Abstract
Background
Although the potential of coronavirus disease 2019 (COVID-19) patients to develop pulmonary embolism (PE) is widely recognized, the underlying mechanism has not been completely elucidated. This study aimed to identify genes common to COVID-19 and PE to reveal the underlying pathogenesis of susceptibility to PE in COVID-19 patients.
Methods
COVID-19 genes were obtained from the GEO database and the OMIM, CTD, GeneCards, and DisGeNET databases; PE genes were obtained from the OMIM, CTD, GeneCards, and DisGeNET databases. We overlapped the genes of COVID-19 and PE to obtain common genes for additional analysis, including functional enrichment, protein–protein interaction, and immune infiltration analysis. Hub genes were identified using cytoHubba, a plugin of Cytoscape, and validated using the independent datasets GSE167000 and GSE13535. The genes validated by the above datasets were further validated in clinical samples.
Results
We obtained 36 genes shared by PE and COVID-19. Functional enrichment and immune infiltration analyses revealed the involvement of cytokines and immune activation. Five genes (CCL2, CXCL10, ALB, EGF, and MKI67) were identified as hub genes common to COVID-19 and PE. CXCL10 was validated in both independent datasets (GSE167000 and GSE13535). Serum levels of CXCL10 in the COVID-19 group and the COVID-19 combined with PE group were significantly higher than those in the healthy control group (P<0.001). In addition, there were significant differences between the COVID-19 group and the COVID-19 combined with PE group (P<0.01).
Conclusion
Our study reveals common genes shared by PE and COVID-19 and identifies CXCL10 as a possible cause of susceptibility to PE in COVID-19 patients.
Introduction
Coronavirus disease 2019 (COVID-19), caused by severe acute respiratory syndrome coronavirus 2 (SARS-CoV-2) infection, remains an acute infectious disease of global pandemic proportions and is a public health disease that critically endangers human health.Citation1,Citation2 COVID-19 patients have multiple complications, including pulmonary embolism (PE).Citation3 PE refers to the clinical and pathophysiological syndrome in which a thrombus occurs in the main trunk of the pulmonary artery or its branches, causing blockage and pulmonary circulation disorders.Citation4,Citation5 PE, with high incidence, missed diagnosis and mortality rates, is the third most common cause of cardiovascular death worldwide after stroke and heart attack.Citation6
Accumulating evidence suggests that inpatients with COVID-19 more frequently develop PE events than inpatients without COVID-19.Citation7–9 A meta-analysis including 3342 COVID-19 patients showed that the incidence of PE events in COVID-19 patients was 16.5%, considerably higher than that in patients without COVID-19.Citation10 After a meta-analysis of 13 postmortem studies of COVID-19 patients, Zuin et al concluded that the possibility of acute PE events in COVID-19 patients has been underestimated in clinical practice.Citation11 Recently, a large Swedish cohort study revealed that compared with the control period, the risk ratio for a first PE during days 1–30 after COVID-19 was 33.05, and the increased risk lasted for six months after COVID-19.Citation12 Early in the COVID-19 epidemic, German researchers performed autopsies on 12 patients who died from the disease and found PE to be the direct cause of death in four of them (1/3).Citation13 Furthermore, compared with the PE patients without COVID-19 infection, PE patients with COVID-19 had an increased risk of in-hospital mortality, septic shock, respiratory failure, and a longer hospital stay.Citation14
PE is widely believed to be one of the forms of venous thrombosis. However, the idea that PE is the result of the migration of blood clots from the venous system is questioned because no initial thrombus is found in many patients.Citation15–17 Histologic analysis of pulmonary vessels in patients with COVID-19 shows widespread thrombosis with microangiopathy,Citation18 and in situ immunothrombosis plays a role in the pathophysiology of COVID-19-associated PE.Citation19,Citation20 This perspective is supported by Katsoularis’ study in which risk ratios during days 1–30 after COVID-19 were 33.05, significantly exceeding that for deep-vein thrombosis (4.98).Citation12 In one study, traditional risk factors for venous thromboembolic disease were not associated with the occurrence of PE in COVID-19 patients.Citation21 In addition, prophylactic anticoagulation did not prevent the occurrence of PE in hospitalized patients.Citation22 Therefore, it is necessary to specifically study COVID-19-associated PE rather than treat it as a form of venous thrombosis.
