Abstract
Background
There is a need to take a broader look at nanotoxicological studies. Eventually, the field will demand that some generalizations be made. To begin to address this issue, we posed a question: are metal colloids on the nanometer-size scale a homogeneous group? In general, most people can agree that the physicochemical properties of nanomaterials can be linked and related to their induced toxicological responses.
Methods
The focus of this study was to determine how a set of selected physicochemical properties of five specific metal-based colloidal materials on the nanometer-size scale – silver, copper, nickel, iron, and zinc – could be used as nanodescriptors that facilitate the grouping of these metal-based colloids.
Results
The example of the framework pipeline processing provided in this paper shows the utility of specific statistical and pattern recognition techniques in grouping nanoparticles based on experimental data about their physicochemical properties. Interestingly, the results of the analyses suggest that a seemingly homogeneous group of nanoparticles could be separated into sub-groups depending on interdependencies observed in their nanodescriptors.
Conclusion
These particles represent an important category of nanomaterials that are currently mass produced. Each has been reputed to induce toxicological and/or cytotoxicological effects. Here, we propose an experimental methodology coupled with mathematical and statistical modeling that can serve as a prototype for a rigorous framework that aids in the ability to group nanomaterials together and to facilitate the subsequent analysis of trends in data based on quantitative modeling of nanoparticle-specific structure–activity relationships. The computational part of the proposed framework is rather general and can be applied to other groups of nanomaterials as well.
Introduction
Materials designed on the nanometer-size scale are becoming increasingly relevant in many areas of physical and life sciences, engineering applications, and advanced technology. Because of the popularity of nanomaterials, an adequate methodology for evaluating the potential impact of engineered nanomaterials on environmental and human health and safety is now a necessity. Published data in the recent literature point to an increase of various biological responses, such as inflammatory or immune responses, after exposure to nanomaterials.Citation1–Citation7 These biological responses have been observed and reported on from either in vitro (cell culture-based) or in vivo (whole-animal) model systems; however, more research should be conducted on relating the physicochemical features of each engineered nanomaterial to the observed biological responses. It is critical to develop computational models that not only establish quantitative relationships between different types of experimental data but can also reliably predict biological responses and/or hazards to nanomaterials. We aim to provide a useful framework for such decision analyses. This effort requires a large and organized effort within the nanomaterial community. Many data sets measuring multiple endpoints are required in order to accurately predict biological responses. While this paper does not model experiments with a biological endpoint, it does propose a framework readily applicable to studies where such variables are measured (see ).
Figure 1 The proposed data collection and processing framework.
Abbreviation: QSARs, quantitative structure-activity relationships.
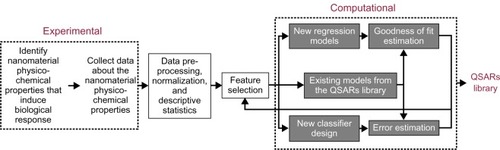
Some of the most interesting and relevant engineered nanomaterials are metal colloids. These materials – including but not limited to gold, silver, iron, and zinc – are often hydrophobic complex crystals, have a valence of zero, are highly charged on their surface, and are synthesized to be very small. Most of the particles used in industry are less than 25 nm in diameter. Because these particular features are common among metal colloids, these particle types have the potential to be “grouped;” thus, the structure–activity relationships (SARs) methodology is a promising approach in designing predictive mathematical models for these nanomaterials.
