Abstract
Background
Aging is recognized as the key risk for intracerebral hemorrhage (ICH). The detailed mechanisms of aging in ICH warrant exploration. This study aimed to identify potential aging-related genes associated with ICH.
Methods
ICH-specific aging-related genes were determined by the intersection of differentially expressed genes (DEGs) between perihematomal tissues and corresponding contralateral parts of four patients with ICH (GSE24265) and 349 aging-related genes obtained from the Aging Atlas database. Gene Ontology (GO), Kyoto Encyclopedia of Genes and Genomes (KEGG) and Gene Set Enrichment Analysis (GSEA) analyses were performed to identify the potential biological functions and pathways in which these ICH-specific aging-related genes may be involved. Then, PPI network was established to identify the hub genes of ICH-specific aging-related genes. Meanwhile, miRNA-mRNA and transcription factor (TF)-mRNA regulatory networks were constructed to further explore the ICH-specific aging-related genes regulation. The relationship between these hub genes and immune infiltration was also further explored. Additional single-cell RNA-seq analysis (scRNA-seq, GSE167593) was used to locate the hub genes in different cell types. Besides, expression levels of the hub genes were validated using clinical samples from our institute and another GEO dataset (GSE206971).
Results
This study identified 24 ICH-specific aging-related genes, including 22 up-regulated and 2 down-regulated genes. The results of GO and KEGG suggested that the ICH-specific aging-related genes mainly enriched in immunity and inflammation-related pathways, suggesting that aging may affect the ich pathogenesis by regulating inflammatory and immune-related pathways.
Conclusion
Our study revealed 24 ICH-specific aging-related genes and their functions highly pertinent to ICH pathogenesis, providing new insights into the impact of aging on ICH.
Introduction
Intracerebral hemorrhage (ICH) is one of the most dangerous stroke diseases, referring to bleeding caused by non-traumatic rupture of blood vessels in the cerebral parenchyma, with a high mortality and disability rate that seriously affects people’s healthy lives.Citation1,Citation2 One-year post-ICH onset, mortality exceeds 50%, with survivors often experiencing functional and cognitive impairments.Citation3,Citation4 In the Global Burden of Disease Study 2019, the global incidence of intracerebral hemorrhage (ICH) was approximately 3.5 million cases, with disproportionately high rates observed in low-income countries, as well as certain regions of Oceania and Southeast Asia.Citation5 Individuals in low-income regions have nearly double the incidence of ICH compared to those in higher-income regions (29.5% compared with 15.8% of all stroke cases in 2019).Citation5 Hypertension, cerebral amyloid angiopathy and anticoagulation are the main causes of ICH in adults.Citation6 Meanwhile, previous studies have also highlighted that patients with haematological disorders could manifest ICH.Citation7,Citation8 The pathological process can be divided into primary injury, which refers to mechanical compression due to a hematoma, and secondary injury, which consists of cytotoxicity of blood, hypermetabolism, excitotoxicity, oxidative stress and inflammation, eventually leading to neuronal apoptosis.Citation9–11
Aging is an inevitable biological process characterized by cell type-specific and tissue-specific changes, leading to numerous chronic and age-related pathologies.Citation12,Citation13 Previous studies have proved that aging is a critical risk factor for ICH. The risk of ICH in elderly individuals is five times higher than their younger counterparts.Citation14 Aging can affect the body in multiple ways, such as cardiovascular and central nervous system changes, interplaying with the multiple risk factors for ICH.Citation15 Cytologically, aging is associated with mitochondrial dysfunction, genomic instability, and cellular senescence.Citation16 Mitochondria play a crucial role in not only supplying energy through ATP production but also in regulating calcium homeostasis and metabolite synthesis, which are essential for ensuring optimal neuronal function and development.Citation17 Mitochondrial dysfunction generally occurs in the initial stage of brain injury, which can exacerbate secondary brain injury.Citation18 Aging-related genes play an important role in the initialization and regulation of cell senescence and may affect neuron and vessel tissues in a sophisticated way.Citation19–21 Recent studies have uncovered the potential roles of aging-related genes in Alzheimer’s Disease,Citation22 periodontitisCitation23 and chronic obstructive pulmonary disease.Citation24 However, how aging-related genes contribute to ICH remains largely unknown.
Single-cell RNA sequencing enables the study of genome-wide express changes at single-cell resolution, offering a powerful solution for analyzing phenotypic variations and intercellular communication dynamics within distinct cell subsets during complex pathological processes.Citation25 Current therapeutic options for ICH include hematoma evacuation, reduction in blood pressure and the administration of haemostatic drugs.Citation26 However, there are no proven medical treatments that can improve the functional outcome of ICH patients. More preclinical studies are needed to elucidate injury mechanisms in ICH and facilitate the development of novel therapies.
Materials and Methods
Data Acquisition
Three hundred and forty-nine aging-related genes were derived from the Aging Atlas database.Citation27 Dataset GSE24265,Citation28 including expression profiles of perihematomal tissues and corresponding contralateral parts (white matter and grey matter) of four patients with ICH, was downloaded from the GEO for further analysis. Meanwhile, dataset GSE206971,Citation29 including microarray data of hematoma basal ganglia tissue of ICH mice model (n = 3, aged 8–10 weeks) and sham operation control, was downloaded to validate the expression of 10 hub ICH-specific aging-related genes.
Different Expression Analysis
The Limma packageCitation30 was used to perform the analysis of differential expression. The expression profiles of perihematomal tissues and normal tissues were compared to identify the differentially expressed genes (DEGs) using the following criteria: | log2 (fold-change) | >1 and adjusted P-value <0.05 (Table S1). The DEGs were intersected with 349 aging-related genes to identify ICH-specific aging-related genes. The Venn diagram of DEGs was generated by the web tool Draw Venn Diagram (Draw Venn Diagram (ugent.be)).
Functional Enrichment Analysis
GSEA of perihematomal tissues was performed by the R package “clusterProfiler”.Citation31 Gene sets were considered significantly enriched genes with a threshold of P <0.05 and false discovery rate (FDR) <0.05. Meanwhile, the GO and KEGG enrichment were also performed using “clusterProfiler”.
Protein–Protein Interaction Network Analysis and Identification of Hub Genes
An online database, STRING,Citation32 which can retrieve the interaction among multiple proteins, was used to predicate protein–protein interactions (PPI) with default parameters. Cytoscape v3.8.2Citation33 was used to visualize the PPI network and Cytohubba,Citation34 a Cytoscape plugin app was used to explore the hub genes.
miRNA–mRNA Interaction Networks
Three databases, miRDB,Citation35 miRTarBase,Citation36 and TargetScan (TargetScanHuman 7.2) were used to predict the potential upstream miRNAs for the ICH-specific aging-related genes based on the regulatory associations. The intersection of the three database results were considered high-confidence miRNAs. Then, a miRNA-mRNA regulatory network was established using Cytoscape software.
