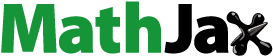
Abstract
Background
Beside the promising application potential of nanotechnologies in engineering, the use ofnanomaterials in medicine is growing. New therapies employing innovative nanocarrier systems toincrease specificity and efficacy of drug delivery schemes are already in clinical trials. Howeverthe influence of the nanoparticles themselves is still unknown in medical applications, especiallyfor complex interactions in neural systems. The aim of this study was to investigate in vitroeffects of coated silver nanoparticles (cAgNP) on the excitability of single neuronal cells and tointegrate those findings into an in silico model to predict possible effects on neuronalcircuits.
Methods
We first performed patch clamp measurements to investigate the effects of nanosized silverparticles, surrounded by an organic coating, on excitability of single cells. We then determinedwhich parameters were altered by exposure to those nanoparticles using the Hodgkin–Huxleymodel of the sodium current. As a third step, we integrated those findings into a well-definedneuronal circuit of thalamocortical interactions to predict possible changes in network signalingdue to the applied cAgNP, in silico.
Results
We observed rapid suppression of sodium currents after exposure to cAgNP in our in vitrorecordings. In numerical simulations of sodium currents we identified the parameters likely affectedby cAgNP. We then examined the effects of such changes on the activity of networks. In siliconetwork modeling indicated effects of local cAgNP application on firing patterns in all neurons inthe circuit.
Conclusion
Our sodium current simulation shows that suppression of sodium currents by cAgNP resultsprimarily by a reduction in the amplitude of the current. The network simulation shows that locallycAgNP-induced changes result in changes in network activity in the entire network, indicating thatlocal application of cAgNP may influence the activity throughout the network.
Introduction
The areas of application of nanomaterials have increased in the last decade and seem to beunlimited. They range from the application in paints and cosmetics to textiles, foods, food-gradepackaging, and various other technical products. The use of nanomaterials in medicine is notablesince it comes with high expectations and hopes for treatment of hitherto untreatable diseases.Nanoparticles (NPs) offer a wide range of sizes, morphologies, and surface features that assurepotential implementation in drug delivery, diagnostics, and therapy.Citation1 A big challenge in current research is to overcome existingdrug-delivery barriers, such as biomembranes and the blood –brain barrier employing nonviralvectors functioning at the nano-to-micro scale. Those systems could be utilized for the systemicdelivery of drugs or genes to target cells for therapy of cancer, inflammation, or for the intendedmodulation of neural activity in brain tissue.Citation2,Citation3
Regarding nanotoxicology, research is in its infancy, and many questions remain unanswered.Studies in biological systems show that the physical parameters of nanosized particles influencetheir nonspecific absorption in cells and their potential to induce cellular responses.Citation4 Existing studies provide limited information in termsof the cellular processes affected by exposure to NPs and their subsequent impact. To understand howNPs of different material, size, and geometry interact with cells, it is necessary to explore theNP-membrane interaction processes on the molecular scale, ie, receptor-binding, endocytosis, andsignaling activation.Citation5,Citation6 Regarding excitable cells, a starting point is the electrophysiologicalbehavior of cells exposed to NPs. Recent nanotoxicology studiesCitation7–Citation9 identified(pure) silver nanoparticles (AgNP) as potentially toxic in tissue, especially in neurons. Xu etal,Citation10 Zhao et al,Citation11 and Lui et alCitation12examined the influence of nanosized CuO, ZnO, and Ag on single neurons in vitro, using the patchclamp method. They observed effects of AgNP on the amplitude and the time course of the sodiumcurrent (INa). The underlying mechanism of the changes in INa was notdetermined.
We have taken a systems approach to the question of the effects NPs brought into contact to a fewcells of a neuronal circuit on network activity of neuronal populations. We carried out patch clamprecordings in neuroendocrine cells and examined the effects of coated silver nanoparticles (cAgNP)on excitability. We observed a suppression of sodium currents and used an established model ofdynamic changes in membrane conductance to model the observed changes in INa. Based onthe fitting results, we computationally reproduced the observed changes in INa parametersin individual neurons and then tested the effects of these changes in a model neuronal network. Theoutcome of this simplified in silico model serves as initial approximation of in vivoneuromodulatory effects of cAgNP in neuronal circuits.
Material and methods
The first objective was to determine the electrophysiological effects of cAgNP on singleexcitable cells. For this purpose we applied cAgNP with an organic coating that prevents particleagglomeration and release of free Ag+, which is suitable for drug delivery. Patch clamprecordings on chromaffin cells were carried out to evaluate the effects of cAgNP on the voltagegated sodium channels (isoform Nav 1.7). We applied the Hodgkin-Huxley (HH) model ofdynamic changes in membrane conductance (HH model)Citation13 and computationally fitted this model to our patch clamp data. The utilization of theDifferential-Evolution Algorithm (DE)Citation14 allowed usan efficient fit of the model to the recorded data. As a consequence, changes in components of theHH model were observed which shed light on the NP membrane interactions. The altered parameters wereapplied to modeled neurons within a neuronal feedback circuit. Thus we explored possible effects ofthose cAgNP on network dynamics in silico, ie, modeling intrinsic single cell dynamics and networkoscillations in a circuit by reverting to an extended HH-type formalismCitation13 and dynamic-synaptic coupling, based on an example of thethalamocortical model introduced by Llinás et alCitation15–Citation18 that served as theoreticalfundament for the computational implementation. We compared the neuronal response dynamics of thenetwork neurons assuming with and without the interference of cAgNP in specific and nonspecificthalamic cells (STC/NSTC) and in reticular thalamic cells (RTN)(all of isoform Nav1.2).
