Abstract
Background
Heart failure (HF) is a global health challenge affecting millions, with significant variations in patient characteristics and outcomes based on ejection fraction. This study aimed to differentiate between HF with reduced ejection fraction (HFrEF) and HF with preserved ejection fraction (HFpEF) with respect to patient characteristics, risk factors, comorbidities, and clinical outcomes, incorporating advanced machine learning models for mortality prediction.
Methodology
The study included 1861 HF patients from 21 centers in Jordan, categorized into HFrEF (EF <40%) and HFpEF (EF ≥ 50%) groups. Data were collected from 2021 to 2023, and machine learning models were employed for mortality prediction.
Results
Among the participants, 29.7% had HFpEF and 70.3% HFrEF. Significant differences were noted in demographics and comorbidities, with a higher prevalence of males, younger age, smoking, and familial history of premature ASCVD in the HFrEF group. HFpEF patients were typically older, with higher rates of diabetes, hypertension, and obesity. Machine learning analysis, mainly using the Random Forest Classifier, demonstrated significant predictive capability for mortality with an accuracy of 0.9002 and an AUC of 0.7556. Other models, including Logistic Regression, SVM, and XGBoost, also showed promising results. Length of hospital stay, need for mechanical ventilation, and number of hospital admissions were the top predictors of mortality in our study.
Conclusion
The study underscores the heterogeneity in patient profiles between HFrEF and HFpEF. Integrating machine learning models offers valuable insights into mortality risk prediction in HF patients, highlighting the potential of advanced analytics in improving patient care and outcomes.
Introduction
Heart failure (HF) is a significant global health burden, affecting over 64 million people worldwide as of January 2023.Citation1,Citation2 This condition is progressive and can lead to severe morbidity and mortality, impacting various bodily systems, including the respiratory,Citation3 renal,Citation4 gastrointestinal (GI), and hepatic systems.Citation5 The etiology of heart failure is diverse, encompassing specific causes such as congenital heart diseases and cardiomyopathies, as well as multifactorial origins that accelerate cardiovascular aging.Citation6 Coronary artery disease is a prominent risk factor, influenced by a range of underlying conditions.Citation7 Ultimately, these etiologies disrupt structural, cellular, neurohormonal, and molecular functions essential for maintaining cardiac physiological homeostasis.Citation8 This disruption impairs blood flow through the vascular system, leading to clinical signs and symptoms of HF, including water overload and inadequate perfusion.Citation9
A critical measure in heart failure diagnosis is the Left Ventricular Ejection Fraction (LVEF), which assesses the systolic function of the left ventricle via two-dimensional echocardiography. Normal LVEF readings range from 53% to 75%, with values below 53% considered abnormal.Citation10,Citation11 LVEF is pivotal in differentiating heart failure types: heart failure with reduced ejection fraction (HFrEF) is characterized by an LVEF of less than 40%, heart failure with mid-range ejection fraction (HFmrEF) is characterized by an LVEF between 40% and 49%, and heart failure with preserved ejection fraction (HFpEF) is defined by an LVEF of 50% or more, accompanied by signs of cardiac dysfunction such as reduced LV filling, elevated filling pressures, and increased natriuretic peptide levels.Citation12 These heart failure types, although sharing many risk factors and comorbidities, differ in their responses to systemic inflammation and cardiac remodeling,Citation13 necessitating divergent management strategies. HFpEF predominantly affects older, female, obese patients and is associated with higher comorbidity rates. Despite current therapeutic options primarily alleviating symptoms, they have limited impact on halting disease progression or reducing mortality rates, although SGLT2 inhibitors have been shown to decrease heart failure hospitalizations.Citation14 This study aims to conduct a comprehensive analysis of factors influencing HFrEF, HFmrEF, and HFpEF, including patient characteristics, risk factors, and comorbidities, and their impact on morbidity and mortality outcomes. Additionally, the study seeks to utilize machine learning models to predict mortality in heart failure patients, thereby introducing an innovative dimension to understanding and managing this complex condition.
