Abstract
Purpose
Inflammatory biomarkers associated with peripheral artery disease (PAD) have been examined separately; however, an algorithm that includes a panel of inflammatory proteins to inform prognosis of PAD could improve predictive accuracy. We developed predictive models for 2-year PAD-related major adverse limb events (MALE) using clinical/inflammatory biomarker data.
Methods
We conducted a prognostic study using 2 phases (discovery/validation models). The discovery cohort included 100 PAD patients that were propensity-score matched to 100 non-PAD patients. The validation cohort included 365 patients with PAD and 144 patients without PAD (non-matched). Plasma concentrations of 29 inflammatory proteins were determined at recruitment and the cohorts were followed for 2 years. The outcome of interest was 2-year MALE (composite of major amputation, vascular intervention, or acute limb ischemia). A random forest model was trained with 10-fold cross-validation to predict 2-year MALE using the following input features: 1) clinical characteristics, 2) inflammatory biomarkers that were expressed differentially in PAD vs non-PAD patients, and 3) clinical characteristics and inflammatory biomarkers.
Results
The model discovery cohort was well-matched on age, sex, and comorbidities. Of the 29 proteins tested, 5 were elevated in PAD vs non-PAD patients (MMP-7, MMP-10, IL-6, CCL2/MCP-1, and TFPI). For prognosis of 2-year MALE on the validation cohort, our model achieved AUROC 0.63 using clinical features alone and adding inflammatory biomarker levels improved performance to AUROC 0.84.
Conclusion
Using clinical characteristics and inflammatory biomarker data, we developed an accurate predictive model for PAD prognosis.
Plain Language Summary
Inflammatory biomarkers associated with peripheral artery disease (PAD) have been examined separately; however, an algorithm that includes an inflammatory protein panel to inform prognosis of PAD may improve predictive accuracy. We developed predictive models for 2-year major adverse limb events (MALE) using clinical characteristics (demographics, comorbidities, and medications) and a panel of 5 PAD-specific inflammatory biomarkers (MMP-7, MMP-10, IL-6, CCL2/MCP-1, and TFPI) that achieved excellent performance on an independent validation cohort (AUROC 0.84). The models developed through this study may support PAD risk-stratification and targeted management strategies.
Introduction
Over 200 million individuals worldwide are impacted by peripheral artery disease (PAD), which involves atherosclerosis and thrombosis of the arteries in the lower limbs.Citation1,Citation2 Despite its important contribution to limb loss and mortality, PAD is poorly treated.Citation3 A key factor contributing to this issue includes the absence of standardized prognostic methods that can risk-stratify patients and guide their subsequent evaluation and treatment.
Inflammatory proteins have been demonstrated to contribute to PAD progression, including IL-6 (interleukin-6),Citation4 MMP-7 (matrix metalloproteinase-7),Citation5 MMP-10 (matrix metalloproteinase-10),Citation6 CCL-2/MCP-1 (monocyte chemoattractant protein),Citation7 and TFPI (tissue factor pathway inhibitor).Citation8 Indeed, over 20 inflammatory biomarkers for cardiovascular diseases have been studied.Citation9–12 We analyzed 29 specific inflammatory proteins because they show the strongest correlations with cardiovascular diseases with potential applications to PAD.Citation9–12 Although previous work has shown associations between these proteins and PAD, few have assessed their prognostic potential by measuring discriminatory and predictive metrics.Citation4–8 Additionally, these proteins have primarily been investigated individually, with no prior exploration of the prognostic potential of a combined panel of these inflammatory markers. Since PAD is a chronic, multifactorial condition with multiple metabolic pathways contributing to disease development,Citation13 we hypothesize that an integrated panel of biomarkers in conjunction with clinical characteristics may attain improved accuracy in PAD prognosis compared to assessing single proteins alone. By leveraging inflammatory biomarker data alongside clinical features correlated with PAD outcomes,Citation14–16 there exists potential to build highly accurate prognostic models for PAD. This work combines clinical and inflammatory biomarker data using predictive modelling techniques to support PAD prognosis and guide clinical decision-making. Importantly, we link biochemical and clinical data to support risk prediction, an aspect that has received limited investigation in PAD. This study has potential to support precision medicine by guiding clinical decision-making and tailoring optimal health care decisions around the individual characteristics of patients including their biomarker profile which can give useful information about disease susceptibility, evolution, and potential response to treatment.Citation17
Materials and Methods
Ethics
The Unity Health Toronto Research Ethics Board approved this study. All patients provided written informed consent and all procedures were conducted according to the principles outlined in the Declaration of Helsinki.Citation18
Design
This prognostic study was conducted using a propensity-matched model discovery cohort and unmatched, real-world validation cohort. Findings were reported using the Transparent Reporting of a multivariable Prediction model for Individual Prognosis or Diagnosis (TRIPOD) statement.Citation19
Patient Recruitment
This study prospectively recruited PAD and non-PAD patients who presented to ambulatory clinics at our institution from May 2018 to March 2021. The definition for PAD was ankle brachial index (ABI) below 0.9 or toe brachial index (TBI) below 0.67 and absent/diminished pedal pulses.Citation20 The definition for non-PAD was ABI ≥ 0.9 and TBI ≥ 0.67 and normal pedal pulses.Citation20 Patients with elevated troponin, acute coronary syndrome, acute limb ischemia, or receiving biological anti-inflammatory medications, within the previous 3 months were excluded. The PAD cohort only included patients who were asymptomatic or had claudication. Patients with chronic limb threatening ischemia (defined as rest pain or tissue loss) were excluded as they would have met the primary endpoint of requiring vascular intervention or major amputation at recruitment.
