Abstract
We show the potential of the nanosilicon structure of the frustules of a typical diatom, Cymatopleura sp., as a new vehicle for drugs. The basic diatom nanostructure is a lattice of SiO2, and computerized methods in a dock project have identified the most likely and the best drug types to load into such a structure.
Introduction
Desired loading and release properties for drug delivery could be achieved through selection of appropriate systems and drug molecules. Designing such new carriers for drug delivery is an important field of research. Nanotechnology as one of the newest fields of technology can help us to use naturally occurring structures in designing new drug vehicles.
Diatoms (Chrysophyta: Bacillariophaceae) are a large group of unicellular microalgae, comprising more than 10,000 species. They exist in vast numbers and are easily available on Earth.Citation1 Their cell wall consists of a siliceous skeleton (frustules) enveloped by an organic case essentially composed of polysaccharides and proteins.Citation2 This structure makes diatoms suitable for use in industry, nanotechnology and drug delivery, as their biosilica makes special nanostructural patterns.Citation2
Diatoms as a group are very diverse, comprising some 12,000–60,000 reported species and commonly between 20–200 microns diameter.Citation3 They are split into two broad groups: centric and pinnate, depending on the symmetry of frustules. All diatoms have parallel striae (furrows or rows of holes in the silica) arranged normal to the structure axis.Citation4
The spacing between adjacent striae is species specific, but typically varies from 0.3 to 2 μm. The line of silica between the striae is called costae.Citation5 Costae tend to be symmetrically arranged in combs or other space filling patterns.Citation6
The cell wall or frustule is pill box like and consists of two valves that fit within each other with the help of a set of girded bands.Citation5 Frustules vary greatly in shape ranging from boxshaped to cylindrical. Frustules display an unparalleled diversity in structure and morphology and they may be possibly exploited in a number of nanotechnology applications:
Immunoisolation
Immunological bioencapsulation could benefit from frustule properties. The key feature of such capsules would be the ability of frustule pores to protect the enclosed tissue from immune rejection while allowing an adequate supply of nutrients and oxygen. One possible drawback could be the immune reactivity of the silica itself.Citation7
Microfabrication
Diatom frustules have potential use in the production of nanomaterials of constant diameter. For example, using a template synthesis method, it may be possible to arrange these pores to form nanometer-sized tubes and/or fibers.
Lithographic applications
Using the costae of pennate diatoms as a mask in the lithographic process led to the formation of a parallel array of uniform channels. Use of centric diatoms, the exposed surface of which is resistant to the etching process, leads to formation of radiating channels.Citation8
Magnetized frustules for pinpoint drug delivery
To produce magnetized frustules, diatoms can be cultured in an iron-rich environment, as described by Wee et al.Citation8 Drugs or vaccines could be loaded into the pores and delivered by magnetic field manipulation at a target location within the human or animal body.
Nanoformulation for drugs using diatom skeleton nanovehicels
Recently investigations have been made to use the specific nanostructure of diatom frustules as nanovehicles for drugs. Simulations show that diatom frustules could have a regular specificity of drug release pattern, which would be extremely useful for nano-drug delivery, especially in cancer treatments.Citation10
Other useful applications of diatoms in nanotechnology include gel and water filtration as well as embedding of diatom frustules in metal film membranes.Citation11
In this study, we first simulated the basic structure of silicon nanochambers, then using these simulations we determine the best drugs for loading in the silicon nanostructures, and finally we describe the structure–function relationship of these diatom-loaded drugs.
Methods
Selection of diatom model
Our simulation model is based on the known and observed properties of a specific, typical diatom: Cymatopleura sp. Cymatopleura sp. is from the pennate group of diatoms, and the nanotube is more half-cylindrical than the classic fully cylindrical shape ().
Simulation and computational methods
Silicon nanostructure was designed and drawn in Chemdraw® Ultra (Cambridge, ChemOffice Suite, 8.0.3, 2004). To understand the behavior of the molecules in a silicon nanochamber, we applied the Arguslab molecular docking Software, (4.01, 2005) to obtain interaction energy (IE) measures between the bioactive compound’s molecule and the silicon surface.