Currently, research on COVID-19-associated PE is dominated by observational studies of incidence, prognosis, and imaging characteristics, whereas few studies have been conducted to investigate the mechanisms of COVID-19-associated PE. Bioinformatics analysis provides a means to explore the genetic relationship between two diseases. Previous studies have indicated that SARS-CoV-2 infection can cause a myriad of complications because there are common genes between COVID-19 and these complications.Citation23–26 Therefore, this study aimed to explore the possible genetic relationship between COVID-19 and PE through bioinformatics to reveal the underlying pathogenesis of susceptibility to PE events in COVID-19 patients.
In this study, we used COVID-19 transcriptome data from the GEO (https://www.ncbi.nlm.nih.gov/geo/) database and COVID-19-related genes and PE-related genes from disease-related databases to perform bioinformatics analyses and then validated common key genes between COVID-19 and PE. This is the first genomics study of COVID-19 and PE.
Materials and Methods
Collection of COVID-19 and PE Genes
By searching the OMIM (http://omim.org/), CTD (http://ctdbase.org/), GeneCards (https://www.genecards.org/), and DisGeNET (https://www.disgenet.org/) databases, we collected genes related to COVID-19 and PE. In addition, microarray expression data of COVID-19 patients were obtained from the GEO database. GSE157103 was selected, which consisted of 126 samples, including 100 patients with COVID-19 and 26 patients without COVID-19, consisting of both ICU and non-ICU patients. Unfortunately, as no human data are available in the GEO database, we only obtained PE genes through the four disease-related databases mentioned above.
We collected the top 500 genes in each database according to each database scoring rule. If there were fewer than 500 genes in the database, all of them were included. The GSE157103 dataset was analyzed using the DESeq2 package, and an adjusted p-value (false discovery rate) <0.05 and∣log2FoldChange∣>1 were used as the screening criteria for important differentially expressed genes (DEGs).
Subsequently, we obtained COVID-19 genes by taking the intersection of the COVID-19-related genes in the disease-related database and the DEGs of GSE157103. We then overlapped the genes of COVID-19 and PE to obtain common genes for further analysis. We accessed these websites on 4 March 2022.
Gene Enrichment Analysis
Gene Ontology (GO) and Kyoto Encyclopedia of Genes and Genomes (KEGG) pathway enrichment analyses were used to investigate biological activities and pathways. To understand the functional characteristics of the common genes of COVID-19 and PE, we executed GO and KEGG enrichment analyses using R’s cluster profile package, with a p-value <0.05 as the cutoff.
Protein–Protein Interaction (PPI) Network Analysis and Screening for Common Hub Genes
We constructed a PPI network based on the STRING database (https://string-db.org/), with a medium confidence score of > 0.4, and the PPI network was visualized via Cytoscape (Version 3.9.1).Citation27 Furthermore, we identified hub genes through five algorithms (BottleNeck, Closeness, Radiality, Betweenness, Stress) of the cytoHubba plugin of Cytoscape. Common hub genes of COVID-19 and PE were obtained by taking the intersection of the top 10 genes in each of the five algorithms.
Immune Infiltration Analysis and Correlations Between Hub Genes and Immune Cell Infiltration
Immune infiltration analysis was performed via the CIBERSORTx tool,Citation28 a deconvolution algorithm that evaluates the expression of related genes based on gene expression, to calculate the ratio of COVID-19 samples. Correlations between each immune cell and hub genes were calculated using the Sangerbox online tool.Citation29
Validation of Hub Genes in External Datasets
To reduce the possibility of false positives, we validated hub genes separately using other COVID-19 and PE datasets from the GEO database. For COVID-19, we selected GSE167000 for validation, which includes 65 SARS-CoV-2-positive patients and 30 SARS-CoV-2-negative patients. Additionally, we used the rat PE model dataset GSE13535 for PE. GSE13535 includes 6 control rats and 16 PE model rats. Models with mild PE were established at 2 hours after modeling and models with severe PE were established at 18 hours later. Expression data for the hub genes were obtained using the GEOquery package.