Methodology
Study Design, Setting, and Data Collection
The data for this study were sourced from the national heart failure registry of Jordan. The characteristics of the JoHF registry and our study protocol were registered at clinicaltrials.gov (NCT04829591). This study adhered to ethical guidelines and standards outlined in the Declaration of Helsinki. Ethical approval was obtained from the Research Committee of the Faculty of Medicine and the Institutional Review Board at the Specialty Hospital, with a waiver of patient consent due to the non-interventional nature of the study and the routine registration of outcomes in heart failure patients.The IRB approval number is (5/1/T/104826).Medical records of heart failure patients from 2020 to 2023 across 21 centers in Jordan were collected and analyzed. The primary inclusion criterion was a documented diagnosis of heart failure by echocardiography, regardless of whether the diagnosis was made in the emergency room for acute HF or in a clinical setting for chronic HF. Data collection encompassed patient characteristics, past medical history, drug and social history, and the diagnosis, presentation, and progression of heart failure. Data collection was conducted by a medically trained team using an online Google form.
Inclusion and Exclusion Criteria
Patients included in the study had a confirmed diagnosis of heart failure, evidenced by echocardiographic findings. The inclusion criteria focused on patients with an LVEF less than 40% (HFrEF), and those with an LVEF of 50% or more (HFpEF). Patients diagnosed with HFpEF met the criteria for left ventricular diastolic dysfunction, which included typical clinical symptoms of heart failure (eg, dyspnea, fatigue, and fluid retention), echocardiographic evidence of diastolic dysfunction (eg, abnormal left ventricular filling pressures), and elevated natriuretic peptide levels. Exclusion criteria included patients with incomplete echocardiographic data, those diagnosed with acute coronary syndromes within three months prior to the study, and patients with significant valvular heart disease requiring surgical intervention.
Outcomes of Interest
The study examined the impact of different heart failure types on overall patient outcomes. The primary outcomes included the need for mechanical ventilation during hospitalization, the number of hospital admissions within a year following the initial heart failure presentation, and mortality rates.
Follow-Up and Treatment Information
The study did not specify the length of follow-up for patients. Information on pharmacological and non-pharmacologic treatments administered to the patients, such as medications and lifestyle interventions, was not provided. This information is essential for understanding variations in patient management and their potential impact on outcomes.
Statistical Analysis
Data were entered into Microsoft Office Excel 2019 and analyzed using IBM SPSS v.25 software. Baseline characteristics, comorbidities, and clinical outcomes were compared using T-tests for continuous variables and Chi-square tests for categorical variables, with a p-value < 0.050 indicating statistical significance. Normality of data distribution was assessed using the Shapiro–Wilk test. For normally distributed variables, descriptive statistics were presented as mean and standard deviation, while median and interquartile range were used for non-normally distributed variables. Categorical variables were summarized as frequencies and percentages. To address missing data, multiple imputations were performed using the “mice” package in R, generating five imputed datasets through Predictive Mean Matching (PMM). Post-imputation, statistical analyses were conducted on each dataset, and results were pooled to provide final estimates, ensuring robust handling of missing data and enhancing the reliability of findings.
Machine Learning Analysis
A machine learning-based approach was employed to predict mortality. Numerical columns underwent median imputation for missing values, while categorical columns were imputed with the most frequent value. All numerical data were standardized using a StandardScaler to ensure feature comparability. Recursive Feature Elimination with Cross-Validation (RFECV) was applied using a RandomForestClassifier to identify the most relevant features. The dataset was split into training, validation, and test sets, with 40% allocated for the combined validation and test sets. To address class imbalance, the training set was resampled using the SMOTEENN technique, a combination of Synthetic Minority Over-sampling (SMOTE) and Edited Nearest Neighbors (ENN) algorithms.