We calculated IE for the molecule as if there were no other molecules in the chamber. The units for IE are kcal/mol. The grid resolution was 0.4 and augment root node was applied false.
The binding site box for docking was 150 × 150 × 150 angstrom, as it was felt these dimensions gave the best position for the molecule if no other molecules are present. The smaller the overall dimension of the binding site, the larger the IE, so molecules with lower index interact more strongly with surface hydroxyl groups.
To better understand the effects of the various parameters controlling the loading of drug into silicon nanochambers, most physicochemical parameters of the drugs were measured using ACDLab/Chemsketch (Version 5.12, 2003).
The measured parameters were: molecular weight; number and percentage of nitrogen and oxygen atoms; polarizability (cm3); monoisotopic mass (Da); density (g/cm3); nominal mass (Da); surface tension (dyne/cm); index of refraction; molar volume (cm3); molar refractivity (cm3); hydration energies (kcal/mol); average mass (Da); parachor (cm3); and the log base 10 of parachor (Log P). These physicochemical properties have been previously shown to be best for describing the controlling factors for drug loading.Citation11–Citation14
We used artificial neural networks (ANN) to evaluate the importance of these parameters within the diatom structure study. A standard feed-forward network, with back propagation rule and with single hidden layer architecture was chosen using the program EasyNN, 8.01 (1999–2001). Physicochemical properties were the inputs and IE the output of the network architecture.
It should be mentioned that some physicochemical descriptors may reflect related or similar molecular/atomic properties and could be correlated in certain cases (even though the analytical representation of those descriptors does not directly imply their colinearity). Therefore, special precautions were taken in selecting the appropriate descriptors for the neural network model. To eliminate cross-correlation among the independent variables, we pre-computed pairwise regressions between all pairs of the descriptors using NCSS (2006 release) software. We then removed those descriptors that correlated with |r| ≥ 0.9. The parameters: monoisotopic mass; parachor; nominal mass; molar refractivity; average mass; and percent of nitrogen and oxygen atoms were shown to be highly correlated in various ways, and were removed from dataset.
ANNs have the capacity to over fit in the training phase, usually produced by more weights due to higher numbers of neurons in input and hidden layers. We addressed this problem by testing the model using artificial datasets with exactly the same attributes but randomly permuted class labels and by minimizing the number of hidden layers. This is typically referred to as the Y-randomization test.
Classification accuracy for randomized datasets was significantly lower than for the original datasets (maximum R2 = 0.228, minimum R2 = 0.007) and hence we concluded that there is no evidence of over fitting in our models (data not shown).
The number of hidden layers was allowed to vary from 10 to 50. ANN models were tested on the artificial datasets, and only those with errors less than 0.05% were retained.
The ideal ANN model therefore was one therefore predicted the parameters well (<0.05% error) and which had the least number of calculation cycles (). Generally, higher numbers of hidden layers did not improve the performance, but could decrease the speed of calculation.
Table 2 The neural network architectures produced with the descriptors for bio-active compound with the error limit of <0.05
Drugs and bioactive molecules
We investigated 160 drug molecules and bioactive compounds. These are shown in .
Table 1 Names of drugs and bioactive molecules used in this study
Results
The surface morphology of the diatom frustules must follow some 3D structure much as . The basic component of the molecules is SiO2. The structure shows a basic atomic arrangement of nanosilicon, similar to what we can see in a carbon nanotube. The major difference between these two structures is that a silicon nanostructure is composed of silicon and oxygen, while a carbon nanotube is composed of only carbon atoms.
After docking the molecules, the best molecules according to IE (greater negative values, to a moderate range) were selected and are shown in .
Figure 2 The highest interacting molecules according to the interaction energies (kcal/mol): In each cell the numbers show the IE (kcal/mol).