Verification of Genes by Clinical Samples
To further verify the genes validated above, we collected 6 blood samples from COVID-19 combined with PE inpatients. COVID-19 was determined by a positive polymerase chain reaction test for SARS-CoV-2 and PE was diagnosed by computed tomography pulmonary angiography. In addition, we collected 6 blood samples from COVID-19-alone inpatients and 4 healthy individuals who were negative for anti-SARS-CoV-2 IgM/IgG. The COVID-19-alone patients and the healthy controls were matched with the COVID-19 combined with PE patients for age and sex. Blood samples were obtained from Henan Provincial People’s Hospital (Henan, China) and centrifuged at 1000×g for 10 min. Levels of CXCL10 in serum were measured using a human ELISA kit (Elabscience, Wuhan, China). This study was approved by the ethics committee of Henan Provincial People’s Hospital (Ethical Review 2023(97)), and written informed consent from all participants was provided for the use of their blood samples.
Statistical Analysis
GraphPad Prism version 8.0.2 was used to analyze the data. The results are displayed as the mean ± SD. Differences between the two groups were compared by an unpaired Student′s t-test. A two-tailed p-value < 0.05 was considered statistically significant.
Results
Collection of COVID-19 and PE Genes
We obtained 880 DEGs from GSE157103 and 500, 500, 500 and 3 COVID-19-related genes from the GeneCards, DisGeNET, CTD and OMIM databases, respectively. Seventy-two COVID-19 genes were obtained by merging and deduplicating the results collected from four databases and taking the intersection with DEGs from GSE157103. Similarly, after merging and deduplicating 500, 93, 500, and 207 PE-related genes from the GeneCards, DisGeNET, CTD, and OMIM databases, respectively, we obtained 1069 PE genes. By taking the intersection of 72 COVID-19 genes with 1069 PE genes, we obtained 36 genes common to both COVID-19 and PE. The screening procedure and results are shown in and .
Table 1 Collection of COVID-19 and PE Genes
Gene Enrichment Analysis
The top 10 GO terms and KEGG pathways are summarized in . GO annotation includes three terms: biological process, cell component, and molecular function. For biological processes, the common genes were enriched in response to chemical and cytokine-mediated signaling pathways (). Cell component items were mainly enriched in the endomembrane system and protein-containing complex (). The molecular function category was mainly enriched in anion binding and carbohydrate derivative binding (). KEGG pathway enrichment analysis included focal adhesion, the cell cycle, and the p53 signaling pathway ().
Figure 2 GO and KEGG pathway enrichment analyses of 36 common genes between COVID-19 and PE. (A) Biological process of GO. (B) Molecular function of GO. (C) Cellular component of GO. (D) KEGG pathway.
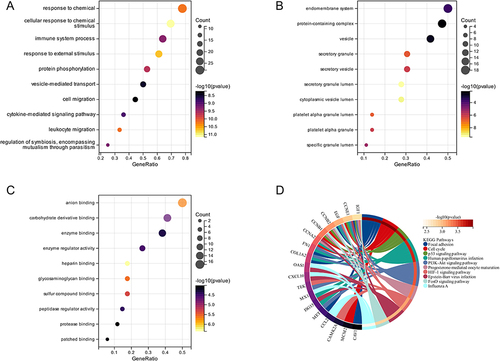
PPI Network Analysis and Screening of Common Hub Genes
To explore protein interactions, we constructed a PPI network of 36 common genes using the STRING database. There were a total of 36 nodes and 152 edges in the network, which was visualized by Cytoscape (). We screened the top 10 hub genes with five algorithms from the cytoHubba plugin. Five hub genes (CCL2, CXCL10, ALB, EGF, and MKI67) were obtained by taking the intersection of the top 10 genes in the five algorithms (). CCL2, CXCL10, EGF, and MKI67 were upregulated in GSE157103; ALB, encoding the most abundant protein in human blood, was downregulated ().