Baseline Characteristics
Baseline characteristics encompassed sex, age, hypertension (defined as diastolic blood pressure ≥ 80 mmHg, systolic blood pressure ≥ 130 mmHg, or utilization of blood pressure lowering therapyCitation21,Citation22), dyslipidemia (indicated by triglyceride levels > 1.7 mmol/L, total cholesterol levels > 5.2 mmol/L, or usage of lipid lowering therapyCitation21,Citation22), diabetes (defined as hemoglobin A1c ≥ 6.5% or use of antidiabetic medicationCitation21,Citation22), current or past smoking status, presence of congestive heart failure (CHF), coronary artery disease (CAD), history of stroke, occurrence of leg pain (self-reported and inclusive of both ischemic and non-ischemic pain), and utilization of cardiovascular risk reduction medications.Citation23 Definitions for cardiovascular risk factors were in accordance with guidelines established by the American College of Cardiology.Citation21,Citation22
Quantification of Plasma Inflammatory Biomarker Levels
Samples of blood were obtained from participants and concentrations of 29 inflammatory proteins in plasma were assessed in duplicate using the LUMINEX assay (Bio-Techne, Minneapolis, United States).Citation24 These proteins were selected because of their involvement in metabolic processes associated with atherosclerosis and important associations with cardiovascular diseases: chemokine (C-C motif) ligand 1 (CCL1)/TCA-3, tumor necrosis factor alpha (TNF-α), MMP-8, cluster of differentiation 163 (CD163), bone morphogenetic protein 10 (BMP-10), BMP-7, BMP-4, CCL3/macrophage inflammatory protein-1 alpha (MIP-1a), CCL13/MIP-1 delta, CCL4/MIP-1b, chemokine (C-X-C motif) ligand 16 (CXCL16), insulin-like growth factor-binding protein-1 (IGFBP-1), oxteoactivin/glycoprotein (transmembrane) NMB (GPNMB) resistin, CXCL9/monokine induced by gamma (MIG), regenerating family member 3 alpha (Reg3α), carcinoembryonic antigen-related cell adhesion molecule 1 (CEACAM1/CD66a), interferon gamma (IFNy), proganulin (PGRN), CCL17/thymus and activation regulated chemokine (TARC), HTRA2/Omi, Serpin A12, Serpin B3/squamous cell carcinoma antigen 1 (SCCA1), CCL11/Eotaxin, IL-6, MMP-7, MMP-10, CCL-2/MCP-1, and TFPI. By analyzing a large number of inflammatory proteins, we aim to identify novel PAD biomarkers. Before analyzing the samples using the MagPix analyzer,Citation25 it was calibrated with Fluidics Verification and Calibration bead kits (Luminex Corp, Texas, United States).Citation26 To reduce intra- and inter-assay variability, all analyses were conducted within 24 hours. Sample inter- and intra-assay coefficients of variability were below 10%. A minimum of fifty beads for each protein were analyzed using Luminex xPonent software.Citation27
Follow-Up and Outcomes
Follow-up clinic visits were conducted at 1-year and 2-years after recruitment. At each visit, a full medical history and physical examination was performed including recording of ABI, study outcomes, and changes in clinical status. The outcome of interest was 2-year major adverse limb events (MALE; major lower extremity amputation above the ankle, need for vascular intervention [open or endovascular lower extremity revascularization], or acute limb ischemia [abrupt decrease in limb perfusion [< 14 days] due to arterial thrombosis or embolism]). Initial analysis showed that all adverse limb events occurred in PAD patients; therefore, prognostic models were developed only on the PAD cohort.
Model Development and Evaluation
The patient sample was split into 2 groups: a discovery cohort and a validation cohort. In the discovery cohort, optimal propensity-score matching without replacement was used to match patients with and without PAD based on baseline demographics and comorbidities. Propensity scores were calculated for each variable using log-odds, and a calibration threshold of 0.1 absolute units was used to match the groups. The remaining non-matched patients were placed in the validation cohort to assess real-world model performance. The purpose of the discovery cohort was to identify proteins that are elevated in PAD patients, while the validation cohort was utilized to assess model performance.
The selected predictive model was the random forest, an ensemble learning method that operates through decision trees.Citation28 These decision trees organize samples into branch-like segments to construct prediction algorithms using multiple covariates.Citation29 Notably, random forest is adept at handling large and intricate datasets owing to its non-parametric nature.Citation29 This model was chosen due to its widespread application in the literature and its demonstrated excellent performance in predicting clinical outcomes.Citation30–32
Using data from the model discovery cohort, the random forest model was trained with ten-fold cross validation to predict PAD prognosis (2-year MALE) using the following input features: 1) clinical characteristics (sex, age, dyslipidemia, hypertension, diabetes, past/current smoking, CHF, CAD, previous stroke, leg pain, ABI, acetylsalicylic acid (ASA), statins, angiotensin converting enzyme inhibitor (ACE-I) or angiotensin II receptor blocker (ARB), calcium channel blocker, beta blocker, hydrochlorothiazide or furosemide, oral antihyperglycemic agent, and insulin), 2) inflammatory proteins that were expressed differentially in PAD and non-PAD patients, and 3) both clinical characteristics and inflammatory marker panel. The reason for building and testing the models in this manner is to understand the relatively importance of the inflammatory marker panel in contributing to risk predictions. This was specifically assessed using net reclassification improvement (NRI), which quantifies how well a new model correctly reclassifies subjects.Citation33 Specific to this study, NRI quantifies how much the addition of inflammatory markers to clinical features improves model performance for predicting PAD prognosis.Citation33 The integrated discrimination improvement (IDI) was also calculated for the similar purpose of assessing the added value of inflammatory markers to model performance when compared to using clinical features alone.Citation34 Once trained, the models were evaluated on the validation cohort and the primary metric for evaluating model performance was area under the receiver operating characteristic curve (AUROC).Citation35 The prognostic model was assessed on the PAD cohort only given that all MALE outcomes occurred in PAD patients. The most influential predictive features were identified using variable importance scores (gain), which measure the relative contribution of individual features to a prediction.Citation36 Model development methods were based on our previous work.Citation37,Citation38
