exist. First, the prognostic value of GLYATL1 in ccRCC was mainly elucidated using public databases, which requires further validation using real-world cohorts. Second, although differences in GLYATL1 expression was validated in two cohorts at the protein level, the association between GLYATL1 expression and survival outcomes was only explored at the mRNA level, which requires further validation at the protein level. Third, function enrichment analysis is inadequate to fully elucidate the potential molecular mechanism of GLYATL1 in ccRCC; therefore, further basic in vitro and in vivo experiments are required. Finally, the nomogram in this study was constructed primarily based on the data downloaded directly from database and the methods of detecting GLYATL1 expression and data processing were unclear. Thus, the model cannot be directly applied for individual patient in this form during clinical practice before it was further verified in multi-center clinical studies.
Conclusions
This study demonstrated that GLYATL1 was downregulated in ccRCC and functioned as a key prognostic biomarker for ccRCC. Owing to its potential role in fatty acid metabolism and EMT, GLYATL1 may be a candidate therapeutic target for ccRCC.
Abbreviations
RCC, renal cell carcinoma; ccRCC, clear cell renal cell carcinoma; GLYAT, glycine-N-acyltransferase; GLYATL1, Glycine-N-acyltransferase-like 1; OS, overall survival; TCGA, The Cancer Genome Atlas; qPCR, quantitative polymerase chain reaction.
Data Sharing Statement
The data sets analyzed in our study are available from online databases: UCSC XENA, and TIMER.
Ethical Approval and Informed Consent
Approval was obtained from the ethics committee of Meizhou People’s Hospital and written informed consent was obtained from each patient. The procedures used in this study adhere to the tenets of the Declaration of Helsinki.
Disclosure
The authors declared no potential conflicts of interest with respect to the research, authorship, and/or publication of this article.
Acknowledgments
We would like to thank TCGA, TIMER and UCSC XENA platform. We also thanked Bullet Edits Limited for linguistic editing and proofreading the manuscript.
Additional information
Funding
References
- Linehan WM, Ricketts CJ. The cancer genome atlas of renal cell carcinoma: findings and clinical implications. Nat Rev Urol. 2019;16(9):539–552. doi:10.1038/s41585-019-0211-5
- Siegel RL, Miller KD, Fuchs HE, Jemal A. Cancer statistics, 2022. CA Cancer J Clin. 2022;72(1):7–33. doi:10.3322/caac.21708
- Singh D. Current updates and future perspectives on the management of renal cell carcinoma. Life Sci. 2021;264:118632. doi:10.1016/j.lfs.2020.118632
- Tacconi EMC, Tuthill M, Protheroe A. Review of adjuvant therapies in renal cell carcinoma: evidence to date. Onco Targets Ther. 2020;13:12301–12316. doi:10.2147/OTT.S174149
- Jonasch E, Walker CL, Rathmell WK. Clear cell renal cell carcinoma ontogeny and mechanisms of lethality. Nat Rev Nephrol. 2021;17(4):245–261. doi:10.1038/s41581-020-00359-2
- Zhang H, Lang Q, Li J, et al. Molecular cloning and characterization of a novel human glycine-N-acyltransferase Gene GLYATL1, which activates transcriptional activity of HSE pathway. Int J Mol Sci. 2007;8(5):433–444.
- Badenhorst CP, van der Sluis R, Erasmus E, van Dijk AA. Glycine conjugation: importance in metabolism, the role of glycine N-acyltransferase, and factors that influence interindividual variation. Expert Opin Drug Metab Toxicol. 2013;9(9):1139–1153. doi:10.1517/17425255.2013.796929
- Eich ML, Chandrashekar DS, Rodriguez Pen AM, et al. Characterization of glycine-N-acyltransferase like 1 (GLYATL1) in prostate cancer. Prostate. 2019;79(14):1629–1639. doi:10.1002/pros.23887
- Nalla AK, Williams TF, Collins CP, Rae DT, Trobridge GD. Lentiviral vector-mediated insertional mutagenesis screen identifies genes that influence androgen independent prostate cancer progression and predict clinical outcome. Mol Carcinog. 2016;55(11):1761–1771. doi:10.1002/mc.22425
- Wang J, Shidfar A, Ivancic D, et al. Overexpression of lipid metabolism genes and PBX1 in the contralateral breasts of women with estrogen receptor-negative breast cancer. Int J Cancer. 2017;140(11):2484–2497. doi:10.1002/ijc.30680
- Guan R, Hong W, Huang J, et al. The expression and prognostic value of GLYATL1 and its potential role in hepatocellular carcinoma. J Gastrointest Oncol. 2020;11(6):1305–1321. doi:10.21037/jgo-20-186
- Sun R, Li S, Zhao K, Diao M, Li L. Identification of ten core hub genes as potential biomarkers and treatment target for hepatoblastoma. Front Oncol. 2021;11:591507. doi:10.3389/fonc.2021.591507
- Goldman MJ, Craft B, Hastie M, et al. Visualizing and interpreting cancer genomics data via the xena platform. Nat Biotechnol. 2020;38(6):675–678. doi:10.1038/s41587-020-0546-8