Table 2 Hub Genes
Immune Infiltration Analysis and Correlations Between Hub Genes and Immune Cells
There is evidence that immune cell infiltration is involved in the development of COVID-19.Citation30 The CIBERSORTx algorithm has been applied to investigate the landscape of immune infiltration in COVID-19. The proportions of 22 immune cell types in COVID-19 are shown in . Specifically, the types of immune cells in COVID-19 were B cells, plasma cells, naive CD4+ T cells, activated memory CD4+ T cells, regulatory T cells, monocytes, and resting mast cells. In addition, we analyzed the correlation between each immune cell and the 5 hub genes. As shown in , CCL2 expression was associated with resting memory CD4+ T cells, gamma delta T cells, and neutrophils; CXCL10 correlated with activated memory CD4+ T cells and activated dendritic cells; ALB correlated with naive CD4+ T cells; EGF correlated with activated memory CD4+ T cells and Tregs; and MKI67 correlated with plasma cells and activated memory CD4+ T cells. In summary, these results suggest that CCL2, CXCL10, ALB, EGF, and MKI67 may contribute to the immune microenvironment of COVID-19.
Validation of Hub Genes in External Datasets
To validate the reliability of the five hub genes, we first verified the expression of these genes in the GSE167000 dataset. As shown in , CXCL10, EGF, and MKI67 in this dataset were significantly upregulated in the SARS-CoV-2-positive group compared with the SARS-CoV-2- negative group. In the GSE13535 dataset, the expression levels of CCL2 and CXCL10 were significantly increased in the PE model group compared with the control group, both at 2 and 18 hours after modeling. The results are shown in and . In summary, only CXCL10 was discrepant in both external datasets. Therefore, we consider CXCL10 to be the most likely key gene involved in COVID-19 and PE.
Figure 5 Validation of the hub genes in the external datasets. (A) The expression of CCL2, CXCL10, ALB, EGF, and MKI67 in the COVID-19 dataset (GSE16700). (B) The expression of CCL2, CXCL10, ALB, EGF and MKI67 in the PE dataset (GSE13535) at 2 hours after modeling. (C) The expression of CCL2, CXCL10, ALB, EGF and MKI67 in the PE dataset (GSE13535) at 18 hours after modeling.
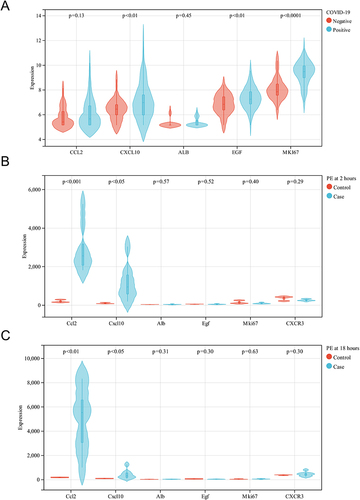
Verification of Serum CXCL10 Levels in Human Samples
To verify whether CXCL10 is a key gene involved in COVID-19 associated with PE, we validated CXCL10 with blood samples. The protein encoded by CXCL10 is a secreted protein, also known as interferon-inducible protein 10 (IP-10), which belongs to the CXC chemokine family of chemokines and is present in serum and plasma. We measured serum levels of CXCL10 in 4 healthy controls, 6 COVID-19 patients, and 6 COVID-19 patients with PE. Levels of CXCL10 in the COVID-19 and the COVID-19 combined with PE groups were significantly higher than those in the healthy control group (p <0.001). In addition, there were significant differences between the COVID-19 group and the COVID-19 combined with PE group (p <0.01), as shown in .
Discussion
During the COVID-19 pandemic, several studies have described an increased risk of developing PE, which contributes to a significant increase in mortality among COVID-19 patients. However, the underlying pathogenesis of susceptibility to PE in COVID-19 is not yet fully understood. In this study, we first performed a genomics analysis of COVID-19 and PE to reveal genetic interrelations between the two diseases.