Coated silver nanoparticles
The cAgNP was prepared by thermal decomposition of silver (Ag) oleate and stabilized byoleylamine. These hydrophobic NP had a mean diameter of 5 nm ± 2 nm. The particles werecapped with an amphiphilic polymer (polyethylene glycol) for transfer in aqueous phase and stericstabilization. For further information, see Pellegrino et al.Citation19 Prior to use, particles were suspended in ultrapure water and thenfiltered through a sterile 0.22 μm membrane. Inductively coupled plasma atomic emissionspectroscopy (ICP–AES) (HORIBA, Jobin Yvon GmbH, Munich, Germany) determination found atotal silver content of 1.3 mMol in the resulting nanoparticle dispersion, including a fraction of0.4 μMol free Ag+. The concentration and the type of molecules and ions on theparticle surface lead to electrostatic interaction in the dilution medium, and this changes thecoated particles’ hydrodynamic diameter to a mean value of 13 nm ± 2 nm in purewater, 16 nm ± 4 nm in Roswell Park Memorial Institute cell culture medium (LifeTechnologies, Carlsbad CA, USA) and 9 nm ± 1 nm in 10 × phosphate-buffered saline(PBS) (Life Technologies). A DynaPro Titan instrument (Wyatt Technology Europe GmbH, Dernbach,Germany) was used to perform dynamic light scattering with a laser wavelength of 831 nm. Themeasured zeta potential (Zetasizer Nano, Malvern Instruments, Malvern, UK) of the nanoparticles was−69 mV in pure water (steric stabilized). Because the solution ingredients bind to thenegatively charged particle surfaces, the zeta potential was significantly reduced when dispersed in10 × PBS r (−6 mV) and Roswell Park Memorial Institute medium (−16 mV).
Chromaffin cell model
Chromaffin cells are neuroendocrine cells which are well characterized electrophysiologically andare ideally suited for voltage clamp analysis of membrane currents due to their small size andspherical shape.Citation20–Citation22 We focused on voltage-gated sodium currents which initiate actionpotentials and propagation in excitable cells.Citation23Xu et al,Citation10 Zhao et al,Citation11 and Lui et alCitation12tested the influences of CuO, ZnO, and uncoated AgNP on sodium currents in hippocampal neurons (Na1.2) by using the patch clamp method. They found effects of AgNP on the amplitude and the timecourse of the sodium current (INa) in their experiments. Since we observed similarfindings on chromaffin Nav 1.7 channels, it is likely that this effect is notNav isoform specific. This is consistent with the fact that the Nav isoformsin hippocampal cells (Nav 1.1 and 1.2) and in chromaffin cells (Nav 1.7) havean amino acid sequence similarity of about 95% and show nearly identical electrophysiologicalbehavior.Citation23–Citation28 We employed our findings on Nav 1.7 channels for themodeling approach, in which we devolve the measured effects of cAgNP on chromaffin cellvoltage-gated sodium currents, to voltage-gated sodium channels of thalamic neurons (STC, NSTC,RTN).
For cell preparation, the adrenal glands from 1-day-old to 3-day-old mice were collected anddigested with 20 units of papain (Worthington Biochemical Corp, Lakewood NY, USA) at 37°Cfor 25 minutes to 30 minutes. After trituration, cells were plated on 25 mm cover glasses and thenincubated at 37°C and 8% CO2. Chromaffin cells were kept in culture medium(ITS–X, DMEM with GlutaMax and 100 units penicillin/streptomycin; Life Technologies), priorto recordings 12–48 hours later.
Patch clamp measurements
Patch clamp measurements in the whole-cell configuration were carried out with3–6MΩ pipettes using an EPC–9 patch clamp amplifier controlled by PULSEsoftware (HEKA Instruments Inc, Lambrecht, Germany). Sodium current measurements were performed onboth cAgNP exposed and naïve cells at a holding potential of −70 mV at roomtemperature. The measurement procedure was the following: In a new dish, four control cells wererecorded first; each recording comprised around ten depolarizations to −10 mV with the stepduration of 30 s. While the last control cell patch was still active, the cAgNP dispersion waspipetted into the chamber and then effects on the patched cell could be measured. Afterward thesodium currents of three to five more cells from the treated dish were collected. This procedure wasrepeated for concentrations of 13 μMol, 16 μMol, 43 μMol, 130 μMol,and 1.3 mMol, respectively. As diluent, the extracellular solution (HEPES-buffered saline solution)was utilized. Altogether, the current traces of 70 control and 45 cAgNP-exposed chromaffin cellswere recorded. The extracellular solution contained 145 mM NaCl; 2.4 mM KCl; 10 mM HEPES; 1.2 mMMgCl2; 2.5 mM CaCl2; and 10 mM glucose (pH 7.5). The pipette solutioncontained 135 mM Cs–aspartate; 10 mM Cs–HEPES; 5 mM Cs–ethylene glycoltetraacetic acid (EGTA); 3 mM CaCl2; 1 mM MgCl2; 2 mM Mg–ATP; and 0.3mM Na2–GTP (pH 7.2).
HH fitting employing Differential Evolution Algorithm
We fit INa from the patch clamp data with the HH modelCitation13 to determine which parameters might be modified under the influenceof cAgNP Those factors directly link to physiological mechanisms involved in action potentialgeneration. The basic HH equationsCitation29 are given by:
The corresponding empirical theorems for the transferrate coefficients are:
Using a voltage clampCitation30 for a voltage step, thetransfer-rate coefficients αm, βm, αh, andβh.change instantly to new values (in steady state). As in steady state, thetransfer-rate coefficients in EquationEquation 3(3) are constant, so the primary differential equation can be readily solved for m and h, giving:
The mathematical term of h is similar to the m in EquationEquation 4(4) . By applying voltage clamp, a voltage step initiates anexponential change in m (and h) from its initial value of m0 (or h0) at t = 0s toward the steady-state value of m∞ (or h∞) at t =∞s. Finally, the sodium current INa that has to be fitted is then given by:
Various problems in applied mathematics have target functions that are noncontinuous, nonlinear,nondifferentiable, flat, noisy, multidimensional, or have many local minima, constraints, orstochasticity. Fitting the steady-state HH model (EquationEquations 1(1) –Equation7
(7) ) to themeasured patch clamp data requires the solution of a high dimensional inverse problem: Since theessential HH model equations that need to be taken into account for the fitting consist of 13independent parameters (constants evaluated by HH) that have to be estimated this problem cannot besolved analytically.