The concept of quantitatively analyzing the SARs of hydrophobic molecules was originally introduced by Hansch in 1969 and subsequently used in a variety of studies.Citation8–Citation13 This methodology is based on the assumption that the variance in biological response to a class of chemical compounds is determined by the variance in their molecular structure. In terms of prediction, this means that if data for some molecular parameters (known as molecular descriptors) for a group of compounds are available only for a part of that group, then there is the possibility to estimate the unknown data from the available molecular descriptors by using a suitable mathematical model.Citation14,Citation15 SARs have shown their utility in cases of “classic” chemicals or compounds.Citation14–Citation18 However, the direct application of the methodology to nanometer-scale materials has been problematic, as discussed by Xia et al, Fourches et al, Zhang et al, Puzyn et al, and Sayes and Ivanov.Citation19–Citation23 The first important step in developing sound quantitative SAR models for predicting the effects of a nanoparticle on a biological system and the environment is to identify measurable physicochemical characteristics of nanoparticles – ie, nanodescriptors. But this is not the only step that is critical to the success of quantitative SAR models for nanomaterials. There is also a need for a conceptual framework for grouping nanomaterials, based on unique material features or properties. Such a framework will help to identify SARs that are applicable within each group of nanomaterials. The currently available data from the published literature and other open sources suggest that there is a high variability in the morphological structure, chemical reactivity, and mechanisms of action among different nanoparticles. Thus, the applicability domain of the SARs should be carefully validated.Citation19–Citation25
In the present study, we investigated how techniques borrowed from statistics and pattern recognition can be used as parts of a general framework that can help to group engineered nanomaterials on the basis of their measurable physicochemical properties. As an example of the proposed framework, we use principle component analysis (PCA) and linear discriminant analysis (LDA) to address the important question of how one can group the specific collection of silver, copper, nickel, iron, and zinc. PCA and LDA are well-known in the fields of statistics and pattern recognition.Citation26–Citation29 These techniques have been considered tools for designing quantitative SARs.Citation30 The overarching goal of this study was to determine if the collection of particles under consideration could be separated into groups using their physicochemical features. The example discussed in this manuscript details some of the important components of the general framework: feature identification, feature extraction/selection, data preprocessing and normalization, descriptive data statistics, unsupervised/supervised learning of data structure, and grouping predictions (see ). This framework can be readily applied not just to the five particles considered in this paper, but also when other nanomaterials (such as metal oxides or carbon-based nanostructures) or endpoints (such as biological/toxicological effects, particle transformations, or eventual fates) are considered.
Materials and methods
Experimental design
The experiments used in the study examined six different physicochemical characteristics of five metal colloids suspended in an aqueous suspension. The characteristics of the particles included engineered size, concentration, agglomerated size in water, zeta potential as a measure of surface charge, pH and age of the suspension, and oxidant production. Data were collected in the absence of cells, serum, and media in order to avoid the added variables created by living organisms (eg, stickiness due to serum or bacterial growth in media), but was replicated 20 times per particle type. Each nanoparticle measurement was collected in two different prepared concentrations (10 and 100 mg/L) and included engineered size (reported in nanometers, nm), zeta potential (reported in millivolts, mV), pH, and reactive oxygen species (ROS) production (measured in relative fluorescence units [RFUs]). Each of these measured nanodescriptors was assessed on the day the samples were taken (t = 0 d), 2 days later (t = 2 d), and 1 week later (t = 7 d). The manufacturer’s reported size was also included in the analyses. Each data set was analyzed using three methods: PCA, correlation analysis (CA), and LDA. The framework for this experimental design is depicted in .
The nanodescriptors, or physicochemical characteristics, selected in this study are relevant features that have been shown to change over time in physiologically relevant conditions. Particle size, pH, ROS concentration, and zeta potential all change when ions dissociate from the nanoparticle surface over time. In addition to the relevance of each of these measured nanodescriptors, they are the most common and routinely reported physicochemical variables in the literature.
Nanoparticle preparation and characterization
Silver, copper, nickel, and zinc were supplied by Sigma-Aldrich (St Louis, MO, USA). Iron was supplied by Sun Innovations Inc., Fremont, CA, USA. A stock suspension of 1000 mg/L was prepared and then diluted to 10 and 100 mg/L to ensure consistency between the suspensions. Each suspension was bath-sonicated for approximately 1 minute, until the particles were visually dispersed (). Sonicating for extended periods of time would initially disperse the particles and then allow for most of the particles to settle out of suspension. The size in water and zeta potential was measured using dynamic light scattering on a ZetaSizer Nano-ZS instrument (Malvern Instruments, Malvern, UK). The instrument uses Brownian motion to measure the particle size and a combination of voltage difference and Brownian motion to measure the zeta potential. The pH was measured using an accumet Basic AB15 pH meter (Thermo Fisher Scientific, Waltham, MA, USA).
Figure 2 Photograph of the nanoparticle samples used in the study.
Notes: The nanoparticles were suspended in either ultrapure water or Dulbecco’s Modified Eagle’s Medium. The concentration of the particles in the aqueous suspension was 100 mg/L. The photograph was taken immediately after sonication.