Statistical Analysis
Baseline features were presented as means (standard deviation) or numbers (percentage). Differences between groups were evaluated using independent t-tests for continuous variables and chi-square tests for categorical variables. Protein levels were compared between PAD and non-PAD patients using independent t-tests. Proteins demonstrating differential expression between PAD and non-PAD patients were further analyzed for model development. Event rates were compared between patients with and without PAD using chi-square tests. Adjusted hazard ratios (HR) for 2-year MALE per one unit increase in each inflammatory marker were determined using Cox proportional hazards analysis, adjusting for sex, age, hypertension, dyslipidemia, diabetes, past and current smoking, CAD, CHF, previous stroke, leg pain, ABI, ASA, statin, beta blocker, ACE-I/ARB, calcium channel blocker, hydrochlorothiazide or furosemide, oral antihyperglycemic agent, and insulin. Using the prognostic model, patients were classified into either low or high risk of developing 2-year MALE based on the optimal ROC threshold of 0.41. This threshold was determined using the Youden Index, optimizing the performance (sensitivity and specificity) of the prediction model.Citation39 Freedom from MALE over 2 years in low vs high-risk patients was analyzed using Kaplan-Meier curves and compared with Cox proportional hazards analysis. This stratified analysis provided insights into the potential clinical significance of the risk predictions made by the prognostic model, helping clinicians understand the divergence in MALE risk trajectories between low and high-risk patients over a 2-year period. Patients lost to follow-up were censored. Statistical significance was set at a two-tailed p-value below 0.05. All statistical analyses were conducted using SPSS version 23.Citation40
Results
Discovery Cohort for Identification of PAD-Specific Proteins
We performed propensity score matching to create a 200-patient discovery cohort, with patients with and without PAD matched on a 1:1 basis. The remaining 509 patients constituted our validation cohort, which was reserved to assess model performance. Within the discovery cohort, the average age was 67 (SD 10) years, with 59 (29%) female patients. Among them, 125 (63%) had hypertension, 136 (68%) had dyslipidemia, 33 (17%) had diabetes, 100 (50%) were former smokers, 46 (23%) were current smokers, 3 (2%) had CHF, 46 (23%) had CAD, 50 (25%) had a previous stroke, and 123 (62%) experienced leg pain. There were no baseline differences in demographics and comorbidities between PAD and non-PAD patients, supporting the effectiveness of our matching process. As expected, patients with PAD had a lower mean ABI (0.58 [SD 0.11] vs 1.01 [SD 0.02], p<0.001) and a greater proportion received cardiovascular risk-reduction medications including statins (72% vs 57%, p<0.001), ASA (79% vs 52%, p<0.001), beta blockers (46% vs 29%, p=0.001), and ACE-I/ARB (69% vs 43%, p=0.001) ().
Table 1 Baseline Characteristics of Propensity-Score Matched Discovery Cohort
Inflammatory Protein Levels
From a 29-protein panel, we identified 5 that were significantly elevated in PAD vs non-PAD patients: MMP-7 (4.83 [SD 0.39] vs 4.40 [SD 0.32] pg/mL, p = 0.004), IL-6 (8.27 [SD 3.01] vs 6.03 [SD 2.10] pg/mL, p = 0.017), MMP-10 (0.69 [SD 0.26] vs 0.42 [SD 0.30] pg/mL, p = 0.021), CCL2/MCP-1 (658.35 [SD 76.06] vs 573.29 [SD 113.40], p = 0.029), and TFPI (22.98 [SD 4.71] vs 19.26 [SD 6.19] pg/mL, p = 0.031) (). These 5 proteins were used in further analyses to build the predictive model.
Table 2 Inflammatory Protein Levels in Patients with vs Without Peripheral Artery Disease in Discovery Cohort
Validation Cohort
The 5 proteins identified in the discovery cohort were confirmed to be elevated in PAD patients in the validation cohort. The validation cohort consisted of 509 individuals (365 PAD and 144 non-PAD). Given that the cohort was not matched, a greater proportion of PAD patients had hypertension (93% vs 80%, p<0.001), diabetes (50% vs 31%, p<0.001), previous stroke (18% vs 10%, p=0.016), and leg pain (64% vs 17%, p<0.001) compared to non-PAD patients. The mean age was 69 (SD 9), 30% were female, 90% had dyslipidemia, 85% were past or current smokers, and 43% had CAD, with no differences in these characteristics between groups. A greater proportion of PAD patients received ASA (72% vs 41%, p<0.001), statins (76% vs 49%, p<0.001), ACE-I/ARB (70% vs 44%, p=0.001), and beta blockers (41% vs 24%, p=0.001) ().
Table 3 Baseline Characteristics and Inflammatory Protein Levels in Validation Cohort
Events
Event rates were determined in the validation cohort. Complete follow-up was obtained on 94% of patients, with 6% lost to follow-up. Over a 2-year follow-up period, all adverse limb events occurred in patients with PAD: 85 (23%) patients developed MALE, 75 (21%) required a vascular intervention, and 21 (6%) underwent a major amputation. No patients developed acute limb ischemia ().
Table 4 Adverse Limb Events Over 2 Years of Follow-Up in the Validation Cohort
Association Between Inflammatory Protein Levels and Adverse PAD-Related Events
Within the validation cohort, there was a statistically significant correlation between every 1 unit increase in plasma concentrations of inflammatory proteins and 2-year MALE with the following adjusted HR’s (95% CI’s): IL-6 [1.13 (1.09–1.26), p = 0.026], MMP-7 [1.09 (1.06–1.17), p = 0.030], and MMP-10 [1.05 (1.02–1.11), p = 0.039] (). Given that these proteins were associated with adverse PAD-related events, they were further investigated in the study.