Approximate solutions can be achieved by the Differential-Evolution (DE) Algorithm, a techniquethat originates from the genetic annealing algorithm, introduced by Price et alCitation14 in 1996. This invention offers a global optimization method bystochastic, population-based optimization and can be utilized to optimize real parameters and realvalued functions, such as the 13 parameters in the HH model-fitting problem. A detailed descriptionof the algorithm can be found in Price et al.Citation14
In terms of the introduced fitting problem, the DE Algorithm was implemented to minimize thedistance between the measured sodium current and the one iteratively calculated by the HH model. Asa consequence, the parameters for the best fit were evaluated. The 13 parameters, that give thefreedom for the curve-fitting process, represent all the empirical coefficients in EquationEquations 1(1) –Equation7
(7) that were estimated by HH in their experimentsin 1952.Citation13 The following equations indicate againthe applied HH formulations, but now with the 13 free parameters expressed by ξ.1− ξ.13:
Thalamocortical interactions
The thalamus provides the major route for afferents to the neocortex and extrasensory regions ofthe brain. The traditional concept treating the thalamus as the sensory gateway to the cortex is anoversimplification, because the cerebral cortex receives input not only from the sense-specificnuclei but also from the nonspecific thalamic nuclei, which have multimodal connections to thecortex, probably governing overall arousal.Citation31 Withrespect to the interactions between the specific and nonspecific thalamic loops, Llinás etal suggested that, rather than a gate, the thalamus is a hub from which any area in the cortex cancommunicate with any other.Citation17 Bidirectionality isthe most remarkable feature of this thalamocortical connectivity. Thalamic nuclei receive reciprocalconnections from the cortical areas that they project to, though the number of corticothalamicfibers is significantly greater than the number of thalamocortical axons.Citation31,Citation32 These reciprocalthalamocortical connections create bidirectional neuronal loops between the thalamus and the cortex.Consequently, distributed neural representations of simultaneous perceptual events or features couldbe related to each other within the thalamocortical system. Binding input from different sensorymodalities into a single cognitive event is assumed to be a consequence. The underlying mechanismhas been proposed as temporal binding, a process based on the synchronization of neuralsignals.Citation33 Different studies have shown thatcertain types of cognitive functions are intimately related to synchronized neuronal oscillations atboth low (4–7/8–13 Hz) and high (18–35/30–70 Hz) frequencies.Citation18,Citation34–Citation38 The activity patterns ofthese oscillations are formed within one or more bounded areas (corresponding to corticalcolumns).Citation17,Citation18,Citation39 A subset of theemployed cortical neurons can generate repetitive, high-frequency burst discharges, referred to aschattering cells. Those can generate bursts with intraburst frequencies of ~300–750 Hz andinterburst frequencies of ~8–80 Hz.Citation40–Citation42 We focused on thethalamocortical activity of those chattering cells since their firing patterns in burst mode containmore information, to evaluate the impact of NP on the circuit’s activity.
Applied thalamocortical network
The simplified in silico model that we used to predict in vivo behavior is based on the detailedmodel of thalamocortical interaction and binding suggested by Llinás et al.Citation15–Citation18 More information about the Llinás model as a basis for the developedcorticothalamic network can be found in the supplementary materials. The principal result of thismodel is that the intrinsic electrical properties of neurons and the dynamic events resulting fromtheir connectivity cause global resonant states. Resonant states in the network will change due tosmall alterations in the neural signaling characteristics. The applied circuit is based on kineticmodels of pyramidal neurons (here: chattering cells, PY),Citation43–Citation46 inhibitory corticalinterneurons (IN),Citation45,Citation47 thalamic cells (TC) including specific thalamic cells and nonspecificthalamic cells,Citation48–Citation50 and reticular thalamic neurons.Citation43,Citation45,Citation51,Citation52Each single neuron model is considered as one compartment (except PY, modeled as two compartments)and is represented by coupled differential equations, according to an extended HH-type scheme.Citation13 The scheme of synaptic connectivity in the developedsimplified thalamocortical network model is represented by . In this model, specific thalamic inputs are represented by a thalamic neuron (STC)that projects to both PY neuron and inhibitory cortical interneurons located in cortical layer 4,after sending axon collaterals to the RTNs. The NSTC represents intralaminar, nonspecific thalamicinputs, and projects to neocortical layer 1 after sending axon collaterals to the reticular thalamicneurons. Specific thalamic cells and nonspecific thalamic cell neurons generate excitatorypostsynaptic potentials that are mediated by fast excitatory receptors in the model. RTNs projectwith inhibitory characteristic to specific and intralaminar nucleus neurons. Inhibitory fast andslow receptors in the thalamic neurons both mediate the inhibitory postsynaptic potentials of thosecells. The RTN neurons also have reciprocal inhibitory synaptic connectivity. The correspondingactivity is mediated by fast inhibitory receptors.
Figure 1 Schematic description of synaptic interconnections in the simplified computational model of thethalamocortical network.
Abbreviations: IN, interneurons; RTN, reticular thalamic cells; PY, pyramidalneurons; STC, specific thalamic cells; NSTC, nonspecific thalamic cells.