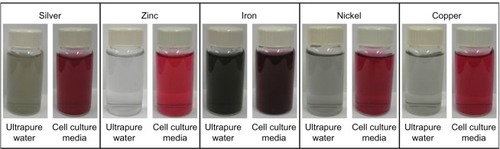
Reactive oxygen species production of the particles in suspension was measured using the fluorescent probe 2′,7′-dichlorfluorescein-diacetate (DCFH) (). The relative amount of reactive oxygen species produced is represented by fluorescence intensity measured on a BioTek Synergy MX (Winooski, VT, USA). Briefly, 300 μL of DCFH was mixed with 200 μL of the nanoparticle suspension. The mixture was stored in the dark in a rotator for 3 hours. Three-hour storage time was chosen to account for the variations between the metals. Mixing times longer than 3 hours interfered with results because particles produced a fluorescence intensity that saturated the spectrophotometer. At the end of the incubation period, the mixture was centrifuged and analyzed.
Figure 3 The surface chemistry properties of the engineered nanoparticles. (A) The autofluorescence of each engineered nanoparticle in ultrapure water over 24 hours. Particle suspensions were sonicated for 1 minute before each fluorescence measurement. (B) The reactive oxygen species production, as measured by 2′,7′-dichlorfluorescein-diacetate fluorescence, of each engineered nanoparticle over 7 days.
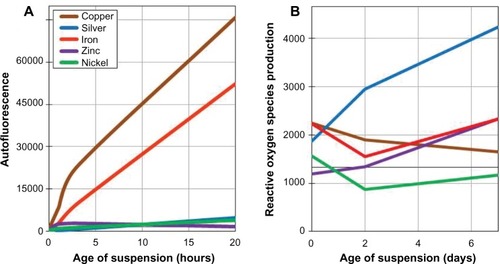
Statistical analysis and predictive modeling
Because one of the main outcomes of the proposed framework was to determine how the measured nanoparticles features could lead to meaningful groupings, the first consideration was to examine if the measured nanoparticle characteristics had comparable scales. The boxplots in show that the different data types in the raw data had markedly different value ranges. That observation suggested that data standardization was an appropriate transformation, which was achieved by dividing each raw data value by the standard deviation in its respective data type. The next step in the data flow process as described by the proposed framework was to determine if the variation present in the data was heavily weighted in any given direction. To this end, we applied PCA. This specific technique is often used to reduce the original data dimensionality. However, our particular data set has only six dimensions or measurements; thus, the application of PCA did not aim to reduce data dimensionality. A check for existing correlations between different data types followed the PCA step, and all of the pair-wise correlations were computed. This CA aimed to determine if the different data types exhibited pair-wise linear dependencies. Finally, the results from the PCA and CA helped to hypothesize possible groupings of the five nanomaterials. To test the strength of the separation between those groupings, we used LDA classification. The LDA classification results can be viewed as the output of the data flow processing under the proposed framework, and they address the important question of possible subgroupings in the original group of metal colloids based on combinations of their measured characteristics or features. The separation strength of the tested sub-groupings was measured by the estimated bolstered re-substitution error, which was an appropriate error estimation method given the relatively small number of data points.Citation25 This particular error estimation method implies that the entire data set was used for the classifier training and testing.
Results
Data standardization and principal component analysis
The first step in the proposed data-flow processing is to examine the raw data descriptive statistics, and to determine if data transformations are needed before proceeding with the subsequent processing steps. shows that the measured nanomaterial properties have very different value ranges, and thus standardization is necessary to represent the variation in different types of data on a scale that facilitates subsequent analyses. Boxplots are useful in visualizing variation as part of the proposed framework, and the rationale for standardization is to scale the variables to a common factor such that the variations of each variable are scale-wise comparable. For example, in raw data for silver, the pH appears to have little variation, while the concentration varies widely. After standardization, it is clear that pH does vary significantly. Concentration, by design, varies much less than what the raw data boxplots suggest. The measurements for the agglomerated size in water show the greatest variation in the case of copper, iron, nickel, and zinc. After standardization, however, that characteristic has less variation when compared to the others.