TF-mRNA Interaction Networks
The TF-mRNA interaction network was constructed according to the previous work: after selecting 53 TFs from the DEGs, we performed Pearson correlation test to explore the correlation between the expression of 24 ICH-specific aging-related genes and 53 differential expression TFs. Based on the cut-off criteria of P <0.0001 and |Pearson’s correlation coefficient| >0.7, 23 TFs and 23 mRNAs were used to establish the TF-mRNA regulatory network. The TF-mRNA regulatory network was visualized by Cytoscape.
Immune Infiltration Analysis
The R package ‘CIBERSORT’Citation37 was used for analysis of immune cell fractions in ICH tissues and normal controls.
Single-Cell RNA-Seq Data Analysis
The single-cell RNA-seq dataset GSE167593Citation38 was downloaded from GEO database, which contained cerebral cortical samples of ICH mice and normal mice. R package ‘Seurat’Citation39 was used to process the data. The specific parameters were as follows: Low-quality sequencing data was filtered out based on the criteria of <500 expressed genes or 20% mitochondrial transcripts. After normalizing the filtered data by the “NormalizeData” function, top 2000 highly variable genes were identified by the ‘FindVariableFeatures’ function. Then, the “RunPCA” function was applied to perform principal component analysis (PCA) to reduce the dimension of the scRNA-seq data based on the top 2000 genes. We choose dims = 8 and clustered the cells through the ‘FindNeighbors’ and “FindClusters” functions (resolution = 0.3) to find the clusters. Cluster annotation was referred to the previous work.Citation38
Drug Selection and Molecular Docking
DGIdb database (https://dgidb.genome.wustl.edu/)Citation40 was used for drug prediction of hub genes in order to screen potential targeting small molecule drugs for aging-related genes. The drug with highest gene–drug interaction scores were selected to perform small-molecule drug-large-molecule protein docking using AutoDock.Citation41
Immunohistochemistry
As previously reported,Citation42 the fresh tissues obtained during operation were embedded using paraffin after being fixed neutral-buffered formalin (G1101, Servicebio, Wuhan, China) with for 24 hours in room temperature. After that, the tissues were cut into 5-μm sections, followed by deparaffinization, rehydration and immersion. The sections were incubated in NCM Blot Blocking Buffer (P30500, NCM Biotech, Suzhou, China) for 1h to block. After washing the sections three times with PBS, the sections were incubated with the corresponding antibodies (listed in Table S2) at 4° overnight. The sections were washed three times with PBS again and incubated with poly-HRP-conjugated Goat Anti-Rabbit IgG (H+L) (RCA054, Recordbio Biological Technology, Shanghai, China) at room temperature for 2h. The sections were then stained with 3.3′-diaminobenzidine solution and hematoxylin counterstain at room temperature before observation. The percentage of positive cells was scored for analysis. The collection and use of clinical samples is in strict conformity with the declaration of Helsinki.
Statistical Analysis
Statistical analysis was performed in R v.4.1.0 and GraphPad Prism v.6.0 (GraphPad Software, La Jolla, California). The Pearson correlation coefficient was used for correlation analysis. The Wilcoxon rank-sum test was used to analyze the statistical significance of continuous variables. Student’s t-test was used for statistical significance analysis of IHC result of normal control and ICH groups. The difference was statistically significant at the level of P < 0.05.
Results
Identification of Aging-Related DEGs in ICH
shows the flowchart of this study. The gene transcriptome data of the GSE24265 dataset from perihematomal tissues and corresponding contralateral parts (white matter and grey matter) of four patients with ICH were downloaded from the GEO dataset. A total of 467 DEGs, including 338 up-regulated and 129 down-regulated genes were identified using the Limma package with a threshold of P-value <0.05 and | log2(fold-change) | >1 (Table S1). A volcano plot for the distribution of DEGs was generated using R package (). After intersecting DEGs with 349 aging-related genes obtained from the Aging Atlas database, 24 aging-related genes were derived. The Venn diagram and the heatmap of the aging-related DEGs were shown in and .
Figure 2 Identification of differentially expressed aging-related genes in ICH. (A) Volcano plot of DEGs with ICH-specific aging-related genes labeled; (B) Venn diagram of ICH-specific aging-related genes. (C) Heatmap of 24 ICH-specific aging-related genes. ICH, intracerebral hemorrhage; DEGs, differentially expressed genes.
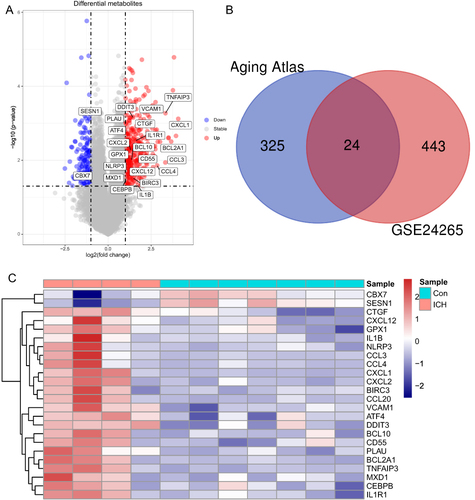
Enrichment Analysis of Aging-Related DEGs
GSEA was performed to elucidate the differentially expressed pathways and GO between the ICH tissues and normal tissues using “clusterProfiler” package. As is shown in , the top 8 enriched GO terms in ICH were ribosomal subunit, neutrophil migration, cytosolic ribosome, granulocyte migration, chemokine receptor binding, myeloid leukocyte migration, humoral immune response and leukocyte chemotaxis, and top 8 enriched KEGG terms in ICH were ribosome, rheumatoid arthritis, coronavirus disease - COVID-19, IL-17 signaling pathway Viral protein interaction with cytokine and cytokine receptor, cytokine–cytokine receptor interaction, Phagosome, and antigen processing and presentation, which indicated that molecular alteration in ICH group was closely related to Inflammation and immune-related pathways.
Figure 3 GSEA analysis of ICH and control groups reveal the potential mechanism involved in ICH. The top 8 gene ontologies (A) and KEGG pathways (B) based on the normalized enrichment score (NES) between ICH and control groups. GSEA, gene set enrichment analysis.