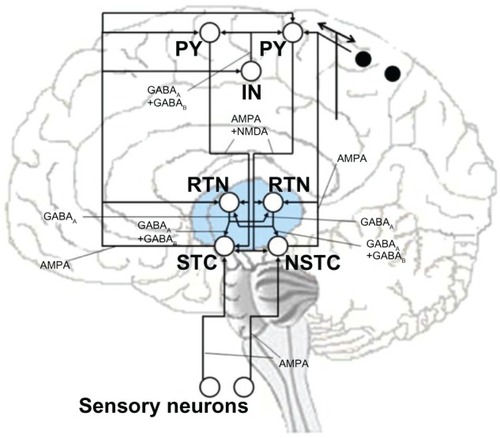
In the model, the cerebral cortex was considered as a simple network model of inhibitory IN andexcitatory PY neurons.Citation15–Citation18 Although this is a highly simplified representationof the neocortex’s multilayered structure, no additional complexity was required for thetheoretical modeling. Consequently, the pyramidal neurons in layers 4 and 5 are described by singleneurons () that receive inputs and projectto both STC and NSTC cells and have axon collaterals to the RTN neurons. In the model, four PYneurons were included, two of which receive input from the STC to provide specific sensory input tothe cortex. This structure represents the specific resonant loop as is shown in on the left. The right side of indicates the nonspecific resonant loop, where three PY neuronsreceive their inputs in a more diffuse way from NSTC to provide the multimodal connectivity to thecortex. The two black spots represent two PY neurons that receive inputs and project back to theNSTC. In this model, the corticofugal excitation of the PY is mediated by both fast and slowexcitatory receptors. In the circuit, all PY neurons receive axons from the cortical inhibitory IN,in which the inhibitory postsynaptic potential elicited by this cell is also mediated by both fastand slow excitatory receptors. Lastly, the essential sensory inputs to activate STC and NSTC areprovided by including two sensory neurons in the present model (). Thus, the synaptic projections from those sensory neuronsactivate the fast receptors of the thalamic nuclei. Every neuron of the simplified thalamocorticalmodel receives various synaptic inputs that are modeled as the sum over all synaptic currents thateach cell receives.Citation53,Citation54 Accordingly, every neuron is described by the generic membraneequation:
Here, i denotes the postsynaptic neuron, and j stands for the specific ionic type. Further,gj is the maximal conductance, m the time and voltage dependent activation variable, h isthe corresponding (time and voltage dependent) inactivation variable and finally (Vi– Ej) is the difference between membrane potential and reversal potential of eachion. The following generic equation represents the synaptic currents in the system:
For computational efficiency, a reduced transmitter release model is used assuming allintervening reactions in the release process are relatively fast and thus can be considered insteady state (instantaneous).Citation55 Consequently, thestationary relationship between the transmitter concentration (T) and presynaptic voltage isdescribed by a simple sigmoidal function:Citation55
In the model, all initial conditions and set parameters representing a single cell or synapticconnection come from electrophysiological measurements on the specific neurons taken from citedreferences. Wang et al,Citation43,Citation56 Golomb et al,Citation46 andDestexhe et alCitation55,Citation58 contain detailed descriptions of the particular equations and thecorresponding cell- and transmitter-specific parameters. According to Destexhe et al,Citation53 different values of Ih and IKLwould cause heterogeneity in the intrinsic properties of the cells. Therefore, the intrinsicconductance of gh and gKL for the STC and the NSTC and gKL for theRTN neuron models are slightly different in the present model.
To simulate the effects of cAgNP in contact with thalamic cells, ie, STC, NSTC, and RTN,respectively, the changes of the intrinsic currents that we identified by fitting our patch clampdata to the HH model were applied to those cells. The kinetics and voltage dependence of the modeledcurrents are very similar to the currents measured in patch clamp experiments (see the chromaffincell model section). MATLAB was used as the simulation environment, in which the differentialequations were solved by employing a fourth-order Runge–Kutta method.
Results
The results of measurements of sodium current amplitudes are shown in . Averages (medians) (x̃) and standard deviations(σ) in INa amplitudes before and after cAgNP addition and differences in median(Δx̃) between controls and cAgNP exposed cells at different concentrations arerepresented there. Since the amplitudes of the controls (base line) vary, the values have beennormalized. indicates the amplitudes ofINa before and after cAgNP addition as total values.
Table 1 Normalized amplitudes of INa before and after cAgNP addition (holding potential−70 mV; depolarization potential −20 mV)
The attenuation of the amplitude occurred rapidly after the application of the cAgNP and was thenrecorded in another three to five cells in the treated dish. Suppression of INa wasobserved in 43 of 45 experiments. In another group of experiments, cAgNP (16 μM) wereapplied locally via an application pipette to chromaffin cells after the recording of control actionpotentials. Current voltage relationships were recorded under control conditions and afterapplication of cAgNP. shows arepresentative experiment. shows thecurrent-voltage relation fit, and showssingle depolarizations to −0 mV, which were applied to track changes in sodium currentamplitude. INa was reduced within seconds of application with maximal block occurringafter about two minutes. In some cases, substantial recovery occurred within 10 minutes ofapplication. There was no significant shift in the voltage-dependence of the INa (3.1± 2.6 mV mean ± SD) and no significant shift in the null potential of INa(39 ± 5.1 mV and 44 ± 4.9 mV; mean ± SD).
Figure 2 Local application of cAgNP to chromaffin cells. (A) A representativecurrrent-voltage (IV)-curve of a cell before and after application of cAgNP (16 μMol, gray;corresponding control, black). (B) records of INa before and after localapplication of cAgNP after 60 seconds (s) and 120 seconds.
Abbreviations: cAgNP, coated silver nanoparticles; INa, sodium current;ms, milliseconds; mV, millivolt; NP, nanoparticles; pA, picoampere.
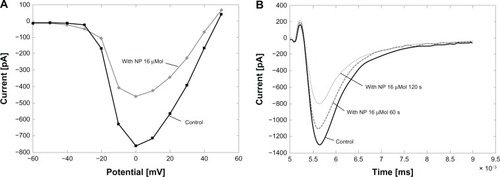
Five representative sodium current curves taken after the application of cAgNP and theircorresponding controls from the 1.3 mM dataset have been selected for the DE fitting procedure.Before processing, a cubic spline interpolation was employed to smooth the curves, to reduce noise,and produce consistent vector length. showsrepresentative sodium currents as continuous lines. shows a control sodium current. The right panel () shows the sodium current of the same cell after the addition of cAgNP.