Another important question that needs to be addressed by the proposed framework is how to determine the structure of the variability present in data. PCA is often used for this purpose. Its objective is to determine which variables account for the greatest variability in the data set, as well as the directions of greatest variability in data. In the raw and standardized data, each metal is described by six variables or features. PCA determines which of these contribute most significantly to the observed data variability. The Pareto plots in show that 90% of the variation for each individual nanoparticle can only be explained with a minimum of three principal components. For example, to explain 90% of the variability in iron and zinc, four principal components are required, while in copper and nickel data, the same percentage of variability can be explained by the first three principal components. Interestingly, when all of the nanoparticles are considered together, there is no clear direction in the variability of data, as shown in . Five out of six principal components are required to explain 90% of the observed variability in the entire data set. These findings indicate that further analysis is necessary to determine the significance of the measured nanomaterial characteristics in order to answer the question of possible subgroupings of the studied nanomaterials.
Figure 5 Principal component analyses Pareto plots. (A) All metal colloids combined in a single data set. (B) Silver data only. (C) Copper data only. (D) Iron data only. (E) Nickel data only. (F) Zinc data only.
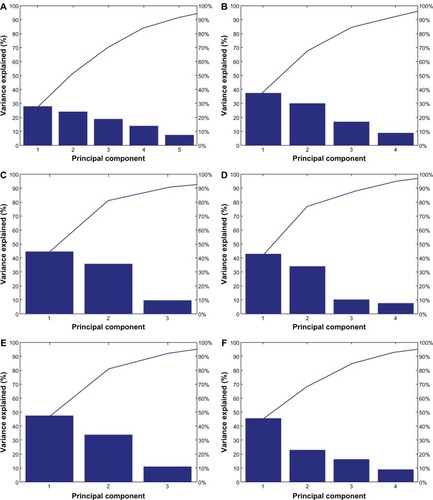
shows the principal components and the squares of their respective loadings for each individual metal. The principal components are ordered according to the percentage of the variation in data they explain, with the convention that the left-most listed principle component explains the largest portion of the observed variation. Each column provides the squares of the loadings for the respective principal component. The loadings indicate how important the contribution of the corresponding original measurement is in the respective principal component. One can see that the first principal component is often composed mostly of three to four original variables.
Table 1 Heat maps representing the relative weight of the original features in the respective principle components
Correlation analysis
An important step of the proposed analysis pipeline is to determine if any of the measurable nanomaterial features are redundant in terms of their detectable linear interdependencies. shows the corresponding pair-wise correlations. The results suggest several possible sub-groupings. Copper and nickel stand out because their respective features have multiple relatively high correlations in common. Specifically, the correlations between (1) agglomerated size in water and concentration, (2) zeta potential and concentration, and (3) zeta potential and agglomerated size in water show high values for one or both of these metals. Thus, one can hypothesize a natural pairing of copper and nickel, which separates them from the other metals. Furthermore, iron stands out because there are several strong correlations that the other metals do not have, such as (1) concentration and oxidant species production and (2) agglomerated size in water and oxidant species production. At the other end of the spectrum, iron is the only metal that had almost no correlation between oxidant species production and age. Thus, we hypothesize that iron naturally separates from the other metals.
Classification analysis
The last step of the proposed computational framework is to determine the strength of the separability between subgroupings suggested by the previous steps of data processing. It is important to emphasize that our goal is not to interpret the results in terms of mechanisms, but instead to propose a framework for grouping nanomaterials based on their characteristics. These groupings may then aid the future quantitative modeling of particles’ SARs (eg, regression models) and potentially help in interpreting biological/toxicological mechanistic-type studies. Given that the multiple stakeholders – such as regulatory agencies and nanomaterial companies – need streamlined approaches to assessing the uncertainties surrounding new materials in research and development activities, the interpretable and reliable grouping of nanomaterials becomes increasingly important activity in this field. Additional studies that include biological endpoints are needed to determine the biological meaning of the resultant grouping found in the discussion below.
Initially, the LDA classification was performed for all of the possible groupings of the metal nanoparticles into two groups. Notably, for each possible separation of the five nanomaterials into two groups, we found that the misclassification error was high. We also observed that, when zinc is present, data appeared ubiquitous and there was no clear grouping. When data points from zinc nanoparticles were excluded, the classification error decreased. That finding prompted a closer look at some of the known facts about zinc. Experimentally, zinc yields a highly soluble and highly cytotoxic material in cell cultures. The correlation analysis results also suggested that zinc stands out from the rest of the metal colloids. Thus, we hypothesized that the difficulty in finding separate groups when zinc is present could be due to zinc ions dissociating from the surface of the zinc particles. Future investigation is thus warranted.