Table 5 Hazard Ratios for 2-Year Major Adverse Limb Events for Every 1 Unit Increase in Inflammatory Protein Levels in Validation Cohort
Model Performance in Validation Cohort
In the validation cohort, the random forest model attained the following performance metrics for prognosticating 2-year MALE with these input features: clinical features alone (AUROC 0.63), 5-inflammatory marker panel (AUROC 0.79), and clinical features and inflammatory marker panel (AUROC 0.84) (). The additional of inflammatory markers significantly improved model prognostic performance compared to clinical features alone, with a NRI of 0.64 and IDI of 0.06. The most important predictive features for the prognostic model were 1) CCL-2/MCP-1, 2) IL-6, 3) TFPI, 4) MMP-10, and 5) MMP-7 ().
Figure 1 Receiver operating characteristic curve for random forest model in predicting 2-year major adverse limb event for patients with peripheral artery disease (PAD) in validation cohort. Area represents area under the receiver operating characteristic curve (AUROC), panel refers to inflammatory marker panel consisting of IL-6 (interleukin-6), MMP-7 (matrix metalloproteinase-7), MMP-10 (matrix metalloproteinase-10), CCL-2/MCP-1 (monocyte chemoattractant protein), and TFPI (tissue factor pathway inhibitor).
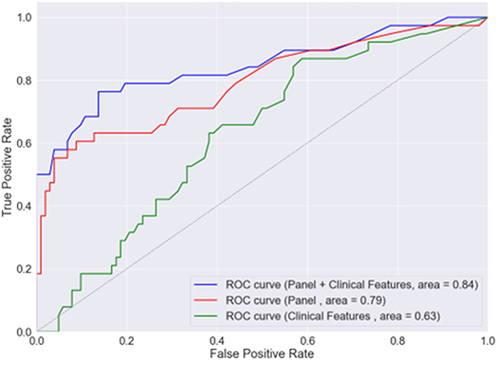
Figure 2 Variable importance scores (gain) for the clinical characteristics and inflammatory markers used as input features for random forest model for prognosis of 2-year major adverse limb events in patients with peripheral artery disease.
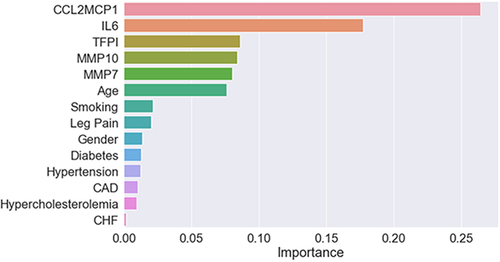
Major Adverse Limb Events in Low vs High-Risk Groups as Predicted by the Model in Validation Cohort
In the validation cohort, the random forest model was utilized to classify individuals into high vs low risk of developing MALE. On Kaplan Meier analysis, individuals predicted to be at high-risk were more likely to develop MALE at both one year (HR 1.78, 95% CI 1.61–1.93) and two years (HR 2.76, 95% CI 2.10–2.98) of follow-up ().
Figure 3 Kaplan-Meier analysis of freedom from major adverse limb events in patients predicted to be at low vs high risk by random forest model. The receiver operating characteristic curve (ROC) threshold used to classify patients into low vs high risk was 0.41. Number of observations: 365 patients with peripheral artery disease. No biological or technical replicates.
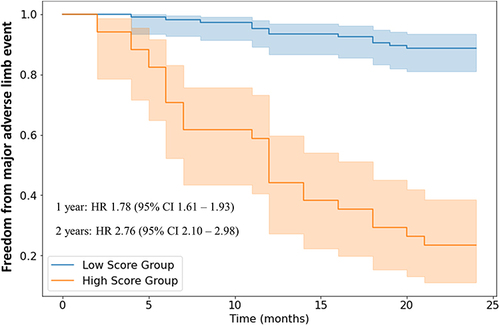
Discussion
Key Findings
In this study, we developed a model utilizing both clinical characteristics and inflammatory biomarker levels to accurately predict PAD prognosis. Several key findings emerged. Firstly, among a panel of 29 inflammatory proteins, five were found to be elevated in PAD compared to non-PAD patients: MMP-7, MMP-10, IL-6, CCL2/MCP-1, and TFPI. Secondly, we demonstrated that relying solely on clinical features for PAD prognosis yielded suboptimal results, with an AUROC of 0.63. However, integrating the 5-biomarker panel significantly enhanced model performance, achieving an AUROC of 0.84. This enhancement was further supported by our feature importance analysis, which highlighted the primary influence of inflammatory biomarker levels on predictive accuracy. Given the significance of these inflammatory proteins, further basic science and translational research is warranted to elucidate their molecular-level relationships with PAD progression, potentially informing targeted therapeutic strategies. Thirdly, we developed predictive models to comprehensively assess the combined impact of clinical features and inflammatory biomarkers on PAD prognosis. Our model exhibited excellent discrimination for PAD prognosis in a validation cohort, achieving an AUROC of 0.84 for 2-year MALE. Lastly, leveraging our prognostic model, we categorized patients into low- and high-risk groups for adverse events. Kaplan-Meier analysis revealed that patients classified as high risk by our model were 2.76 times more likely to develop 2-year MALE compared to those deemed low risk. This underscores the clinical relevance of our model, providing clinicians with valuable insights into the future trajectory of their PAD patients in terms of the risk of adverse limb events.
Comparison to Existing Literature
Ross et al recently published a predictive model for Major Adverse Cardiac and Cerebrovascular Events (MACCE) in PAD patients using electronic health records data.Citation41 Their models, developed with retrospectively-collected diagnostic, procedural, and medical information, aimed to predict MACCE within 30 days following PAD diagnosis, achieving an AUROC of 0.81.Citation41 However, these models had limitations. Firstly, they lacked validation on an independent cohort, potentially inflating performance.Citation42 Secondly, they did not incorporate biomarker data, despite its significant influence on PAD prognosis, as demonstrated by our study and others.Citation4–8 Our models addressed these shortcomings by including inflammatory biomarker levels as predictive features and evaluating performance on an independent validation cohort. Consequently, we observed improved model performance metrics, achieving an AUROC of 0.84 for predicting 2-year MALE. This underscores the importance of developing predictive models that integrate inflammatory biomarker data, potentially enhancing performance compared to relying solely on clinical characteristics. Moreover, assessing model performance on independent validation datasets provides a more accurate assessment of real-world applicability.