A grid search with fivefold cross-validation was conducted to tune hyperparameters for the models. The study evaluated four machine learning models: Random Forest Classifier (RFC), Logistic Regression (LR), Support Vector Machine (SVM), and Extreme Gradient Boosting (XGBoost). The models’ effectiveness was assessed using accuracy, precision, recall, F1 score, and the Area Under the ROC Curve (AUC). SHapley Additive exPlanations (SHAP) values were employed to interpret the contribution of each feature to the models’ predictions, providing insights into the factors driving mortality risk predictions.
Results
Demographics, Baseline Characteristics, and Comorbidities
The study enrolled 1861 individuals diagnosed with heart failure (HF), comprising 553 with HF with preserved ejection fraction (HFpEF) and 1308 with HF with reduced ejection fraction (HFrEF). highlights the differences in demographics, baseline characteristics, and comorbidities between patients with HFpEF and those with HFrEF. There was a significant variation in gender distribution between the two groups (P-value = <0.001); males constituted 66.3% of the HFrEF group while only constituting 43.6% of the HFpEF group. Moreover, significant differences were noted across age groups (P-value = <0.001); patients aged 70 or above formed the majority of the HFpEF group (59.8%), while those formed only 39.5% of patients with HFrEF. Patients aged less than 40 accounted for 5.6% of the HFrEF group, compared to only 0.9% in the HFpEF group. Comorbidities, namely diabetes mellitus (DM), hypertension (HTN), and obesity were significantly more prevalent in the HFpEF group compared to the HFrEF group: 73.7% vs 67.0% (P-value = 0.006), 88.9% vs 77.0% (P-value = <0.001), and 13.0% vs 6.6% (P-value = <0.001), respectively. In contrast, smoking exhibited a higher prevalence in the HFrEF group, with a rate of 36.2% compared to 22.1% in the HFpEF group (P-value = 0.000). Notably, dyslipidemia rates showed no significant difference between both groups.Furthermore, patients with HFrEF were more likely to have a familial history of premature atherosclerotic cardiovascular disease (ASCVD) compared to patients with HFpEF (6.8% vs 3.8%, P-value = 0.016). On the other hand, a history of arrhythmia was more prevalent in patients with HFpEF compared to patients with HFrEF (37.3% vs 30.0%, P-value = 0.010). Rates of having ASCVD, implanted devices, and structural heart disease were not significantly different between both groups.
Table 1 Demographics, Baseline Characteristics, and Comorbidities
Clinical Outcomes
Assessment of the clinical outcomes revealed no statistically significant differences between patients with HFpEF and those with HFrEF across the studied variables. () The number of admissions or office visits for HF in the past 12 months was comparable for both groups. Additionally, rates of mechanical ventilation (4.6% vs 4.8%) and mortality (8.7% vs 9.0%) were nearly identical. The mean length of hospitalization was 6.5 days for HFpEF and 6 days for HFrEF.
Table 2 Clinical Outcomes
Prediction of Death Using Machine Learning Models
We evaluated the performance of various machine learning models to predict mortality in patients with Heart Failure. The Random Forest Classifier (RFC) achieved an accuracy of 0.9002, with a precision of 0.50, a recall of 0.28, an F1 Score of 0.36, and an AUC of 0.7556. Logistic Regression showed an accuracy of 0.7332, a precision of 0.21, a recall of 0.63, an F1 Score of 0.32, and an AUC of 0.7722. The Support Vector Machine (SVM) model resulted in an accuracy of 0.7935, a precision of 0.23, recall of 0.44, an F1 Score of 0.30, and an AUC of 0.7375. Lastly, the XGBoost model demonstrated an accuracy of 0.8979, with a precision of 0.48, a recall of 0.37, an F1 Score of 0.42, and an AUC of 0.7542. ROC curves for all models are shown in .