Classification is a general pattern recognition technique that attempts to identify combinations of features that determine a decision boundary that separates two states of nature with the smallest possible misclassification error. We elected to use one of the simplest and least-complex classification rules, LDA, because of the potential for over-fitting phenomena. As suggested by the correlation analysis, we focused on two potential groupings for the nanomaterials. The first grouping considers silver, copper, and nickel together and iron as a separate group. The second grouping considers the group composed of iron and silver versus the group composed of copper and nickel. illustrates the first grouping and shows the best possible – in terms of the respective LDA misclassification error – plane that separates Group 1 (which contains only iron) from Group 2 (which contains silver, copper, and nickel). The three features providing the separation of the two groups and position of the separating plane are the
Figure 7 Linear discriminant analysis for iron versus the group of silver, copper, and nickel. (A) The features of concentration, zeta potential, and agglomerated size in water provide the best separation for the two groups when the entire data set is considered. (B) Zeta potential, agglomerated size in water, and age provide the best separation when only data for high concentrations (100 mg/L) are considered. (C) Zeta potential, agglomerated size in water, and age provide the best separation when only data for low concentrations (10 mg/L) are considered.
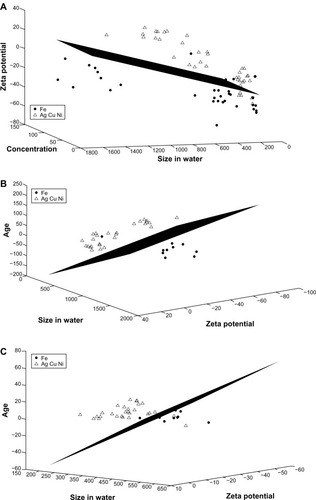
concentration,
zeta potential, and
agglomerated size in water.
It is important to notice that, when single-feature classification is considered, the concentration performs poorly (next to the worst single-feature classifier), with an estimated error rate greater than 30%. The agglomerated size in water and the zeta potential are the two top-performing single features. However, even the error rates of the respective classifiers, based on each one of them, are relatively high (>18%). Interestingly, combining these three features together exhibits a synergistic effect that lowered the misclassification error to 12.47%.
illustrates the application of the LDA classification for the second case of groupings. Group 1 included iron and silver, whereas group 2 included copper and nickel. In this particular analysis, the three features with the greatest ability to discriminate between the two groups are
Figure 8 LDA for the copper-and-nickel group versus the silver-and-iron group. Zinc is excluded from the analyses. (A) The features of concentration, zeta potential, and ROS production provide the best separation for the two groups when the entire data set is considered. (B) Zeta potential, pH, and ROS production provide the best separation when only data for high concentrations (100 mg/L) are considered. (C) Zeta potential, agglomerated size in water, and ROS production provide the best separation when only data for low concentrations (10 mg/L) is considered.
Abbreviations: LDA, linear discriminant analysis; ROS, reactive oxygen species.
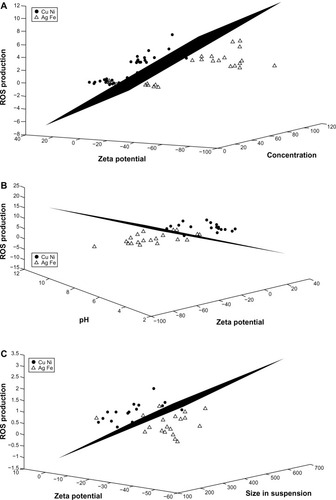
concentration,
zeta potential, and
ROS production.
However, the misclassification error rate is high (19.99%), which shows that this particular grouping of the four metal colloids is not as strong as when iron is considered against the other three metals.
Because of the importance of concentrations in toxicological studies, a separate LDA was performed separately for the above-mentioned two potential groupings of metal colloids for the cases of low (10 mg/L) and high (100 mg/L) concentrations. That separate analysis shows that, at both concentrations, the three variables with the greatest separating power for the potential grouping of iron versus copper, silver, and nickel are
zeta potential,
agglomerated size in water, and
age.