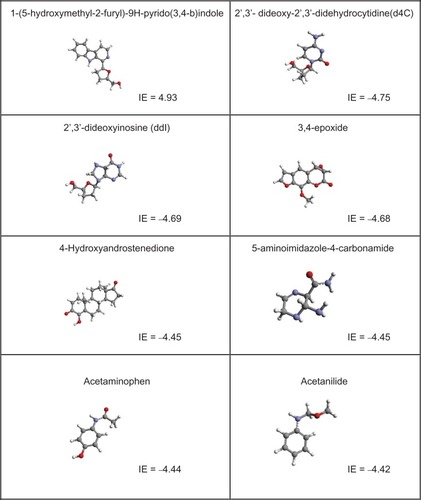
To better understand the possible behavior of the molecules in silicon nanostructures, it is helpful to consider the relationships between IE and the various relevant physicochemical properties of molecule. compares IE and the molecule size. Broadly, the larger the molecule, the lower the IE, and hence the molecule loads more easily into the diatom’s silicon nanostructure. This improvement will be limited by other physical factors. Strong binding of the molecules to the nanovehicles could impair the release of the molecules from the nanovehicle. Furthermore, larger mass and size of the accumulated voluminous functional groups of the molecules will lead to increasing spatial hindrance around the molecules and thus to less hydrogen binding formations, which in turn decrease the IE.
Log P () represents the degree to which the compound is partitioned between water and octanol (or any other non-miscible solvent). Hence, Log P is a criterion of differential solubility of the compound between these two kinds of solvents and indicates how hydrophilic or hydrophobic is any given chemical substance.
The other important physicochemical properties are hydration energy, molar refractivity (a measure of the volume occupied by an atom or group), and surface tension (shown in , , and respectively).
The ANN procedure, a multilayer perceptron with optimized weights, was accomplished by Easy NN-plus 8.01 (1999–2001). To produce the optimum architecture, powerful enough to model the functions and not create errors more than 0.05%, the number of hidden layer neurons was varied from 1 to 50.Citation15 The following architectures were produced that met the error limit condition, which was defined as the least number of calculation cycles (). Higher numbers of hidden layers did not improve the performance. Architectures 11-1-1, 11-2-1 and 11-3-1 produced network models with poor reliability and robustness, whereas architecture 11-7-1 produced the overall best performing model.
The sum of absolute weights connected to the next layer of the architecture is presumed as a criterion for the importance of a parameter. Therefore, importance of an input descriptor is determined by the sum of the absolute values of the weights of all the outgoing architecture connections from the input node to the next layer. Using the best case ANN architecture11-7-1, the most important factors following this methodology were surface tension, molar volume, and Log P.
The correlation coefficient between the experimental and the predicted value obtained by ANN architecture 11-7-1 connected to the next layer of the architecture is 0.88 (). Descriptors molar volume, index of refraction, and Log P had highest relative sensitivity.
Cross validation was performed using the 0.29 m2 leave -n- out (n = 4) method. This showed that average absolute learning error was 1.61% and average correct after rounding was 94.29%.
A positive control set of 10 known bioactive compounds was simulated using the best case (11-7-1) ANN model and resulted in regression correlation of R2 = 0.88.
Discussion
Desired loading and release properties could be achieved through selection of appropriate drug delivery systems and drug molecules. Nowadays, designing new carriers for drug delivery is an important field of research. Nanotechnology, as one of the newest fields of technology, enables natural structures to be used to design new drug vehicles.Citation16 Our simulation results indicate the atomic arrangement of silicon nanostructures is similar to carbon nanotubes and with sizes ranging between 10 and 500 nm, are composed of silicon and oxygen atoms.Citation17 Some advantages of these structures in nano-drug delivery are:
The benefit of the porous “honeycomb” structure provides a large surface area which is an ideal matrix for high capacity and efficient drug loading and release.
Drug release kinetics factors could be controllable by altering the physical properties of the biosilicon matrix, eg, by adjusting the level of porosity, altering the pore size or particle size.
Applicability for a wide range of therapeutic entities from small molecules to peptides and proteins including hydrophobic and hydrophilic entities; drug loading does not require chemical modification of the molecule.
There are no changes in drug structure or activity after loading and subsequent release.
Simulation of these patterns to understand factors that may have a role in dynamics of silica-like nanotubes could be helpful in elucidation of mechanisms which govern dynamics of material movement and release from diatom frustules with silica walls. In this study, nanosilicon structure of frustules was simulated and then the interaction of a wide range of drugs and bioactive compounds with this nanosilicon structure was investigated.