Model Interpretation and Feature Importance
The SHAP plot provides an intuitive understanding of the key features influencing the model’s predictions, as seen in . It indicates that a longer Length of Hospital Stay, the requirement for Mechanical Ventilation, and a higher Number of Hospital Admissions for HF in the Past 6 Months are associated with a higher probability of the adverse outcome predicted for patients with heart failure (HF). These features, along with Age ≥70 and Dyslipidemia, stand out as the top risk factors according to the model’s calculations. In contrast, Alcohol Consumption is shown to have the least influence on the predictions, with its SHAP values clustered around zero, indicating a neutral impact on the outcome. Additionally, the presence of chronic kidney disease, Diabetes Mellitus, and Atrial Fibrillation are observed to moderately influence the model’s output, suggesting that these conditions contribute to the risk assessment in HF patients, albeit to a lesser extent than the top factors. The SHAP values also reveal that Sex, Smoking, and History of Implanted Devices have varied impacts on the model’s predictions, with some instances increasing the likelihood of the adverse outcome and others decreasing it. This variability underscores the complex interplay of individual patient characteristics in determining health outcomes.
Figure 2 SHAP Summary Plot of Predictive Factors for Mortality in HFrEF and HFpEF Patients Using Machine Learning-Based Death Prediction Models.The features are listed on the y-axis in order of importance. The top feature has the most significant impact on the model’s predictions. The x-axis represents the SHAP value. A positive SHAP value indicates that the feature increases the likelihood of the predicted outcome (in this case, mortality), while a negative SHAP value suggests a decrease in the likelihood. Each dot in the plot represents a SHAP value for an individual patient. The color represents the feature value (eg, red for high values and blue for low values).
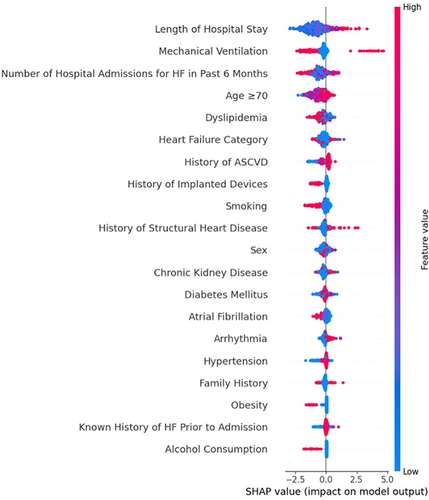
Discussion
Heart failure (HF) represents a growing health problem, with ejection fraction (EF) serving as a universal discriminator that classifies it into two main categories: HFpEF (Heart Failure with preserved Ejection Fraction) and HFrEF (Heart Failure with reduced Ejection Fraction). The primary objective of this study was to explore and compare the distinct characteristics and clinical outcomes of individuals falling into the HFpEF and HFrEF categories. Out of the total 1861 individuals diagnosed with heart failure, 29.7% (553 individuals) were identified with HFpEF (EF ≥ 50%), and 70.3% (1308 individuals) with HFrEF (EF <40%). The study revealed substantial differences in both gender distribution and age groups between HFrEF and HFpEF. Males constituted 66.3% ofHFrEF compared to 43.6% in HFpEF; notably, our study found an association between ischemic causes and male gender, reinforcing the predominance of males in the HFrEF group. Furthermore, male gender is considered a significant predictor among the various factors associated with heart failure readmissions.Citation1 The higher proportion of females in HFpEF further supports the recognition of the female gender as a predictor for this phenotype.Citation2 Moreover, our analysis revealed significant age differences. Patients aged 70 or above were notably prevalent in the HFpEF group. Therefore, both female gender and older age are consistently associated with HFpEF, as observed in multiple registries, including Saudi (HEARTS), Egyptian, Framingham, JCARE-CARD, and GWTG-HF.Citation1–8 Comorbidities such as hypertension (HTN), atrial fibrillation/flutter, and obesity exhibited a significantly higher prevalence in the HFpEF group compared to the HFrEF group in our study. Conversely, smoking was more prevalent in the HFrEF group. These findings align with the Results presented by Shah et al.Citation5 In contrast to our study, Shah et al’s results indicated that patients with HFrEF more frequently presented with a history of dyslipidemia, coronary artery disease, and prior myocardial infarction.Citation5,Citation15,Citation16 However, our study revealed no significant difference in dyslipidemia or a history of atherosclerotic cardiovascular disease (ASCVD) rates between the HFpEF and HFrEF groups. Furthermore, a higher prevalence of diabetes mellitus (DM) was observed in the HFpEF group in our study. Considering the high association of comorbidities with HFpEF, addressing these comorbidities when indicated becomes essential.Indeed, further research is crucial to establish comprehensive guidelines that address the management and treatment of HFpEF.