We identified 36 common genes shared in COVID-19 and PE. In a sense, these common genes suggest that there are relationships between COVID-19 and PE. Enrichment analysis showed that these genes were involved in cytokine-mediated signaling pathways, and immune infiltration analysis indicated that immune activation was involved in COVID-19. SARS-CoV-2 can continuously activate the body’s natural immune system and, as a result, cause the release of inflammatory cytokines.Citation30,Citation31 Cytokines play critical roles in the pathogenesis of COVID-19.Citation32–34 In particular, uncontrolled cytokines could lead to a systemic inflammatory response syndrome and release “messenger substances”, which cause thrombosis and blood vessel blockage.Citation13 This is consistent with clinical studies in which histologic analysis of the lung in patients with COVID-19 showed infiltration of multiple immune cells and widespread microthrombosis.Citation18,Citation35,Citation36 Inflammation may be a major contributor to PE in the context of COVID-19.
We then performed a comprehensive analysis to construct the PPI network, and a strong genetic, protein-based relationship was found among common genes shared in COVID-19 and PE. CCL2, CXCL10, ALB, EGF, and MKI67 were identified as hub genes, with CXCL10 validated by external datasets and human samples. These results suggest that CXCL10 is a key gene common to both COVID-19 and PE and that it may be responsible for susceptibility to PE in COVID-19 patients.
CXCL10, also termed IP-10, is secreted after interferon-gamma production by a wide variety of cell types, such as endothelial cells, fibroblasts, monocytes, and T lymphocytes.Citation37 CXCL10 is a key regulator of the “cytokine storms” caused by SARS-CoV-2 infectionCitation38 and tends to be elevated earlier in COVID-19 patients than other inflammatory cytokines.Citation39 There is sufficient evidence that in COVID-19 patients, CXCL10 levels are significantly elevated and associated with adverse clinical outcomes.Citation40–42 Consistent with these findings, our study showed elevated levels of CXCL10 in the COVID-19 group with or without PE compared with healthy controls.
The role of CXCL10 in atherosclerosis and myocardial infarction has been extensively described.Citation43,Citation44 However, it is not clear whether CXCL10 plays a role in the development of PE. Studies have shown that CXCL10 induces not only chemoattraction of inflammatory cells but also migration and proliferation of endothelial cells and vascular smooth muscle cells.Citation45,Citation46 CXCR3, the receptor for CXCL10, has been reported to be expressed in pulmonary artery endothelial cells (PAECs), and CXCL10 can lead to PAEC dysfunction.Citation47 Improved endothelial healing prevents arterial thrombosis, but CXCL10 can inhibit endothelial healing.Citation48 Therefore, CXCL10 may be involved in the formation of arterial thrombosis. In our study, the CXCL10 levels in the COVID-19 combined with PE group were significantly higher than those in the COVID-19 group.
In summary, we hypothesize that CXCL10, as an early upregulated inflammatory factor in COVID-19, plays a dual role by recruiting diverse inflammatory cells to infiltrate and release “messenger substances”, promoting thrombosis, while also potentially causing dysfunction of PAECs and contributing to pulmonary thrombosis in COVID-19 patients. In the future, we will focus on elucidating the underlying mechanisms by which CXCL10 may contribute to the development of pulmonary artery thrombosis in COVID-19 patients.
Limitations of the Study
The study has several limitations, which must be acknowledged. Given the paucity of available human datasets for PE, we used the dataset from rats to validate hub genes. However, CXCL10 has also been validated in clinical samples. In addition, limited clinical samples were available for this study, and we only compared CXCL10 levels in 4 healthy controls, 6 COVID-19 patients, and 6 COVID-19 patients with PE. We will further validate our conclusions by expanding the sample size.
Conclusion
In this study, we obtained 36 common genes, established a coexpression network between COVID-19 and PE, and identified and validated CXCL10 as a common key gene in both diseases that may be responsible for susceptibility to PE events in COVID-19 patients. Consequently, our research first describes the possible genetic relationship between COVID-19 and PE to further reveal the mechanisms of COVID-19-associated PE.
Abbreviations
COVID-19, coronavirus disease 2019; SARS-CoV-2, severe acute respiratory syndrome coronavirus 2; PE, pulmonary embolism; DEGs, differentially expressed genes; GO, Gene Ontology; KEGG, Kyoto Encyclopedia of Genes and Genomes; PPI, protein–protein interaction; PAECs, pulmonary artery endothelial cells.
Ethical Approval and Consent to Participate
This study was performed in accordance with the Helsinki declaration and was approved by the ethics committee of Henan Provincial People’s Hospital (Ethical Review 2023(97)), and written informed consent from all participants was provided for the use of their blood samples.