Explanation of Findings
The 5 inflammatory markers discovered to be elevated in PAD patients in this study were important predictors of PAD prognosis. These proteins contribute to several cellular and molecular pathways that are important to PAD progression.Citation4–8 IL-6 plays a key role in immune activation and systemic inflammation, and Levin et al (2021) showed significant associations between IL-6 signalling and PAD.Citation4 Specifically, a missense variant in the IL-6 receptor was protective against PAD, demonstrating the importance of IL-6 signalling in PAD development.Citation4 MMP’s are important mediators of the synthesis and breakdown of the vascular extracellular matrix, which are critical factors in atherosclerosis.Citation43 Moreno-Ajona et al showed that elevated circulating levels of MMP-7 predicts adverse cardiovascular events in individuals with carotid artery stenosis.Citation5 Similarly, Martinez-Aguilar et al (2015) demonstrated that MMP-10 levels correlate with disease severity in PAD patients.Citation6 CCL-2/MCP-1 is a mononuclear cell attractant that mediates monocytes-macrophage entry into atherosclerotic lesions, thereby contributing the plaque development.Citation44 This inflammatory marker was one of the most influential predictive features in our model, which corroborates previous findings by Petrkova et al who demonstrated that PAD patients had increased levels of CCL-2/MCP-1.Citation7 TFPI is a protein that inhibits tissue factor, which is an important initiator of the coagulation cascade contributing to atherosclerosis and thrombotic complications.Citation45 Blann et al showed that reduced TFPI levels contributed to higher levels of tissue factor, thereby contributing to atherogenesis and thrombosis in individuals with cardiovascular disease.Citation8 Taken together, these findings explain the importance of these inflammatory biomarkers in predicting PAD prognosis. Similarly, Sapienza et al (2019) demonstrated that plasma levels of tumour necrosis factor-α, IL-6, and MMP-2 and −9 were predictive for vein graft occlusion and wound healing failure after lower extremity arterial bypass.Citation46 These results support the ability for circulating inflammatory biomarkers to predict PAD-related complications.Citation46 Furthermore, leg pain was an important clinical predictor of PAD prognosis, suggesting that our model may accurately differentiate ischemic from non-ischemic pain to identify PAD patients with claudication.Citation47 Second, our study revealed a significant incidence of adverse limb events in PAD patients, with more than 20% of the cohort experiencing 2-year MALE. These findings underscore the urgent need for proactive measures to mitigate adverse outcomes in this population, including the development of more effective prognostic tools. Third, our predictive model demonstrated robust performance for several reasons. Unlike traditional statistical models like logistic regression, which assume linear relationships between covariates and the dependent variable’s log value, machine learning (ML) technology is not bound by such linearity assumptions and can effectively model complex and non-linear relationships between inputs and outputs.Citation48,Citation49 This flexibility is particularly advantageous in healthcare data, where outcomes are influenced by numerous factors.Citation50 This is also relevant in models that consider biomarker data, as various proteins operate in distinct molecular pathways and may interact via complex mechanisms to impact disease processes.Citation51 Our choice of random forest likely contributed to the model’s excellent performance.Citation52 This ensemble learning method aggregates multiple decision trees, reducing variance, efficiently handling large datasets, and mitigating overfitting.Citation52 Overall, our findings underscore the benefits of employing a predictive model that integrates a panel of biomarkers, leading to superior performance compared to models relying solely on individual biomarkers or clinical data. Given PAD’s chronic and multifactorial nature, involving numerous biological pathways, previous research has emphasized the importance of a panel-based approach for enhancing PAD prognosis.Citation53 Our study reaffirms this notion, illustrating that by employing advanced techniques to analyze clinical data alongside inflammatory biomarkers, highly accurate risk prediction tools for PAD can be developed.
Implications
Our predictive models offer practical implications for guiding clinical decision-making across various scenarios. Firstly, our tool can be utilized to screen patients for asymptomatic PAD, particularly beneficial in family practice settings. General practitioners can integrate a 5-protein plasma panel with their clinical assessment to determine a patient’s PAD risk using our automated algorithm.Citation54 Those screening positive can then undergo further vascular evaluation, such as arterial duplex ultrasound, to confirm PAD presence and assess blood flow.Citation55 Upon PAD confirmation, our algorithm can aid in evaluating a patient’s risk of adverse PAD-related events using the same 5 proteins and clinical data. Patients at low risk can continue care with their family physician, focusing on optimizing risk factors through measures like ASA, statins, and lifestyle changes.Citation56 Conversely, patients identified as high risk should be referred to a vascular surgeon for additional evaluation and management.Citation57 Subsequently, vascular surgeons can utilize the algorithm alongside clinical judgment to identify higher-risk individuals for additional vascular imaging,Citation58 medical management with low-dose rivaroxaban,Citation59 and/or interventions for limb salvage in the highest risk patients.Citation60,Citation61 Given that our PAD cohort only included patients who were asymptomatic or had claudication, our study demonstrates the potential utility of the identified biomarkers for prognostication in early stages of PAD. Overall, our automated tool has the potential to enhance care for PAD patients in both generalist and specialist settings, streamlining PAD screening, risk stratification, and early identification of individuals at high risk for adverse limb events. This, in turn, can minimize unnecessary specialist referrals, improve PAD outcomes, and reduce healthcare costs.Citation62
Limitations
Our study has several limitations. Firstly, it was conducted at a single center, necessitating future validation in other institutions to assess the generalizability of our model. Secondly, the reported outcomes were based on a 2-year follow-up period, highlighting the need for longer-term follow-up to comprehensively understand the prognostic value of our algorithm, particularly given the chronic nature of PAD. Thirdly, the biomarkers analyzed in our study are predominantly used in research settings, indicating the necessity for further translational research and implementation science to demonstrate the clinical value and feasibility of incorporating these inflammatory biomarkers into routine care for PAD patients.