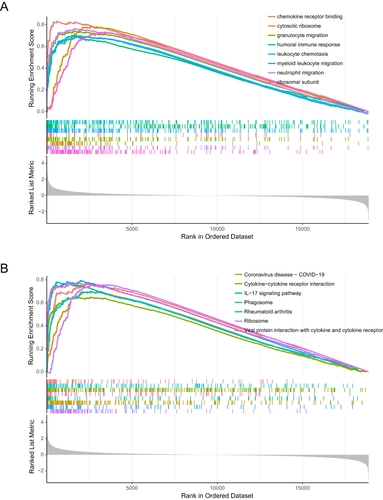
The R package “clusterProfiler” was used to perform GO and KEGG enrichment analyses of ICH-specific aging-related genes to further understand the biological process that would be affected. The annotations of the GO terms suggested the 24 aging-related DEGs were most involved in cellular response to biotic stimulus, cellular response to lipopolysaccharide, cellular response to molecule of bacterial origin, response to lipopolysaccharide, response to molecule of bacterial origin, cytokine-mediated signaling pathway, tertiary granule, RNA polymerase II transcription regulator complex, chemokine activity, chemokine receptor binding, cytokine activity, cytokine receptor binding, G protein-coupled receptor binding and CXCR chemokine receptor binding (). The KEGG enrichment analysis showed that the aging-related genes play a key role in NF-kappa B signaling pathway, TNF signaling pathway, lipid and atherosclerosis, rheumatoid arthritis, IL-17 signaling pathway, viral protein interaction with cytokine and cytokine receptor, cytokine–cytokine receptor interaction, NOD-like receptor signaling pathway, chemokine signaling pathway and human cytomegalovirus infection pathway (). It is worth noting that the enrichment results of 24 aging-related genes are nearly identical to the GSEA results of ICH, which indicates the potentially important role of the 24 aging-related genes in ICH.
Protein–Protein Interactions Network and Identification of Hub Aging-Related DEGs
The 24 aging-related DEGs were uploaded to the STRING online website to derive the PPI network. According to the result, a total of 22 DEGs were consequently extracted among 24 genes uploaded with default parameters. The PPI network containing 22 nodes and 85 edges was visualized by Cytoscape (). shows the degree of each gene calculated by CytoHubba, and CXCL2, CXCL12, CCL4, CCL3, VCAM1, IL1R1, TNFAIP3, IL1B, CCL20 and CXCL1 were defined as hub genes according to these degrees (). To further understand the correlation of the ten hub genes, we explored the correlation between their expression levels. Interestingly, all of these hub genes showed a significant co-expression trend. Furthermore, KEGG and GO enrichment analyses were also performed based on the ten hub genes. Similar to the result of 24 ICH-specific aging-related genes, the 10 hub genes also enriched in inflammatory and immune-related pathways ( and ), further illustrating the critical role of these hub genes in ICH.
Table 1 The Top 10 KEGG Enriches the ten Hub Genes Based on p-value
Table 2 The Top 10 GO Enriches the ten Hub Genes Based on p-value
Figure 5 Protein–protein interaction (PPI) network of ICH-specific aging-related genes (A) PPI network of 22 IHC-specific aging-related genes analyzed using STRING online database. (B) The degree of each ICH-specific aging-related gene calculated by the cytoHubba of Cytoscape. (C) PPI network of hub genes with top 10 degree. (D) The correlation between the expression of 24 ICH-specific aging-related genes with 10 hub genes highlighted. (* P<0.05, Pearson correlation test).
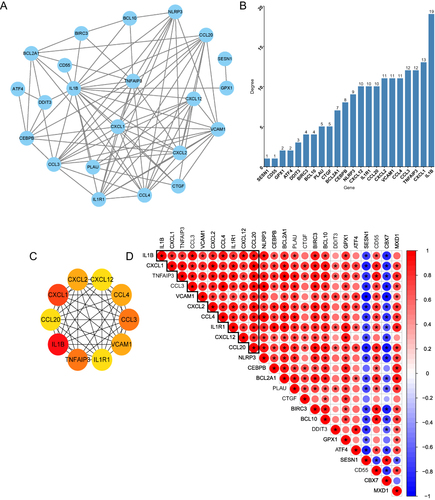
miRNA Regulatory Network
TargetScan, miRTarBase and miRDB databases were used to predict miRNAs that might play a regulatory role over the 24 ICH-specific aging-related genes. The results of three databases were intersected in order to obtain the high confidence miRNAs. Finally, a miRNA-mRNA regulatory network containing 14 mRNA nodes, 89 miRNA nodes and 98 edges was established and visualized by Cytoscape (). Has-miR-374a-5p, has-miR-21-5p, has-miR-125b-5p, has-miR-23a-3p, has-miR-19a-3p, has-miR-19b-3p, has-miR-29a-3p, has-miR-23b-3p may function as ceRNAs among these 89 miRNAs.
TF Regulatory Network
To elucidate the potential regulatory mechanisms of aging-related DEGs, we built a TF regulatory network. According to previous study,Citation43 the TF regulatory network was established in three steps: (1) 53 different expressed TFs and 24 aging-related DEGs were selected. (2) The correlation test between the expression of each TF and each aging-related DEGs was performed using Pearson correlation test with a threshold of |Pearson’s correlation coefficient| >0.7 and P-value <0.0001, which was shown in Table S3. (3) Cytoscape was used to construct and visualize the regulatory network according to TFs and aging-related DEGs that were significantly linked at transcriptional level. As shown in , PLEK regulated most of the aging-related DEGs, suggesting the dominant position of PLEK in the regulatory network. IL1R1 was predicted to have the greatest TF abundance.
Immuno-Correlation Analysis of ICH Tissues and Aging-Related Hub Genes
ICH results in the activation of multiple immune cells. R package “CIBERSORT” was used to analyze the differences in the immune landscape between ICH tissues and normal controls. and illustrate the immune infiltration in ICH tissues and normal controls. T cells CD8, T cells follicular helper and Neutrophils were significantly upregulated in normal control tissues, while T cells gamma delta were upregulated in ICH tissues. Considering that the aging-related hub genes are mainly enriched in inflammation-related pathways, we further analysed the relationship between these aging-related hub genes and immune cell infiltration (). Interestingly, the relationship between these aging-related genes and immune cells showed the same trend that most aging-related genes are negatively correlated with neutrophils, macrophages M2, B cells naïve and T cells follicular helper. However, they are strongly positively correlated with mast cells activated. Mast cells are located along blood vessels in the brain.Citation44 In the early stages of ICH, the activation of mast cells contributed to inflammation leading to blood–brain barrier disruption, brain edema and hematoma expansions after ICH.Citation45 The strong correlation between mast cells and aging-related hub genes further suggests that aging-related genes further exacerbate brain damage after ICH mainly by promoting inflammation, which is the same as the results of the previous KEGG enrichment analysis.