Disclosure
The authors declare that they have no conflicts of interest.
Acknowledgments
We sincerely thank the public databases GEO, OMIM, CTD, GeneCards, DisGeNET, and STRING for providing data for our research and Cytoscape, CIBERSORTx, and Sangerbox for analyzing data.
Data Sharing Statement
We provide details of the materials and methods in our manuscript.
Additional information
Funding
References
- Yuan Y, Jiao B, Qu L, et al. The development of COVID-19 treatment. Front Immunol. 2023;14:1125246. doi:10.3389/fimmu.2023.1125246
- Zhang JJ, Dong X, Liu GH, et al. Risk and protective factors for COVID-19 morbidity, severity, and mortality. Clin Rev Allergy Immunol. 2023;64(1):90–107. doi:10.1007/s12016-022-08921-5
- Dou Q, Wei X, Zhou K, et al. Cardiovascular manifestations and mechanisms in patients with COVID-19. Trends Endocrinol Metab. 2020;31(12):893–904. doi:10.1016/j.tem.2020.10.001
- Agnelli G, Becattini C. Anticoagulant treatment for acute pulmonary embolism: a pathophysiology-based clinical approach. Eur Respir J. 2015;45(4):1142–1149. doi:10.1183/09031936.00164714
- Essien EO, Rali P, Mathai SC. Pulmonary Embolism. Med Clin North Am. 2019;103(3):549–564. doi:10.1016/j.mcna.2018.12.013
- Konstantinides SV, Meyer G, Becattini C, et al. 2019 ESC Guidelines for the diagnosis and management of acute pulmonary embolism developed in collaboration with the European Respiratory Society (ERS). Eur Heart J. 2020;41(4):543–603. doi:10.1093/eurheartj/ehz405
- Poissy J, Goutay J, Caplan M, et al. Pulmonary embolism in patients with COVID-19: awareness of an increased prevalence. Circulation. 2020;142(2):184–186. doi:10.1161/CIRCULATIONAHA.120.047430
- Helms J, Tacquard C, Severac F, et al. High risk of thrombosis in patients with severe SARS-CoV-2 infection: a multicenter prospective cohort study. Intensive Care Med. 2020;46(6):1089–1098. doi:10.1007/s00134-020-06062-x
- Piroth L, Cottenet J, Mariet AS, et al. Comparison of the characteristics, morbidity, and mortality of COVID-19 and seasonal influenza: a nationwide, population-based retrospective cohort study. Lancet Respir Med. 2021;9(3):251–259. doi:10.1016/S2213-2600(20)30527-0
- Suh YJ, Hong H, Ohana M, et al. Pulmonary embolism and deep vein thrombosis in COVID-19: a systematic review and meta-analysis. Radiology. 2021;298(2):E70–E80. doi:10.1148/radiol.2020203557
- Zuin M, Engelen MM, Bilato C, et al. Prevalence of acute pulmonary embolism at autopsy in patients with COVID-19. Am J Cardiol. 2022;171:159–164. doi:10.1016/j.amjcard.2022.01.051
- Katsoularis I, Fonseca-Rodriguez O, Farrington P, et al. Risks of deep vein thrombosis, pulmonary embolism, and bleeding after covid-19: nationwide self-controlled cases series and matched cohort study. BMJ. 2022;377:e069590. doi:10.1136/bmj-2021-069590
- Wichmann D, Sperhake JP, Lutgehetmann M, et al. Autopsy findings and venous thromboembolism in patients with COVID-19: a Prospective Cohort Study. Ann Intern Med. 2020;173(4):268–277. doi:10.7326/M20-2003
- Safiriyu I, Fatuyi M, Mehta A, et al. Impact of COVID-19 infection on the clinical outcomes of pulmonary embolism hospitalizations: a nationwide analysis. Curr Probl Cardiol. 2023;48(7):101669. doi:10.1016/j.cpcardiol.2023.101669