Conclusion
In this study, we utilized a panel of 5 inflammatory biomarkers alongside clinical characteristics to construct a model that effectively predicts PAD prognosis in an independent validation cohort. Our model holds promise for PAD screening and risk-stratification, facilitating early identification and targeted management of the condition. Notably, high-risk patients can be promptly referred for further vascular evaluation and may derive significant benefit from more aggressive medical interventions. The continuous learning and automation features of our ML algorithms bolster their practicality in clinical settings, offering potential enhancements in PAD patient care. Moreover, our findings illuminate directions for future research. Sole reliance on clinical characteristics proves insufficient in predicting PAD prognosis, underscoring the importance of integrating inflammatory biomarkers into prediction models to optimize performance. This underscores the imperative for both basic and translational investigations exploring the mechanistic interplay between inflammatory proteins and PAD development/progression. Such endeavors hold promise for advancing our comprehension of underlying pathogenesis and may guide the development of targeted therapeutic approaches. Crucially, our study furnishes compelling grounds for conducting clinical trials evaluating the impact of predictive algorithms on PAD outcomes. By assessing the real-world effectiveness of these models, we stand to glean invaluable insights into their potential to inform clinical decision-making and enhance PAD prognosis.
Author Contributions
All authors made a significant contribution to the work reported, whether that is in the conception, study design, execution, acquisition of data, analysis and interpretation, or in all these areas; took part in drafting, revising or critically reviewing the article; gave final approval of the version to be published; have agreed on the journal to which the article has been submitted; and agree to be accountable for all aspects of the work. B. Li and A. Zamzam: methodology, statistical analysis, data analysis and interpretation, writing - original draft, revising the manuscript for important intellectual content, and approval of the final manuscript draft submitted for publication. F. Shaikh, H. Younes, M. Syed, and R. Raphael: acquisition, analysis, and/or interpretation of data, revising the manuscript for important intellectual content, and approval of the final manuscript draft submitted for publication. R. Abdin and M. Qadura: study concept and design, methodology, data analysis and interpretation, writing - original draft, revising the manuscript for important intellectual content, and approval of the final manuscript draft submitted for publication.
Disclosure
The authors report no conflicts of interest in this work.
Acknowledgments
We would like to thank Dr. David Szalay for his thoughtful contributions to discussions regarding study design and methodology for this manuscript.
Data Sharing Statement
The original contributions presented in the study are included in the article, further inquiries can be directed to the corresponding author.
Additional information
Funding
References
- Zemaitis MR, Boll JM, Dreyer MA. Peripheral Arterial Disease. In: StatPearls. StatPearls Publishing; 2021 Available from: http://www.ncbi.nlm.nih.gov/books/NBK430745/. Accessed June 14, 2021.
- Olin JW, Sealove BA. Peripheral artery disease: current insight into the disease and its diagnosis and management. Mayo Clin Proc. 2010;85(7):678–692. doi:10.4065/mcp.2010.0133
- Mehta A, Dhindsa DS, Hooda A, et al. Premature atherosclerotic peripheral artery disease: an underrecognized and undertreated disorder with a rising global prevalence. Trends Cardiovasc Med. 2021;31(6):351–358. doi:10.1016/j.tcm.2020.06.005
- Levin MG, Klarin D, Georgakis MK, et al. A missense variant in the IL-6 receptor and protection from peripheral artery disease. Circ Res. 2021;129(10):968–970. doi:10.1161/CIRCRESAHA.121.319589
- Moreno-Ajona D, Irimia P, Rodríguez JA, et al. Elevated circulating metalloproteinase 7 predicts recurrent cardiovascular events in patients with carotid stenosis: a prospective cohort study. BMC Cardiovasc Disord. 2020;20:93. doi:10.1186/s12872-020-01387-3
- Martinez-Aguilar E, Gomez-Rodriguez V, Orbe J, et al. Matrix metalloproteinase 10 is associated with disease severity and mortality in patients with peripheral arterial disease. J Vasc Surg. 2015;61(2):428–435. doi:10.1016/j.jvs.2014.09.002
- Petrkova J, Szotkowska J, Hermanova Z, Lukl J, Petrek M. Monocyte chemoattractant protein-1 in patients with peripheral arterial disease. Mediators Inflamm. 2004;13(1):39–43. doi:10.1080/09629350410001664752
- Blann AD, Amiral J, McCollum CN, Lip GY. Differences in free and total tissue factor pathway inhibitor, and tissue factor in peripheral artery disease compared to healthy controls. Atherosclerosis. 2000;152(1):29–34. doi:10.1016/s0021-9150(99)00444-x
- Ceasovschih A, Sorodoc V, Onofrei (Aursulesei) V, et al. Biomarker utility for peripheral artery disease diagnosis in real clinical practice: a prospective study. Diagnostics. 2020;10(9):723. doi:10.3390/diagnostics10090723