Single-Cell Analysis of Hub Genes
The Seurat package was used to perform the single-cell analysis. Cluster analysis through uniform manifold approximation and projection (UMAP) showed that the cells from both control and ICH groups could be divided into 7 clusters: oligodendrocyte, microglia, endothelial cell, neuroblast, astrocyte, choroid plexus cell and T cell ( and ). Marker genes used to identify each subgroup were shown in Figure S1. As shown in and , Cxcl2, Ccl4, Ccl3, Tnfaip3 and Il1b were obviously upregulated in the endothelial cell in the ICH model. The endothelial cell is an important component of the blood–brain barrier, maintaining a safe and homeostatic milieu for proper neuronal function and synaptic transmission.Citation46 Previous works had reported that proinflammatory cytokines, chemokines, adhesion molecules and matrix metalloproteinases (MMPs) broke down the blood–brain barrier (BBB) and destroyed surrounding tissues, which contributed to ICH-induced brain injury.Citation46 Considering the strong correlation between these hub genes and activated mast cells, we speculated that activated mast cells could lead to inflammatory responses in endothelial cells by upregulating Cxcl2, Ccl4, Ccl3, Tnfaip3 and Il1b, resulting in damage to the blood–brain barrier. Hence, we queried the GDIdb database for prospective small-molecule drugs. The result of CCL4 and IL1B targeting drugs was shown in . The molecule drug with the highest gene–drug interaction scores was selected to perform molecular docking using AutoDock (). The energy required for docking clodronic to CCL4 is –3.98 Kcal/mol, and the energy required for docking TT-301 to IL1B is −7.85 Kcal/mol. Amino acid residues GLU-30, THR-31 and ALA-39 in CCL4 and clodronic form hydrogen bonds tightly. Amino acid residues GLN-38 in IL1B and TT-301 form hydrogen bonds tightly. Overall, it showed that CCL4 and IL1B had a high affinity for their docked compounds.
Table 3 Identification of Drugs for CCL4 and IL1B in ICH
Validation of the Expression Levels of Hub Genes in ICH Tissues
To verify the expression of part of hub genes at the protein levels in ICH tissues, we collected 3 cases of ICH samples and 3 cases of peritumoral samples for immunohistochemistry (IHC). The clinical characteristics of patients were described in Table S4. The results showed that CCL20, CXCL1 and CXCL12 were significantly upregulated in ICH tissues ( and ). Meanwhile, we also downloaded the gene expression profile of hematoma basal ganglia tissue of ICH mice model GSE206971 from GEO. As shown in and , the expression of Ccl4, Ccl3, Cxcl12 and Il1r1 were significantly up-regulated in ICH models compared to the sham operation models (n = 3, aged 8–10 weeks). Meanwhile, the expression of Tnfaip3, Ccl20, II1b, Cxcl2, Vcam1 and Cxcl1 also showed an upregulation trend in the ICH sample, although not statistically significant.
Figure 11 Validation of hub ICH-specific aging-related genes using ICH tissues and GSE206971. P-values were calculated using a two-sided unpaired Student’s t-test. (A–D) The expression of CCL20, CXCL1 and CXCL12 was upregulated in ICH at protein level. (E–N) The RNA-Seq expression of hub ICH-specific aging-related genes. (*p < 0.05, **p<0.01, ***p<0.001; ns, non-significance. Student’ t-test, n=3, aged 8–10 weeks).
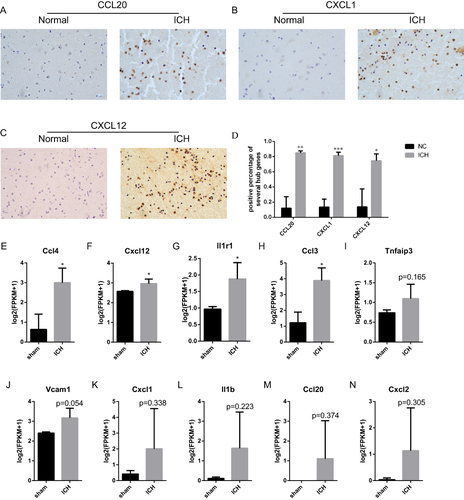
Discussion
Elevated systemic chronic inflammation is a pivotal hallmark of aging and exerts a crucial impact on multiple age-related conditions in the elderly population.Citation47 Numerous studies have proved that the controversial role of inflammation in the secondary brain injury of ICH.Citation48,Citation49 Based on the results of enrichment analysis of aging-related genes, we suggested that aging could promote inflammatory responses in the development of ICH. 24 ICH-specific aging-related genes were identified through different expression analysis. Our GO and KEGG enrichment analysis revealed that ICH-specific genes participated in inflammatory, cellular communication and immune-related pathways, indicative of the prominent biological significance of ICH-specific aging-related genes in ICH pathogenesis. Using the PPI network, we further explored the potential interactions between ICH-specific aging-related genes. The ten hub genes (CXCL2, CXCL12, CCL4, CCL3, VCAM1, IL1R1, TNFAIP3, IL1B, CCL20, CXCL1) were selected according to degree calculated by CytoHubba. Additionally, the KEGG enrichment analysis of hub genes showed similarities to ICH-specific aging-related genes, illustrating the importance of hub genes. According to Wasserman et.al, aging is associated with an increased inflammatory response after ICH, but it does not have a significant impact on neuron death.Citation50 Meanwhile, Zhang et al also founded that ICH induced more pronounced histone mark redistribution in neuroinflammation-associated genes in late-aging rat brains.Citation51 Interestingly, more than half of hub aging-related genes belong to chemokines, which are critical for the function of the innate immune system, including CCL3, CCL4, CCL20, CXCL1, CXCL2 and CXCL12.Citation14 According to previous studies, chemokines play an important role in neuroinflammation evoked by intracerebral blood.Citation52 CCL3 displays diverse proinflammatory functions. CCL3 is secreted by microglia, astrocytes, hippocampal neurons, and cerebral endothelial cells in the central nervous system.Citation53 It has implications in several conditions, including ischemic stroke, seizures, and traumatic injuries.Citation54–56 Earlier investigations have indicated that cytokines and lipopolysaccharides can upregulate CCL3 expression in human brain endothelial cells (ECs).Citation57 Our single-cell analysis also highlights the predominant upregulation of CCL3 in ECs after intracerebral hemorrhage (ICH), underscoring its significant role in blood-brain barrier (BBB) disruption resulting from ICH. Previous study reported that CCL4 was increased in the monocytes and blood of elderly individuals.Citation58 CCL4 may affect numerous immune cell functions including migration, growth, and differentiation which are altered during aging, yet its exact impaction on the decline of immune function and the increase in disease with aging remains to be elucidate. CCL20 is upregulated in ischemic brain injury and subarachnoid hemorrhage, leading to an inflammatory cascade reaction. CCL20 knockdown in neurons could decrease the percentage of apoptotic neurons.Citation59 Circulating CXCL1 was elevated significantly after traumatic brain injury and associated with