- Tadlock MD, Chouliaras K, Kennedy M, et al. The origin of fatal pulmonary emboli: a postmortem analysis of 500 deaths from pulmonary embolism in trauma, surgical, and medical patients. Am J Surg. 2015;209(6):959–968. doi:10.1016/j.amjsurg.2014.09.027
- Ahuja J, Shroff GS, Benveniste MF, et al. In situ pulmonary artery thrombosis: unrecognized complication of radiation therapy. AJR Am J Roentgenol. 2020;215(6):1329–1334. doi:10.2214/AJR.19.22741
- Cao Y, Geng C, Li Y, et al. In situ pulmonary artery thrombosis: a previously overlooked disease. Front Pharmacol. 2021;12:671589. doi:10.3389/fphar.2021.671589
- Ackermann M, Verleden SE, Kuehnel M, et al. Pulmonary vascular endothelialitis, thrombosis, and angiogenesis in Covid-19. N Engl J Med. 2020;383(2):120–128. doi:10.1056/NEJMoa2015432
- Poor HD. Pulmonary thrombosis and thromboembolism in COVID-19. Chest. 2021;160(4):1471–1480. doi:10.1016/j.chest.2021.06.016
- Quartuccio L, Sonaglia A, Casarotto L, et al. Clinical, laboratory and immunohistochemical characterization of in situ pulmonary arterial thrombosis in fatal COVID-19. Thromb Res. 2022;219:95–101. doi:10.1016/j.thromres.2022.09.012
- Fauvel C, Weizman O, Trimaille A, et al. Pulmonary embolism in COVID-19 patients: a French multicentre cohort study. Eur Heart J. 2020;41(32):3058–3068. doi:10.1093/eurheartj/ehaa500
- Bompard F, Monnier H, Saab I, et al. Pulmonary embolism in patients with COVID-19 pneumonia. Eur Respir J. 2020;56(1). doi:10.1183/13993003.01365-2020
- Chen Z, Chen C, Chen F, et al. Bioinformatics analysis of potential pathogenesis and risk genes of immunoinflammation-promoted renal injury in severe COVID-19. Front Immunol. 2022;13:950076. doi:10.3389/fimmu.2022.950076
- Li Y, Liu Y, Duo M, et al. Bioinformatic analysis and preliminary validation of potential therapeutic targets for COVID-19 infection in asthma patients. Cell Commun Signal. 2022;20(1):201. doi:10.1186/s12964-022-01010-2
- Lv Y, Zhang T, Cai J, et al. Bioinformatics and systems biology approach to identify the pathogenetic link of Long COVID and Myalgic Encephalomyelitis/Chronic Fatigue Syndrome. Front Immunol. 2022;13:952987. doi:10.3389/fimmu.2022.952987
- Zhang W, Di L, Liu Z, et al. TIMELESS is a key gene mediating thrombogenesis in COVID-19 and antiphospholipid syndrome. Sci Rep. 2022;12(1):17248. doi:10.1038/s41598-022-21694-3
- Shannon P, Markiel A, Ozier O, et al. Cytoscape: a software environment for integrated models of biomolecular interaction networks. Genome Res. 2003;13(11):2498–2504. doi:10.1101/gr.1239303
- Chen B, Khodadoust MS, Liu CL, et al. Profiling tumor infiltrating immune cells with CIBERSORT. Methods Mol Biol. 2018;1711:243–259. doi:10.1007/978-1-4939-7493-1_12
- Shen W, Song Z, Zhong X, et al. Sangerbox: a comprehensive, interaction-friendly clinical bioinformatics analysis platform. iMeta. 2022;1(3):e36. doi:10.1002/imt2.36
- Mehta P, McAuley DF, Brown M, et al. COVID-19: consider cytokine storm syndromes and immunosuppression. Lancet. 2020;395(10229):1033–1034. doi:10.1016/S0140-6736(20)30628-0
- Ramasamy S, Subbian S. Critical determinants of cytokine storm and Type I interferon response in COVID-19 pathogenesis. Clin Microbiol Rev. 2021;34(3). doi:10.1128/CMR.00299-20
- Coperchini F, Chiovato L, Ricci G, et al. The cytokine storm in COVID-19: further advances in our understanding the role of specific chemokines involved. Cytokine Growth Factor Rev. 2021;58:82–91. doi:10.1016/j.cytogfr.2020.12.005