- Kremers B, Wübbeke L, Mees B, Ten Cate H, Spronk H, Ten Cate-Hoek A. Plasma biomarkers to predict cardiovascular outcome in patients with peripheral artery disease: a systematic review and meta-analysis. Arterioscler Thromb Vasc Biol. 2020;40(9):2018–2032. doi:10.1161/ATVBAHA.120.314774
- Zakynthinos E, Pappa N. Inflammatory biomarkers in coronary artery disease. J Cardiol. 2009;53(3):317–333. doi:10.1016/j.jjcc.2008.12.007
- Gyanwali B, Lai MKP, Lui B, et al. Blood-based cardiac biomarkers and the risk of cognitive decline, cerebrovascular disease, and clinical events. Stroke. 2021;52(7):2275–2283. doi:10.1161/STROKEAHA.120.032571
- Parvar SL, Fitridge R, Dawson J, Nicholls SJ. Medical and lifestyle management of peripheral arterial disease. J Vasc Surg. 2018;68(5):1595–1606. doi:10.1016/j.jvs.2018.07.027
- Del Río V C, Mostaza J, Lahoz C, et al. Prevalence of peripheral artery disease (PAD) and factors associated: an epidemiological analysis from the population-based Screening PRE-diabetes and type 2 DIAbetes (SPREDIA-2) study. PLoS One. 2017;12(10):e0186220. doi:10.1371/journal.pone.0186220
- Duval S, Massaro JM, Jaff MR, et al. An evidence-based score to detect prevalent peripheral artery disease (PAD). Vasc Med Lond Engl. 2012;17(5):342–351. doi:10.1177/1358863X12445102
- Gouda P, Ramasundarahettige C, Anand S, et al. Clinical factors associated with peripheral artery disease in patients with documented coronary artery disease: a post hoc analysis of the COMPASS trial. Atherosclerosis. 2021;331:38–44. doi:10.1016/j.atherosclerosis.2021.07.003
- Ielapi N, Andreucci M, Licastro N, et al. Precision medicine and precision nursing: the era of biomarkers and precision health. Int J Gen Med. 2020;13:1705–1711. doi:10.2147/IJGM.S285262
- World Medical Association. World medical association declaration of Helsinki: ethical principles for medical research involving human subjects. JAMA. 2013;310(20):2191–2194. doi:10.1001/jama.2013.281053
- Collins GS, Reitsma JB, Altman DG, Moons KGM. Transparent Reporting of a multivariable prediction model for Individual Prognosis or Diagnosis (TRIPOD): the TRIPOD statement. Ann Intern Med. 2015;162(1):55–63. doi:10.7326/M14-0697
- Gul F, Janzer SF. Peripheral Vascular Disease. In: StatPearls. StatPearls Publishing; 2021 http://www.ncbi.nlm.nih.gov/books/NBK557482/. Accessed June 30, 2021.
- Grundy SM, Stone NJ, Bailey AL, et al. 2018 AHA/ACC/AACVPR/AAPA/ABC/ACPM/ADA/AGS/APhA/ASPC/NLA/PCNA Guideline on the Management of Blood Cholesterol. J Am Coll Cardiol. 2019;73(24):e285–e350. doi:10.1016/j.jacc.2018.11.003
- Whelton PK, Carey RM, Aronow WS, et al. 2017 ACC/AHA/AAPA/ABC/ACPM/AGS/APhA/ASH/ASPC/NMA/PCNA guideline for the prevention, detection, evaluation, and management of high blood pressure in adults. J Am Coll Cardiol. 2018;71(19):e127–e248. doi:10.1016/j.jacc.2017.11.006
- Syed MH, Zamzam A, Khan H, et al. Fatty acid binding protein 3 is associated with peripheral arterial disease. JVS-Vasc Sci. 2020;1:168–175. doi:10.1016/j.jvssci.2020.08.003
- Luminex Assays, Multiplex Immunoassays. Bio-Techne. Available from: https://www.bio-techne.com/. Accessed May 6, 2023.
- MAGPIX® System. xMAP Instrument | luminex Corporation. Available from: https://www.luminexcorp.com/magpix-system/. Accessed December 18, 2021.
- Luminex Assays - CA. Available from: //www.thermofisher.com/ca/en/home/life-science/antibodies/immunoassays/procartaplex-assays-luminex.html. Accessed December 18, 2021.
- xPONENT® Software for xMAP® Instruments. Luminex Corporation. Available from: https://www.luminexcorp.com/xponent/. Accessed May 6, 2023.
- Rigatti SJ. Random Forest. J Insur Med N Y N. 2017;47(1):31–39. doi:10.17849/insm-47-01-31-39.1
- Song YY, Lu Y. Decision tree methods: applications for classification and prediction. Shanghai Arch Psychiatry. 2015;27(2):130–135. doi:10.11919/j.issn.1002-0829.215044
- Elfanagely O, Toyoda Y, Othman S, et al. Machine learning and surgical outcomes prediction: a systematic review. J Surg Res. 2021;264:346–361. doi:10.1016/j.jss.2021.02.045
- Bektaş M, Tuynman JB, Costa Pereira J, Burchell GL, van der Peet DL. Machine learning algorithms for predicting surgical outcomes after colorectal surgery: a systematic review. World J Surg. 2022;46:3100–3110. doi:10.1007/s00268-022-06728-1
- Senders JT, Staples PC, Karhade AV, et al. Machine learning and neurosurgical outcome prediction: a systematic review. World Neurosurg. 2018;109:476–486.e1. doi:10.1016/j.wneu.2017.09.149
- Kerr KF, Wang Z, Janes H, McClelland RL, Psaty BM, Pepe MS. Net reclassification indices for evaluating risk-prediction instruments: a critical review. Epidemiol Camb Mass. 2014;25(1):114–121. doi:10.1097/EDE.0000000000000018
- Pencina MJ, Demler OV, Demler OV. Novel metrics for evaluating improvement in discrimination: net reclassification and integrated discrimination improvement for normal variables and nested models. Stat Med. 2012;31(2):101–113. doi:10.1002/sim.4348
- Hajian-Tilaki K. Receiver operating characteristic (ROC) curve analysis for medical diagnostic test evaluation. Casp J Intern Med. 2013;4(2):627–635.