Figure 3 Measured sodium current. Measured sodium current without (A) and with(B) cAgNP (solid lines, holding potential −70 mV, depolarization potential−20 mV), and the curve fits by DE (dashed lines).
Abbreviations: pA, picoampere;INa, sodium current; cAgNP, coated silvernanoparticles; NP, nanoparticles; DE, Differential-Evolution Algorithm.
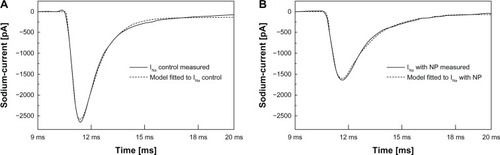
The dashed lines in represent thecorresponding model fittings to these curves by the DE Algorithm. The fitting process generatedestimates of the 13 free coefficients (ξ) in EquationEquations 9(9) and Equation10
(10) . There were conspicuous changes in parameters ξ.1,2,6,7,8,9,10, and 12 (EquationEquations 9
(9) and Equation10
(10) ). By transferring those findings back into a more macroscopic(transfer-rate coefficients) level of the H equations, the transfer-rate coefficientsαh, αm, βm, and the reversal potentialVNa (EquationEquation 9
(9) and EquationEquation 10
(10) ) were found to be potentiallymodified. Since the reduction in amplitude of INa occurred without an appreciable shiftin either activation voltage or null potential, it is unlikely that a change in VNa isinvolved in the effects of cAgNP on INa. We therefore fixed VNa and ran thesimulation again. As expected very good fits could be achieved when only changes in thetransfer-rate coefficients αh, αm, and βmwere allowed. shows the changes of thesevariables after particle exposition in a normalized bar diagram. Changes in these parameters wererequired for a good fit to the cAgNP data.
Figure 4 Differences in percentages.
Notes: Differences of model fitted αh, βh,αm, βm, and GNamax between the measured sodiumcurrent with cAgNP in 130 (μMol concentration and the corresponding controls. Theξ.13 (stim–time) is not considered.
Abbreviation: cAgNP, coated silver nanoparticles.
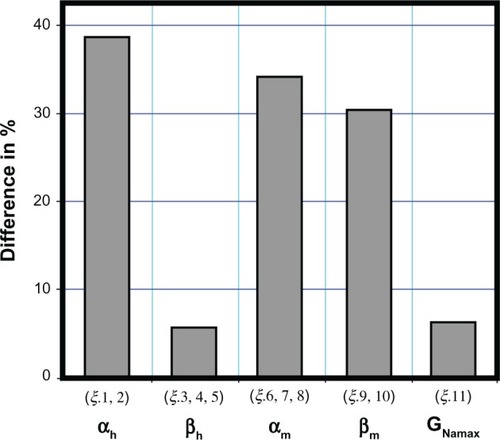
shows the output of a representativesodium current fit with the relevant parameter changes (dashed lines). To estimate the impact ofcAgNP on the activity in a neuronal feedback circuit, the simulated results of the first model wereemployed to modify the properties of INa of thalamic cells of the network model ofcorticothalamic interactions. The initial conditions for all gating variables of each neuron modelinvolved were calculated according to their steady-state functions, whereas the membranepotential’s initial conditions were taken from cited references. To activate the feedbackcircuit, a single action potential was generated in the model sensory neuron which directly projectsto STC by utilizing a brief depolarization current pulse of Iapp = 6μA/cm2. This leads to a small depolarization in STC and as a consequence of thespecific synaptic parameters, all neurons fire in a 6–8 Hz bursting oscillation mode. Theresulting membrane potentials of the cortical PY neuron 1, 2, 3, and 4 are shown in for a time interval of 4 seconds.
Figure 5 Currents for control. DE fittings (dashed lines) utilizing just the seven identifiedHodgkin–Huxley equation parameters (αh, αm, andβm) and the corresponding electrophysiologically measured currents for control(A) and cAgNP (130 μMol) transfected (B).
Abbreviations: pA, picoampere; INa, sodium current; cAgNP, coated silvernanoparticles; NP, nanoparticles; DE, Differential-Evolution Algorithm; ms, milliseconds.
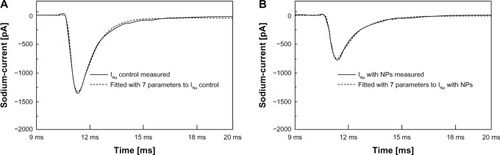
Figure 6 Firing pattern differences of pyramidal neurons.
Note: Differences in firing patterns of PY1, PY2, PY3, and PY4 neuron for 4 secondsbefore and after cAgNP application in thalamic neurons.
Abbreviations: PY, pyramidal neurons; mV, millivolt; cAgNP, coated silvernanoparticles; NP, nanoparticles; ms, milliseconds.
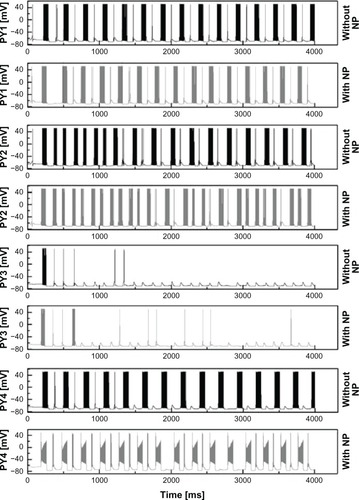
illustrates the resulting membranepotentials of the cortical inhibitory IN, RTN1, RTN2, STC, and the NSTC neuron for 4 seconds. Theblack graphs in both figures show the behavior of the engaged cells after the initial AP in thesensory neuron under normal physiological conditions.