poor prognosis, suggesting the potential biomarker role of CXCL1 in traumatic brain injury.Citation60 Besides, the abnormal activation of CXCL1-IL-8 signaling contributes to acute and chronic seizures in mice, representing a potential new target for status epilepticus.Citation61 Meanwhile, circulating CXCL12 was enhanced after ICH and could serve as a novel biomarker for severity and prognosis following hemorrhagic stroke.Citation62 The expression of CXCL2 is notably elevated in ECs post ICH.Citation57 A prior study has demonstrated that inhibiting CXCR2, the receptor for CXCL2, contributes to functional recovery in ICH mice. Also, Matsushita et al revealed that interruption of CXCL2 signaling could ameliorate neurological deficits after ICH, providing a promising target for ICH treatment.Citation63 Meanwhile, Li reported that CXCL12 could stimulate endothelial progenitor cells (EPCs) to initiate angiogenesis via activation of the CXCR4 pathway following ICH.Citation64 Additional hub aging-related genes also contribute significantly to ICH. Meng et al reported that overexpressing TNFAIP3 in ICH-afflicted mice attenuates brain edema and reduces neuronal necrosis after ICH. While localized TNFAIP3 overexpression might not suffice in lowering post-ICH mortality rates.Citation65 Simultaneously, Lu et al found that TNFAIP3 could suppress microglial necroptosis following ICH by ubiquitinating RIP3, implying TNFAIP3’s novel potential as an ICH therapeutic target.Citation66 IL1R1 can interact with the RIP1/RIP3 necrotic complex, thereby triggering neuronal necroptosis. Targeting the assembly of IL-1R1/RIP1/RIP3 shows promise as a therapeutic approach for ICH patients.Citation67 VCAM1 functions as both an extracellular matrix (ECM) and cell adhesion protein. It facilitates interactions between mast cells (MCs) and other cells, as well as components of the ECM, fostering structures such as adherens junctions and focal adhesion sites, thereby initiating a signaling cascade.Citation68 We observed significantly heightened VCAM1 expression in choroidal cells subsequent to ICH, specifically within endothelial cells. Furthermore, a substantial correlation between VCAM1 expression and MCs presence was noted. This underscores the crucial role of VCAM1 in mediating MCs enrichment within the choroid post ICH. Further work will be needed to explore these aging-related genes’ role in ICH.
Meanwhile, our study also highlights the role of mast cell in ICH. Mast cells are located along blood vessels in the brain and are important source of early inflammatory molecules following ICH.Citation44 The pro-inflammatory effects of activated mast cells could induce rapid opening of the blood–brain barrier while promoting brain edema and hematoma expansions after ICH.Citation44,Citation69 Aging-related hub genes are strongly correlated with activated mast cells. Meanwhile, Cxcl2, Ccl4, Ccl3, Tnfaip3 and Il1b were mainly upregulated in endothelial cells after ICH. It suggested that these aging-related genes could play an important role in activated mast cell-mediated damage to blood–brain barrier, providing novel therapeutic targets for ICH. Mast cell-mediated endothelial injury is prevalent in the central nervous system,Citation70 respiratory system,Citation71 cardiovascular system,Citation72 and intestinal system.Citation73 Previous research has demonstrated that mast cells can trigger inflammatory responses in human cerebral microvascular endothelial cells through degranulation, leading to disruption of the blood–brain barrier (BBB) during neurological inflammation caused by ICH.Citation44,Citation74 This disruption facilitates inflammatory cell infiltration, ultimately resulting in cerebral edema and hemorrhage.Citation44,Citation74 Nevertheless, the extent to which the heightened inflammation observed in aging individuals contributes to the pro-inflammatory actions of mast cells necessitates further investigation. The result of molecular docking showed that CCL4 and IL1B had a high affinity for colonic and TT-301, respectively. Prior research indicates that TT-301 administration post-injury enhances outcomes in mouse models of traumatic brain injury and intracerebral hemorrhage by modulating neuroinflammation.Citation75 Clodronic acid, a non-nitrogen bisphosphonate effective in treating osteoporosis, also depletes brain perivascular macrophages, reducing blood–brain barrier permeability around arterioles.Citation76,Citation77 This suggests potential benefits in treating intracerebral hemorrhage-related secondary injuries. However, introducing clodronic acid in murine intracerebral hemorrhage models diminishes microglia, impacting hematoma clearance and neurological recovery.Citation78 Addressing how clodronic acid can target perivascular macrophages without affecting microglia is crucial for successful intracerebral hemorrhage treatment.
Nevertheless, there are several limitations in this study. Firstly, although we validated the results using another dataset, our interpretation may contain some bias due to relatively smaller samples, and our conclusion needs to be verified using a larger ICH cohort. Secondly, due to constraints on sample size, we refrained from investigating cerebral haemorrhage across distinct clinical presentations. A subset of cerebral hemorrhage patients present with a lacunar syndrome and exhibit favorable prognoses,Citation79,Citation80 suggesting potential variations in the involvement of age-related genes. Future studies will require larger clinical cohorts to elucidate this further. Thirdly, only the transcriptional levels of ICH-specific aging-related genes were verified in the gene expression profiles. The mechanisms underlying the functions of these aging-related genes were not explored in cellular and animal experiment. Further exploration is required in the future.
Conclusion
In brief, 24 ICH-specific aging-related genes associated were identified using bioinformatic analysis. Our study demonstrates that these genes may affect the ICH pathogenesis by regulating inflammatory and immune-related pathways, such as chemokine receptor binding, humoral immune response, neutrophil migration, IL-17 signaling pathway, cytokine–cytokine receptor interaction pathway. In addition, we have identified two small molecule drugs TT-301 and clodronic, targeting IL-1B and CCL4 separately through molecular docking. Meanwhile, further cellular and animal-level experiments are needed in the future to further explore the specific mechanisms of these hub genes and the effectiveness of small-molecule drugs.