The clinical outcomes assessment revealed no statistically significant differences between patients with HFpEF and those with HFrEF across the studied variables in our study. The number of admissions or office visits for heart failure (HF) was comparable for both groups. Additionally, mechanical ventilation and mortality rates were nearly identical, with the mean length of hospitalization being 6.5 days for HFpEF and 6 days for HFrEF.These findings align with results from multiple other studies, including Shah et al’s 5-year outcomes study based on the GWTG registry. In their study, risk-adjusted analyses demonstrated similar outcomes, indicating that mortality and rehospitalization were comparable for both HFrEF and HFpEF.Citation5 These findings are consistent with results from the JCARE-CARD study, which indicated that HF patients with preserved ejection fraction (EF) had a mortality risk and re-hospitalization rates comparable to those with reduced EF.Citation7 Interestingly, other studies have shown that mortality is lowest in patients with ejection fractions greater than or equal to 50%.Citation8 Moreover, findings from another study revealed that among patients hospitalized with HF, those with HFpEF had slightly lower mortality and higher all-cause readmission risk than patients with HFrEF. However, the mortality differences did not persist after risk adjustment.Citation6 Nevertheless, this does not undermine the fact that heart failure (HF), irrespective of ejection fraction (EF), significantly affects mortality, hospitalization, and admissions, making it a growing public health problem.Citation15–21 Evidence suggests that among patients hospitalized with HF, individuals across the EF spectrum share a similarly poor survival. The study by Shah et al revealed that all patients, regardless of EF, experienced a remarkably high mortality rate at 5 years from the index.Citation5 Other studies have indicated that patients with HFpEF are associated with a higher risk of all‐cause mortality.
In contrast, patients with HFrEF were found to be related to a higher risk of cardiovascular mortality.Citation9 In our study, the machine learning (ML) models, particularly the Random Forest Classifier, demonstrated significant predictive capability for mortality among heart failure (HF) patients. This aligns with Shin et al,Citation22 who emphasized the potential of ML over conventional statistical models in predicting heart failure outcomes. Notably, our analysis identified key predictors such as length of hospital stay, need for mechanical ventilation, and number of hospital admissions, which are crucial in assessing patient risk. This is in line with the findings of Álvarez-García et al,Citation23 who highlighted the significance of hospital stay length and mechanical ventilation as predictors of mortality. This study has several limitations. The retrospective nature of the registry data may introduce selection and information biases. Detailed analyses of the causes leading to mechanical ventilation and management of comorbidities were not performed, which could affect the interpretation of these outcomes. Additionally, the national registry may not capture all variations in practice patterns and patient populations. Moreover, we did not evaluate the prognostic role of the Charlson comorbidity index (CCI) and the CHA2DS2-Vasc score. Both indices are important for assessing the burden of comorbidity and thromboembolic risk in heart failure patients. Recent studies have demonstrated their prognostic significance in heart failure.Citation21,Citation24–26 Including these indices could provide additional insights and improve the prognostic evaluation of our patient cohort. This is an area for future research where we plan to incorporate these indices to enhance our understanding of heart failure outcomes.Additionally, our study did not include heart failure patients with mildly reduced ejection fraction (HFmrEF), defined as those with LVEF between 41% and 49%. HFmrEF represents a significant subgroup that may occur either as a recovery from HFrEF or a deterioration from HFpEF.Citation27 Future analyses should consider including HFmrEF patients to provide a more comprehensive understanding of heart failure phenotypes.Future research should focus on prospective studies to validate these findings and incorporate more detailed analyses of comorbidities and their management. Further exploration into the specific causes of critical outcomes like mechanical ventilation could enhance our understanding and improve patient care.