Figure 7 Firing pattern differences in IN, RTN1, RTN, STC, NSTC neurons.
Note: Differences in firing patterns of IN, RTN1, RTN2, STC, and NSTC neuron for 4seconds before and after cAgNP application in RTN and STC neurons.
Abbreviations: IN, interneurons; mV, millivolt; STC, specific thalamic cells; NSTC,nonspecific thalamic cells; RTN, reticular thalamic cells; cAgNP, coated silver nanoparticles; ms,milliseconds, NP, nanoparticles.
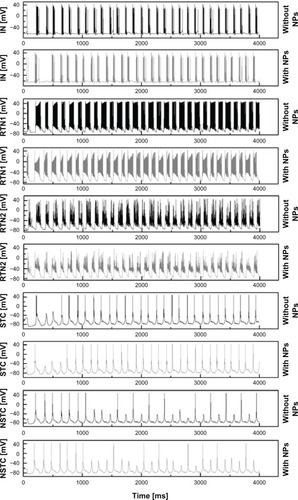
The gray lines show the behavior of the same cells in the network after altering INaof thalamic cells, ie, NSTC, STC, and RTNs consistent with the changes in INa observedafter cAgNP application. Variations in the firing patterns of all cells embedded in the simplifiednetwork model were apparent after this manipulation. Changes in activity can be seen in as mean values, including the correspondingstandard deviations (σ) for interburst intervals, the resulting interburst frequencies andthe intraburst frequencies before and after hypothesized cAgNP presence in thalamic cells for 4seconds (only the bursting neurons were considered for the statistics).
Table 2 Changes in neuronal bursting after cAgNPs application
reveals that the interburst frequenciesare slightly increased after cAgNP application in the thalamus core. The intraburst frequencies arefound to be slightly decreased for PY1, PY2, and for IN. In contrast, the intraburst frequencies forboth RTN1 and RTN2 are slightly increased whereas the intraburst frequency for PY4 is more thandoubled after NP addition.
Discussion
The patch clamp measurements show that the application of cAgNP to chromaffin cells reduces theamplitude of sodium currents. The changes were rapid partially reversible and dose-dependent. Basedon the HH fitting, the cAgNP may affect the electrically charged h–particles by thealteration to the noninactivating (toward open) state at changed transferrate coefficientαh. Additionally, the m–particles are influenced by the transition to theopen and closed state as αm plus βm is altered. However, thelack of a rightward shift of the IV curves indicate that any effects on the voltage sensors orgating lead to failure to gate or a decreased channel conductance, which are not overcome bystronger depolarization. A shift in null potential, which might result from a change in ionselectivity, also cannot explain the reduced INa. We cannot distinguish between these twopossibilities based on our data.
It is possible that cAgNP may produce mechanical effects on the ion channels leading to lowerconductivity or fewer channels that reach the open state. An interaction of cAgNP with the referenceelectrode can be ruled out, since the holding current was not affected by cAgNP addition.
In any case, the net effects of cAgNP on neuronal feedback circuits will be a reducedexcitability of affected cells, and we have simulated these effects. The simulation indicates thatapplication of cAgNP in the thalamus will result in dramatic changes in thalamocortical activity.This could occur, for instance, if cAgNP used as a drug carrier crossed the round window membrane ofthe cochlea and were retrograde transported. Praetorius et alCitation59 evaluated the safety and distribution of cyanin fluorescent dye(Cy3)-labeled silica NP in in vivo experiments by placing them on the round window membrane of adultmice. After 4 days, SiO2–NP signals could be found in the superior olivarycomplex. Their observation proposes a retrograde axonal transport for the applied NP. Our developedin silico model enables the investigation of potential effects of NP which enter the central nervoussystem.
Our simulations examine the systemic influences of cAgNP in neuronal systems. Though ourcomputational model of the thalamocortical network is highly simplified and does not consider inputsor projections to other involved brain regions, it indicates that reduced excitability of a fewneurons in such a circuit has dramatic effects on network activity. It is able to predict possibleconsequences of cAgNP introduction to neuronal feedback circuits.
The in vivo effects of such NP on any network will likely be complicated by additional effects ofNP. We introduced changes in voltage-dependent sodium currents in our model. NP-induced changes inmechanisms affecting excitability, such as other ion channels, were not examined though effects onpotassium channels have been reported.Citation60 In thefuture, we will investigate cAgNP effects on other aspects of membrane excitability and includethese findings into our model.
Conclusion
The extension of our results in single chromaffin cells to neuronal circuits via modeling of thethalamocortical network allow us to make initial predictions as to what effects coated silvernanoparticles’ suppression of sodium currents will have on thalamocortical circuits.Alteration of the properties of RTN, STC, or NSTC neurons, based on our HH model fits ofINa after treatment with cAgNP, shows that NP brought into contact with few cells of aneuronal feedback circuit can dramatically alter network rhythms of large neuronal populations.
Supplementary materials
The Llinás model as basis for the developed corticothalamic network
Llinás et al suggested a reasonably detailed model of thalamocortical interaction andbinding.Citation1–Citation4 Their principal finding is that the intrinsic electrical properties ofneurons and the dynamic events resulting from their connectivity cause global resonant states.Resonant states in the network will change by just small alterations in the neural signalingcharacteristics. The Llinás model exposing the thalamocortical circuit can be found inLlinás et al.Citation3 For our purpose, we focuson two types of thalamic cells: first, specific thalamic cells (STC), also known as thalamic corecells due to their focused projection to an individual cortical area and, second, intralaminarnon-specific thalamic cells (NSTC), also known as thalamic matrix cells as they project acrosslarger neocortical areas in a more dispersed way.Citation5We utilized two thalamocortical resonant loops for our model. The description of neural interactionsand dynamics can be found in the subsequent section. It was proposed by Llinás et al thatnone of these two systems alone is able to generate cognition, and consistent with this model,damage to the nonspecific thalamus induces deep disturbances of consciousness while damage to thespecific systems causes loss of particular modality.Citation3 Their statement implies that these two systems can only generate a cognitive experiencesynced, based on the summation of nonspecific and specific activity along the dendritic tree of thecortical element, by coincidence detection at the pyramidal neuron.Citation3,Citation4 To conclude, thesystem operates on the basis of thalamocortical resonant columns that can support global cognitiveexperiences. In this context, the specific system provides the content that relates to the externalworld while the nonspecific system would give rise to the temporal conjunction (binding circuit).This well explored and accepted model serves as the theoretical fundament for our investigations onnanoparticle-induced signaling modifications in neuronal circuits.