Ethical Approval and Consent to Participate
The study involving human participants was reviewed and approved by the Ethics Committee at Zhongnan Hospital of Wuhan University (Kelun-2017005). Written informed consent to participate in this study was provided by the participants’ legal guardian/next to kin. No animals were used for studies that are the basis of this research.
Disclosure
The authors report no conflicts of interest in this work.
Data Sharing Statement
The gene expression profiles used in this study are available in the Gene Expression Omnibus (GSE24265, GEO Accession viewer (nih.gov); GSE167593, GEO Accession viewer (nih.gov); GSE206971, GEO Accession viewer (nih.gov)). The results generated during the present study are available from the corresponding author on request.
Additional information
Funding
References
- Duan T, Li L, Yu Y, et al. Traditional Chinese medicine use in the pathophysiological processes of intracerebral hemorrhage and comparison with conventional therapy. Pharmacol Res. 2022;179:106200. doi:10.1016/j.phrs.2022.106200
- Tatlisumak T, Cucchiara B, Kuroda S, Kasner SE, Putaala J. Nontraumatic intracerebral haemorrhage in young adults. Nat Rev Neurol. 2018;14(4):237–250. doi:10.1038/nrneurol.2018.17
- Poon MT, Fonville AF, Al-Shahi SR. Long-term prognosis after intracerebral haemorrhage: systematic review and meta-analysis. J Neurol Neurosurg Psychiatry. 2014;85(6):660–667. doi:10.1136/jnnp-2013-306476
- Moulin S, Labreuche J, Bombois S, et al. Dementia risk after spontaneous intracerebral haemorrhage: a prospective cohort study. Lancet Neurol. 2016;15(8):820–829. doi:10.1016/S1474-4422(16)00130-7
- Feigin VL, Stark BA, Johnson CO. Global, regional, and national burden of stroke and its risk factors, 1990-2019: a systematic analysis for the Global Burden of Disease Study 2019. Lancet Neurol. 2021;20(10):795–820. doi:10.1016/S1474-4422(21)00252-0
- Sheth KN. Spontaneous intracerebral hemorrhage. N Engl J Med. 2022;387(17):1589–1596. doi:10.1056/NEJMra2201449
- Arboix A, Besses C. Cerebrovascular disease as the initial clinical presentation of haematological disorders. Eur Neurol. 1997;37(4):207–211. doi:10.1159/000117444
- Dorgalaleh A, Farshi Y, Haeri K, Ghanbari OB, Ahmadi A. Risk and management of intracerebral hemorrhage in patients with bleeding disorders. Semin Thromb Hemost. 2022;48(3):344–355. doi:10.1055/s-0041-1740566
- Xu J, Chen Z, Yu F, et al. IL-4/STAT6 signaling facilitates innate hematoma resolution and neurological recovery after hemorrhagic stroke in mice. Proc Natl Acad Sci U S A. 2020;117(51):32679–32690. doi:10.1073/pnas.2018497117
- Chen S, Peng J, Sherchan P, et al. TREM2 activation attenuates neuroinflammation and neuronal apoptosis via PI3K/Akt pathway after intracerebral hemorrhage in mice. J Neuroinflammation. 2020;17(1):168. doi:10.1186/s12974-020-01853-x
- Luo X, Zhu Q, Zhang J, Huang Q, Xie Z, Cheng Y. The double roles of the prostaglandin e2 EP2 receptor in intracerebral hemorrhage. Curr Drug Targets. 2017;18(12):1377–1385. doi:10.2174/1389450117666151209122826
- Selman M, Pardo A. Fibroageing: an ageing pathological feature driven by dysregulated extracellular matrix-cell mechanobiology. Ageing Res Rev. 2021;70:101393. doi:10.1016/j.arr.2021.101393
- Partridge L, Fuentealba M, Kennedy BK. The quest to slow ageing through drug discovery. Nat Rev Drug Discov. 2020;19(8):513–532. doi:10.1038/s41573-020-0067-7
- Watson N, Bonsack F, Sukumari-Ramesh S. Intracerebral hemorrhage: the effects of aging on brain injury. Front Aging Neurosci. 2022;14:859067. doi:10.3389/fnagi.2022.859067
- Camacho E, Lopresti MA, Bruce S, et al. The role of age in intracerebral hemorrhages. J Clin Neurosci. 2015;22(12):1867–1870. doi:10.1016/j.jocn.2015.04.020
- Carlos L, Maria AB, Linda P, Manuel S, Guido K. The hallmarks of aging. Cell. 2013;153(6):1.
- Li Y, Liu H, Tian C, et al. Targeting the multifaceted roles of mitochondria in intracerebral hemorrhage and therapeutic prospects. Biomed Pharmacother. 2022;148:112749. doi:10.1016/j.biopha.2022.112749
- Ahluwalia M, Kumar M, Ahluwalia P, et al. Rescuing mitochondria in traumatic brain injury and intracerebral hemorrhages - a potential therapeutic approach. Neurochem Int. 2021;150:105192. doi:10.1016/j.neuint.2021.105192
- Zhai WY, Duan FF, Chen S, et al. An Aging-Related gene Signature-Based model for risk stratification and prognosis prediction in lung squamous carcinoma. Front Cell Develop Biol. 2022;2022:10.
- Kritsilis M, Rizou S, Koutsoudaki PN, Evangelou K, Gorgoulis VG, Papadopoulos D. Ageing, cellular senescence and neurodegenerative disease. Int J Mol Sci. 2018;19(10). doi:10.3390/ijms19102937
- Donato AJ, Machin DR, Lesniewski LA. Mechanisms of dysfunction in the aging vasculature and role in Age-Related disease. Circ Res. 2018;123(7):825–848. doi:10.1161/CIRCRESAHA.118.312563
- Zhang Q, Li J, Weng L. Identification and validation of Aging-Related genes in Alzheimer’s disease. Front Neurosci. 2022;16:905722. doi:10.3389/fnins.2022.905722
- Peng L, Chen H, Wang Z, He Y, Zhang X. Identification and validation of a classifier based on hub aging-related genes and aging subtypes correlation with immune microenvironment for periodontitis. Front Immunol. 2022;13:1042484. doi:10.3389/fimmu.2022.1042484
- Chen S, Zhan Y, Chen J, et al. Identification and validation of genetic signature associated with aging in chronic obstructive pulmonary disease. Aging (Albany NY). 2022;14(20):8568–8580. doi:10.18632/aging.204358
- Zhang S, Li X, Lin J, Lin Q, Wong KC. Review of single-cell RNA-seq data clustering for cell-type identification and characterization. RNA. 2023;29(5):517–530. doi:10.1261/rna.078965.121
- Schrag M, Kirshner H. Management of intracerebral hemorrhage: JACC focus seminar. J Am Coll Cardiol. 2020;75(15):1819–1831. doi:10.1016/j.jacc.2019.10.066
- Aging Atlas Consortium. Aging Atlas: a multi-omics database for aging biology. Nucleic Acids Res. 2020;49(D1):1.