At 100 mg/L, the misclassification error rate drops to 10.16%. Interestingly, at the lower concentration of 10 mg/L, the error rate increases to 19.3%. illustrates the separation in the case of high concentrations for that grouping, while shows the separation for the low-concentration case. Similarly, show the separation when high and low concentrations are considered separately for the other potential: Group 1 composed of copper and nickel, versus Group 2 composed of silver and iron. At 100 mg/L, the combination of three variables that provides the best separation is
pH,
zeta potential, and
ROS production,
and the misclassification error rate is 13.24%, which is a significant decrease from the case when the data from the two concentrations were considered together. Similar to the case when iron was considered separately from the group of silver, copper, and nickel, the LDA shows poor separation for the case of a low concentration of 10 mg/L. The three variables with the greatest separating power for the low-concentration case are size in water, zeta potential, and ROS production. However, the misclassification error rate is very high, at 48.28%, which confirms the poor separation of the two groups at low concentrations.
Discussion
We aimed to develop a computational framework, utilizing the mathematical and statistical techniques previously described, that will assist researchers and regulators in evaluating potential responses of a specific biological system to a nanomaterial exposure. There is an urgent need for new tools, standardized practices, meaningful interpretation, and an iterative framework to help address the challenges presented by nanomaterials. Tools that could arm regulators and other decision-makers would have a great effect on the commercialization of nanomaterial-enabled goods. Nanomaterials do not fit into established models of material behavior because of their complexity. It is important to identify measurable physicochemical characteristics of nanoparticles for hazard identification, exposure analysis, risk assessment, and regulatory and policy decisions. Many different sectors of the community are waiting for either experimental or computational methodologies that relate nanomaterial physicochemical properties to induced toxicological responses. The trick is that this type of methodology will likely need both experimental and computational components working together in an iterative process. The result, ideally, would be a framework that would produce meaningful groupings of particles in an effort to generalize some of the common features and resultant common responses that so many particles on the nanometer-size scale seem to induce. For example, particles less than 10 nm in diameter not only have different optical properties, but also have different translocation tendencies in cellular systems. Metal-based nanoparticles (metal colloids) tend to leach metal ions at different rates and at different pH levels. In general, crystalline materials produce more oxidant species than amorphous materials. These types of observations can be made when considering the literature as a whole. Of course, there are always exceptions to the generalization.
Conclusion
The focus of this study was to identify the properties of five specific metal-based colloidal materials on the nanometer-size scale – silver, copper, nickel, iron, and zinc – that could change over time or in differing aqueous suspensions, and that can be used in a general framework for the grouping of nanomaterials.Citation23 The metal-based colloidal materials considered in this paper have received much attention from federal and international regulatory agencies. Metal-based nanoparticles are believed to modulate numerous intracellular signal transduction pathways and cause alterations in cellular functions.Citation6,Citation14–Citation16 These effects may result in the induction of apoptosis, the inhibition of cellular proliferation, and the promotion of cytokine secretion.Citation17,Citation18 The ability of nanoparticles to elicit a wide array of cellular effects highlights the need for additional studies linking the biological mechanism of action with specific nanomaterial properties. In this respect, the computational part of the proposed framework could facilitate the future development of specific Quantitative structure-activity relationships that model nanomaterial exposure to specific biological responses.
Acknowledgments
This work was funded by the Department of Veterinary Physiology and Pharmacology at Texas A&M University, the Norman Hackerman Advanced Research Program, and the Product Quality Research Institute.
Disclosure
The authors report no conflicts of interest in this work.