Conclusion
These findings emphasize the urgent need for comprehensive strategies to address heart failure and promptly treat HF, considering both preserved and reduced EF, to improve outcomes and enhance the overall well-being of individuals affected by HF. Our machine learning models provided a good tool for the identification of high-risk patients. However, the precision and recall of our models were not the highest due to class-imbalanced data. Future studies in the Middle East should implement such models on more extensive and more balanced datasets.
Disclosure
The authors report no conflicts of interest in this work.
References
- Savarese G, Becher PM, Lund LH, Seferovic P, Rosano GMC, Coats AJS. Global burden of heart failure: a comprehensive and updated review of epidemiology. Cardiovasr Res. 2023;118(17):3272–3287. doi:10.1093/cvr/cvac013
- Mosterd A, Hoes AW. Clinical epidemiology of heart failure. Heart. 2007;93(9):1137–1146. doi:10.1136/hrt.2003.025270
- Cross TJ, Kim CH, Johnson BD, Lalande S. The interactions between respiratory and cardiovascular systems in systolic heart failure. J Applied Physiol. 2020;128(1):214–224. doi:10.1152/japplphysiol.00113.2019
- Schefold JC, Filippatos G, Hasenfuss G, Anker SD, von Haehling S. Heart failure and kidney dysfunction: epidemiology, mechanisms and management. Nat Rev Nephrol. 2016;12(10):610–623. doi:10.1038/nrneph.2016.113
- Correale M, Tarantino N, Petrucci R, et al. Liver disease and heart failure: Back and forth. Eur J Internal Med. 2018;48:25–34. doi:10.1016/j.ejim.2017.10.016
- Triposkiadis F, Xanthopoulos A, Parissis J, Butler J, Farmakis D. Pathogenesis of chronic heart failure: cardiovascular aging, risk factors, comorbidities, and disease modifiers. Heart Failure Rev. 2022;27(1):337–344. doi:10.1007/s10741-020-09987-z
- Lala A, Desai AS. The role of coronary artery disease in heart failure. Heart Fail Clinics. 2014;10(2):353–365. doi:10.1016/j.hfc.2013.10.002
- Tanai E, Frantz S. Pathophysiology of Heart Failure. Compr Physiol. 2015;6:187–214.
- Jain CC, Borlaug BA. Hemodynamic assessment in heart failure. Catheterization and cardiovascular interventions. Off j Soc Cardiac Angiograph Interven. 2020;95(3):420–428. doi:10.1002/ccd.28490
- Kosaraju A, Goyal A, Grigorova Y, Makaryus AN. Left Ventricular Ejection Fraction. In StatPearls. StatPearls Publishing; 2023.
- King M, Kingery J, Casey B. Diagnosis and evaluation of heart failure. Am Family Phys. 2012;85(12):1161–1168.