It is believed that liberation of a drug from a nanostructure cavity and solubilization in surrounding aqueous medium are the key parameters in determination of the release rate of drug from nanocapsule as well as drug partition coefficient.Citation18 Our ANN-based study reported here indicates that surface tension, molar volume, and Log P are the most important factors in drug loading. These properties would lead to formation of a layer of loaded drug molecules in the nanochamber very quickly and would prevent the alveolar collapse phenomenon during the drug release.
Our results indicate that increase in surface tension and hydration energies of bioactive compound would increase IE whereas increasing molecular size and molecular refractivity would tend to decrease IE.
Surface tension of the drug molecule affects how easily the drug itself moves around and into the inner diameter. Lower surface tension energy promotes coalescence.
The effect of octanol–water partition coefficient on loading of drug molecules in the silicon nanochamber was evaluated. It is known that the molecules that have the highest partition coefficients are the most lipophilic drugs and our result shows that such molecules have better loading values (defined by IE) in the nanochamber. Since aqueous solubility is inversely proportional to the partition coefficient, the molecules with lower aqueous solubility have higher interaction energies in nanospheres. shows a representative trend with two peaks of IE at approximately −4 kcal/mol and −4.1 kcal/mol.
Additionally, we have shown molar volume, molar refractivity, and Log P are descriptors with the highest relative sensitivity. Sensitivity is a measure of how the outputs change when the inputs are changed. Since molar refraction is a combined parameter it is in essence an adjustable molecular volume and thus equates to the molar polarizability and tends to measure the contribution due to size and shape of organic compounds.
We have shown significant differences between properties of compounds of higher errors in ANN models with the other bioactive compounds of the dataset. The result of t-test for equality of means (P = 0.05%, SPSS 15) shows that there are significant difference between molecular weight, number of oxygen atoms, molar volume and polarizability of two groups of bioactive compounds. On the other hand, Log P, hydration energy, index of refraction, surface tension and density are not significant.
Increase in IE of larger molecules could be a result of the physical size of the bioactive compounds. Smaller molecules would better penetrate into the grooves of the nanostructure and easily self-position to become rather more stable conformations. On the other hand, larger molecules would not be located as deeply in any interaction position and could be displaced more easily.
It should be mentioned that the interacting surface of a bioactive compound with hydrogen bonding hydroxyl groups attached to silicon structure could have an acidic property. This could interact with regions of a bioactive compound which have a base nature and those with larger numbers of hydrogen bonds, forming functional groups.
The more hydrogen bonds between the drug and curved surface at the correct penetration position, the stronger the interaction. This depends on spatial hindrance around hydroxyl groups of the surface. For example, in alcoholic groups hydrogen bonds form between the oxygen of an alkoxy group of the bioactive compound, thereby crowding hydroxyl groups of the nanostructure surface. Because of these formed functional groups, attraction is decreased (for example, 5-aminoimidazole-4-carbonamide, see ).
Smaller molecules that have both hydroxyl and alkoxy groups will have increased interaction because of low crowding of hydrogen bonding and also the attraction between alkoxy and hydroxyl onto the nanostructure increases. Although basicity is important, it does not appear to play a very crucial role. Agents like caffeine, histamine, and lysine which are scattered through the energy range could be indicative of this point.
However, we should mention that the overall energy range difference between the highest and lowest values do not exceed 2 kcal/mol, in contrast to diversity of structures studied here.
It might be possible to use diatom shells as delivery vehicles for drugs. They have a uniform nanoscale pore structure and are chemically inert and biocompatible. Diatoms could then be loaded with a drug that would then leach out into the bloodstream at a rate dependent on the diatom species used. By incorporating ferromagnetic particles within the diatom structure, it might be possible to use a magnet to guide the drug to the right organ.
Therefore it is necessary that methods for efficiently incorporating ferromagnetic particles like magnesium within diatom structures be developed rapidly to reach this goal. Much more investigation is needed and many questions, such as the immune reactivity of the silica itself, remain to be answered.