Table S1 Amplitudes of INa before and after cAgNP addition as total values
References
- LlinásRRibaryUCoherent 40-Hz oscillation characterizes dream state in humansProc Natl Acad Sci U S A1993905207820818446632
- LlinásRRibaryUJoliotMWangXJContent and context in temporal thalamocortical bindingBuzsákiGChristenYTemporal Coding in the Brain (Research and Perspectives in Neurosciences)Heidelberg, GermanySpringer-Verlag1994252272
- LlinásRRibaryUContrerasDPedroarenaCThe neuronal basis for consciousnessPhilos Trans R Soc Lond B Biol Sci19983531377184118499854256
- LlinásRLeznikEUrbanoFJTemporal binding via cortical coincidence detection of specific and nonspecificthalamocortical inputs: a voltage-dependent dye-imaging study in mouse brain slicesProc Natl Acad Sci U S A200299144945411773628
- ShepherdGMThe Synaptic Organization of the Brain5th edOxford, New YorkOxford University Press2001
Disclosure
The authors report no conflicts of interest in this work.
References
- GuptaAKGuptaMSynthesis and surface engineering of iron oxide nanoparticles for biomedicalapplicationsBiomaterials200526183995402115626447
- ZhangYSatterleeAHuangLIn vivo gene delivery by nonviral vectors: overcoming hurdles?Mol Ther20122071298130422525514
- LamarreBRyadnovMGSelf-assembling viral mimetics: one long journey with short stepsMacromol Biosci201111450351321165940
- ChithraniBGhazaniAChanWDetermining the size and shape dependence of gold nanoparticle uptake into mammaliancellsNano Lett20066466266816608261
- JiangWKimBYRutkaJTChanWCNanoparticle-mediated cellular response is size-dependentNat Nanotechnol20083314515018654486
- UnfriedKAlbrechtCKlotzLVon MikeczAGrether-BeckSSchinsRCellular responses to nanoparticles: target structures and mechanismsNanotoxicology2007115271
- OberdörsterGSafety assessment for nanotechnology and nanomedicine: concepts ofnanotoxicologyJ Intern Med201026718910520059646
- El-AnsaryAAl-DaihanSOn the toxicity of therapeutically used nanoparticles: an overviewJ Toxicol2009200975481020130771
- WijnhovenSPeijnenburgWHerbertsCNano-silver – a review of available data and knowledge gaps in human andenvironmental risk assessmentNanotoxicology200932109138
- XuLZhaoJZhangTRenGYangZIn vitro study on influence of nanoparticles of CuO on CA1 pyramidal neurons of rathippocampus potassium currentsEnviron Toxicol200924321121718623077
- ZhaoJXuLZhangTRenGYangZInfluences of nanoparticle zinc oxide on acutely isolated rat hippocampal CA3pyramidal neuronsNeurotoxicology200930222023019146874
- LiuZRenGZhangTYangZAction potential changes associated with the inhibitory effects on voltage-gatedsodium current of hippocampal CA1 neurons by silver nanoparticlesToxicology2009264317918419683029
- HodgkinAHuxleyAA quantitative description of membrane current and its application to conduction andexcitation in nerveJ Physiol1952117450054412991237
- PriceKStornRLampinenJDifferential Evolution: A P ractical Approach to Global OptimizationHeidelberg, GermanySpringer-Verlag2005
- LlinásRRibaryUCoherent 40-Hz oscillation characterizes dream state in humansProc Natl Acad Sci U S A1993905207820818446632
- LlinásRRibaryUJoliotMWangXJContent and context in temporal thalamocortical bindingBuzsákiGChristenYTemporal Coding in the Brain (Research and Perspectives in Neurosciences)Heidelberg, GermanySpringer-Verlag1994252272
- LlinásRRibaryUContrerasDPedroarenaCThe neuronal basis for consciousnessPhilos Trans R Soc Lond B Biol Sci19983531377184118499854256
- LlinásRLeznikEUrbanoFJTemporal binding via cortical coincidence detection of specific and nonspecificthalamocortical inputs: a voltage-dependent dye-imaging study in mouse brain slicesProc Natl Acad Sci U S A200299144945411773628
- PellegrinoTMannaLKuderaSHydrophobic nanocrystals coated with an amphiphilic polymer shell: a general route towater soluble nanocrystalsNano Lett200444703707
- FenwickEMMartyANeherEA patch clamp study of bovine chromaffin cells and of their sensitivity toacetylcholineJ Physiol19823315775976296371
- KobayashiHShiraishiSYanagitaTRegulation of voltage-dependent sodium channel expression in adrenal chromaffincells: involvement of multiple calcium signaling pathwaysAnn N Y Acad Sci200297112713412438102
- TischlerASChromaffin cells as models of endocrine cells and neuronsAnn N Y Acad Sci200297136637012438154
- GoldinALBarchiRLCaldwellJHNomenclature of voltage-gated sodium channelsNeuron200028236536811144347
- CatterallWAGoldinALWaxmanSGInternational union of pharmacology. XLVII. Nomenclature and structure-functionrelationships of voltage-gated sodium channelsPharmacol Rev200557439740916382098
- GoldinALResurgence of sodium channel researchAnnu Rev Physiol20016387189411181979
- LorinczANusserZMolecular identity of dendritic voltage-gated sodium channelsScience2010328598090690920466935
- RoyeckMHorstmannMRemySReitzeMYaariYBeckHRole of axonal NaV1.6 sodium channels in action potential initiation of CA1 pyramidalneuronsJ Neurophysiol200810042361238018650312