- Heidenreich PA, Bozkurt B, Aguilar D, et al.; Writing Committee Members (2022). 2022 American college of cardiology/American heart association/heart failure society of America guideline for the management of heart failure: Executive summary. J Card Fail. 2022;28(5):810–830. doi:10.1016/j.cardfail.2022.02.009
- Simmonds SJ, Cuijpers I, Heymans S, Jones EAV. Cellular and Molecular Differences between HFpEF and HFrEF: A step ahead in an improved pathological understanding. Cells. 2020;9(1):242. doi:10.3390/cells9010242
- Gard E, Nanayakkara S, Kaye D, Gibbs H. Management of heart failure with preserved ejection fraction. Austr Prescr. 2020;43(1):12–17. doi:10.18773/austprescr.2020.006
- Lee DS, Gona P, Vasan RS, et al. Relation of disease pathogenesis and risk factors to heart failure with preserved or reduced ejection fraction: insights from the Framingham heart study of the national heart, lung, and blood institute. Circulation. 2009;119(24):3070–3077. doi:10.1161/CIRCULATIONAHA.108.815944
- Shah KS, Xu H, Matsouaka RA, et al. Heart failure with preserved, borderline, and reduced ejection fraction: 5-year outcomes. J American Col of Card. 2017;70(20):2476–2486. doi:10.1016/j.jacc.2017.08.074
- AlHabib KF, Elasfar AA, AlBackr H, et al. Design and preliminary results of the heart function assessment registry trial in Saudi Arabia (HEARTS) in patients with acute and chronic heart failure. European j Heart Failure. 2011;13(11):1178–1184. doi:10.1093/eurjhf/hfr111
- Hassanein M, Abdelhamid M, Ibrahim B, et al. Clinical characteristics and management of hospitalized and ambulatory patients with heart failure-results from ESC heart failure long-term registry-Egyptian cohort. ESC Heart Failure. 2015;2(3):159–167. doi:10.1002/ehf2.12046
- Tsuchihashi-Makaya M, Hamaguchi S, Kinugawa S, et al.; JCARE-CARD Investigators (2009). Characteristics and outcomes of hospitalized patients with heart failure and reduced vs preserved ejection fraction. Report from the Japanese Cardiac Registry of Heart Failure in Cardiology (JCARE-CARD). Circu Offil j Japanese Circu Soc. 2009;73;10:1893–1900. doi:10.1253/circj.cj-09-0254
- Cheng RK, Cox M, Neely ML, et al. Outcomes in patients with heart failure with preserved, borderline, and reduced ejection fraction in the Medicare population. Am Heart J. 2014;168(5):721–730. doi:10.1016/j.ahj.2014.07.008
- Philbin EF, Rocco TA Jr, Lindenmuth NW, Ulrich K, Jenkins PL. Systolic versus diastolic heart failure in community practice: clinical features, outcomes, and the use of angiotensin-converting enzyme inhibitors. Am J Med. 2000;109(8):605–613. doi:10.1016/s0002-9343(00)00601-x
- Shin S, Austin PC, Ross HJ, et al. Machine learning vs. conventional statistical models for predicting heart failure readmission and mortality. ESC Heart Fail. 2021;8(1):106–115. doi:10.1002/ehf2.13073
- Roedl K, Jarczak D, Thasler L, et al. Mechanical ventilation and mortality among 223 critically ill patients with coronavirus disease 2019: a multicentric study in Germany. Aust Crit Care. 2021;34(2):167–175. doi:10.1016/j.aucc.2020.10.009
- Pernenkil R, Vinson JM, Shah AS, Beckham V, Wittenberg C, Rich MW. Course and prognosis in patients > or = 70 years of age with congestive heart failure and normal versus abnormal left ventricular ejection fraction. Am J Cardiol. 1997;79(2):216–219. doi:10.1016/s0002-9149(96)00719-9
- Liang M, Bian B, Yang Q. Characteristics and long-term prognosis of patients with reduced, mid-range, and preserved ejection fraction: a systemic review and meta-analysis. Clin Cardiol. 2022;45(1):5–17. doi:10.1002/clc.23754
- Wei D, Sun Y, Chen R, Meng Y, Wu W. The Charlson comorbidity index and short-term readmission in patients with heart failure: a retrospective cohort study. Medicine. 2023;102(6):e32953. doi:10.1097/MD.0000000000032953
- Zhou Q, Li P, Zhao H, et al. Heart failure with mid-range ejection fraction: a distinctive subtype or a transitional stage? Front Cardiovasc Med. 2021;8:678121. doi:10.3389/fcvm.2021.678121