- Anna R, Anna V, Teresa G, et al. Brain perihematoma genomic profile following spontaneous human intracerebral hemorrhage. PLoS One. 2017;6(2):1.
- Zheng Y, Fan L, Xia S, et al. Role of complement C1q/C3-CR3 signaling in brain injury after experimental intracerebral hemorrhage and the effect of minocycline treatment
. Front Immunol. 2022;13:1.
- Wettenhall JM, Smyth GK. LimmaGUI: a graphical user interface for linear modeling of microarray data. Bioinformatics. 2004;20(18):3705–3706. doi:10.1093/bioinformatics/bth449
- Yu G, Wang L, Han Y, He Q. ClusterProfiler: an R package for comparing biological themes among gene clusters. Omics. 2012;16:5. doi:10.1089/omi.2011.0118
- Szklarczyk D, Franceschini A, Wyder S, et al. STRING v10: protein-protein interaction networks, integrated over the tree of life. Nucleic Acids Res. 2015;43:D447–D452. doi:10.1093/nar/gku1003
- Shannon P, Markiel A, Ozier O, et al. Cytoscape: a software environment for integrated models of biomolecular interaction networks. Genome Res. 2003;13(11):2498–2504. doi:10.1101/gr.1239303
- Chin C, Chen S, Wu H, Ho C, Ko M, Lin C. CytoHubba: identifying hub objects and sub-networks from complex interactome. BMC Syst Biol. 2014;8. doi:10.1186/1752-0509-8-S4-S11
- Chen Y, Wang X. MiRDB: an online database for prediction of functional microRNA targets. Nucleic Acids Res. 2020;48(D1):D127–D131. doi:10.1093/nar/gkz757
- Huang H, Lin Y, Li J, et al. MiRTarBase 2020: updates to the experimentally validated microRNA-target interaction database. Nucleic Acids Res. 2020;48(D1):1.
- Chen B, Khodadoust MS, Liu CL, Newman AM, Alizadeh AA. Profiling tumor infiltrating immune cells with CIBERSORT. Methods Mol Biol. 2018;1711:243–259. doi:10.1007/978-1-4939-7493-1_12
- Shi X, Luo L, Wang J, et al. Stroke subtype-dependent synapse elimination by reactive gliosis in mice. Nat Commun. 2021;12(1):6943. doi:10.1038/s41467-021-27248-x
- Satija R, Farrell JA, Gennert D, Schier AF, Regev A. Spatial reconstruction of single-cell gene expression data. Nat Biotechnol. 2015;33(5):495–502. doi:10.1038/nbt.3192
- Cotto KC, Wagner AH, Feng YY, et al. DGIdb 3.0: a redesign and expansion of the drug-gene interaction database. Nucleic Acids Res. 2018;46(D1):D1068–D1073. doi:10.1093/nar/gkx1143
- Eberhardt J, Santos-Martins D, Tillack AF, Forli S. AutoDock Vina 1.2.0: new docking methods, expanded force field, and python bindings. J Chem Inf Model. 2021;61(8):3891–3898. doi:10.1021/acs.jcim.1c00203
- Wang B, Wei W, Long S, et al. CENPA acts as a prognostic factor that relates to immune infiltrates in gliomas. Front Neurol. 2022;13:1015221. doi:10.3389/fneur.2022.1015221
- Wang Z, Zhu J, Liu Y, et al. Development and validation of a novel immune-related prognostic model in hepatocellular carcinoma. J Transl Med. 2020;18(1):67. doi:10.1186/s12967-020-02255-6
- Strbian D, Tatlisumak T, Ramadan UA, Lindsberg PJ. Mast cell blocking reduces brain edema and hematoma volume and improves outcome after experimental intracerebral hemorrhage. J Cereb Blood Flow Metab. 2007;27(4):795–802. doi:10.1038/sj.jcbfm.9600387
- Marinkovic I, Mattila OS, Strbian D, et al. Evolution of intracerebral hemorrhage after intravenous tPA: reversal of harmful effects with mast cell stabilization. J Cereb Blood Flow Metab. 2014;34(1):176–181. doi:10.1038/jcbfm.2013.189
- Chen S, Li L, Peng C, et al. Targeting oxidative stress and inflammatory response for Blood-Brain barrier protection in intracerebral hemorrhage. Antioxid Redox Signal. 2022;37(1–3):115–134. doi:10.1089/ars.2021.0072
- Li X, Li C, Zhang W, Wang Y, Qian P, Huang H. Inflammation and aging: signaling pathways and intervention therapies. Signal Transduct Target Ther. 2023;8(1):239. doi:10.1038/s41392-023-01502-8
- Tschoe C, Bushnell CD, Duncan PW, Alexander-Miller MA, Wolfe SQ. Neuroinflammation after intracerebral hemorrhage and potential therapeutic targets. J Stroke. 2020;22(1):29–46. doi:10.5853/jos.2019.02236
- Ohashi SN, Delong JH, Kozberg MG, et al. Role of inflammatory processes in hemorrhagic stroke. Stroke. 2023;54(2):605–619. doi:10.1161/STROKEAHA.122.037155
- Wasserman JK, Yang H, Schlichter LC. Glial responses, neuron death and lesion resolution after intracerebral hemorrhage in young vs. Aged rats. Eur J Neurosci. 2008;28(7):1316–1328. doi:10.1111/j.1460-9568.2008.06442.x
- Zhang Q, Kong WL, Yuan JJ, et al. Redistribution of histone marks on inflammatory genes associated with intracerebral Hemorrhage-Induced acute brain injury in aging rats. Front Neurosci. 2022;16:639656. doi:10.3389/fnins.2022.639656
- Koper OM, Kamińska J, Sawicki K, Kemona H. CXCL9, CXCL10, CXCL11, and their receptor (CXCR3) in neuroinflammation and neurodegeneration. Advan Clin Experim Med. 2018;27(6). doi:10.17219/acem/68846
- Wang J, Bian L, Du Y, et al. The roles of chemokines following intracerebral hemorrhage in animal models and humans. Front Mol Neurosci. 2022;15:1091498. doi:10.3389/fnmol.2022.1091498
- Huang L, Ma Q, Li Y, Li B, Zhang L. Inhibition of microRNA-210 suppresses pro-inflammatory response and reduces acute brain injury of ischemic stroke in mice. Exp Neurol. 2018;300:41–50. doi:10.1016/j.expneurol.2017.10.024
- Arisi GM, Foresti ML, Katki K, Shapiro LA. Increased CCL2, CCL3, CCL5, and IL-1beta cytokine concentration in piriform cortex, hippocampus, and neocortex after pilocarpine-induced seizures. J Neuroinflammation. 2015;12:129. doi:10.1186/s12974-015-0347-z
- Ciechanowska A, Popiolek-Barczyk K, Pawlik K, et al. Changes in macrophage inflammatory protein-1 (MIP-1) family members expression induced by traumatic brain injury in mice. Immunobiology. 2020;225(3):151911. doi:10.1016/j.imbio.2020.151911
- Chui R, Dorovini-Zis K. Regulation of CCL2 and CCL3 expression in human brain endothelial cells by cytokines and lipopolysaccharide. J Neuroinflammation. 2010;7:1. doi:10.1186/1742-2094-7-1
- Cheng N, Chen X, Kim J, et al. MicroRNA-125b modulates inflammatory chemokine CCL4 expression in immune cells and its reduction causes CCL4 increase with age. Aging Cell. 2015;14(2):200–208. doi:10.1111/acel.12294
- Liao L, Zhang M, Gu Y, Sun X. Targeting CCL20 inhibits subarachnoid hemorrhage-related neuroinflammation in mice. Aging. 2020;12(14):14849–14862. doi:10.18632/aging.103548
- Chen Y, Wang Y, Xu J, et al. Multiplex assessment of serum chemokines CCL2, CCL5, CXCL1, CXCL10, and CXCL13 following traumatic brain injury. Inflammation. 2022;2022:1.