- Loh WY, Zhou P. Variable Importance Scores. J Data Sci. 2021;19(4):569–592. doi:10.6339/21-JDS1023
- Li B, Shaikh F, Zamzam A, Syed MH, Abdin R, Qadura M. A machine learning algorithm for peripheral artery disease prognosis using biomarker data. iScience. 2024;27(3):109081. doi:10.1016/j.isci.2024.109081
- Li B, Nassereldine R, Zamzam A, et al. Development and evaluation of a prediction model for peripheral artery disease-related major adverse limb events using novel biomarker data. J Vasc Surg. 2024. doi:10.1016/j.jvs.2024.03.450
- Ruopp MD, Perkins NJ, Whitcomb BW, Schisterman EF. Youden index and optimal cut-point estimated from observations affected by a lower limit of detection. Biom J Biom Z. 2008;50(3):419–430. doi:10.1002/bimj.200710415
- SPSS Software; 2021. Available from: https://www.ibm.com/analytics/spss-statistics-software. Accessed December 18, 2021.
- Ross EG, Jung K, Dudley JT, Li L, Leeper NJ, Shah NH. Predicting future cardiovascular events in patients with peripheral artery disease using electronic health record data. Circ Cardiovasc Qual Outcomes. 2019;12(3):e004741. doi:10.1161/CIRCOUTCOMES.118.004741
- Liu Y, Chen PHC, Krause J, Peng L. How to read articles that use machine learning: users’ guides to the medical literature. JAMA. 2019;322(18):1806–1816. doi:10.1001/jama.2019.16489
- Chistiakov DA, Sobenin IA, Orekhov AN. Vascular extracellular matrix in atherosclerosis. Cardiol Rev. 2013;21(6):270–288. doi:10.1097/CRD.0b013e31828c5ced
- Valente AJ, Rozek MM, Sprague EA, Schwartz CJ. Mechanisms in intimal monocyte-macrophage recruitment. A special role for monocyte chemotactic protein-1. Circulation. 1992;86(6 Suppl):III20–25.
- Caplice NM, Mueske CS, Kleppe LS, Simari RD. Presence of tissue factor pathway inhibitor in human atherosclerotic plaques is associated with reduced tissue factor activity. Circulation. 1998;98(11):1051–1057. doi:10.1161/01.CIR.98.11.1051
- Sapienza P, Mingoli A, Borrelli V, et al. Inflammatory biomarkers, vascular procedures of lower limbs, and wound healing. Int Wound J. 2019;16(3):716–723. doi:10.1111/iwj.13086
- Patel SK, Surowiec SM. Intermittent Claudication. In: StatPearls. StatPearls Publishing; 2023 http://www.ncbi.nlm.nih.gov/books/NBK430778/. Accessed July 16, 2023.
- Stoltzfus JC. Logistic regression: a brief primer. Acad Emerg Med off J Soc Acad Emerg Med. 2011;18(10):1099–1104. doi:10.1111/j.1553-2712.2011.01185.x
- Kia B, Mendes A, Parnami A, George R, Mobley K, Ditto WL. Nonlinear dynamics based machine learning: utilizing dynamics-based flexibility of nonlinear circuits to implement different functions. PLoS One. 2020;15(3):e0228534. doi:10.1371/journal.pone.0228534
- Chatterjee P, Cymberknop LJ, Armentano RL. Nonlinear systems in healthcare towards intelligent disease prediction. Nonlinear Syst Theor Asp Recent Appl. 2019;1(1):e88163. doi:10.5772/intechopen.88163
- Smith BJ, Silva-Costa LC, Martins-de-Souza D. Human disease biomarker panels through systems biology. Biophys Rev. 2021;13(6):1179–1190. doi:10.1007/s12551-021-00849-y
- Couronné R, Probst P, Boulesteix AL. Random forest versus logistic regression: a large-scale benchmark experiment. BMC Bioinf. 2018;19:270. doi:10.1186/s12859-018-2264-5
- Flores AM, Demsas F, Leeper NJ, Ross EG. Leveraging machine learning and artificial intelligence to improve peripheral artery disease detection, treatment, and outcomes. Circ Res. 2021;128(12):1833–1850. doi:10.1161/CIRCRESAHA.121.318224
- Nakar S, Vinker S, Neuman S, Kitai E, Yaphe J. Baseline tests or screening: what tests do family physicians order routinely on their healthy patients? J Med Screen. 2002;9(3):133–134. doi:10.1136/jms.9.3.133
- Akbari CM, Stone L. Accreditation and credentialing in the vascular laboratory. Semin Vasc Surg. 2002;15(3):178–181. doi:10.1016/S0895-7967(02)70029-X
- Burns P, Gough S, Bradbury AW. Management of peripheral arterial disease in primary care. BMJ. 2003;326(7389):584–588. doi:10.1136/bmj.326.7389.584
- Koh CE, Walker SR. Vascular surgery consults: a significant workload. ANZ J Surg. 2007;77(5):352–354. doi:10.1111/j.1445-2197.2007.04058.x
- Nishimiya K, Matsumoto Y, Shimokawa H. Recent Advances in Vascular Imaging. Arterioscler Thromb Vasc Biol. 2020;40(12):e313–e321. doi:10.1161/ATVBAHA.120.313609
- Eikelboom JW, Connolly SJ, Bosch J, et al. Rivaroxaban with or without aspirin in stable cardiovascular disease. N Engl J Med. 2017;377(14):1319–1330. doi:10.1056/NEJMoa1709118
- Farber A, Menard MT, Conte MS, et al. Surgery or Endovascular Therapy for Chronic Limb-Threatening Ischemia. N Engl J Med. 2022;387:2305–2316. doi:10.1056/NEJMoa2207899
- Caetano AP, Conde Vasco I, Veloso Gomes F, et al. Successful Revascularization has a significant impact on limb salvage rate and wound healing for patients with diabetic foot ulcers: single-centre retrospective analysis with a multidisciplinary approach. Cardiovasc Intervent Radiol. 2020;43(10):1449–1459. doi:10.1007/s00270-020-02604-4
- Margolis J, Barron JJ, Grochulski WD. Health care resources and costs for treating peripheral artery disease in a managed care population: results from analysis of administrative claims data. J Manag Care Pharm JMCP. 2005;11(9):727–734. doi:10.18553/jmcp.2005.11.9.727