- Costela-Ruiz VJ, Illescas-Montes R, Puerta-Puerta JM, et al. SARS-CoV-2 infection: the role of cytokines in COVID-19 disease. Cytokine Growth Factor Rev. 2020;54:62–75. doi:10.1016/j.cytogfr.2020.06.001
- Hsu RJ, Yu WC, Peng GR, et al. The role of cytokines and chemokines in severe acute respiratory syndrome Coronavirus 2 infections. Front Immunol. 2022;13:832394. doi:10.3389/fimmu.2022.832394
- Wang S, Yao X, Ma S, et al. A single-cell transcriptomic landscape of the lungs of patients with COVID-19. Nat Cell Biol. 2021;23(12):1314–1328. doi:10.1038/s41556-021-00796-6
- Khismatullin RR, Ponomareva AA, Nagaswami C, et al. Pathology of lung-specific thrombosis and inflammation in COVID-19. J Thromb Haemost. 2021;19(12):3062–3072. doi:10.1111/jth.15532
- Luster AD, Ravetch JV. Biochemical characterization of a gamma interferon-inducible cytokine (IP-10). J Exp Med. 1987;166(4):1084–1097. doi:10.1084/jem.166.4.1084
- Zhang N, Zhao YD, Wang XM. CXCL10 an important chemokine associated with cytokine storm in COVID-19 infected patients. Eur Rev Med Pharmacol Sci. 2020;24(13):7497–7505. doi:10.26355/eurrev_202007_21922
- Lu Q, Zhu Z, Tan C, et al. Changes of serum IL-10, IL-1beta, IL-6, MCP-1, TNF-alpha, IP-10 and IL-4 in COVID-19 patients. Int J Clin Pract. 2021;75(9):e14462. doi:10.1111/ijcp.14462
- Gudowska-Sawczuk M, Mroczko B. What is currently known about the role of CXCL10 in SARS-CoV-2 infection? Int J Mol Sci. 2022;23(7). doi:10.3390/ijms23073673
- Chen Y, Wang J, Liu C, et al. IP-10 and MCP-1 as biomarkers associated with disease severity of COVID-19. Mol Med. 2020;26(1):97. doi:10.1186/s10020-020-00230-x
- Kalinina O, Golovkin A, Zaikova E, et al. Cytokine storm signature in patients with moderate and severe COVID-19. Int J Mol Sci. 2022;23(16). doi:10.3390/ijms23168879
- Heller EA, Liu E, Tager AM, et al. Chemokine CXCL10 promotes atherogenesis by modulating the local balance of effector and regulatory T cells. Circulation. 2006;113(19):2301–2312. doi:10.1161/CIRCULATIONAHA.105.605121
- Koten K, Hirohata S, Miyoshi T, et al. Serum interferon-gamma-inducible protein 10 level was increased in myocardial infarction patients, and negatively correlated with infarct size. Clin Biochem. 2008;41(1–2):30–37. doi:10.1016/j.clinbiochem.2007.10.001
- Wang X, Yue TL, Ohlstein EH, Sung CP, Feuerstein GZ. Interferon-inducible protein-10 involves vascular smooth muscle cell migration, proliferation, and inflammatory response. J Biol Chem. 1996;271(39):24286–24293. doi:10.1074/jbc.271.39.24286
- Taub DD, Lloyd AR, Conlon K, et al. Recombinant human interferon-inducible protein 10 is a chemoattractant for human monocytes and T lymphocytes and promotes T cell adhesion to endothelial cells. J Exp Med. 1993;177(6):1809–1814. doi:10.1084/jem.177.6.1809
- Zabini D, Nagaraj C, Stacher E, et al. Angiostatic factors in the pulmonary endarterectomy material from chronic thromboembolic pulmonary hypertension patients cause endothelial dysfunction. PLoS One. 2012;7(8):e43793. doi:10.1371/journal.pone.0043793
- Lupieri A, Smirnova NF, Solinhac R, et al. Smooth muscle cells-derived CXCL10 prevents endothelial healing through PI3Kgamma-dependent T cells response. Cardiovasc Res. 2020;116(2):438–449. doi:10.1093/cvr/cvz122