Considering other nanostructures in future and comparing the results of those studies with the present study, would help clarify some remaining ambiguities and more clearly show the potential for these techniques.
Conclusions
The actual, rather than full, efficacy of a drug-based remedy depends on many factors as well as the bioactivity of the pharmaceutical compound. Among the effective factors the manner by which the compound is made available to the target is of great importance, since a favorable delivery mechanism greatly improves the availability of the compound and provides a more confident base for prediction of the time, duration, and location of impact as well as usable dosage of the bioactive compound. One possible option to improve this availability is the use of a nanovehicles, such as those we have introduced.
ANN-based models helped us to better understand the capabilities of the designed silicon nanocarrier as the method for quantifying the reaction patterns of an extensive range of compounds.
We have determined the physicochemical properties of bioactive compounds that have the most important impact on efficiency and prediction of delivery patterns of the nano structure. The bioactive compounds were selected to ensure a wide range of structures and chemical properties, giving some confidence these outcomes would be a reliable guide for prediction of the general behavior of similar compounds in addition to those specific compounds used in this trial.
Based on the common properties of each group of the compounds, we have presented some physical and chemical arguments to justify the observed interaction patterns.
Comparison between groups of compounds with high ANN model errors with those with low ANN errors has allowed some insight into the abilities and limits of an ANN based strategy in in silico investigation of the nanocarrier, and the variables that may have an important role in optimization of the models.
Disclosure
The authors report no conflicts of interest in this work.
References
- VyvermanWVerleyenESabbeKHistorical process constrains patterns in global diatom diversityEco200788819241931
- GilstadMSakshaugEGrowth rates of ten diatom species from the Barents Sea at different irradiances and day lengthsMar Ecol Prog Ser199964169173
- Pickett-HeapsJDTippitDHAndreozziJACell division in the pennate diatom pinularia, valve morphogenesisBiol Cell197935199205
- GordonRSterrenburgFSandhageKHA Special Issue on Diatom NanotechnologyJ Nanosci Nanotech2005514
- NortonTAMelkonianMAndersenRAAlgal biodiversityPhycologia199635308326
- WichardTPouletSAHalsband-LenkCSurvey of the chemical defense potential of diatoms: screening of fifty one species for alpha, beta, gamma, delta-unsaturated aldehydesJ Chem Ecol20053194995816124261
- SumperFHamppMNanostructure of diatom surface and biomimetic analoguesNano Letter200259195
- WeeKMRogersTNAltanBSEngineering and medical applications of diatomsJ Nanosci Nanotech200558891
- SoltaniSSaidiSSardariSSimulation of silicon nanostructures for the study of natural products in drug discoveryIPSC 2005: Proceedings of the 10th Iranian pharmaceutical science conferenceSep 10–13Iran Tehran2005O49
- HammCEMerkelRSpringerOArchitecture and material properties of diatom shells provide effective mechanical protectionNature200342184184312594512
- TaskinenJYliruusiJPrediction of physicochemical properties based on neural network modelingAdv Drug Deliv Rev2003551163118312954197
- BehlCRPimplaskarHKSilenoAPEffects of physicochemical properties and other factors on systemic nasal drug deliveryAdv Drug Deliv Rev1998298911610837582
- BallardBENelsonJPhysicochemical properties of drugs that control absorption rate after subcutaneousPharmacol Exp Ther1962135120127
- MiyajimaMKoshikaAOkadaJThe effects of drug physico-chemical properties on release from copoly (lactic/glycolic acid) matrixInt J pharmaceutics1998169255263
- SardariSDezfulianMEvaluation of SAR for amphotericin B derivatives by artificial neural networkTrop J Pharma Res20054517521
- TuchmanMLTheriotECStoermerEFEffects of low level salinity concentrations on the growth of Cyclotella meneghinianaKütz Arch Protistenk1984128319326
- LopezPJGautierCLivageJCoradinTMimicking biogenic silica nanostructures formationCurr Nanosci200517181
- Memisoglu-BlinsoyEMuratSHincalAEffect of drug physicochemical properties on in vitro characteristics of amphiphilic cyclodextrin nanospheres and nanocapsulesJ Microencapsulation200623596816830977