- Li T, Fan J, Wang B, et al. TIMER: a web server for comprehensive analysis of tumor-infiltrating immune cells. Cancer Res. 2017;77(21):e108–e110. doi:10.1158/0008-5472.CAN-17-0307
- Jiang H, Chen H, Wan P, Chen N. Decreased expression of HADH is related to poor prognosis and immune infiltration in kidney renal clear cell carcinoma. Genomics. 2021;113(6):3556–3564. doi:10.1016/j.ygeno.2021.08.008
- Subramanian A, Tamayo P, Mootha VK, et al. Gene set enrichment analysis: a knowledge-based approach for interpreting genome-wide expression profiles. Proc Natl Acad Sci U S A. 2005;102(43):15545–15550. doi:10.1073/pnas.0506580102
- Iasonos A, Schrag D, Raj GV, Panageas KS. How to build and interpret a nomogram for cancer prognosis. J Clin Oncol. 2008;26(8):1364–1370. doi:10.1200/JCO.2007.12.9791
- Fenlon C, O’Grady L, Doherty ML, Dunnion J. A discussion of calibration techniques for evaluating binary and categorical predictive models. Prev Vet Med. 2018;149:107–114. doi:10.1016/j.prevetmed.2017.11.018
- Longato E, Vettoretti M, Di Camillo B. A practical perspective on the concordance index for the evaluation and selection of prognostic time-to-event models. J Biomed Inform. 2020;108:103496. doi:10.1016/j.jbi.2020.103496
- van der Sluis R, Badenhorst CP, Erasmus E, van Dyk E, van der Westhuizen FH, van Dijk AA. Conservation of the coding regions of the glycine N-acyltransferase gene further suggests that glycine conjugation is an essential detoxification pathway. Gene. 2015;571(1):126–134. doi:10.1016/j.gene.2015.06.081
- Badenhorst CP, Erasmus E, van der Sluis R, Nortje C, van Dijk AA. A new perspective on the importance of glycine conjugation in the metabolism of aromatic acids. Drug Metab Rev. 2014;46(3):343–361. doi:10.3109/03602532.2014.908903
- Amelio I, Cutruzzolá F, Antonov A, Agostini M, Melino G. Serine and glycine metabolism in cancer. Trends Biochem Sci. 2014;39(4):191–198. doi:10.1016/j.tibs.2014.02.004
- Lino Cardenas CL, Bourgine J, Cauffiez C, et al. Genetic polymorphisms of glycine N-acyltransferase (GLYAT) in a French Caucasian population. Xenobiotica. 2010;40(12):853–861. doi:10.3109/00498254.2010.519407
- Tian X, Wu L, Jiang M, et al. Downregulation of GLYAT facilitates tumor growth and metastasis and poor clinical outcomes through the PI3K/AKT/snail pathway in human breast cancer. Front Oncol. 2021;11:641399. doi:10.3389/fonc.2021.641399
- Bianchi C, Meregalli C, Bombelli S, et al. The glucose and lipid metabolism reprogramming is grade-dependent in clear cell renal cell carcinoma primary cultures and is targetable to modulate cell viability and proliferation. Oncotarget. 2017;8(69):113502–113515. doi:10.18632/oncotarget.23056
- Di Meo NA, Lasorsa F, Rutigliano M, et al. The dark side of lipid metabolism in prostate and renal carcinoma: novel insights into molecular diagnostic and biomarker discovery. Expert Rev Mol Diagn. 2023;23(4):297–313. doi:10.1080/14737159.2023.2195553
- Lucarelli G, Rutigliano M, Loizzo D, et al. MUC1 tissue expression and its soluble form CA15-3 identify a clear cell renal cell carcinoma with distinct metabolic profile and poor clinical outcome. Int J Mol Sci. 2022;23(22):13968. doi:10.3390/ijms232213968
- Vuong L, Kotecha RR, Voss MH, Hakimi AA. Tumor microenvironment dynamics in clear-cell renal cell carcinoma. Cancer Discov. 2019;9(10):1349–1357. doi:10.1158/2159-8290.CD-19-0499
- Tamma R, Rutigliano M, Lucarelli G, et al. Microvascular density, macrophages, and mast cells in human clear cell renal carcinoma with and without bevacizumab treatment. Urol Oncol. 2019;37(6):355.e311–355.e319. doi:10.1016/j.urolonc.2019.01.025
- Ghini V, Laera L, Fantechi B, et al. Metabolomics to assess response to immune checkpoint inhibitors in patients with non-small-cell lung cancer. Cancers. 2020;12(12):3574. doi:10.3390/cancers12123574
- Corcoran SE, O’Neill LA. HIF1α and metabolic reprogramming in inflammation. J Clin Invest. 2016;126(10):3699–3707. doi:10.1172/JCI84431
- Denk D, Greten FR. Inflammation: the incubator of the tumor microenvironment. Trends Cancer. 2022;8(11):901–914. doi:10.1016/j.trecan.2022.07.002
- Lin H, Fu L, Li P, et al. Fatty acids metabolism affects the therapeutic effect of anti-PD-1/PD-L1 in tumor immune microenvironment in clear cell renal cell carcinoma. J Transl Med. 2023;21(1):343. doi:10.1186/s12967-023-04161-z
- Jeffries KA, Dempsey DR, Farrell EK, et al. Glycine N-acyltransferase-like 3 is responsible for long-chain N-acylglycine formation in N18TG2 cells. J Lipid Res. 2016;57(5):781–790. doi:10.1194/jlr.M062042
- Waluk DP, Schultz N, Hunt MC. Identification of glycine N-acyltransferase-like 2 (GLYATL2) as a transferase that produces N-acyl glycines in humans. FASEB J. 2010;24(8):2795–2803. doi:10.1096/fj.09-148551