- Toledo-AralJJMossBLHeZIdentification of PN1, a predominant voltage-dependent sodium channel expressedprincipally in peripheral neuronsProc Natl Acad Sci U S A1997944152715329037087
- MalmivuoJPlonseyRBioelectromagnetism: Principles and Applications of Bioelectric and BiomagneticFieldsNew YorkOxford University Press1995
- HamillOMartyANeherESakmannBSigworthFImproved patch clamp techniques for high-resolution current recording from cells andcell-free membrane patchesPflugers Arch19813912851006270629
- ShepherdGMThe Synaptic Organization of the Brain5th edOxford, New YorkOxford University Press2001
- JonesEGThalamic circuitry and thalamocortical synchronyPhilos Trans R Soc Lond B Biol Sci200235714281659167312626002
- SingerWNeuronal synchrony: a versatile code for the definition of relations?Neuron1999241496510677026
- HughesSWErringtonALörinczMLNovel modes of rhythmic burst firing at cognitively relevant frequencies inthalamocortical neuronsBrain Res20081235122018602904
- GrayCMSingerWStimulus-specific neuronal oscillations in orientation columns of cat visualcortexProc Natl Acad Sci U S A1989865169817022922407
- RibaryUIoannidesAASinghKDMagnetic field tomography of coherent thalamocortical 40-Hz oscillations inhumansProc Natl Acad Sci U S A1991882411037110411763020
- SingerWSynchronization of cortical activity and its putative role in information processingand learningAnnu Rev Physiol1993553493748466179
- GregoriouGGottsSZhouHDesimoneRHigh-frequency, longrange coupling between prefrontal and visual cortex duringattentionScience200932459311207121019478185
- SukJRibaryUCappellJYamamotoTLlinásRAnatomical localization revealed by MEG recordings of the human somatosensorysystemElectroencephalogr Clin Neurophysiol19917831851961707790
- GrayCMMcCormickDAChattering cells: superficial pyramidal neurons contributing to the generation ofsynchronous oscillations in the visual cortexScience199627452841091138810245
- SteriadeMTimofeevIDürmüllerNGrenierFDynamic properties of corticothalamic neurons and local cortical interneuronsgenerating fast rhythmic (30–40 Hz) spike burstsJ Neurophysiol19987914834909425218
- BrumbergJCNowakLGMcCormickDAIonic mechanisms underlying repetitive high-frequency burst firing in supragranularcortical neuronsJ Neurosci200020134829484310864940
- WangXJGolombDRinzelJEmergent spindle oscillations and intermittent burst firing in a thalamic model:specific neuronal mechanismsProc Natl Acad Sci U S A19959212557755817777551
- WangXJCalcium coding and adaptive temporal computation in cortical pyramidalneuronsJ Neurophysiol1998793154915669497431
- GolombDWangXJRinzelJPropagation of spindle waves in a thalamic slice modelJ Neurophysiol19967527507698714650
- GolombDShedmiACurtuRErmentroutGBPersistent synchronized bursting activity in cortical tissues With low-magnesiumconcentration: a modeling studyJ Neurophysiol20069521049106716236776
- WangXJBuzsákiGGamma oscillation by synaptic inhibition in a hippocampal interneuronal networkmodelJ Neurosci19961620640264138815919
- DestexheABalTMcCormickDASejnowskiTJIonic mechanisms underlying synchronized oscillations and propagating waves in amodel of ferret thalamic slicesJ Neurophysiol1996763204920708890314
- BazhenovMTimofeevISteriadeMSejnowskiTJCellular and network models for intrathalamic augmenting responses during 10-HzstimulationJ Neurophysiol1998795273027489582241
- GolombDAmitaiYPropagating neuronal discharges in neocortical slices: computational and experimentalstudyJ Neurophysiol1997783119912119310412
- DestexheAContrerasDSejnowskiTJSteriadeMA model of spindle rhythmicity in the isolated thalamic reticularnucleusJ Neurophysiol19947228038187527077
- DestexheAContrerasDSteriadeMSejnowskiTJHuguenardJRIn vivo, in vitro, and computational analysis of dendritic calcium currents inthalamic reticular neuronsJ Neurosci19961611691858613783
- DestexheAContrerasDSteriadeMMechanisms underlying the synchronizing action of corticothalamic feedback throughinhibition of thalamic relay cellsJ Neurophysiol199879299910169463458
- BazhenovMTimofeevISteriadeMSejnowskiTJModel of thalamo-cortical slow-wave sleep oscillations and transitions to activatedstatesJ Neurosci200222198691870412351744
- DestexheAMainenZFSejnowskiTJKinetic models of synaptic transmissionKochCSegevIMethods in Neuronal Modeling: From Ions to Networks (Computational Neuroscience)2nd edCambridgeThe MIT Press1998125
- WangXJRinzelJAlternating and synchronous rhythms in reciprocally inhibitory modelneuronsNeural Comput1992418497
- GolombDWangXJRinzelJSynchronization properties of spindle oscillations in a thalamic reticular nucleusmodelJ Neurophysiol1994723110911267807198
- DestexheAMainenZFSejnowskiTJAn efficient method for computing synaptic conductances based on a kinetic model ofreceptor bindingNeural Comput1994611418
- PraetoriusMBrunnerCLehnertTranssynaptic delivery of nanoparticles to the central auditory nervoussystemActa Otolaryngol2007127548649017453474
- LiuZRenGZhangTYangZThe inhibitory effects of nano-Ag on voltage-gated potassium currents of hippocampalCA1 neuronsEnviron Toxicol201126555255820549616