References
- GrassianVHO’ShaughnessyPTAdamcakova-DoddAPettiboneJMThornePSInhalation exposure study of titanium dioxide nanoparticles with a primary particle size of 2 to 5 nmEnviron Health Perspect2007115339740217431489
- ZhuSOberdörsterEHaaschMLToxicity of an engineered nanoparticle (fullerene, C60) in two aquatic species, Daphnia and fathead minnowMar Environ Res200662S5S916709433
- OberdörsterGOberdörsterEOberdörsterJNanotoxicology: an emerging discipline evolving from studies of ultrafine particlesEnviron Health Perspect2005113782383916002369
- SchanenBCKarakotiASSealSDrakeDR3rdWarrenWLSelfWTExposure to titanium dioxide nanomaterials provokes inflammation of an in vitro human immune constructACS Nano2009392523253219769402
- YamashitaKYoshiokaYHigashisakaKCarbon nanotubes elicit DNA damage and inflammatory response relative to their size and shapeInflammation201033427628020174859
- RomoserAAFigueroaDESooreshADistinct immunomodulatory effects of a panel of nanomaterials in human dermal fibroblastsToxicol Lett2012210329330122342292
- RomoserAAChenPLBergJMQuantum dots trigger immunomodulation of the NFκB pathway in human skin cellsMol Immunol20114812–131349135921481475
- HanschCQuantitative approach to biochemical structure-activity relationshipsAcct Chem Res196928232239
- CabantchikZIKnaufPARothsteinAThe anion transport system of the red blood cell. The role of membrane protein evaluated by the use of ‘probes’Biochim Biophys Acta1978515323930229666
- HanschCDunnWJ3rdLinear relationships between lipophilic character and biological activity of drugsJ Pharm Sci19726111194550859
- LeesonPDSpringthorpeBThe influence of drug-like concepts on decision-making in medicinal chemistryNat Rev Drug Discov200761188189017971784
- LeoAHanschCElkinsDPartition coefficients and their usesChem Rev1971716525616
- SouthallNTDillKAHaymetADJA view of the hydrophobic effectJ Phys Chem B20021063521533
- EomHJChoiJOxidative stress of silica nanoparticles in human bronchial epithelial cell, Beas-2BToxicol In Vitro20092371326133219602432
- EomHJChoiJOxidative stress of CeO2 nanoparticles via p38-Nrf-2 signaling pathway in human bronchial epithelial cell, Beas-2BToxicol Lett20091872778319429248
- GojovaAGuoBKotaRSRutledgeJCKennedyIMBarakatAIInduction of inflammation in vascular endothelial cells by metal oxide nanoparticles: Effect of particle compositionEnviron Health Perspect2007115340340917431490
- HanleyCThurberAHannaCPunnooseAZhangJWingettDGThe influences of cell type and ZnO nanoparticle size on immune cell cytotoxicity and cytokine inductionNanoscale Res Lett20094121409142020652105
- JengHASwansonJToxicity of metal oxide nanoparticles in mammalian cellsJ Environ Sci Health A Tox Hazard Subst Environ Eng200641122699271117114101
- XiaXRMonteiro-RiviereNARiviereJEAn index for characterization of nanomaterials in biological systemsNat Nanotechnol20105967167520711178
- FourchesDPuDTassaCQuantitative nanostructure-activity relationship modelingACS Nano20104105703571220857979
- ZhangHJiZXiaTUse of metal oxide nanoparticle band gap to develop a predictive paradigm for oxidative stress and acute pulmonary inflammationACS Nano2012654349436822502734
- PuzynTLeszczynskaDLeszczynskiJToward the development of “Nano-QSARs”: advances and challengesSmall20095222494250919787675
- SayesCMIvanovIComparative study of predictive computational models for nanoparticle-induced cytotoxicityRisk Anal201030111723173420561263
- SayesCMReedKLWarheitDBAssessing toxicity of fine and nanoparticles: comparing in vitro measurements to in vivo pulmonary toxicity profilesToxicol Sci200797116318017301066
- WarheitDBHokeRAFinlayCDonnerEMReedKLSayesCMDevelopment of a base set of toxicity tests using ultrafine TiO2 particles as a component of nanoparticle risk managementToxicol Lett200717139911017566673
- ChenSZhuYZhangDYangJ-YFeature extraction approaches based on matrix pattern: MatPCA and MatFLDAPattern Recognit Lett200526811571167
- LuJWPlataniotisKNVenetsanopoulosANFace recognition using kernel direct discriminant analysis algorithmsIEEE Trans Neural Netw200314111712618237995
- PangSOzawaSKasabovNIncremental linear discriminant analysis for classification of data streamsIEEE Trans Syst Man Cybern B Cybern200535590591416240767
- SchneiderTGriffiesSMA conceptual framework for predictability studiesJ Climate1999121031333155
- JiangJOberdörsterGBiswasPCharacterization of size, surface charge, and agglomeration state of nanoparticle dispersions for toxicological studiesJ Nanopart Res20091117789