- Di Sapia R, Zimmer TS, Kebede V, et al. CXCL1-CXCR1/2 signaling is induced in human temporal lobe epilepsy and contributes to seizures in a murine model of acquired epilepsy. Neurobiol Dis. 2021;158(prepublish):105468. doi:10.1016/j.nbd.2021.105468
- Schutt RC, Burdick MD, Strieter RM, Mehrad B, Keeley EC. Plasma CXCL12 levels as a predictor of future stroke. Stroke. 2012;43(12):3382–3386. doi:10.1161/STROKEAHA.112.660878
- Matsushita H, Hijioka M, Ishibashi H, et al. Suppression of CXCL2 upregulation underlies the therapeutic effect of the retinoid Am80 on intracerebral hemorrhage in mice. J Neurosci Res. 2014;92(8):1024–1034. doi:10.1002/jnr.23379
- Li Y, Chang S, Li W, et al. Cxcl12-engineered endothelial progenitor cells enhance neurogenesis and angiogenesis after ischemic brain injury in mice. Stem Cell Res Ther. 2018;9(1):139. doi:10.1186/s13287-018-0865-6
- Meng Z, Zhao T, Zhou K, et al. A20 ameliorates intracerebral Hemorrhage-Induced inflammatory injury by regulating TRAF6 polyubiquitination. J Immunol. 2017;198(2):820–831. doi:10.4049/jimmunol.1600334
- Lu J, Sun Z, Fang Y, et al. Melatonin suppresses microglial necroptosis by regulating deubiquitinating enzyme a20 after intracerebral hemorrhage. Front Immunol. 2019;10:1360. doi:10.3389/fimmu.2019.01360
- Chu X, Wu X, Feng H, et al. Coupling between Interleukin-1R1 and necrosome complex involves in Hemin-Induced neuronal necroptosis after intracranial hemorrhage. Stroke. 2018;49(10):2473–2482. doi:10.1161/STROKEAHA.117.019253
- Pastwinska J, Zelechowska P, Walczak-Drzewiecka A, Brzezinska-Blaszczyk E, Dastych J. The art of mast cell adhesion. Cells-Basel. 2020;9(12). doi:10.3390/cells9122664
- Akyol GY, Manaenko A, Akyol O, et al. IVIG activates FcgammaRIIB-SHIP1-PIP3 Pathway to stabilize mast cells and suppress inflammation after ICH in mice. Sci Rep. 2017;7(1):15583. doi:10.1038/s41598-017-15455-w
- Wu ML, Xie C, Li X, Sun J, Zhao J, Wang JH. Mast cell activation triggered by SARS-CoV-2 causes inflammation in brain microvascular endothelial cells and microglia. Front Cell Infect Microbiol. 2024;14:1358873. doi:10.3389/fcimb.2024.1358873
- Joulia R, Puttur F, Stolting H, et al. Mast cell activation disrupts interactions between endothelial cells and pericytes during early life allergic asthma. J Clin Invest. 2024;134(6). doi:10.1172/JCI173676
- Harper RL, Fang F, San H, et al. Mast cell activation and degranulation in acute artery injury: a target for post-operative therapy. FASEB J. 2023;37(7):e23029. doi:10.1096/fj.202201745RR
- Bischoff SC. Mast cells in gastrointestinal disorders. Eur J Pharmacol. 2016;778:139–145. doi:10.1016/j.ejphar.2016.02.018
- Parrella E, Porrini V, Benarese M, Pizzi M. The role of mast cells in stroke. Cells-Basel. 2019;8(5). doi:10.3390/cells8050437
- James ML, Wang H, Cantillana V, et al. TT-301 inhibits microglial activation and improves outcome after central nervous system injury in adult mice. Anesthesiology. 2012;116(6):1299–1311. doi:10.1097/ALN.0b013e318253a02a
- Mccloskey E, Paterson AH, Powles T, Kanis JA. Clodronate. Bone. 2021;143:115715. doi:10.1016/j.bone.2020.115715
- Santisteban MM, Ahn SJ, Lane D, et al. Endothelium-Macrophage crosstalk mediates Blood-Brain barrier dysfunction in hypertension. Hypertension. 2020;76(3):795–807. doi:10.1161/HYPERTENSIONAHA.120.15581
- Wei J, Dai S, Pu C, et al. Protective role of TLR9-induced macrophage/microglia phagocytosis after experimental intracerebral hemorrhage in mice. CNS Neurosci Ther. 2022;28(11):1800–1813. doi:10.1111/cns.13919
- Arboix A, Massons J, García-Eroles L, Targa C, Comes E, Parra O. Clinical predictors of lacunar syndrome not due to lacunar infarction. BMC Neurol. 2010;10(1):31. doi:10.1186/1471-2377-10-31
- Arboix A, Garcia-Eroles L, Massons J, Oliveres M, Targa C. Hemorrhagic lacunar stroke. Cerebrovasc Dis. 2000;10(3):229–234. doi:10.1159/000016061